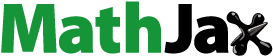
ABSTRACT
Many countries including Ukraine use battle management systems (BMS) like Delta that enable command to share situation awareness information; this study focuses on the distribution of information across a warfighting network. Similar to natural systems, where autonomous agents, such as ants and bees, follow a set of simple rules, a BMS is a network of bases and electronic warfighting platforms that have military assets as agents within the network, guided by the defense doctrine. The rationale for the workability of such a system is based on each subsystem being reliable when multiple subsystems interact. However, the potential permutations and combinations of interactions can cause unpredictable negative or positive feedback loops, resulting in unpredictable and unwanted outcomes. The results of emergent behavior are unexpected and sometimes unwanted in areas such as intelligence, and wireless networks. Understanding emergent behavior is imperative in understanding complex engineering systems, and to present new insights, and take practical steps toward improving complex systems design and analysis. This paper presents the BMS and networks with examples of user-defined system integration of the network soldier concept. We believe that Ukrainian and other armies can directly benefit from utilising meta cybernetics, meta metasystem model analysis to control emergence.
Introduction
Modern digital armies are centered on sharing relevant situational awareness information within and between dismounted teams (soldiers) and beyond to other levels of command (headquarters or HQ) and flanking elements (mobile platforms and other assets). Previously, all communication and information were analogue and relatively inefficient in the theater of war. In the current wartime situation in Ukraine and the wide usage of IT supported battlefield systems, the chosen topic is very important to the Ukrainians and western world.
The structure of the paper is the following: First, introduce the battle management systems (BMS) which focus on distributing information across a warfighting network and is basically explained. Secondly, the meta systems, meta methodology, meta cybernetics and emergent behavior in systems are explained and integrated in detailed diagrams presented. Next the state space framework and the polynomial nonlinear state space model are introduced. Finally, the proposed method is recommended for the BMS meta systems by meta cybernetics to control emergence.
Today, battle management systems (BMS) focus on distributing information across a warfighting network. BMS are a network of bases and electronic warfighting platforms. Military conflicts, especially those involving land combat forces, have recently grown rapidly (Chen et al. Citation2014). The rise of automation in many systems and technologies presents complex operational environments that require a high level of collaborative, complex adaptive systems of systems (CASoS) solutions.
One example is the Delta real-time battle management system as part of the large-scale event Tide Spirit of the North Atlantic Treaty Organization (NATO). The Delta real-time battle management system (DBMS), which is designed to address an army’s transformation from analogue to digital capabilities and provide military advantage in intelligent warfare situation awareness. DBMS provides a comprehensive understanding of the battlefield in real time, integrates information about the enemy from various sensors and sources, including intelligence on a digital map. These operations require agile systems of systems (SoS) that must be continually updated to meet the challenging pressures of the operational war environment. Ukrainian army and many others the major problem is the shortfall of operational control of units and to instantly relay information on enemy forces movement to other units and headquarters and includes friendly fire where soldiers are left vulnerable. This is explained in this paper DBMS and network soldier system and meta non linier model to solve problems.
In understand the non-linear model which is compared with other system representations, several examples are introduced, and the results are extended to create prediction error input-output models for multivariable non-linear stochastic systems. The graph theory is an important area in mathematics. A network is a graph-based representation representing a problem as a graph to provide a different point of view on the problem. A problem is much simpler when represented as a graph since it can provide the appropriate tools for solving it. Hence, a graph or network acts as an excellent modeling tool in representing several fundamental issues in the network, such as connectivity, routing, data gathering, mobility, topology control, traffic analysis, finding the shortest path and load balancing.
In mathematics the Lanchester (Citation1999) presented a collection of joined ordinary differential equations known as the Lanchester equations (LEs); the roots of the LEs are process models for reducing strength or effectiveness in modern warfare (Engel and Gass Citation2001). They are a collection of differential equations describing the time dependence of the strengths of two armies, A (green force) and B (red force), as a function of time, c2n22 = c1n. Thus, the fighting strengths of both forces are equal when the products of the squares of the numerical strengths times the coefficients of effectiveness are equal (Chen et al. Citation2011). Osipov and Maksimov (Citation2018) independently devised a series of differential equations known as Lanchester’s Square Law (Engel and Gass Citation2001) to demonstrate the power relationships between opposing forces. With the design and development of BMS complex systems, understanding differential equations is important. The Lotka-Volterra equations (Lanchester Citation1999) are used to model the dynamics of interacting “predator-prey populations” (Washburn et al. Citation2016).
An older example is the battle of Iwo Jima, where (US) and
(Japanese) are the number of troops on the island, and r(t) is the rate at which the US troops landed (Rawson Citation2012). Experimenting with the model with different values of the parameters α and β or different reinforcement schedules would have resulted in different outcomes (Chen et al. Citation2014). The parameters α and β comprise units of opposing casualties per man per day of combat and were chosen to fit the record of all that happened (Washburn et al. Citation2016). The explanation is that US troops substantially outnumbered their Japanese counterparts during most of the battle.
Diagram 1. Variety Engineering, System Incorporating viable system model (VSM)
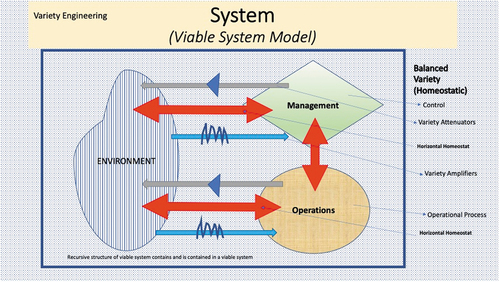
The BMS is focused on the distribution of information across a network. Systems with numerous components are complex, and their intricate interactions are inevitable (Chen et al. Citation2014). Examples include natural systems that range from animal flocks to socio-ecological systems and leading-edge engineering (artificial) systems, such as the internet and social networks. These systems are called complex adaptive systems (CAS) and exhibit behaviors from non-linear spatio-temporal interactions among multiple components and subsystems (Kaisler and Madey Citation2009). These interactions may lead to properties that are often called emergent and cannot be derived from individual components. While numerous attempts have been made to define emergence (Holland Citation2007), consensus has not been reached on a general definition. Some of the most cited works to date that have explored the classification of emergent behaviors are by Singh et al. (Citation2017), Johnson (Citation2016), Holland (Citation2007), and Bar-Yam (Citation2004b). The System Engineering Body of Knowledge (SEBoK; 2020) describes emergent system behavior as a consequence of the interactions and relationships between system elements rather than the behavior of individual elements.
