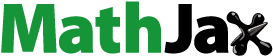
ABSTRACT
Farming is more than just a job of Ethiopia’s smallholder farmers, who live in a low productivity environment. This study employed stochastic frontier approach agricultural efficiency in Ethiopia. Furthermore, Tobit model results show that loss due to wild animals had a negative impact on technical efficiency, while slope of the land and off-farm income had a positive impact. Regarding to the determinants of allocative efficiency, uses of mobile have positive impacts and the off-/non-farm income have negative impacts. In terms of economic efficiency determinants, land slope has a positive influence, while loss due to wild animals and off-farm income have a negative influence. According to the study findings, farmers in the study area kill wild animals, such as monkeys, pigs, and apes, because the loss of wild animals has a high impact on agricultural production during the pre-harvest season. Thus to increase efficiency of farmers and boosting agricultural output, Ethiopian governments policies and strategies should be directed toward providing tourism, construction of soil bunds, tree planting, planting grass, fencing and use of natural fertilizer to maintain the fertility of steep slopes, and supporting farmers by providing network facilities for mobile users that boost farmers’ maize productive efficiency.
Introduction
Globally, the impact of the COVID-19 pandemic on cereal surge in prices contributed to higher food inflation in many countries, especially those adversely affected by the pandemic-driven economic hardship, further reducing access to food (FAO, 2021). Due to increase in demand for food, motivating farmers for sustainable agricultural production and development play an important role in reduction of poverty and food insecurity (Zakari et al. Citation2022). The surge in prices contributed to higher food inflation in many countries, especially those adversely affected by the pandemic-driven economic hardship, further reducing access to food. However, the risk of reverting to poverty and food insecurity remains high, particularly for rural livelihoods that rely on rained agriculture (World Bank Citation2016). Farming techniques in rain-based agricultural country including Ethiopia have largely remained unchanged over the centuries, resulting in low outputs and making farmers vulnerable to the effects of erratic weather patterns (Tefaye and Beshir Citation2014). As a result of inefficient farm production and rising population growth, agriculture has become increasingly stagnant (Nandeeswara and Bealutukela Citation2015). However, in the country, farmers cultivate 1 hectare or less of land, and farming techniques have changed little over the centuries, yielding low outputs and leaving farmers vulnerable to the effects of unpredictable weather patterns (ATA Citation2018). In cognizant challenges over the last decade, the Ethiopian government has increased agricultural productivity by implementing yield-enhancing agricultural technologies. However, it is insufficient to meet the country’s production needs, so the country continues to rely on food aid from western countries (Tura et al. Citation2010; Sorsie et al. Citation2015; Kitila and Alemu Citation2014). Hence, increments of technological-based output capacity and investments research based boost the productive efficiency (Abdulrasheed et al. Citation2021). However, the efficiency difference is due to heterogeneities in conditions, and the diversity of environments in which farmers operate does not have to be unique (Mechri et al. Citation2017). The major food crops are grown in almost every region of the country, with variations in volume due to the amount of land devoted to each crop type, weather change, and a shift in crop preference (CSA Citation2019). Despite the fact that maize is an important food crop in the Gudeya Bila districts of western Ethiopia, its productivity remains below its potential despite efforts to develop and disseminate improved technologies. As a result of food insecurity and an increase in commodity prices, the community may be unable to educate their children; there will be no health improvements, no means of subsistence, and the presence of social conflicts, resulting in severe underdevelopment. In Ethiopia, since social developments are dynamic and geographically diverse. Hence, increase in farming inefficiency affects yield. The depletion of soil fertility, landlessness, climate change, deforestation, and degradation of natural resources are serious problems of farm productivity improvements (Rafael et al., Citation2021). Optimization of input resources for the agricultural production can sustain yield with application of reduced quantity of family labor and size of land (Dagar et al. Citation2021). Moreover, the technological input like synthetic chemical fertilizers has increased the acidity of soil from time to time (Gebissa Citation2021). Thus, it is critical to update current information and knowledge gap on the level and determinants of the smallholder farmer’s technical, allocative, and economic efficiency for maize in the study area is believed to be instrumental for policy design and formulation. Thus, this study investigates efficient technical, allocative, and economic efficiency and identifies efficiency determinants by incorporating new variables such as loss due to wild animals and the use of mobile devices for information transmission between farmers and government agencies that increase productive efficiency.
