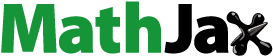
ABSTRACT
This paper aims to introduce a new approach to enhance college English learning through an intelligent method that constructs a learning environment from a demand analysis perspective. The proposed model utilizes a bionic algorithm to structure the algorithm and adopts a highly efficient energy generation method to calculate the interaction energy. This study aims to find the optimal solution to the constraint problem by using a process of continuous generation, expansion, and replacement of magnetosomes. The methodology employed in this research involved constructing the college English learning environment in colleges and universities from a demand analysis perspective. This was achieved by analyzing the needs and requirements of college students and designing an ecological virtual learning environment (EVLE) that meets their demands. The findings of this study indicate that the proposed EVLE for college English is effective in improving the effectiveness of college English learning. The experimental verification results support the effectiveness of the proposed model in creating an interactive and engaging learning environment that facilitates the acquisition of English language skills. It is an innovative approach to college English learning that utilizes intelligent methods to construct a demand-driven learning environment that enhances students’ learning outcomes.
Introduction
At present, while enjoying the fruits of rapid economic and social development, people also appear impetuous and eager for quick success. In this big atmosphere, as members of society, we will be somewhat “indoctrinated” a little bit. At the same time, we are more or less utilitarian when we consider problems, and lack a kind of natural tranquility of humanistic care. It is also very likely to affect family members and school members and indirectly affect the motivation and enthusiasm of students to study, or directly affect the motivation of students to study.
In addition, many things in the family, including family harmony, economic status, learning atmosphere, etc., all affect students’ mood and learning initiative (Yuliansyah and Ayu Citation2021).Whether it is the quality of hardware facilities such as campuses, classrooms, and teaching equipment, or whether the software conditions such as school ethos, teaching staff (Liu, Wang, and Saravanan Citation2021).
At present, from the perspective of educational environment, the school environment is the learning environment in a narrow sense. Education and cultivating talents are not the responsibility of the school, let alone its power. It is necessary to coordinate the forces of schools, families, society and other parties to shape an effective school environment and work together for the healthy and sustainable development of China’s education. When relying on the family, there is nowhere to be scattered. In addition, when relying on social coordination, it is complex and has a large time span. Therefore, only by relying on the platform of the school to lead and shape an effective learning environment, can the functions of “organizing the thread” and “tying the pigtails” be better performed (Apriani and Hidayah Citation2019).
In this regard, the school adopts monthly sampling, annual coverage and event triggering methods to implement it, and focuses on equal communication with parents, so that it can abandon the wrong idea of “sending a child to the school is in a safe, and let them ignore it by themselves” (Godwin-Jones Citation2018). Recognize the importance and rigor of the student learning environment. Encourage them to actively invest in the construction (Pérez-Paredes, Ordoñana Guillamón, and Aguado Jiménez Citation2018).
At the same time, the only-child generation has also made them develop some bad habits such as selfishness and self-righteousness, as well as the temptation of mobile phones, Internet, games, etc (Bin et al. Citation2019). Teachers innovate teaching ideas, teach students in accordance with their aptitude, and at the same time, improve management, communicate with parents in a timely manner, and jointly teach students to jointly guide students to play the positive role of mobile phones (Todd Citation2020).
The learning community formed by different learners according to certain needs is an organic group, and various supportive conditions. All learners interact with various supportive conditions and form an ecosystem with specific functions (AlGhamdi Citation2018). therefore. The virtual learning environment can realize its ecological representation. EVLE refers to a learner-centered environment that can reflect the basic laws and principles of ecology, possess the ability of self-regulation, self-improvement, and self-development. The integration of various factors that directly or indirectly affect a learner’s learning. Like the human ecological environment, the “lack of resources” will cause the “desertification” of the virtual learning environment. “Information pollution” will cause an “ecological crisis” (Xu and Shi Citation2018). The establishment of an effective control mechanism can make the organization more reasonable, the function is more optimized (Toto and Limone Citation2021).
