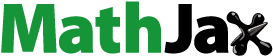
ABSTRACT
It is true that with society entering a new era, the pressure of competition for employment of college students is increasing, and society has higher requirements for the quality of high-tech talents, which can result in mental health issues for college students. Therefore, focusing on psychological education for college students and improving their psychological quality is essential. The analysis and countermeasure research on psychological characteristics has become crucial in this context. To address this issue, this work proposes an Improved Particle Swarm Optimization (IPSO) Deep Belief Network (DBN) method to analyze the psychological characteristics of college students. The proposed method first uses DBN to analyze the psychological characteristics of college students. Secondly, the learning factors and inertia weights in PSO are dynamically adjusted to construct IPSO to address the problem of slow overall convergence speed caused by the initial randomization of weights in the DBN training process. IPSO is then combined with DBN to adjust the initial weights for DBN training. This work also proposes a series of countermeasures from different aspects to strengthen mental health education for college students. The validity of IPSO-DBN for college students’ psychological characteristics analysis is verified through comprehensive and systematic experiments, and the feasibility of college students’ mental health education countermeasures is confirmed via comparison. Overall, this work provides an innovative and effective approach for analyzing college students’ psychological characteristics and developing countermeasures to improve their mental health. It shows the potential of combining artificial intelligence with psychological education and highlights the importance of strengthening psychological health education for college students.
Introduction
Psychological education is a kind of education that cultivates good psychological quality and prevents and treats mental diseases. As a college student, they have access to a wide range of courses and programs designed to help you enhance your psychological well-being and your ability to contribute to the growth of your school and your community. At this point in time, society has high criteria for talent, not only professional knowledge and moral standards, but also good psychological quality, capable of actively dealing with many obstacles and properly handling various matters. Quality education should be executed in order to satisfy the needs of the times, and psychological quality education is an important component of quality education. When fostering high-quality talent, schools and universities should pay special attention to the psychological quality of their students’ education. Having a healthy mentality is an important foundation for everyone to study, work and live normally (Du et al. Citation2017; Grant, Lust, and Chamberlain Citation2019; Selvaraj and Bhat Citation2018; Sharp and Theiler Citation2018; Zhang et al. Citation2018).
It has become an indisputable fact that the employment of college graduates from ordinary colleges and universities is becoming more and more difficult. As college students, they know the severity of their professional employment situation through certain channels. Conflicts among some students’ majors, interests, employment goals, and personality characteristics make them feel conflicted, causing them to have emotions such as fear, anxiety, and irritability. This makes some college students lack confidence and courage, thinking that they cannot face the fierce competition. On the other hand, they are afraid of enduring hardships and suffering, and are unwilling to start at the grassroots level, hoping to find a job with high income and a good environment. This contradiction has caused a large number of college students’ employment psychological problems. College students are in the age group where their minds are gradually mature, and they have a strong need for interpersonal communication. Due to the great differences in living habits, interests and hobbies, and the fact that college students have developed self-centered living habits since childhood, they lack the spirit of teamwork and the concept of collective. Conflicts and frictions will inevitably arise in communication, and if they are not handled properly, it will lead to tensions in interpersonal relationships. The psychological problems of some college students have a great relationship with their native families. The economic status of the family, the degree of family harmony, and the family’s upbringing style all affect mental health to a large extent. Negative emotions or thoughts that are not dealt with will often appear later in life. Negative thoughts about self or others due to childhood experiences when faced with similar situations (Busari et al. Citation2019; Ebert et al. Citation2019; Lipson et al. Citation2019; Pasinringi et al. Citation2022; Tang, Hu, and Yang Citation2020).