Review of Literature
The stochastic frontier method allows to decompose growth into changes in input use, changes in technology, and changes in efficiency, and deviations from extreme represent individual inefficiencies (Belotti et al. Citation2013). The stochastic frontier function model is used to utilizes the productive farm isoquant to gauge economic efficiency and to disintegrate this measure into technical efficiency (Sharma et al. Citation2021). SFA approach provides a framework, where production relationship is estimated also as a conditional average (of outputs given inputs and other factors, in the case of production function), but the total deviation from the regression curve is decomposed into two terms – statistical noise and inefficiency (Kumbhakar, Parmeter, and Zelenyuk Citation2020). The stochastic frontier is the most appropriate technique for efficiency studies. This is because of the fact that this technique accounts for measuring inefficiency factors and technical errors occurring during measurement and observation (Coelli Citation1998). So as to take into account effects of these errors, stochastic frontier model was used in this study. Technical inefficiency is related to deviations from the frontier isoquant and allocative inefficiency reflects deviations from the minimum cost input ratios. Thus, economic efficiency is also defined as the capacity of a firm to produce a predetermined quantity of output at minimum cost for a given level of technology (Marta and Katarzyna Citation2020). Government loans and tax breaks likely determine the efficiency that in turn increases businesses production capacities (Muhammad et al. Citation2021).
From the above review, we can conclude that policy implications drawn from some of the above empirical works may not allow in designing area specific policies to be compatible with its socio-economic as well as agro-ecologic conditions(). Also the above researcher neither have nor included the impacts of wild animals, use of mobile phone as determinants of efficiency. Therefore, this study intends to fill these gaps.
Methods
Description of the Study AreaThis study was conducted in western Ethiopia, i Gudeya Bila districts(). The district’s farming system is primarily based on mixed crop-livestock production, in which livestock provides manure for a crop, and crop residue is used as a source of feeding for livestock. Agriculture is distinguished primarily by a rain-fed production system used for livelihood sustenance. Maize is one of the most important cereals grown in the study area, where farming is mostly done by oxen, and there is no mechanized farming system. The district is bounded on the east by the districts of Jima Ganeti and BakoTibe, on the west by the districts of Guto Gida and Sibu Sire, and on the north by the district of Abe Dongoro district, and on the south by Gobbuu Sayyoo district. It is 104 kilometers from the zonal capital and 274 kilometers from Addis Ababa, Ethiopia’s capital. It is situated between latitudes 370 01’ 28” N and 90 17ʹ23” The elevation varies between 500 and 3500 meters above sea level (GBWOANR Citation2020). Because this study involves human participants, the researcher obtained permission from the Gudeya Bila office of agriculture to collect data from sample households; however, due to the political situation at the time of data collection, consent was verbal.
Sampling Techniques and Questionaries’ Design
Following that, multi-stage sampling techniques were used in this study. Gudeya Bila was chosen in the first stage from western Ethiopia, because maize was the dominant crop in the district and the researcher was close to the study area. In the second stage, three Kebeles were purposefully chosen from the district, namely Zangi, Gute Chancho, and Abay Dale, due to maize being the dominant food crop grown and the option for livelihood sustenance in the study area. In the third stage, simple random sampling was used to select 60, 24, and 70 sample households from three kebeles. Finally, using a probability proportional to sample size method, 154 maize-producing households were chosen. A cross-sectional survey with a structured questionnaire distributed to the sample households was used in this study to collect amounts of output produced, inputs used, socioeconomic, demographic, institutional, farm-specific characteristics, and input costs, such as labor cost, land rental cost, oxen cost, and seed and fertilizer cost. Additionally to collect qualitative information, focus group discussion was employed.