Many authors, such as Singh et al. (Citation2017), Johnson (Citation2016), Holland (Citation2007), Fromm and International Society (2021), and Bar-Yam (Citation2004a), agree that the notion of emergence involves the existence of levels in a system. Therefore, emergence can be summarized as a characteristic of a system. The properties appear at the system (macro) level, are not explicitly implemented, and arise dynamically from the interactions between entities at the component (micro) level (Singh et al. Citation2017). Moreover, using Fromm’s (2021) taxonomy of emergent behaviors, it is considered that the development of a suitable framework should provide a platform for simulating and analyzing behaviors in multi-agent systems (Rainey and Mittal Citation2015) as the taxonomy of different types of emergent behaviors is based on the relationship between these macro and micro levels (O’Toole, Nallur, and Clarke Citation2014).
BMS Behavior Phenomenon
A Theoretical View
Similar to natural systems, where autonomous agents, such as ants and bees, follow a set of simple rules, the system – in this case, a network of bases and electronic warfighting platforms – has military assets as agents within the network that are guided by defense departments (army, navy, and air force). Although each subsystem is reliable, when multiple subsystems interact, the potential permutations and combinations of interactions can cause unpredictable negative and positive feedback loops, resulting in unpredictable and unwanted outcomes (Henshaw Citation2015). BMS Function and Performance Specification (FPS) is developed by defense departments for contractors and provided to define and validate a set of requirements for BMS material systems (Henshaw Citation2015). Interactions that may result in emergent behavior will manifest at the interfaces between systems, between systems and operators, and between systems and BMS agile software development elements. Examples include developing stories/epics/feature designs (SEFDs) and a stable understanding of warfighting operations and strategies during combat (Loerch and Rainey Citation2007). The epic and feature designs are important to the development of BMS software; similarly, it is important to recognize the positive and negative emergent behaviors in software development. The physical result of emergent behavior in the BMS is a goal-seeking element that may have probabilistic, unanticipated behavior.
The results of emergent behavior are unexpected and sometimes unwanted in areas of intelligence, cybersecurity, weapons on target and wireless networks, integrated power hubs, sensors, end-user devices (EUDs), tactical routers, and network-enabled technologies (O’Toole, Nallur, and Clarke Citation2014). During agile software development, positive emergent behaviors are a preferred choice, whereas negative behaviors are unwanted and should be eliminated, if possible. Software developed using agile processes can be analyzed from the perspective of graph theory and based on cognitive science methods.
BMS software in a battlefield environment permits participants to successfully allow network data to be combined and analyzed with more sophisticated algorithms and techniques in the operational environment. Emergent behavior occurs in the communications systems interface, the configuration of the combat network for land dismounted wireless networking, sensors, and systems that include human biosensors, targeting, shot detection, uncrewed aerial vehicles (UAVs), small arms digital sights, range finders, and data.
Diagram 2. BMS central command communication network
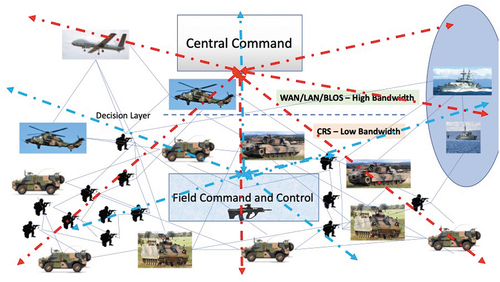
The emergent behavior in BMS is not based on a priori knowledge. The method used to analyze emergence in a real-time warlike hostile environment draws from the perspective of graph theory and cognitive science methods that are applied early in system development. At this stage, knowledge is independent of experience, and it is not easy to clearly recognize, analyze, and validate where the emergent behavior exists. However, agent-based modeling (ABM) and simulation to assess the presence of emergent behavior in BMS may be effective.
Literature Review and Taxonomy of Emergent Behavior
An extensive literature review suggests that the critical foundation to a safe and efficient operational capability is the underlying integrity of meta-systems of emergent behavior occurrence (Genesereth Citation1983).
Diagram 3. Meta System incorporating System VSM
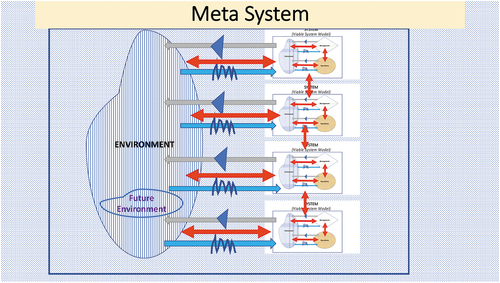
The literature suggests several techniques to detect emergent behavior, ranging from statistical analysis to formal approaches. For the current study, variable-based techniques are the most appropriate choice (Chen et al. Citation2014; Holland Citation2007; O’Toole, Nallur, and Clarke Citation2014). The variable-based approach is used to design, develop, and implement information systems for BMS and many other SoS. Emergent behaviors in the SoS comprise three elements: agents, their interactions, and the environment. Each agent has a set of attributes that describe the state of the agent and several specified policies/rules that define how the agent behaves with respect to the changes in its environment (Lee et al. Citation2018).
Emergent behavior is often seen in computer systems such as the BMS; however, while it can appear in such systems, it is difficult to design. As large-scale behavior results from unpredictable interactions among simple agents, there is no guarantee that any given set of simple agents will exhibit a particular kind of behavior (Singh et al. Citation2017). Emergent behavior often appears in large systems in the form of unexpected results, which are most often classified as “bugs” in the code. An example of this can be found in communication networks. In massively parallel computers, simple properties arising from interactions of simple rules lead to poor performance because of congestion in internal routing networks. However, these resulting bugs may exhibit emergent behavior that can be put to interesting and unexpected uses as in the cases of Y2K, the Dhahran incident, and the Blue Screen of Death (BSOD) that occurred during a live Windows 98 presentation.