For college students, they should not only master modern scientific and cultural knowledge, but also have good psychological quality, so as to rationally deal with various difficulties and problems. However, college students in colleges and universities are still in the developmental stage, that is, they are basically mature physically, but not really mature psychologically. Especially in the psychological aspect, there are often all kinds of contradictions or conflicts. Under the background of the coexistence of multiple social values and multiple economic components of the market economy, some hedonism and money worship have emerged. This affects psychology s to a certain extent, aggravates psychological conflict for college students, makes students have a psychological state of confusion and doubt, and it is difficult to choose outlook. If the inner contradictions as well as conflicts are not effectively channeled, it is likely to form psychological problems. In particular, contemporary college students are affected by excessive parental protection and lack of life experience, and some students are psychologically fragile and weak in willpower. After entering the university, it is difficult to bear the small difficulties and setbacks in study and making friends. Over time, psychological problems will occur, and the phenomenon of dropping out of school may occur. In severe cases, suicide may occur. Therefore, to avoid occurrence of above situation, colleges should strengthen psychological education, so as to be able to spend the college life happily and smoothly. Strengthening psychological quality education also promotes the healthy growth of college students to a certain extent, which is beneficial to their future development (Chen, Sun, and Feng Citation2020; Ebert et al. Citation2019; Harrer et al. Citation2019; Lin, Zheng, and Alexandropoulos Citation2020; Rafal, Gatto, and DeBate Citation2018).
The motivation of the research is to address the increasing pressure and mental health issues among college students due to the growing competition for employment and higher expectations from society for high-tech talents. The focus is on strengthening psychological education and improving the psychological quality of college students.
The research proposes an Improved Particle Swarm Optimization (IPSO) Deep Belief Network (DBN) method to analyze the psychological characteristics of college students. The method utilizes DBN for analyzing psychological characteristics and IPSO for dynamically adjusting learning factors and inertia weights to address the problem of slow overall convergence speed caused by the initial randomization of weights in the DBN training process. The proposed method is validated through comprehensive and systematic experiments.
The contribution of the conducted research is providing an innovative and effective approach to analyzing college students’ psychological characteristics and developing countermeasures to improve their mental health. The research shows the potential of combining artificial intelligence with psychological education and highlights the importance of strengthening psychological health education for college students.
The key findings of the research are the effectiveness of the proposed IPSO-DBN method in analyzing college students’ psychological characteristics and the validity of the proposed countermeasures to improve their mental health. The research confirms the feasibility of the proposed method and countermeasures through comparison with other approaches.
The rest of the paper is structured as follow:
2. Related Work
Review the related literature on psychological education, mental health issues among college students, and artificial intelligence methods for analyzing psychological characteristics.
Highlight the gaps and limitations of the existing research and how the proposed method addresses them.
3. Method
Describe the IPSO-DBN method for analyzing the psychological characteristics of college students.
Explain the steps involved in the method, including DBN analysis, IPSO weight initialization, and IPSO-DBN combination.
Provide a detailed explanation of the learning factors and inertia weights in PSO and how they are dynamically adjusted in IPSO to improve overall convergence speed.
4. Experiment and Analysis
Describe the data sets used in the experiments and how they were collected.
Present the experimental results of using IPSO-DBN to analyze the psychological characteristics of college students.
Compare the results with other approaches to verify the effectiveness and validity of the proposed method.
Analyze the findings and draw conclusions on the contribution and implications of the research.
5. Conclusion
Summarize the research problem, the proposed method, and the experimental results.
Reiterate the contribution of the research to the field of psychological education and mental health among college students.
Discuss the limitations and future directions of the research.
Provide recommendations for practitioners and policymakers based on the findings of the research.
Related Work
Literature (Kitzrow Citation2003) believes that the research scope of mental health education should be expanded to cover psychological problems such as career choice, employment, social interaction anxiety, mental illness, suicide, addiction, love and sex. It probes into problem for mental health management in colleges from three aspects, such as work concept, subject development and management organization. Literature (Hunt and Eisenberg Citation2010) believes that college chat rooms can play a significant role in releasing psychological pressure, and students’ problems can be solved in chat. It believes that 15.6% of undergraduates in colleges and universities suffer from depression or anxiety, 10% of graduate students, 2% of students with suicidal ideation, and difficult family conditions are more prone to psychological disorders. Literature (Storrie, Ahern, and Tuckett Citation2010) put forward its own concept in the development of mental health management in colleges. It believes colleges should not only focus on cultivating intellectual talents, but must take emotional as a goal. Literature (Hefner and Eisenberg Citation2009) believes that in the management of psychological education, attention should be paid to all-round development of students, including the cultivation of all aspects of physical, psychological, spiritual, belief and environment. With the development of society, the attention of government departments, and the needs of students, college psychological counseling institutions have been established one after another, basically in every university. Literature (Eisenberg, Downs, and Golberstein Citation2009) clearly pointed out that if you want to work as a psychological educator, you must have a professional psychological education qualification certificate. After World War II, psychological counseling and counseling has become a professional discipline in western developed countries, and more and more people have joined this work. With the increased investment in mental health by the state and the government, especially in colleges, focusing on actual life, psychological counseling and counseling has become a key discipline. Its purpose is to promote college students’ study and quality of life in school.