Model Specification for Efficiency and Its Determinants
A stochastic frontier model was used to estimate the parameters of the production function as well as the level of efficiency. This is due to the fact that this technique takes into account inefficiency factors as well as technical errors that occur during measurement and observation (Coelli, Rao, and Battese Citation1998). The stochastic frontier model was used in this study to account for the effects of these errors. For this study, the stochastic frontier model defined below was used, as proposed by Aigner, Lovell, and Schmidt (Citation1977) and Meeusen and van Den Broeck (Citation1977)
where Yi measures the quantity of output of the ith farm, Xi is the vectors of explanatory variables used by the farmer ith. The is the vector of unknown parameters. The functional specification
is Cobb–Douglas production function. The disturbance term
is intended to capture the effects of the stochastic noise and it is assumed to be
~N (0, δ2). The disturbance, ui, captures the technical inefficiencies.
Technical efficiency was calculated from Cobb–Douglas frontier model from above equation. Technical efficiency levels are calculated in this study by comparing observed output (Yi) of food maize to the corresponding frontier output (Yi*) using available technology (Sharma, Leung, and Zalleski Citation1999)
In general, the estimation of cost efficiency using stochastic frontier requires information on input prices and output quantities. Assuming that the production function in Equationequation (1)(1)
(1) is self- dual (e.g. Cobb–Douglas), the dual cost function of the Cobb–Douglas production function can be specified as: the cost frontier function is also specified as
The farm specific economic efficiency is calculated as the ratio of the minimum observed total production cost (C*) to the actual total production cost (C) from the above dual cost
Following Farrell (Citation1957), the allocative efficiency index can be derived as the ratio of economic efficiency to technical efficiency
After estimating the level of technical, allocative, and economic efficiency from the stochastic production frontier, it was regressed on farm-specific independent variables that showed disparity in efficiency across farms using a censored Tobit model. The reason why we used Tobit model is the efficiency score is bounded between 1 and 0. Tobit regression (Gujarati Citation2004) is defined as follows:
where latent variable represents the technical, allocative, and economic efficiency scores of maize producers in the study area obtained from stochastic production frontier and dual cost methods.
a vector of the unknown parameters to be estimated.
a vector of explanatory variables m(m=1, 2, … n) for maize producers such as
X1 = loss due to wild animals such as apes, pigs, and monkeys during the pre-harvest stage
X2 = education levels of household in the year of schooling
x3 = family size in man equivalent
x4 = sex of household in dummy (1 if male zero if female heeded household)
x5 = farm size in hectare
x6 = livestock holding in tropical livestock unit
X7 = livestock in tropical livestock unit
X8 = slope of land measured in dummy (1 if flat and 0 if steep)
X9 = fertility which is either fertile or infertile
X10 = distance to plot in minute
X11 = land conservation which is one for a plot that is conserved zero otherwise
X12 = plowing frequency in the number
X13 = distance to the markets market in a minute
X14 = extension contacts in the frequency of contacts during the production season
X15 = use of radio measured dummy (1 if farmers use radio and 0 if not)
X16 = uses of mobile measured as a dummy (1 if farmers used mobile and 0 if not)
X17 = credit uses measured in dummy (1 if farmers received credit and 0 if not)
X18 = off-farm income dummy (1 if farmers receive in off-farm activities and 0 if not)
error term that is independently and normally distributed with zero mean and variance
Denoting Ei as observed variables,
Adopting following McDonald and Moffitt (Citation1980) from the likelihood function, decomposition of marginal effects’ two-limit Tobit model is:
The unconditional expected value of the dependent variable
The expected value of the dependent variable is conditional upon being between the limits
The probability of being between the limits
where, φ(.) = the cumulative normal distribution,
∅(.) = the normal density function
ZL = and Zu = are standardized variables that came from the likelihood function given the limits of y* and σ = standard deviation of the model.
Empirical Results and Discussion
Estimation Maximum Likelihood Estimate
displays the best-fit maximum likelihood estimates of the parameters of the frontier production functions. Three variables (seed, land, and labor) had a significant effect on maize productivity out of a total of five variables considered in the stochastic production function. As a result, a 1% increase in land yields a 0.34% increase in maize production, assuming all other factors remain constant. The scale coefficient was determined to be 1.031, indicating that the returns to scale are increasing (). This implies that maize producers have the potential to increase their output, because they are in stage I of the production surface, where resource use and output are thought to be underutilized. The diagnostic statistics of inefficiency component reveals that sigma squared () 0.499 was statistically significant at 1%. This indicates goodness of fit, and the correctness of the distributional form assumed for the composite error term. The ratio of the standard error of
to standard error
known as lambda (
) was 4.929. Depending on the value of lambda gamma value is derived using the formula
, the gamma (γ) was 96.04%. It also shows that about 96.04% of the variations in output of maize farmers are caused by technical inefficiency. The remaining 3.06% variation was due to random noise that is beyond the control of the farmers.