Systematism emphasizes the interrelationship, interaction and functional unity of the elements that make up the system. EVLE is a functional entity formed by the interaction of learners and various supporting conditions. It is the integration of many constituent elements and is systematic. A well-systematic and EVLE. Its various ecological factors are interdependent and conditional on each other, which can realize the unification of functions and functions, and help to optimize the overall function of the system (Meng-Yue, Dan, and Jun Citation2020). For example, in a virtual learning environment. The arrangement of learning resources should be consistent with the design of learning activities, and the selection of learning strategies should be consistent with the design of learning content and activities. In this way, learners can feel smooth and coherent when learning, so as to absorb knowledge to the greatest extent and construct an understanding of the meaning of knowledge (Rinantanti, Bin-Tahir, and Suriaman Citation2019).
Balance refers to the ecological balance of the virtual learning environment. That is, a state in which the learners and the virtual learning environment and the ecological factors in the learning environment are highly adapted and coordinated in all aspects within a certain period of time. At this time, the internal structure and external functions of the virtual learning environment are relatively stable. An excellent EVLE should have a good balance. Thus, the resource advantage in the network learning environment can be maximized (Shadiev and Yang Citation2020). The ecological balance of virtual learning environment is a dynamic balance, and the influence of various internal and external factors may break this balance. Cause the ecological imbalance of the virtual learning environment, and even the outbreak of ecological crisis. For example, in a virtual learning environment. The number of learners and facilitators should always be kept in a certain proportion, so as to achieve a balance between the instructional needs of the learners and the instructional ability of the facilitators. At this time, the learning effect of learners and the utilization of human resources will be optimized. Once this balance is broken, the ecological imbalance of the virtual learning environment will be caused, resulting in a decline in the learning effect of learners or waste of human resources (Khasawneh Citation2021).
EVLE is a subset of human social ecological environment. At the same time, it is the product of a very open network virtual world, so it is not a closed system. It is open and requires constant exchange of matter, energy and information with the outside world (Hollenstein et al. Citation2018).
Self-organization is the ability of self-regulation, self-improvement and self-development of the system itself. In the EVLE, the formation of its self-organization ability should be realized through the design of the core monitoring and adjustment mechanism, which can be monitored manually or by artificial intelligence. E.g. When the system finds that the learner encounters learning difficulties through monitoring. The cause of the problem can be analyzed by a diagnostic system is the lack of learning resources. There is also a problem with teaching strategies. Or learning activities are not properly arranged: and then take action based on the analysis results. Or adjust the learning content, or change the teaching strategy. Or provide learners with individualized guidance and assistance, and ultimately solve problems and improve the virtual learning environment (Xiao-Dong and Hong-Hui Citation2020).
The proposed method in this article presents several novel contributions to the field of college English learning. Firstly, the method utilizes a bionic algorithm inspired by, which has not been previously applied in the context of language learning. This algorithm is highly efficient in generating optimal solutions to complex constraint problems.
Secondly, the proposed model employs a demand analysis perspective to construct the college English learning environment. This perspective emphasizes the importance of meeting the specific needs and requirements of college students, which can vary greatly depending on factors such as their level of English proficiency, learning styles, and cultural backgrounds.
Thirdly, the model uses an ecological virtual learning environment (EVLE), which integrates various digital tools and resources to create an interactive and engaging learning environment. The EVLE allows for personalized learning experiences that can be adapted to individual student needs and preferences.
The added value of this article lies in its contribution to the field of college English learning by introducing a new approach that leverages intelligent methods, demand analysis, and technology to enhance learning outcomes. The experimental verification results provide evidence of the effectiveness of the proposed EVLE for college English, which can be applied in various educational settings to improve language learning. Overall, this article provides a valuable contribution to the field and offers new insights into the design and implementation of effective language learning environments.
Model Algorithm
Bacterial Bionic Algorithm
Among the various forms of, the most special form exists in multicellular aggregates. Through further study on the morphological structure of such magnetotactic microorganisms, they are called multicellular magnetotactic prokaryotes. Most of the MMPs currently found in appearance are round. The number of magnetosomes contained in each cell of multicellular magnetotactic prokaryotes is as high as 50, and they mainly exist in the form of iron sulfide type single magnetic domain crystals. They are distributed at the cell edge in planar arrays of two to five strands in the cytoplasm in a planar population and according to certain rules, as shown in .