Literature (Auerbach, Alonso, and Axinn Citation2016) pointed out that the psychological committee system is the demand, it is necessary to optimize development of psychological education management from five aspects: concept, subject, content, mechanism and form. It proposes that to reduce the probability of malignant events caused by psychological problems in the students of new undergraduate colleges, the mode of intervention psychological crisis is adopted to carry out the psychological education management of undergraduate colleges. Literature (Pedrelli, Nyer, and Yeung Citation2015) stated that quality development training can cultivate innovative spirit and strong will. Integrating it into psychological management can guide students to release pressure and improve their personality. And to implement hardware guarantee for management, change the traditional teaching form, strengthen the curriculum construction, and improve the level of the teaching staff. Literature (Blanco, Okuda, and Wright Citation2008) pointed out the great significance of cooperation between schools and families, and proposed effective methods to improve preventive interventions from four aspects: broadening breadth, tempering depth, self-cultivation depth, and enhancing confidence. Literature (Zivin, Eisenberg, and Gollust Citation2009) shows that the traditional psychological education management mode is limited by various subjective factors, and most of them are carried out in a one-size-fits-all manner, resulting in low effectiveness. Psychological data can be mined to the fullest extent possible by implementing a big-data approach to psychological education management. Literature (Nguyen-Feng et al. Citation2017) found through an investigation on the status quo of local undergraduate comprehensive colleges and universities that it is a matter of time for schools to offer mental health courses. Students do not pay attention to their own quality and other issues, so they propose countermeasures to improve their understanding, build a curriculum system, and carry out model activities. Literature (Canby et al. Citation2015) puts forward countermeasures such as giving full play to invisible educational resources through in-depth analysis of the lack of in-depth understanding, lack of pertinence, and lack of perfect counseling system and mechanism in the management of mental health education. Literature (Eisenberg, Golberstein, and Gollust Citation2007) think it is necessary to deconstruct old model of psychological management in newly-built undergraduate colleges, and put forward the innovative exploration of network reconstruction to carry out reform and development in response to the existing problems in psychological education management. Literature (McGuire and Miranda Citation2008) believes that psychological education courses have outdated concepts that cannot meet the characteristics of students’ development, lag in content construction and cannot play the actual effect, and unscientific evaluation cannot reflect the real psychological level of students. Literature (Levecque et al. Citation2017) pointed out that in order to cultivate the high-quality innovative talents urgently needed by the society, it is necessary to create a multi-faceted joint force led by the school and taught by the family, to promote high-quality development of psychological quality. Literature (Kim et al. Citation2017) finds the existing problems through the current situation for mental education, proposes a new method to strengthen teachers and build a dynamic network. It proposes to encourage the construction of new associations for special groups of college students, and to continue to care for the psychological security of these groups. Literature (Eisenberg, Hunt, and Speer Citation2013) proposes to focus on problem students to pay attention to all students, from negative psychological problems to positive personality cultivation, from theoretical teaching to emphasizing emotional experience, so as to promote the healthy growth of students.
Method
This work proposes an IPSO-DBN method to analyze the psychological characteristics of college students. First, DBN is utilized to analyze psychological characteristics of college students. Secondly, in view of the problem of slow overall convergence speed caused by the initial randomization of weights in the DBN training process, the learning factors and inertia weights in PSO are dynamically adjusted to construct IPSO. Then, IPSO is combined with DBN to adjust the initial weights for DBN training. Finally, this work proposes a series of countermeasures from different aspects to strengthen mental health education.