A mechanism of indirect coordination of agents that cannot communicate directly with one another but must engage indirectly through a medium is known as Stigmergy. Stigmergy is used to analyze self-organizing activities in various domains, such as robotics, society, and engineering (Adams et al. Citation2014). A network of computers allows the possibility of many kinds of emergent meta-level behaviors because computers interact in highly complex ways (Genesereth Citation1983). The emergent behaviors found in computing contexts can be desirable and intentional (Burbeck Citation2007), or constitute malware, such as computer viruses, botnets, digital propaganda, and cyber-warfare, which are undesirable and problematic.
Gaps in the Literature
Information on systemic thinking and cybernetics and how they provide building blocks of framework elements and methods used in constructing a meta-methodological model is unclear and lacking.
As emergence is a property of the aggregate structures of warfighting systems and cannot be anticipated, to establish a theoretical framework for modeling and simulation, it is necessary to first establish a taxonomy of emergent behaviors, which is currently unclear.
The Evidence is lacking on emergent behavior present in constituent systems that support the systems designs. Combinations of systems operating together within SoS contribute to the overall capabilities. Combining systems can lead to emergent behaviors that may either improve performance or degrade it and may similarly decrease or increase costs.
BMS in Its Application to the Networked Soldier
Scenarios are used to reveal the dynamics of change and use these insights to arrive at sustainable solutions to the challenges at hand. They help stakeholders break through communication barriers and understand how current and alternative development paths may affect the future. The ability to illuminate issues and break impasses makes them extremely effective in opening new horizons, strengthening leadership, and enabling strategic decisions. Therefore, it is reasonable to invite outsiders such as major customers, key suppliers, regulators, consultants, and academics into the process. The aim is to envisage the future broadly in terms of fundamental trends and uncertainties. First, line managers develop basic ideas, and then, staff, such as planners, develop the written versions, fill in the gaps, and find new data. Schwartz’s (Citation2012) meta-system and living system model summarizes most of the knowledge on CAS but, owing to its succinctness, it remains a generic graphic meta-model.
Technological issues can be categorized as direct (e.g., “How will high-bandwidth wireless affect landline telephony?”), enabling (e.g., “Will X-ray lithography bring in the next chip revolution?”), and indirect (e.g., “Will biotech allow easy ‘body hacking’ and compete with more traditional forms of entertainment?”). Listing the driving forces is useful to look past the everyday crises that occupy our minds and examine the long-term forces that ordinarily operate well beyond our concerns. These powerful forces usually catch us unawares. Once these forces are enumerated, we can see that from our perspective, some of them can be considered “predetermined;” this is not exactly a philosophical stance but one describing how they are completely outside our control and will play out in any story we develop about the future. Not all forces are as evident or easy to calculate, but when we build our stories, predetermined elements figure in each one.
Cyber-Physical Systems and Next-Generation BMS in Its Application to the Network Soldier
The ability to interact with and expand the capabilities of the physical world through computation, communication, and control is key to future technological developments. Opportunities and research challenges include the design and development of next-generation airplanes and space vehicles, hybrid gas-electric vehicles, fully autonomous urban driving, and prostheses that allow brain signals to control physical objects. Increased efficiency of information or data flow alone changes the entire organizational construct within which the system operates. Directions for future research in Cyber Physical Systems (CPSs) are as follows:
Standardized abstractions and architectures that permit modular design and the development of CPSs are urgently needed.
CPS applications involve components that interact through a complex, coupled physical environment. Reliability and security pose particular challenges in this context – new frameworks, algorithms, and tools are required.
Future CPSs will require hardware and software components that are highly dependable, reconfigurable, and in many applications, certifiable. Trustworthiness must also extend to the system level.
Designing CPSs is challenging because:
the vast network and information technology environment connected with physical elements involves multiple domains, including controls, communication, analogue and digital physics, and logic;
the interaction with the physical world varies widely based on time and context; and
using multi-domain models that capture such variability is critical to successful CPS design.
Diagram 4. Meta cybernetics and cyber physical system presentation coupled through use of cybernetics orders VSM and control of emergence.
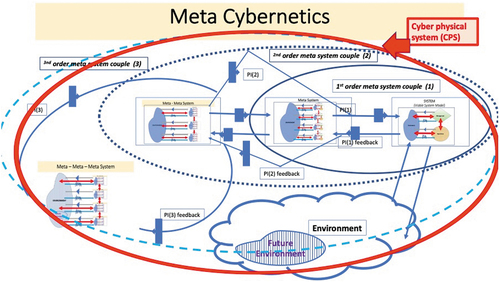
CPSs link cyberspace with the physical world through a network of interrelated elements such as sensors and actuators, robotics, and computational engines. These systems are highly automated, intelligent, and collaborative. Examples of CPSs include energy-neutral buildings, zero-fatality highways, and personalized medical devices. CPSs require detailed modeling of the dynamics of the environment and a clear understanding of the interactions between the dynamics of the embedded system and its environment (Sage and Gass Citation2016).
The networked soldier CPS offers a good scenario for design and analysis because of the integration of BMS, process, computation, and networking, where embedded computers and networks can monitor and control the networked soldier’s behavior, and combat physiological monitoring systems with feedback loops in which the networked soldier’s behavior and actions can affect computation and vice versa. Present-day CPSs integrate computation and physical processes to perform various mission-essential or safety-critical tasks.
Benefits
Wearable sensors for medical purposes (e.g., measuring temperature or heart rate) can be used to identify whether a soldier is in medical distress. In the past, it was not possible to obtain this information remotely unless the soldier radioed in and offered it. With this CPS connected to the BMS and tactical network, the condition can be identified before the soldier may even be aware of it, and an alert may be raised. If the alert is raised on an entire company, the system will “know” that a stressor of some kind is impacting the soldiers and some action is necessary. Data from a networked soldier can be used to simulate different scenarios for test and analysis purposes and identify areas where the safety and security of soldiers as a system or subsystem exist.
Analysis
Analysis is a process of examining possible future events by considering potential alternative outcomes (sometimes called “alternative worlds”). The ideal scenario test is a credible, complex, compelling, and motivating story with an easy-to-evaluate outcome (Henshaw Citation2015). The research method is based on the methodological level in a system design, which applies to communication, control (cybernetics), and system thinking (Sage and Gass Citation2016). The application of cybernetics science in engineering is commonly used to analyze failures and systems accidents where a small error or deviation from the standard operating environment can result in a disaster (Sage and Gass Citation2016).