If the remanent magnetic moment carried by each bacterial cell i is denoted as . In magnetism, the ratio of remanence at saturation magnetization is often used to characterize the magnetic domain state of particles. Here, we have
If the degree of magnetic optimization is denoted as DMO, we have
Formula (2) is a normalization process that represents the maximum dipole used under the constraint that the chain shaft is fixed in space. The numerator term represents the actual contribution of each dipole to the generation of the residual magnetic moment
, that is, the actual dipole used to generate the residual magnetic moment
.
Each magnetosome chain is regarded as an infinitely long cylinder in the magnetic field B, then the model used to estimate the interaction force between two parallel bacterial cells is shown in . Among them, H represents the distance from one cylinder surface to another cylinder surface, and D represents the diameter of the cylinder. In this way, the energy stored by the can be estimated according to formula (3).
The Basic Principle of the Optimization Algorithm of Magnetic Bacteria
We assume that the interaction energy between two cells in multicellular magnetotactic prokaryotes can be calculated as:
Among them, n and m are the number of magnetosomes in the two cells, respectively, that is, n=m, when the energies of the two selected cells are also equal, that is, there is , there is
According to formula (3), the energy of each cell can be estimated. If the orientation of the magnetic field is ignored, there is
Based on the above description, the following definitions are made for the optimization algorithm of magnetic bacteria:
Definition 1:
If L is used to represent a feasible solution of the optimization problem to be solved, then bacterial cell is an eigenvector of L, and X is a set of feasible solutions.
Definition 2:
The is composed of a group of cells, and is regarded as one of the cell populations, that is, the feasible solution of the problem to be optimized, and N is the size of the cell population.
Definition 3:
The function represents the distance between two cells in the MMP, the function
of the distance D represents the interaction energy between the cells in the MMP, and the moment M is generated by the interaction between the cells and the magnetic field.
Definition 4:
For the search and optimization process of the solution of the optimization problem to be sought, we can use a ternary function to represent it, which can be expressed in the following form:
Definition 5:
The magnetic bacteria optimization algorithm is an optimization algorithm based on the torque principle of the magnetosome to find the optimal solution of the problem, and is ternary. Among them,
is the function of generating the cell population and calculating the corresponding cost J, and
is the termination condition.
Based on the previous description, it can be concluded that the magnetic bacteria optimization algorithm generally includes the following five processes:
Initialization
The initial population of the magnetic bacteria optimization algorithm consists of n randomly generated L-dimensional real vectors. We can let (among them, there is
) represent bacterial cells of the i-th generation. The way of random generation is as expressed in formula (9)
Among them, and
are the upper and lower bounds of randomly generated bacteria
, respectively, and rand is a random value in (0,1).
Calculation of the Interaction Energy Between Cells
Before the interaction between bacterial cells can be calculated, the distance between cells is defined as follows: First, two bacterial cells are randomly selected from the generated population and denoted as respectively. Among them, there is
. D is defined as the distance between two bacterial cells and it is calculated as follows
Among them, is the Euclidean length of two bacterial cells.
The interaction energy produced between bacterial cells can be calculated according to the following formula
in the formula represents the difference between
and
in bacterial cell
, and
and
are both constants.
The Production of Magnetosomes
Here, for the convenience of analysis, a simplification work is to be done. If it is assumed that the overall moment of the magnetosome is parallel to the generated magnetic field, there is . According to the above equation
, that is
. The formula for calculating the torque can be obtained as follows
If the total moment is assumed to be , the moment of the magnetosome can be specified as follows
Among them, p and q are randomly selected from sets and
, respectively, and rand is a random value in (0,1).
Magnetosome Scale Expansion
After the magnetosomes in bacteria are produced, they will expand in scale in the following way: We set the magnetic field strength B = 3. If there is rand>0.5, the magnetosomes in bacteria will scale up in the following way
Otherwise, the way it expands is
Among them, is a randomly generated L-dimensional vector, and each dimension value of it is between 0 and 1.