DBN Algorithm
DBN is a network structure composed of multiple layers of neurons, and its internal structure is composed of RBM. To overcome the problems of local minimum value as well as low training efficiency of neural network, DBN adopts a different training mechanism from BP neural network. DBN firstly trains the internal RBM network layer by layer, and then performs reverse fine-tuning, so as to achieve the goal of adjusting the entire network. BM is an energy-based neural network model, which defines the network state as energy. Therefore, the training of the network is actually minimizing the energy function. When the energy function can be minimized, it can be considered that the training of the network has reached the most ideal state. Compared with BM, the difference between RBM and BM is that there is no data link in the hidden layer and visible layer, its structure is demonstrated in .
In order to analyze the structure of the RBM, the energy function of the RBM is first established. It is the link between the value of each visible layer neuron and the value of each hidden layer neuron that is referred to as the energy function. The visible layer unit and the hidden layer unit produce the following energy.
The joint probability distribution of the energy model in which the visible layer and hidden layer are in a certain state is:
When the visible layer vector is known, the conditional probability of each unit input in the hidden layer is:
When the hidden layer vector is known, the probability formula of each unit of the visible layer is:
The training process of RBM is generally carried out in an iterative manner. The reason is to obtain the most suitable parameters to minimize the energy of the entire model. The most direct and effective way is to adjust the internal parameters. According to the principle of maximum likelihood, if the energy function is to be minimized, the logarithm of the likelihood function of the sample must be maximized. The input layer of DBN is the first RBM network visible from the bottom up in the network topology of DBN. The DBN’s first hidden layer is also the first RBM’s hidden layer and the second RBM’s visible layer. Second RBM is the hidden layer of the second RBM, which is also visible in the third RBM network, and so on.
Pre-training and fine-tuning make up the bulk of the DBN training procedure. An unsupervised, bottom-up approach to pre-training is used. DBN can train the internal RBM network layer by layer to get a set of weights that are close to ideal. And get the internal hidden layer feature vector, weaken some less important information features. This ensures that each RBM network is independent, so that the weights of each layer are optimal for that layer, not based on the entire network. Fine-tuning is a top-down supervised training process that uses the BP neural network to propagate the error information down layer by layer in reverse, and adjust the error size layer by layer. Each time a layer of RBM is passed, the internal weights are fine-tuned. This can speed up training speed and reduce training time, network model can be optimized. The last layer of DBN is a classifier, and BP network is commonly used, but other network models can also be selected according to actual needs. On the whole, the training process of DBN is equivalent to the directed initialization of the internal weights of a BP network by multiple RBM networks. The training method of DBN can well solve the defects of random initialization of weights and bias values of BP neural network, and to a certain extent, it can avoid the problem that the whole network is easy to fall into the local optimal solution and the training time is too long.
PSO Algorithm
PSO searches for the optimum globally through probability, which has fast convergence speed, good robustness, less parameter adjustment, and is suitable for engineering applications. The DBN has a certain self-learning ability, and the network structure is relatively rich. Using PSO to optimize the random initial weights of DBN training can not only give play to advantages of PSO, but also improve overall learning and convergence speed of the prediction model. Therefore, this work optimizes DBN based on PSO.
PSO is based on population-based stochastic optimization, and each optimization problem in the algorithm is a particle. Particles cooperate and compete to achieve efficient search in multi-dimensional complex spaces. Search space particles fly at a particular speed to discover the global optimal solution and have their own position and speed vectors as well as the fitness value determined by the optimal function. For the particle’s current position, two extreme values must be sought: the particle’s own ideal solution and the global optimal solution in the area where it is located. The position and velocity update methods of the particle swarm algorithm are:
The PSO pipeline is demonstrated in .
DBN Optimization with Improved PSO
The learning factor and the inertia weight have a significant impact on the algorithm’s performance in practice. As the particle learns, its flight inertia can be altered. This is the learning factor. Both the particle’s individual cognition and the group’s collective cognition are reflected in the first learning element. The inertia weight is a measure of how the past velocity of a particle affects its present velocity. There are many practical applications where the particle swarm optimization algorithm should be able to focus on its global search ability in order to avoid falling into a local minimum early on. Later, focus on the ability to search locally, which can lead to a global optimal. This improves the accuracy and efficiency of the algorithm.