Table 1. Review on determinants of efficiency differential.
Table 2. Results of Cobb–Douglas stochastic frontier production function.
Table 3. Technical, allocative, and economic efficiency score.
The dual cost function derived analytically from the stochastic production function is given as follows, which is the basis for computing allocative and economic efficiency
where is the cost of producing maize;
refers to the price of seed;
is the cost of land;
is the cost of NPS;
is the cost of UREA;
is the cost of oxen;
is the cost of labor and
is output adjusted for any statistical noise; ith refers to the ith sample household.
Kernel Density Estimate for Technical, Allocative, and Economic Efficiency
Kernel density function measures variations in inefficiency of individual farmers. In addition, a test for normality technical, allocative, and economic efficiency was also made using Kernel density estimate. Kernel density estimate graph resembles the normally distributed curve ().
Technical, Allocative, and Economic Efficiency Score
According to the results of the frontier model, farmers in the study area were relatively good in terms of allocative efficiency than in terms of technical and economic efficiency of maize production in the district’s low land. The mean technical efficiency level was 63.86%, ranging from 4.04% to 94.44%, indicating that maize-producing farmers have the opportunity to efficiently utilize resources and thus increase current maize output by 36.14% using existing technology. The average allocative efficiency of farmers in the study area was 68.41%, with ranges ranging from 37.73% to 88.5%, indicating that maize producer farmers can save 31.59% of their current input costs if resources are used efficiently. The mean economic efficiency level of sample households was 43.65%, as shown in , with minimum and maximum efficiency scores of 1.78% and 79.79%, respectively. This demonstrates that a producer with an average level of economic efficiency could reduce the current average cost of production by 56.35% in order to achieve the potential minimum cost level without reducing output levels (). This analysis yielded a technical efficiency result that is higher than the result of Gosa and Jema (Citation2016). Gosa and Jema (Citation2016) discovered that allocating efficiency is below finding.
Distribution of Technical, Allocative, and Economic Efficiency
In terms of efficiency distribution, 75.9%, 91.55%, and 64.28% of respondents have a technical, allocative, and economic efficiency score greater than 50%, while 24.02%, 8.44%, and 35.71% of respondents have a technical, allocative, and economic efficiency score less than 50% (). This result shows that the farmers in the study area are relatively efficient more than half.
Determinants of Technical, Allocative, and Economic Efficiency of Maize and Its Marginal Effects
In the study area, most losses are caused by wild animals, such as apes, monkeys, and pigs, consuming the maize crop during the day and night during the pre-harvest stage. As a result, farmers find it difficult to control such wild animals, even if they devote more time to doing so during that time. The coefficient of maize crop loss due to consumption by wild animals such as apes, monkeys, and pigs for technical efficiency and economic efficiency is negative and statistically significant at 5% significance levels, respectively. This is because farmers spend more time protecting wild animals from crops than they do on crop weeding, which increases crop yields. These marginal effect results show that farmers are more likely to be technically and economically efficient of 0.3% and 0.019%, respectively, with improvements in mean technical and economic efficiency of 0.4% and 0.3%, respectively, and an overall increase in technical and economic efficiency of 0.5% and 0.4%.
At the 5% and 10% level of significance, the results also showed that using mobile had a positive sign and statistically significant effect on allocative efficiency and economic efficiency (). This implies that mobile-enabled households have higher levels of allocative efficiency on average. This is because the use of mobile phones allows a household to better understand production methods and information, because Ethiopian telecom distributes production, price, and rainfall information to farms via telephone. This outcome corresponds to Sisay et al. (Citation2015). The marginal effect results in a 1.3% and 0.94% increase in the probability of farmers being allocatively and economically efficient, a 1.7% and 2.3% increase in mean allocative and economic efficiency, and a 3.8% and 1.6% increase in overall probability and mean efficiency.