Smartphone Ad-Hoc Networking (SPAN) Mesh: The Local Network Topology and Future Soldier System’s Physiological Concept Design
Networks are mathematical structures mainly used to describe complex systems like the brain and the internet. Therefore, in fundamental topological, structural and geometrical properties emerge complex geometry. Thus, characterizing the geometrical properties of these networks has become increasingly relevant for routing problems, inference, and data mining. Moreover, the nonequilibrium dynamic rules of these networks will generate scale-free networks with clustering and groups. These geometric networks are present and describe the technological system as well as biological and social. Graph theory works on treatable structures when we examine the difference between a network and a graph. The networks focus on data features like sparsity and inhomogeneities frameworks extension and the use of a classical random graph to a general class of inhomogeneous arbitrary graph model and a general framework for analyzing a large type of model.
Physiological Monitoring
The ability to remotely monitor the physical condition of each soldier in a dismounted unit has become an essential component of the unit’s safety, efficiency, and effectiveness. The physiological monitoring system collects, stores, and transmits physiological data from the soldiers to the commander. The system comprises a set of wearables – minimally invasive sensors that collect data and monitor several parameters of the soldier’s body, such as an electrocardiogram (ECG), a heart rate monitor (HR), and thermometers for core and skin temperatures – and an algorithm to collect, correlate, and distribute the data efficiently.
Diagram 5. Network soldier sensor and communication
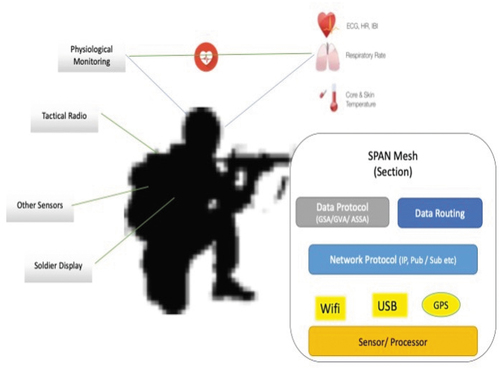
Human-Machine Interface
Significant progress has been made in ensuring that the C4I computer and BMS software suit the needs of a dismounted soldier. Although the system has operational value for mission planning and situational awareness when on the halt, the current solution provides limited means for situational awareness while on the move. Additional technologies and solutions, such as voice control, in-ear earphones, and see-through glasses must be explored to provide a holistic solution that is usable during all phases of the dismounted soldier’s mission. The soldier system must be sufficiently flexible to allow any combination of sensors, processors, user interfaces, and communications at different fitment locations to create an operational outcome.
System Modularity
The future soldier system is required to provide an optimized solution for several soldier roles in various mission types. The system must be modular and configurable to support multiple configurations using the same set of building blocks. Its ability to link soldiers in a section and with the broader army communication landscape is key to delivering the SPAN mesh networks (nodes).
Diagram 6. Basic mesh network (kinetic)
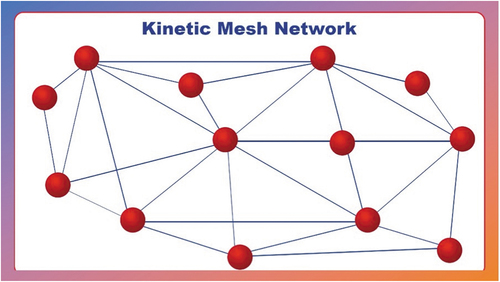
The network needs to allow future support for the increasing range of sensors and field intelligence capabilities. The SPAN solution is an innovative mesh network for sharing data among soldiers in a section and between the command and the section. The mesh network will be built on a standardized technology platform and will support a set of standard data exchanges based on the generic vehicle (GVA) and soldier (GSA) architecture models. This will allow the SPAN mesh to provide a network for all sensors.
Diagram 7. Network soldier user interface
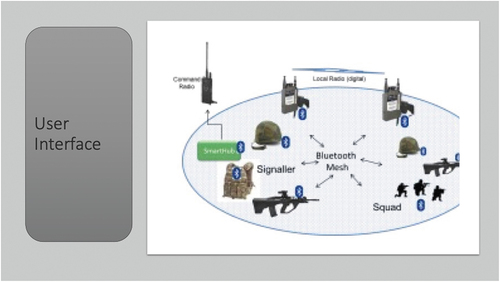
The SPAN mesh at the soldier and section levels will leverage several existing wireless technologies with new and evolving technology to create a low-power mesh network such as through Bluetooth/wi-fi and ultra-wideband (UWB). Creating a data standard over the mesh network will allow any sensor, device, or computer to connect as a node and collect or share data with other nodes in the network. The mesh network’s routing capability would enable data to flow through the entire section. Thus, a dispersed section would still be able to share data through the links between individual soldiers over a significant distance. Due to the low size, weight, and power (SWaP) of these network components, many sensors can be self-contained and will not require a large separate power supply. The SPAN will be integrated with the broader army network by being connected to an existing very high frequency (VHF) network, broadband, and future waveforms. Combining some of the existing radio knowledge with the new SPAN mesh and local higher capacity network will create a link with the army backbone network. A section commander, signaler, or vehicle can all carry the SPAN transceiver and tactical radio to allow this data exchange. With the creation of the SPAN mesh, multiple sensors can be fused to create higher-order information. Connecting sensors via the mesh networks to a processing capability in the BMS will allow combining and analyzing network data with more sophisticated algorithms and techniques. Sensors such as shot and electronic warfare detection and range finders can be combined to create red tracks for sharing across the section and the wider BMS system. To create situational awareness, images and videos from local support can be integrated with ranger finders, BMS, and UAV data.