The inertia weight in PSO is connected to the algorithm’s global and local search capabilities. The algorithm’s global search capability improves as the inertia weight increases, whereas the algorithm’s local search capability degrades. On the other hand, the more powerful the algorithm’s local search capabilities are, the weaker its global search capabilities will be. So the appropriate inertia weight should have a higher initial weight but lower later on. A weight dynamic value method, or an inertial weight adaptive strategy, is employed in this study. As the number of iterations increases, so may the value of this approach. The inertia weight reduces with increasing iteration steps.
In particle swarm optimization, two learning factors represent the particle’s self-cognition and group cognition respectively. After research, it is found that when the first learning factor is larger, the particle should have larger self-awareness. This facilitates a complete search of the algorithm in the entire space without prematurely falling into local minima. When the second learning factor is larger, the particles have larger group cognition, which is beneficial for the algorithm to converge to global optimum. Since the optimal learning factor should be to have a large early stage and a small later stage, it should be a small early stage and a large later stage for each learning factor. The following dynamic adjustment method is employed in this project.
DBN is formed by stacking multiple layers of RBM, and in the training process of DBN, the weights are randomly initialized. In this way, it is easy to fall local optimization, it will increase network iterations, which will slow down the convergence speed. Therefore, this paper uses an improved PSO (IPSO) to optimize random initial weights during training. It connects the number of weights in training to the number of particles in PSO, connects fitness to error in DBN training. IPSO-DBN pipeline is demonstrated in .
Psychoeducational Countermeasure
This study aims to improve college students’ mental health education by proposing a number of remedies to help college students.
The first countermeasure is to strengthen mental health education. Under conditions for continuous in-depth reform of colleges, mental health education courses have been offered in some colleges and universities. Although some development has been achieved, it is still in the primary stage, and there are still certain deficiencies in the mental health system, professional teachers, and professional teaching methods, so it needs to be further strengthened. This goal can be achieved by beginning with these elements. To begin, make it clear what the purpose of mental health education is and pair it with political and ideological instruction. Students’ outlook on life, values, and morality can be cultivated in this way, and their psychological well-being can be enhanced as a result. As a second step, create up college students’ psychological and health counseling institutions to help college students deal with the stress of college life and grow up healthy. Mental health education for college students must follow a student-centered approach and improve students’ psychological self-regulation skills through mental health teaching, in other words.
The second strategy is to cultivate the good personality. Colleges help college students develop good personalities and lay the foundation for their long-term future development. First, let students know themselves correctly, face up to their own situation, actively face the setbacks they encounter, take scientific and effective methods to solve difficulties, and turn setbacks into the driving force for progress in life. The second is to cultivate students’ attitude toward life and love of learning, to get along well with the people around them, and to enhance students’ interpersonal skills. The third is to guide students to actively participate in various campus activities and social practice activities, through these activities to enrich students’ spare time life, and constantly tap students’ potential ability, so that they can integrate into the collective and adapt to the society.
Create an ideal learning and living environment as the third countermeasure. The growth of college students’ mental health is affected by changes in both their internal and external surroundings. Because of this, college students place a great deal of importance on the surrounding environment. It is imperative that educational institutions work to foster a sense of community among their pupils, improve the quality of their instruction, and create a conducive learning environment for their inmates. It’s also critical that students have a pleasant place to live, so they can focus on their studies without distractions.
Experiment and Analysis
IPSO-DBN Analysis
This work collects mental health data from colleges as the training data and test data of IPSO-DBN, which contains a total of 69,728 samples, of which 50,286 samples constitute the training set. Each sample contains an 8-dimensional mental health feature, as demonstrated in , and its label is the corresponding mental feature classification.
Table 1. Detailed mental health feature.
First, this work compares IPSO-DBN with other machine learning methods for psychological characteristics analysis of college students. The compared methods include logistic regression, decision tree, KNN, SVM and BP. The comparison results of precision and recall are demonstrated in .
According to the data shown on the way, IPSO-DBN can obtain the highest precision and recall rate when analyzing the psychological characteristics of college students. Compared with the BP algorithm, performance improvements of 2.2% and 1.6% can be obtained.
IPSO-DBN uses improved inertia weights to optimize PSO. To prove the effectiveness of this improved strategy, the precision and recall rates when using the improved inertial weight (IIW) and the traditional inertial weight (TIW) are compared respectively, and the comparison data is demonstrated in .