Table 4. Determinants of technical efficiency and its marginal effects.
Table 5. Tobit model estimates for a determinant of allocative efficiency and its marginal effects.
At the 1% level of significance, the slope of land had a positive effect on technical and economic efficiency. This means that farmers who plot maize on steep slopes are more efficient than farmers who plot on flat slopes. This could be because flat slopes are less prone to soil erosion and fertilizers are not displaced from their sown location. This result was consistent with the findings of Mustefa (Citation2017); Kitila and Alemu (Citation2014). Furthermore, the results of the Tobit model’s marginal effect show that the probability of farmers being technically and economically efficient due to the slope of the land being flat is 1% and 0.095%, and the mean technical and economic efficiency due to the slope of the land being flat is 1.5% and 1.3%, with an overall increase in probability and efficiency due to the slope of the land being flat being 1.8% and 3.9%.
The coefficient for livestock holding was positive and had a significant influence on allocative and economic efficiency at 10% level(). The result reveals that having largest number of livestock holding helps to shift cash constraint, provide manure, and to satisfy all needs of farmers in the study area. Each unit increase in the value of livestock holding would increase the probability of a farmer being allocatively and economically by 0.2% and 0.019% and the expected value of allocative and economic efficiency of about 0.3% and 0.4%, with an overall increase in the probability and the level of efficiencies of 0.4% and 0.5%.
Table 6. Tobit model estimates for the determinant of economic efficiency and its marginal effects.
In this study, the coefficient of off-farm income was statistically significant at 5%, with the level of significant effect relating to technical, allocative, and economic efficiency all together. Off-farm activities may have a negative impact on efficiency, because farmers who engage in off-farm activities, such as petty trade, handcraft, and carpentry, may shift to off-farm activities. The outcome is consistent with the findings of Kifle (Citation2017); Gizachew (Citation2018). According to the Tobit model, the probability of farmers increasing their technical, allocative, and economic efficiency as a result of off-farm income was 1.5%, 1%, and 0.13%, the mean increase in technical, allocative, and economic efficiency as a result of off-farm income was 2.8%, 1.8%, and 2.4%, and the overall increase in probability and mean efficiency was 3.3%, 2.2%, and 6.8%.
The sign of education was negative effect on technical and economic efficiency. This implies that more educated farmers are less technically and economically efficient than those who have relatively less education(). This could be because educated farmers have shift from farming to trade or other managerial activities rather than farming. Moreover, an 1-year increase in educational level of the household head decreases the probability of the farmer being technically and economically efficient of 1% and 0.06% and change in the expected value of technical and economic efficiency of 0.17% and 0.15% with an overall increase in the probability and levels of TE and EE of 0.19% and 0.15%, respectively. This result is consistent with finding of Isyanto, Sudrajat, and Yusuf (Citation2021).
Family size had a negative impact on economic efficiency(). This suggested that small family size was more efficient than their larger counterparts. This is due to the reason that the large family size consumes most of the agricultural product and difficult to manage their household expense in addition to agricultural expense. The marginal effect after Tobit model shows that the probability of farmers being technically and economically efficient due to decrease in household family size was 0.3% and 0.06%, the means technical and economic efficiency increased by 0.5% and 1.5% due to decrease in household experience, and the overall increase in probability and mean technical and economic efficiency decrease by 0.5% and 1.5% (Berhanu et al. Citation2021).
Managerial Implication
As information obtained from qualitative information, there is problem of maize productivity efficiency. Thus, to solve this issues, different departments of the country can act community problems. Community management is not the task of a single position but needs to be accomplished jointly by employees of different departments and hierarchical levels. First one is that there are three developmental agents responsible for disseminating the information and providing assistance for techniques of production. The upstanding knowledge of those developmental agents is crucial by government organization and hiring the second is the kebele leader who is responsible for providing the administration. As information obtained the focus group discussion, the political instability is the main problem that decreases the productive efficiency, so the kebele leader has to work with the higher governments officials to stabilize the politics in the country.