Cybernetics: “The New Paradigm”
During the Second World War, mathematicians Wiener (Citation1961)developed a new branch of applied science, naming it the science of information feedback systems cybernetics (McCulloch and Foerster Citation1995). Fourth-order cybernetics is called emergent cybernetics and considers what happens when a system redefines itself. It implies that a system will “immerge” into the environment of which it is a part. The axioms or elements of systems theories are the centrality, contextual, goal, operational, viability, design, and information elements. Using cybernetics management (Beer Citation1972), this literature review examines emergent behavior through the theory of critical system thinking (D’Andreamatteo et al. Citation2019) and cybernetics methodology. The cybernetics methodology, called the “new paradigm,” has attracted numerous researchers and practitioners and introduced them to the discipline of systematic management (Sage and Gass Citation2016). Meta-cybernetics represents the higher cybernetic orders in living system agencies (Yolles Citation2021). Agencies are complex and viable and require stability and uncertainty reduction to survive. Meta-cybernetics is defined through a metasystem hierarchy and is mostly known through first- and second-order cybernetics (Yolles Citation2021).
Diagram 8. Meta Cybernetics and coupled from 2nd − 4th order cybernetic.
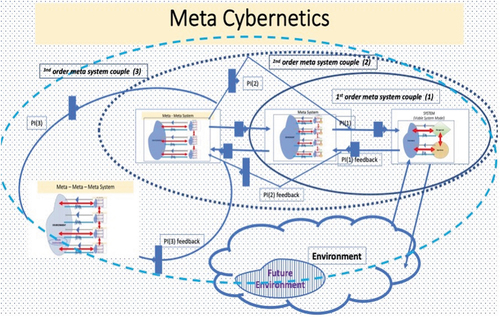
Applying cybernetics management (Beer Citation1984) to complex systems analysis, this paper examines problem solving through the theory of critical system thinking (D’Andreamatteo et al. Citation2019) and cybernetics. Cybernetics began as a questioning of the ideas of systems in and out of control in first- and second-order behaviors. The law of requisite variety makes it clear that control has limits. When Ashby (Citation1965) described first- and second-order effects, he was not thinking of autonomy or intelligent SoS, although he undoubtedly understood the possibilities of emergent behavior. Emergence, as a property of the aggregate systems of warfighting systems, cannot be anticipated (O’Toole, Nallur, and Clarke Citation2014). Simulations employing the same perceptual engines as found in vessels are currently being developed as experiments with different contexts by examining what is expected and unexpected and whether emergent behavior can be forecast within some limits of confidence (O’Toole, Nallur, and Clarke Citation2014).
Cybernetics and System of Systems
Cybernetics and systems science focus on complex systems, such as organisms, ecologies, minds, societies, and machines (Bar-Yam Citation2004b). They regard these systems as complex, multi-dimensional information system networks. Cybernetics presumes that some underlying principles and laws can be used to unify the understanding of such seemingly disparate types of systems (Sage and Gass Citation2016). The characteristics of cybernetic systems directly affect the nature of cybernetic theory, resulting in serious challenges to traditional methods. Some of these characteristics, as identified by Sage and Gass (Citation2016), are:
Complexity: Cybernetic systems are complex structures.
Mutuality: The many components interact in parallel, cooperatively, and in real-time, creating multiple simultaneous interactions among subsystems.
Complementarity: These many simultaneous modes of interaction lead to subsystems that participate in multiple processes and structures.
Evolvability: Cybernetic systems tend to evolve and grow opportunistically.
Constructivity: Cybernetic systems are constructive in that they tend to increase in size and complexity.
Reflexivity: Cybernetic systems are rich in internal and external feedback, both positive and negative.
SoS Agent-Based Modeling and Simulation
ABM and simulation can demonstrate that emergent behavior exists in the BMS. Emergent behavior can be determined using ABM and simulation, or some other applicable modeling and simulation (M&S) tool applied to a given SoS engineering application (Lee et al. Citation2018). Designing a multi-BMS system first requires specifying how each system agent exists and acts in the environment. This is represented in behavioral ontology (Burbeck Citation2007). This description is then transformed and expressed in the language of the simulation engine and provided as input for execution. There is no evidence of the presence of emergent behavior in constituent systems that supports systems design. Combinations of systems operating together within the SoS contribute to the overall capabilities and lead to emergent behaviors, which may improve or degrade performance and decrease or increase costs. In the System Engineering Body of Knowledge, SoS are important for capability objectives and understanding their interrelationships.
MITRE (Citation2021) defines the SoS as a system with characteristics. It comprises a collection of systems, each capable of independent operation, that interoperate together to achieve additional desired capabilities. Maier (Citation1998) defined SoS as operational and managerial independencies. These two independencies have distinguished characteristics in applying the term SoS. Therefore, any system that does not display these two characteristics is not SoS regarding its components’ complexity or geographical distribution. The constituent systems of the SoS will have different owners supporting defense organizational structures beyond the SoS management. The SO/IEC/IEEE 21,839 (ISO/IEC/IEEE 2019) standard defines the SoS and constituent systems as follows:
Systems of Systems (SoS)—Set of systems or system elements that interact to provide a unique capability that none of the constituent systems can accomplish on its own. Note: Systems elements can be necessary to facilitate the interaction of the constituent systems in the SoS.
Constituent Systems—Constituent systems can be part of one or more SoS. Note: Each constituent is a useful system by itself, having its own development, management goals, and resources, but interacts within the SoS to provide the unique capability of the SoS.
Rainey and Jamshidi (Citation2018) shared advice regarding setting a research objective by choosing a given/specific SoS to explore for the presence of emergent behavior to identify it, understand what may constitute both positive and negative emergence, use Monterey Phoenix (MP) (https://wiki.nps.edu/display/MP/Monterey+Phoenix+Home) to remove negative emergence, and ensure that only positive emergence remains. The point, as stated above, is to consider one incident/venue to investigate from which general conclusions can be made that apply across the board/population of SoS (Rainey and Jamshidi Citation2018) This is further explained in Rainey and Jamshidi book, Engineering Emergence: A Modeling and Simulation Approach (Rainey and Jamshidi Citation2018), which describes architecture and modeling in complex systems. For analysis and modeling purposes, Rainey et al, (Citation2015) recommended identifying SoS that require exploration for emergent behavior and explaining why this SoS was chosen for examination from which the conclusions can be drawn for all SoS (Loerch and Rainey Citation2007; Rainey and Jamshidi Citation2018).