Replacement of Magnetosomes
After the magnetosome is expanded in scale, it is possible to produce some magnetosomes with better torque than before the expansion. Based on the principle of optimization, some previous magnetosomes with less torque will be replaced by these magnetosomes with better torque. The change of the magnetosome moment follows the following form
Among them, p and q are randomly selected from sets and
respectively,
is a randomly generated L-dimensional vector and the value of each dimension is between 0 and 1.
By comparing the steps of the above magnetic bacteria optimization algorithm, we can obtain the flow chart of the magnetic bacteria optimization algorithm shown in .
The evolution strategy chooses the form of decimal real numbers to describe the problem. Correspondingly, the mutation operation can be divided into two forms: binary and ternary:
1) Binary expression. In this method, the individual consists of two aspects: one is the target variable part X, the other is the standard deviation part , both of which include n components:
The relationship between X and is:
Among them, is the global coefficient, which is often taken as 1, and the random values N(0,1) and
both obey the standard normal distribution, but the latter is generated for the i-th component.
2) Ternary expression. This method incorporates a factor called the coordinate rotation angle based on the binary representation to improve the convergence rate of the evolution strategy:
The relationship between and
is:
Among them, is the rotation angle between the components of the i-axis and j-axis of the parent individual, which reflects their covariance.
is the rotation angle of the offspring individuals between the components of the i-axis and the j-axis,
is a constant coefficient, and
depends on the normally distributed random numbers of
and
.
Since the global optimum can be achieved when the initial step size of is close to 0, in order to move better in the search space, the initial step size of each individual in the initial population is initialized according to the following formula
In the formula, n is the number of decision variables, is the upper and lower boundaries of decision variable i, and
is an approximate value. The flowchart of the simple multivariate evolution strategy is shown in .
If the size of the group is set to be represents the fitness of
, the probability of
being selected is:
The flowchart of the genetic algorithm is shown in .
Differential Evolution (DE) is based on differential calculation to carry out relatively easy mutation. It chooses a point-to-point competition method, and can dynamically track the optimization situation by relying on its unique memory ability to adjust the optimization method at any time. Similar to the steps of other evolutionary methods, it is also divided into three operations: mutation, crossover and selection.
(1) Each will create a mutant individual
in the following way,
Among them, NP is the population size, N is the spatial dimension, and
are the current population and the
-th individual in the population, respectively. Meanwhile,
and
are three individuals randomly selected from the population and have
is a variation factor between 0 and 2.
(2) The parent individual and the variant individual
can be crossed to obtain the test individual
according to the following methods:
The setting of the integer j_rand in [1,N] makes the test individual get at least one element from the mutant individual.
(3) Differential evolution adopts the greedy selection strategy of selecting an individual with the best fitness value from all parent individuals and test individuals to enter the next generation, and the fitness function is recorded as fitness
, then there are
The flowchart of the differential evolution algorithm is shown in .
Particle Swarm Optimization (PSO) is a process of simulating the process of bird flock searching for the best position by repeatedly iterating the process of obtaining the optimal particle solution.
We might as well assume that the “solution space” is an N-dimensional search space.The best places currently found by the ith particle and the entire population are and
, respectively. If there is
at time t, there is
such that
, and there is
, and at time
, there is
When there is , there is
The optimization methods involved in the comparison in this paper are all standard optimization methods that have not been improved.
The flow chart of the particle swarm algorithm is shown in .
Construction of College English Learning Environment in Colleges and Universities from the Perspective of Demand Analysis
From an ecological point of view. The virtual learning environment is a learner-centered, complex functional entity composed of physical environment, social environment and normative environment. The three types of environments are used as ecological factors in the system. Interdependence and mutual influence together constitute an ecological virtual learning environment, as shown in . The ecological virtual learning environment should have the functions of self-regulation, self-improvement and self-development. As the ecological factors in the system, the three types of environments are interdependent and influence each other to form an EVLE, as shown in .
The EVLE model is shown in .