Conclusion
Food production in developing countries can be increased by improving efficiency and addressing the issue of production efficiency, as the use of improved technologies is prohibitively expensive, because farmers are subsistence farmers. Since monkeys, pigs, and apes have a negative impact on maize production in the study area. These have two consequences: crop yield loss in one direction and species extinction in the other. Thus, in order to protect those wild animals from extinction and the loss of agricultural products, agricultural policies and strategies that provide a controlling mechanism for those animals through tourism are critical. The Ethiopian government must provide educational opportunities for people living in remote areas to learn how to use mobile phones, as well as low-cost mobile phones for agricultural information transformation and the construction of network facilities for rural communities. Furthermore, improving land productivity status by implementing new soil conservation practices on their farm through improved sustainable land management practices, particularly planting grass and soil bund, is encouraged, particularly on steep slopes where soil erosion is a risk. Finally, additional research on the effects of environmental degradation on agricultural efficiency, as well as a comparative analysis of agricultural efficiency in different agroecologies of the study area in particular and Ethiopia in general, is required.
Additionally, further research has to done for efficiency of smallholder farmers on off-/non-farm activities and integration of crop livestock efficiency.Further research can be done on sustainable land management’s efficiency and risk adjusted efficiency. The main challenge faced during data collection was fear of the respondents to answer the question because of the political situation in the country and the existence of COVID 19.Even though there is no good situation, the researchers overcome the limitation by smooth relationships and close supervision during data collection.This research is based on cross-sectional data but does not show inter-temporal differences in efficiency levels of households. So further research has to be done by time-varying stochastic frontier model by applying panel data collection across the different time intervals to assess the farmers’ technical, allocative, and economic efficiency increase or decrease over the time period. This study is limited to the application of frontier model on economic efficiency only. So further application of stochastic frontier on profit and risk on agricultural productivities has to be done.
It is clearly fact that for resource poor farmers such as Ethiopia, efficient utilization of resource is a major means to obtain maximum output. So it is through stochastic frontier model to know the proper allocation of resource and minimizing input usage to achieve maximum output, thus the governments have to trains extension agents who are specialized on techniques of farm managements who know frontier model on proper allocation of resources.
Abbreviation
ATA = Agricultural Transformation Agency, CSA = Central Statistical Agency, TE = technical efficiency, AE = allocative efficiency, EE = economic efficiency, GBWOANR = Gudeya Bila Woreda office of Agriculture and Natural Resource.
Ethics approval and consent to participate: not applicable.
Consent for publication: I agree to publish this work.
Competing interests: Author has no conflict of interest.
Availability of data and material: The author wants to declare that he can submit the data at whatever time based on your request. The datasets used and/or analyzed during the current study will be available from the corresponding author on reasonable request.
Authors’ contributions: Authors have collected data from sample respondent, analyzed interpreted and wrote the article.
Funding: No fund is received.
Acknowledgments: We gratefully acknowledge Wollega University and Gudeya bila office of agriculture for their support during data collection.
Authors’ information: Authors’ information (optional): Author was from Ethiopia. Author was graduate by Bachelor of Science in agricultural resource economics and management from Wollega University and won the gold medal in 2015 and studied Msc in Agricultural economics from Jimma University. Currently served as lecturer in Wollega University for 4 years and engaged in research activities.
Acknowledgments
We gratefully acknowledge the Gudeya bila office of agriculture for their support during data collection.
References
- Agricultural Transformation Agency.(2018). Woreda-Level crop production rankings in Ethiopia: A pooled data approach. International Food Policy Research Institute (IFPRI) Agricultural annual report of Ethiopia.
- Aicha, M., P. Lys, and F. Cachia.(2017). Productivity and efficiency measurement in agriculture. Technical Report Series.
- Aigner, D., C. K. Lovell, and P. Schmidt. 1977. Formulation and estimation of stochastic frontier production function models. Journal of Econometrics 6 (1):21–2803. doi:10.1016/0304-4076(77)90052-5.
- Alemu, G., and J. Haji. 2016. Economic efficiency of sorghum production for smallholder farmers in Eastern Ethiopia: The case of Habro district. Journal of Economics and Sustainable Development 7:15.