In literature during the last decades, there has been a tendency toward nonlinear modeling in various application fields. An excellent starting point for nonlinear modeling is Jonas et al. (Citation1995). However, a significant drawback is the lack of a general nonlinear framework. However, a class of nonlinear systems has intensively been studied and covers a broad spectrum of “nice” nonlinear behavior, namely the class of Wiener systems. This class of systems stems from the Volterra – Wiener theory (Rugh Citation1981; Schetzen Citation1981) and will be employed here as a framework to develop the initialization procedure of the Polynomial Nonlinear State Space (PNLSS) model. The network is a graph-based presentation of a problem (in many cases) and provides a different viewpoint to the analyst. This paper first presents the BMS and networks with examples of user-defined system integration of the network soldier concept. We believe that Ukrainian command and soldiers can directly benefit from integrating meta cybernetics, meta metasystem, and cyber-physical systems (Rainey and Tolk, Citation2015). For the systems of systems agent-based modeling and simulation in nonlinear devices and class systems, we proposed Volterra -Wiener theory, which can be used as a framework to develop the early procedure and initialize the polynomial nonlinear state space model.
In the following paper: Practical Modeling Concepts for Engineering Emergence in Systems of Systems, Giammarco (Citation2018) states that positive emergence is what remains after thoroughly exposing and removing negative emergence and provides a five-step algorithm for executing the same. A dynamic evolutionary meta-model analysis of the vulnerability of complex systems can have severe consequences and is often seen as the core problem of complex systems’ multilayer networks. To understand emergent behavior in SoS, MP facilitates modeling and simulation of systems of systems (SoS) across many application domains and enables exposure and control of associated emergent behaviors. With MP, the presence of emergence in a model of the SoS can be detected (MP also permits the modeling of an SoS) and negative emergence can be deleted such that only positive emergence remains. The upshot/impact of this tool is to preclude potential negative influences on the SoS and lead to potential force multipliers therein. Dr Kristin Giammarco developed the MP modeling tool that can detect the presence of emergence in a model of the SoS.
The key point is that MP provides a means and or capability to model and simulate the SoS. Most importantly, it facilitates the capability to examine for the presence of both positive and negative emergence. In addition, it facilitates the deletion of negative emergence such that only positive emergence remains, integral because negative emergence can potentially be a significant detriment to the SoS’ mission. Thus, it is a force multiplier for the SoS’ mission (Rainey and Jamshidi Citation2018) As a powerful method for CAS modeling, ABM has gained growing popularity among academics and practitioners. ABM demonstrates how the agents’ simple behavioral rules and local interactions at the micro-scale can generate surprisingly complex patterns at the macro-scale.
Architecting Principles of Emergent Behavior
In 2013, Maier described the architecture of SoS to comprise communications and noted their nonphysical nature, constituting a set of standards that allow for meaningful communication among the components (Maier Citation1998). SoS and systems components are configurations of tangible and intangible elements, such as mechanical, electrical, electronic, software, knowledge, and natural objects. These objects perform functions and behaviors to meet a specific purpose and fit within the description of emergent behavior as defined by Maier (Citation1998). The objects serve a purpose in their own right. However, such a system could be considered exosystemic in situations where there are hidden states. That is, the SoS of machines exist that must be designed, manufactured, and operated to deliver their purpose. An example is a communications SoS (such as satellites, land stations, submarine cables, and facilities) that aims to enable household and business transactions, manufacturing, the control of autonomous vehicles in mines, and the management of a battlespace. Within these SoS, their components are systems in their own right. For the systems to meet their purpose, other complex SoS must be in place. The components of this system include elements such as human skills, machine learning, measures of performance, tools, knowledge, and facilities. This system has two main subsystems: social and technical. Whereas the social system describes the functions and behaviors humans apply to a maintenance system, the technical system describes the technology functions and behaviors that deliver the required purpose (Rainey and Jamshidi Citation2018)
Diagram 9. Emergence complexity progress from deterministic to stochastic system
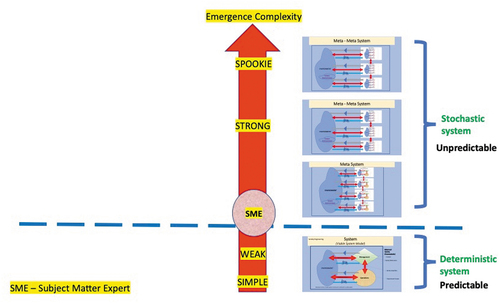
Considering the combination and permutation of systems elements or components, evidently, issues such as their completeness and order must be considered. Polanyi’s statement that “We know more than we can tell (Lundberg Citation1949; Polanyi and Allen Citation1997) is an appropriate description of the situation. Several interacting systems exist, and because of relationships such as sneak circuits, there may be more going on in the systems than we can tell. The total behavior events of the combined systems working alone or collectively must be visible from the strategic requirement of system performance to the implementation of the system to sustain purpose. In the SoS, it is important to identify the critical set of systems that affect the objectives and to understand their interrelationships.
Diagram 10. Meta metasystem, meta cybernetics and emergence complexity incorporated
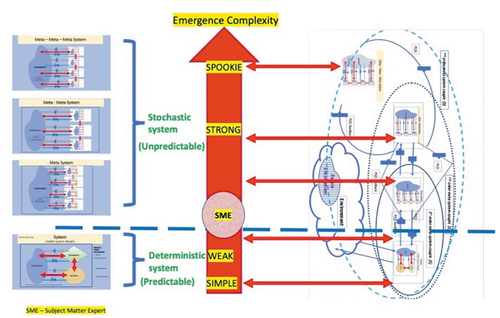
The SoS operating within other such systems contribute to overall capabilities. Combining these SoS can lead to more emergent behaviors than are usually seen in single systems. These behaviors may either improve or degrade performance. The challenge of design in the SoS is to leverage the functional and performance capabilities of the constituent systems to achieve the desired SoS capability. The crosscutting characteristics of the SoS ensure that they meet the broader user needs.
Findings
An Overview of the Research Evidence
The SoS emergent behavior is relevant to engineering and natural systems and is not well understood. Evidence may include any systematic observation to establish facts and arrive at conclusions. This literature review examines emergent behavior through the theory of critical system thinking (D’Andreamatteo et al. Citation2019) and cybernetics. In complex problem solving, we can assume that all systemic properties will be investigated; however, this is where the nature of the problem is revealed. Therefore, cybernetics and system thinking give rise to a new concept in problem-solving, which is currently not well defined, understood, or clearly tangible to the assessment of the operations within engineering. In complex problem solving, we can assume to have all the systemic properties investigated, which is where the nature of a problem is revealed. The introduction of systemic thinking and cybernetics provides the framework elements and methods used to build the meta-methodological model that remains unclear or unavailable.