Based on the comparative data analysis, after improving the traditional inertia weight, IPSO-DBN can achieve 1.6% and 1.3% improvement in precision and recall.
IPSO-DBN uses improved learning factor to optimize PSO. To prove the effectiveness of this improved strategy, the precision and recall rates when using the improved learning factor (ILF) and the traditional learning factor (TLF) are compared respectively, and the comparison data is demonstrated in .
Based on the comparative data analysis, after improving the traditional learning factor, IPSO-DBN can achieve 1.4% and 0.9% improvement in precision and recall.
IPSO-DBN uses the improved PSO to optimize DBN. In order to verify the feasibility of IPSO to optimize DBN, the training convergence time and network performance of DBN, PSO-DBN and IPSO-DBN are compared respectively. The comparison data is demonstrated in .
Table 2. Comparison of different DBNs.
Based on the comparative data shown in the table, the accuracy and recall of the traditional DBN network are the lowest, and the training convergence time is the longest. After optimization with PSO, the performance can be improved effectively. However, after improving the PSO and then optimizing the DBN, the highest accuracy and recall rate can be obtained, and the training time is greatly shortened.
Psychoeducational Countermeasure Analysis
This work proposes several countermeasures to improve the mental health education of college students. In order to verify that these countermeasures can effectively improve the mental health of college students, this work conducts corresponding comparative experiments to compare the mental health scores of college students before and after using these strategies. The compared indicators are the same as the eight indicators in , and the specific comparison data are demonstrated in .
According to the data comparison of the two figures, after using the mental health education countermeasures for college students proposed in this work, the mental health indicators of college students have improved to varying degrees. This proves the feasibility and superiority of this educational strategy.
Conclusion
Psychological education is the main channel for colleges to conduct mental health education. It can prevent and treat mental diseases, improve psychological adjustment, and promote psychological development. Therefore, the effect of college students’ psychological education directly affects the physical and mental development, also affects educational effect of mental health education and political education. It is a proposition that colleges and universities need to study urgently to explore the psychological conditions of college students, and to pursue the reasons for the formation of these psychological characteristics, so as to carry out targeted mental health and ideological and political education. This work proposes an IPSO-DBN method to analyze the psychological characteristics of college students. First, DBN is utilized to analyze psychological characteristics of college students. Secondly, in view of the problem of slow overall convergence speed caused by the initial randomization of weights in the DBN training process, the learning factors and inertia weights in PSO are dynamically adjusted to construct IPSO. Then, IPSO is combined with DBN to adjust the initial weights for DBN training. Finally, this work proposes a series of countermeasures from different aspects to strengthen the mental health education of college students. This work verifies the validity of IPSO-DBN for college students’ psychological characteristics analysis through comprehensive and systematic experiments, and also verifies the feasibility of college students’ mental health education countermeasures through comparison.
Here are some study limitations:
Limited data availability: The research may have been limited by the availability of data sets, which could affect the generalizability of the findings.
Subjectivity of psychological characteristics: The analysis of psychological characteristics may be subjective and dependent on the individual perceptions of the students and the raters, which could limit the reliability and validity of the findings.
Limited scope of countermeasures: The proposed countermeasures may have limited scope and applicability, as they may not be suitable for all types of mental health issues among college students.
Lack of long-term follow-up: The research may not have included long-term follow-up of the students to determine the sustained effectiveness of the proposed countermeasures in improving their mental health.
Potential bias in the experimental design: The experimental design may have included potential biases, such as selection bias or measurement bias, which could affect the internal and external validity of the findings.
Some possible future research directions that could be explored in this field:
Investigation of the effectiveness of the proposed method with larger and more diverse data sets: Future research could expand the sample size and demographic diversity of the data sets used in this study to determine whether the proposed IPSO-DBN method is effective and reliable across different populations.
Evaluation of the long-term impact of the proposed countermeasures: Future research could follow up with college students who participated in the proposed countermeasures to determine their long-term effects on mental health outcomes.
Exploration of the potential of other artificial intelligence methods: Future research could investigate the potential of other artificial intelligence methods, such as machine learning, deep learning, and natural language processing, for analyzing psychological characteristics and improving mental health among college students.