- Ali, Q., M. Ashfaq, and M. Tariq Iqbal Khan. 2016. Analysis of off-season cucumber production efficiency in Punjab: A DEA approach. Journal of Experimental Biology and Agricultural Sciences 4 (6):2320–8694. doi:10.18006/2016.4(Issue6).653.661.
- Bati, M., M. Tilahun, and R. Kumar Parabathina. 2017. Economic efficiency in maize production in Ilu Ababor zone, Ethiopia. Research Journal of Agriculture and Forestry Sciences 5 (12):1–8.
- Belotti, F., S. Daidone, G. Ilardi, and V. Atella. 2013. Stochastic frontier analysis using Stata. The Stata Journal 13 (4):719–58. doi:10.1177/1536867X1301300404.
- Birhanu, F. Z., A. S. Tsehay, and D. A. Bimerew. 2021. Heterogeneous effects of improving technical efficiency on household multidimensional poverty: Evidence from rural Ethiopia. Heliyon 7 (12):e08613. doi:10.1016/j.heliyon.2021.e08613.
- Coelli, T., D. S. P. Rao, and G. E. Battese. 1998. An introduction to efficiency effects in stochastic frontier function for panel data. Empirical Economics 20:325–32.
- CSA(Central Statistical Agency).(2019). The federal democratic republic of Ethiopia central statistical agency agricultural sample survey 2019/20. Volume I report on area and production of major crops. Addis Ababa, Ethiopia
- Dagar, V., M. K. Khan, R. Alvarado, M. Usman, A. Zakari, A. Rehman, M. Murshed, and B. Tillaguango. 2021. Variations in technical efficiency of farmers with distinct land size across agro-climatic zones: Evidence from India. Journal of Cleaner Production 315:128109. doi:10.1016/j.jclepro.2021.128109.
- Debebe, S., J. Haji, D. Goshu, and A.-K. Edriss. 2015. Technical, allocative, and economic efficiency among smallholder maize farmers in Southwestern Ethiopia: Parametric approach. Journal of Development and Agricultural Economics 7 (8):283–92.
- Degefa, K., Jaleta, M., Legesse, B. 2017. Economic efficiency of smallholder farmers in maize production in Bako Tibe District. Ethiopia, Developing Country Studies. Vol.7, No. 2.
- Deme, S., N. Matthews, and J. Henning. (2015). Analysis of factors affecting technical efficiency of smallholder maize farmers in Ethiopia, Conference Paper.
- Fantu, N., G. Berhane, S. Asrat, G. Getachew, A. S. Taffesse, and J. Hoddinott. 2011. Sources of inefficiency and growth in agricultural output in subsistence agriculture: A stochastic frontier analysis http://www. ifpri. org/sites/default/files/publications/esspwp19. pdf .
- Farrell, M.J., 1957. The measurement of productive efficiency. Journal of the Royal Statistical Society: Series A (General), 120(3): 253–281.
- GBWOANR (Gudeya Bila Woreda office of Agriculture and Natural Resource).(2020). Gudeya Bila Woreda office of agriculture and natural resource, the annual report. Nekemte, Ethiopia.
- Gizachew, F. 2018. Allocative efficiency of smallholder wheat producers in Damot. Journal of Food Science and Quality Management 72:27–35
- Gujarati, D. 2004. Basic Econometrics. Tokyo: McGraw-Hill Companies.
- Guth, M., and K. Smędzik-Ambroży. 2020. Economic resources versus the efficiency of different types of agricultural production in regions of the European Union. Economic Research-Ekonomska Istraživanja 33 (1):1036–51. doi:10.1080/1331677X.2019.1585270.
- Isyanto, A. Y., S. Sudrajat, and M. N. Yusuf (2021). Determinants of technical in-efficiencies in swamp rice farming-Ciamis district, Indonesia. In E3S Web of Conferences EDP (Vol. 226, p. 00005). EDP Sciences.
- Kamran Khan, M., S. Fahim Babar, B. Oryani, V. Dagar, A. Rehman, A. Zakari, and M. Owais Khan. 2021. Role of financial development, environmental-related technologies, research and development, energy intensity, natural resource depletion, and temperature in sustainable environment in Canada. Environmental Science and Pollution Research 29: 11356–021-15421.