Diagram 11. Meta model methodology design by Thomann (Citation1973).
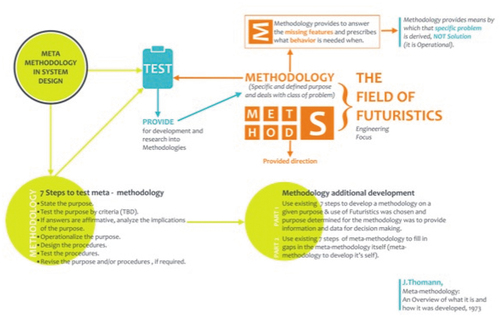
A meta methodology is a way of developing and testing a method for a specific, defined purpose. An overview of Meta-Methodology and how it was designed (Thomann Citation1973). To establish a theoretical framework for modeling and simulation, it is necessary to first establish a taxonomy of emergent behaviors, which is unclear thus far. Further, evidence is lacking on the emergent behavior that is present in constituent systems that support the system’s design. Combinations of systems operating together within the SoS can contribute to overall capabilities. Combining systems can lead to emergent behaviors that may improve or degrade performance and decrease or increase costs.
Conclusion
This paper concludes that the concepts, ideas, theories, tools and general methodologies of nonlinear dynamics and complex systems theory show enormous, almost total, potential for not just providing better solutions for some existing issues of land combat, but for fundamentally altering our general understanding of the fundamental processes of war, at all levels. Indeed, the new science’s most significant legacy may, in the end, be not just a set of creative answers to old questions but an entirely new set of questions to be asked about what happens on the battlefield. The central thesis of this paper is that land combat is a complex adaptive system. Land combat is a nonlinear dynamical system composed of many interacting semi-autonomous and hierarchically organized agents continuously adapting to a changing environment.
The BMS focuses on distributing information across a warfighting network and is a network of bases and electronic warfighting platforms. The rise of automation in multiple systems and technologies presents a complex operational environment. Such environments require highly collaborative, CASoS solutions. Combining systems may lead to more emergent behaviors than is usually observed in single systems (Kaisler and Madey Citation2009). The emergent behavior is imperative in developing a framework to safely and securely deliver large and complex engineering projects to produce new insights and practical steps to improve complex project success (Juli Citation2011).
Emergence may be positive or negative and may take shape (types) in various systems that range from simple to complex. Therefore, a mechanism that provides a structured approach for analyzing and controlling such behaviors is required. We make a case for a framework to explore emergent behaviors in a multi-agent system (O’Toole, Nallur, and Clarke Citation2014). The aim is to demonstrate that if any emergent behavior system, that is, a complex (multi-agent) system exhibiting emergence, is represented formally using the developed framework, this would render it easy for a modeler to analyze and study the causal relationships between the micro and macro layers of the system. It is possible to use a case study to demonstrate how the BMS framework can be beneficial in implementing and classifying emergent behaviors using existing and known approaches in the literature. The challenge of design in the SoS is to leverage the functional and performance capabilities of the constituent systems to achieve the desired capability (Juli Citation2011). To establish the theoretical framework for modeling and simulation, it is necessary to first establish a taxonomy of emergent behaviors in a project, which is not always clear. In examining emergent behavior in BMS and vis-à-vis cyber-physical systems, the current study makes a significant contribution to the literature by offering insights into a domain that has not been examined in as much depth and detail thus far, and the resulting additions to the literature can be valuable in shaping future research and policymaking in the field. Further, it presents some novel perspectives that could expand the scope of extant literature on defense.
Disclosure statement
No potential conflict of interest was reported by the author(s).
Additional information
Funding
References
- Adams, K. M., P. T. Hester, J. M. Bradley, T. J. Meyers, and C. B. Keating. 2014. Systems theory as the foundation for understanding systems. Systems Engineering 17 (1):112–3833. doi:10.1002/sys.21255.
- Ashby, W. R. 1965. Introduction to cybernetics. London: Chapman & Hall, Ltd.
- Bar-Yam, Y. 2004a. Multiscale variety in complex systems. Complexity 9 (4):37–45. doi:10.1002/cplx.20014.
- Bar-Yam, Y. 2004b. Making things work: Solving complex problems in a complex world. NECSI-Knowledge Press.
- Beer, S. 1972. Brain of the firm: A development in management cybernetics. New York: Herder & Herder.
- Beer, S. 1984. The viable system model: Its provenance, development, methodology and pathology. The Journal of the Operational Research Society 35 (1):7–25. doi:10.1057/jors.1984.2.
- Burbeck, S. 2007. Complexity and the evolution of computing: Biological principles for managing evolving systems. doi:10.13140/RG.2.1.3021.0722.
- Chen, X., J. Cao, J. Qui, Y. Jing, L. Yang, and B. Zhang. 2014. Optimal control of a class of warfare dynamic systems based on Lanchester (2,2) attrition model. Paper presented at the 27th Chinese Control and Decision Conference (CCDC), Qingdao, China, May 23–25.
- Chen, X., Y. Jing, C. Li, and X. Liu. 2011. Optimal strategies for winning in military conflicts based on Lanchester equation. Control and Decision 26 (6):946–50.
- D’Andreamatteo, A., L. Ianni, A. Rangone, F. Paolone, and M. Sargiacomo. 2019. Institutional pressures, isomorphic changes and key agents in the transfer of knowledge of Lean in Healthcare. Business Process Management Journal 25 (1):164–84. doi:10.1108/BPMJ-06-2017-0174.
- Engel, J. H. 2001. Lanchester’s equations. In Encyclopedia of operations research and management science, ed. S. I. Gass. New York: Springer. doi:10.1007/1-4020-0611-X_515.
- Genesereth, M. R. 1983. An overview of meta-level architecture. Paper presented at the National Conference on Artificial Intelligence, Austin, Texas.
- Giammarco, K. M. 2018. Naval Postgraduate School. Faculty profile directory: Kristin M Giammarco. Systems Engineering Naval Postgraduate School, Graduate School of Engineering and Applied Sciences. https://nps.edu/faculty-profiles/-/cv/kmgiamma.