Development of more comprehensive and tailored countermeasures: Future research could develop more comprehensive and tailored countermeasures to address specific mental health issues among college students, such as stress, anxiety, depression, or burnout.
Investigation of the role of technology in mental health education: Future research could explore the potential of using technology, such as virtual reality, social media, or mobile applications, to enhance mental health education and improve psychological well-being among college students.
These future research directions can help to advance the field of psychological education and mental health among college students and provide practical solutions for addressing the increasing pressure and mental health issues among this population.
Disclosure statement
No potential conflict of interest was reported by the authors.
Additional information
Funding
References
- Auerbach, P., R. J. Alonso, and W. G. Axinn. 2016. Mental disorders among college students in the World Health Organization World Mental Health Surveys. Psychological Medicine 46 (14):2955–1243. doi:10.1017/S0033291716001665.
- Blanco, C., M. Okuda, and C. Wright. 2008. Mental health of college students and their non–college-attending peers: Results from the National Epidemiologic Study on Alcohol and Related Conditions. Archives of General Psychiatry 65 (12):1429–37. doi:10.1001/archpsyc.65.12.1429.
- Busari, A. S., Huq, K. M. S, S. Mumtaz, Y. Fang, D. C. Sicker, S. Al-Rubaye, and A. Tsourdos. 2019. Generalized hybrid beamforming for vehicular connectivity using thz massive MIMO. IEEE Transactions on Vehicular Technology 68 (9):8372–83. doi:10.1109/TVT.2019.2921563.
- Canby, K. N., Cameron, I. M, and A. T. Calhoun. 2015. A brief mindfulness intervention for healthy college students and its effects on psychological distress, self-control, meta-mood, and subjective vitality. Mindfulness 6 (5):1071–81. doi:10.1007/s12671-014-0356-5.
- Chen, B., J. Sun, and Y. Feng. 2020. How have COVID-19 Isolation policies affected young people’s mental health–Evidence from Chinese College Students. Frontiers in Psychology 11:1529. doi:10.3389/fpsyg.2020.01529.
- Du, J., C. Jiang, Z. Han, H. Zhang, S. Mumtaz, and Y. Ren. 2017. Contract mechanism and performance analysis for data transaction in mobile social networks. IEEE Transactions on Network Science and Engineering 6 (2):103–15. doi:10.1109/TNSE.2017.2787746.
- Ebert, D., and D. Mortier P, and F. Kaehlke, R. Bruffaerts, H. Baumeister, R. P. Auerbach, J. Alonso, G. Vilagut, K. U. Martínez, C. Lochner, P. Cuijpers. 2019. Barriers of mental health treatment utilization among first‐year college students: First cross‐national results from the WHO World Mental Health international college student initiative. International Journal of Methods in Psychiatric Research 28 (2):1782. doi:10.1002/mpr.1782.
- Eisenberg, D., M. F. Downs, and E. Golberstein. 2009. Stigma and help seeking for mental health among college students. Medical Care Research and Review 66 (5):522–41. doi:10.1177/1077558709335173.
- Eisenberg, D., E. Golberstein, and S. E. Gollust. 2007. Help-seeking and access to mental health care in a university student population. Medical Care 1 (7):594–601. doi:10.1097/MLR.0b013e31803bb4c1.
- Eisenberg, D., J. Hunt, and N. Speer. 2013. Mental health in American colleges and universities: Variation across student subgroups and across campuses. The Journal of Nervous and Mental Disease 201 (1):60–67. doi:10.1097/NMD.0b013e31827ab077.
- Grant, E., J. Lust, and S. R. Chamberlain. 2019. Problematic smartphone use associated with greater alcohol consumption, mental health issues, poorer academic performance, and impulsivity. Journal of Behavioral Addictions 8 (2):335–42. doi:10.1556/2006.8.2019.32.
- Harrer, M., S. H. Adam, H. Baumeister, P. Cuijpers, E. Karyotaki, R. P. Auerbach, R. C. Kessler, R. Bruffaerts, M. Berking, and D. D. Ebert. 2019. Internet interventions for mental health in university students: A systematic review and meta‐analysis. International Journal of Methods in Psychiatric Research 28 (2):1759. doi:10.1002/mpr.1759.