- Kitila, G. M., and B. A. Alemu. 2014. Analysis of technical efficiency of small holder maize growing farmers of Horo Guduru Wollega Zone, Ethiopia: A stochastic frontier approach. Science, Technology and Arts Research Journal 3 (3):204–12. doi:10.4314/star.v3i3.33.
- Kumbhakar, S. C., C. F. Parmeter, and V. Zelenyuk. 2020. Stochastic frontier analysis: Foundations and advances I. Handbook of Production Economics 1: 1–40.
- Lemessa, S. D., M. A. Yismawu, M. D. Daksa, and M. D. Watabaji. 2017. Risk adjusted production efficiency of maize farmers in Ethiopia: Implication for improved maize varieties adoption. Turkish Journal of Agriculture-Food Science and Technology 5 (9):1099–107. doi:10.24925/turjaf.v5i9.1099-1107.1339.
- Mcdonald, J. F., and R. A. Moffitt. 1980. The use of Tobit Analysis. Review of Economics and Statistics 62 (2):318–21. doi:10.2307/1924766.
- Meeusen, W., and J. van Den Broeck. 1977. Efficiency estimation from Cobb-Douglas production functions with composed error. International Economic Review 1: 435–44.
- Meja, M. F., B. A. Alemu, and M. Shete. 2021. Total factor productivity of major crops in Southern Ethiopia: A dis-aggregated analysis of the growth components. Sustainability 13 (6):3388. doi:10.3390/su13063388.
- Park, D.-Y, K Choi, and D.-H. Kang. 2020. Measuring the meta efficiency and its determinants on efficiency in the Korean coffee shop franchise. 12:2398. doi:10.3390/su12062398.
- Rafael, Alvarado, Brayan Tillaguango , Vishal Dagar , Munir Ahmad , Cem Is¸k, Priscila M´endez , Elisa Toledo h. 2021. Ecological footprint, economic complexity and natural resources rents in Latin America: Empirical evidence using quantile regressions, Journal of Cleaner Production (318): 128585.
- Rao P, N., and Bealutukela. 2015. Analyzing productivity in maize production: The case of Boricha Woreda in Sidama zone, southern Ethiopia. International Journal of Recent Scientific Research 6 (10):6984–89.
- Sharma, U., P. Chetri, S. Minocha, A. Roy, T. Holker, A. Patt, and J. Joerin. 2021. Do phone-based short message services improve the uptake of agri-met advice by farmers? A case study in Haryana, India. Climate Risk Management 33. doi:10.1016/j.crm.2021.100321.
- Sharma, K. R., P. Leung, and H. M. Zalleski. 1999. The technical, allocative, and economic efficiencies in swine production in Hawaii: A comparison of parametric and non-parametric approaches. Agricultural Economics 20:23–35. doi:10.1111/j.1574-0862.1999.tb00548.x.
- Tefaye, W., and H. Beshir. 2014. Determinants of technical efficiency in maize production: The case of smallholder farmers in Dhidhessa District of Illuababora Zone, Ethiopia. Journal of Economics and Sustainable Development 5 (12):274–84.
- Tura, M., Aredo, D., Tsegaye, T., La Rovere, R., Kassie, G.T., Mwangi, W.M. and Mwabu, G., 2010. Adoption and continued use of improved maize seeds: Case study of Central Ethiopia.
- World Bank. 2016. Federal democratic republic of Ethiopia priorities for ending extreme poverty and promoting shared prosperity systematic country diagnostic , USA: world bank.
- Yigezu Wendimu, G. 2021. The challenges and prospects of Ethiopian agriculture. Cogent Food & Agriculture 7 (1):1923619. doi:10.1080/23311932.2021.1923619.
- Zakari A, A., J. Toplak, M. Ibtissem, V. Dagar, and M. Kamran Khan. 2021. Impact of Nigeria’s industrial sector on level of inefficiency for energy consumption: Fisher ideal index decomposition analysis. Heliyon 7 (2021):e06952. doi:10.1016/j.heliyon.2021.e06952.
- Zakari, A., I. Khan, D. Tan, R. Alvarado, and V. Dagar. 2022. Energy efficiency and sustainable development goals (SDGs). Energy 239:122365. doi:10.1016/j.energy.2021.122365