- Henshaw, M. 2015. Good practice in Systems of Systems Engineering (SoSE). In SCI276 Lecture Series. CSO, North Atlantic Treaty Organization.
- Holland, O. T. 2007. Taxonomy for the modeling and simulation of emergent behavior systems. Paper presented at the 2007 Spring Simulation Multiconference 2, Norfolk, March 25–29. doi:10.1145/1404680.1404684.
- Johnson, B. R. 2016. Design computing: An overview of an emergent field. New York: Routledge.
- Jonas, S., Q. Zhang, L. Ljung, A. Benveniste, B. Delyon, P.-Y. Glorennec, H. Hjalmarsson, and A. Juditsky. 1995. Nonlinear black-box modeling in system identification: A unified overview. Automatica 31(12) : 1691–724. ISSN 0005-1098. doi:10.1016/0005-1098(95)00120-8.
- Juli, T. 2011. The five team leadership principles for project success. Paper presented at the PMI® Global Congress, Dallas, Texas, October 22–25.
- Kaisler, S. H., and G. Madey. 2009. Complex adaptive systems: Emergence and self-organisation. In Tutorial presented at the HICSS-42. 5 January Hawaiʻi: Big Island. http://www3.nd.edu/~gmadey/Activities/CAS-Briefing.pdf.
- Lanchester, F. W. 1999. Aircraft in warfare: The dawn of the fourth arm. Sunnyvale, CA: Lanchester Press, Inc.
- Lee, J., S. Shin, M. Park, and C. Kim. 2018. Agent-based simulation and its application to analyse combat effectiveness in network-centric warfare considering communication failure environments. Mathematical Problems in Engineering 2018:1–9. doi:10.1155/2018/2730671.
- Loerch, A. G., and L. B. Rainey. 2007. Methods for conducting military operational analysis. In Military Operations Research Society and LMI Research Institute.
- Lundberg, G. A. 1949. POLANYI, MICHAEL. Science, Faith and Society. The Annals of the American Academy of Political and Social Science 262(1) : 238–39. Pp. 80. London and New York: Oxford University Press, 1946, 1948. 1.00. doi:10.1177/000271624926200184.
- Maier, M. W. 1998. Architecting principles for systems‐of‐systems. Systems Engineering: The Journal of the International Council on Systems Engineering 1 (4):267–84. doi:10.1002/(SICI)1520-6858(1998)1:4<267:AID-SYS3>3.0.CO;2-D.
- McCulloch, W. S. 1995. Summary of the points of agreement reached in the previous nine conferences on cybernetics. Cybernetics: Circular-causal and feedback mechanisms in biological and social systems. In Transactions of the 10th conference on cybernetics, ed. H. V. Foerster, M. Mead and H. L. Teuber, 69–80. New York: Josiah Macy Jr. Foundation.
- MITRE. 2021. Treating system of systems as systems. Accessed December 2021. https://www.mitre.org/publications/systems-engineering-guide/enterprise-engineering/systems-of-systems/treating-systems-of.
- Osipov, Y. S., and V. I. Maksimov. 2018. Tracking the solution to a nonlinear distributed differential equation by feedback laws. Numerical Analysis and Applications 11 (2):158–69. doi:10.1134/S1995423918020064.
- O’Toole, E., V. Nallur, and S. Clarke. 2014. Towards decentralised detection of emergence in complex adaptive systems. Vol. 2014 IEEE Eighth International Conference on Self-Adaptive and Self-Organizing Systems, 60–69. doi:10.1109/SASO.2014.18.
- Polanyi, M., and R. T. Allen. 1997. Society, economics and philosophy. New Brunswick NJ: Transaction Publishers.
- Rainey, L. B., and M. Jamshidi, ed. 2018. Engineering emergence: A modeling and simulation approach. USA: CRC Press.
- Rainey, L. B., and S. Mittal. 2015. Harnessing emergence: The control and design of emergent behavior in system of systems engineering. In Proceedings of the Conference on Summer Computer Simulation, 1–10.
- Rainey, L. B., and A. Tolk, ed. 2015. Modeling and simulation support for system of systems engineering applications. Hoboken:John Wiley & Sons, Inc.
- Rawson, A. 2012. Battle story: Iwo Jima 1945. Gloucestershire, UK: The History Press.
- Rugh, W. J. 1981. Nonlinear system theory, 12–90. Baltimore: Johns Hopkins University Press.
- Sage, A. 2016. Cybernetics and complex adaptive systems. In Encyclopedia of operations research and management science, ed. S. I. Gass. Boston, MA: Springer. doi:10.1007/978-1-4419-1153-7_205.
- Schetzen, M. Dec 1981. Nonlinear system modeling based on the Wiener theory. Proceedings of the IEEE 69(12):1557–73. doi: 10.1109/PROC.1981.12201.
- Schwartz, S. H. H. 2012. An overview of the Schwartz theory of basic values. Online online Readings in Psychology and Culture 2 (1):1. doi:10.9707/2307-0919.1116.
- Singh, S., S. Lu, M. M. Kokar, P. A. Kogut, and L. Martin. 2017. Detection and classification of emergent behaviors using multi-agent simulation framework (WIP). In Proceedings of the Symposium on Modeling and Simulation of Complexity in Intelligent, Adaptive and Autonomous Systems, Virginia Beach, Virginia, April 23–26. doi:10.5555/3108414.3108417.
- Thomann, J., 1973. “Meta-methodology: An Overview of What It is and How It Was Developed.” Paper presented at the 58th American Educational Research Association Annual Meeting, New Orleans, Louisiana, February 26 – March 1.
- Washburn, J. D., K. A. Bird, G. C. Conant, and J. C. Pires. 2016. Convergent evolution and the origin of complex phenotypes in the age of systems biology. International Journal of Plant Sciences 177 (4):305–18. doi:10.1086/686009.
- Wiener, N. 1961. Cybernetic or control and communication in the animal and the machine. 2nd ed. New York: MIT Press/Wiley.
- Yolles, M. 2021. Metacybernetics: Towards a general theory of higher order cybernetics. Systems 9 (2):34. doi:10.3390/systems9020034.