- Hefner, J., and D. Eisenberg. 2009. Social support and mental health among college students. The American Journal of Orthopsychiatry 79 (4):491–99. doi:10.1037/a0016918.
- Hunt, J., and D. Eisenberg. 2010. Mental health problems and help-seeking behavior among college students. Journal of Adolescent Health 46 (1):3–10. doi:10.1016/j.jadohealth.2009.08.008.
- Kim, P. Y., Kendall, D. L, and H. S. Cheon. 2017. Racial microaggressions, cultural mistrust, and mental health outcomes among asian American college students[J. The American Journal of Orthopsychiatry 87 (6):663. doi:10.1037/ort0000203.
- Kitzrow, A. 2003. The mental health needs of today’s college students: challenges and recommendations. Journal of Student Affairs Research and Practice 41 (1):167–81. doi:10.2202/1949-6605.1310.
- Levecque, K., F. Anseel, A. Beuckelaer, J. Van der Heyden, and L. Gisle. 2017. Work organization and mental health problems in PhD students. Research Policy 46 (4):868–79. doi:10.1016/j.respol.2017.02.008.
- Lin, S., B. Zheng, and G. C. Alexandropoulos. 2020. Adaptive transmission for reconfigurable intelligent surface-assisted OFDM wireless communications[J. IEEE Journal on Selected Areas in Communications 38 (11):2653–65. doi:10.1109/JSAC.2020.3007038.
- Lipson, S. K., Lattie, E. G, and D. Eisenberg. 2019. Increased rates of mental health service utilization by US college students: 10-year population-level trends (2007–2017)[J. Psychiatric Services 70 (1):60–63. doi:10.1176/appi.ps.201800332.
- McGuire, TG, and J. Miranda. 2008. New evidence regarding racial and ethnic disparities in mental health: policy implications. Health Affairs 27 (2):393–403. doi:10.1377/hlthaff.27.2.393.
- Nguyen-Feng, V. N., Greer, C. S, and P. Frazier. 2017. Using online interventions to deliver college student mental health resources: evidence from randomized clinical trials. Psychological Services 14 (4):481. doi:10.1037/ser0000154.
- Pasinringi, M. A. A., Vanessa, A. A, and G. Sandy. 2022. The relationship between social support and mental health degrees in emerging adulthood of students. Golden Ratio of Social Science and Education 2 (1):12–23. doi:10.52970/grsse.v2i1.162.
- Pedrelli, P., M. Nyer, and A. Yeung. 2015. College students: mental health problems and treatment considerations. Academic Psychiatry 39 (5):503–11. doi:10.1007/s40596-014-0205-9.
- Rafal, G., A. Gatto, and R. DeBate. 2018. Mental health literacy, stigma, and help-seeking behaviors among male college students. Journal of American College Health 66 (4):284–91. doi:10.1080/07448481.2018.1434780.
- Selvaraj, R. P., and C. S. Bhat. 2018. Predicting the mental health of college students with psychological capital. Journal of Mental Health 27 (3):279–87. doi:10.1080/09638237.2018.1469738.
- Sharp, J., and S. Theiler. 2018. A review of psychological distress among university students: pervasiveness, implications and potential points of intervention. International Journal for the Advancement of Counselling 40 (3):193–212. doi:10.1007/s10447-018-9321-7.
- Storrie, K., K. Ahern, and A. Tuckett. 2010. A systematic review: students with mental health problems—a growing problem. International Journal of Nursing Practice 16 (1):1–6. doi:10.1111/j.1440-172X.2009.01813.x.
- Tang, W., T. Hu, and L. Yang. 2020. The role of alexithymia in the mental health problems of home-quarantined university students during the COVID-19 pandemic in China. Personality and Individual Differences 165:110131. doi:10.1016/j.paid.2020.110131.
- Zhang, M., J. Zhang, F. Zhang, L. Zhang, and D. Feng. 2018. Prevalence of psychological distress and the effects of resilience and perceived social support among Chinese college students: does gender make a difference. Psychiatry Research 267:409–13. doi:10.1016/j.psychres.2018.06.038.
- Zivin, K., D. Eisenberg, and S. E. Gollust. 2009. Persistence of mental health problems and needs in a college student population. Journal of Affective Disorders 117 (3):180–85. doi:10.1016/j.jad.2009.01.001.