The effect of the EVLE of college English proposed in this paper is verified, and the promotion effect of the learning environment proposed in this paper on the learning effect of college English in colleges and universities is calculated, and the statistics are obtained as shown in .
Table 1. Verification of the effect of EVLE of college English.
It can be seen from the experimental verification that the EVLE of college English proposed in this paper can effectively improve the effect of college English learning.
Conclusion
The learning space in a smart learning environment is a cutting-edge approach to education that offers a flexible and personalized learning experience. It is designed to enable learners to access learning materials anytime, anywhere, and through any device, making education accessible to all. The smart learning environment utilizes intelligent perception of learning situations, data mining, and analysis to provide tailored recommendations for high-quality learning resources and tasks.
The smart learning environment is more than just a virtual learning platform. It is an innovative learning field that seamlessly integrates physical and virtual environments to create a more immersive and effective learning experience. The environment utilizes a range of advanced technologies such as sensors, artificial intelligence, and big data analytics to perceive learning situations and identify learner characteristics. It provides a range of learning resources, tools, and interactive features that enable learners to engage with the material in a meaningful way.
This paper focuses on the development of a college English learning environment, which has been constructed using intelligent methods to meet the specific needs of college English learners. The paper provides a detailed analysis of the demands of college English learners and identifies the key features required to enhance their learning experience. The proposed Environment for Virtual Learning English (EVLE) was designed to incorporate these features and provide an effective and personalized learning experience for college English learners.
The effectiveness of the proposed EVLE was evaluated through experimental verification, which demonstrated that it can significantly improve the learning outcomes of college English learners. The personalized learning environment created by the EVLE enhances the learner’s engagement, motivation, and retention of the material, resulting in more successful language acquisition. The results of this study highlight the importance of smart learning environments and the potential they offer for the future of education.
There are several potential future directions for the development of smart learning environments that could significantly enhance the learning experience for students. Some of these directions include:
Integration of Augmented Reality and Virtual Reality: Smart learning environments could incorporate augmented reality and virtual reality technologies to create more immersive and engaging learning experiences. Learners could interact with 3D models, simulations, and virtual environments, making learning more interactive and exciting.
Gamification of Learning: Gamification is the use of game elements in non-game contexts, such as education. Smart learning environments could utilize gamification techniques to enhance student motivation and engagement. This could involve incorporating game elements such as points, badges, and leaderboards into the learning environment.
Personalized Learning: Smart learning environments could use advanced algorithms to create a personalized learning experience for each student. These algorithms could analyze student performance data to identify individual learning needs, and provide tailored learning resources and feedback.
Collaborative Learning: Smart learning environments could facilitate collaborative learning experiences among students by incorporating tools for online collaboration, such as discussion forums and video conferencing. This could create a more social and interactive learning environment, allowing students to learn from one another.
Adaptive Learning: Adaptive learning involves using algorithms to adjust the learning experience based on student performance. Smart learning environments could incorporate adaptive learning techniques to provide students with personalized challenges and support based on their individual learning needs.
Overall, the future of smart learning environments is exciting, and there are many opportunities to enhance the learning experience for students through the integration of advanced technologies and personalized approaches to education.
Disclosure Statement
No potential conflict of interest was reported by the author(s).
Data Availability Statement
Data will be provided upon request to authors.
Additional information
Funding
References
- AlGhamdi, M. A. 2018. Arabic learners’ preferences for Instagram English lessons. English Language Teaching 11 (8):103–1441. doi:10.5539/elt.v11n8p103.
- Apriani, E., and J. Hidayah. 2019. The ICT used by the English lecturers for non English study program students at STAIN curup. Vision: Journal of Language and Foreign Language Learning 8 (01):26–37. doi:10.21580/vjv8i13280.
- Bin, Y., D. Mandal, A. Farouk, and D. Zhen. 2019. English teaching practice based on artificial intelligence technology. Journal of Intelligent & Fuzzy Systems 37 (3):3381–91. doi:10.3233/JIFS-179141.
- Godwin-Jones, R. 2018. Second language writing online: An update. Language Learning & Technology 22 (1):1–15.
- Hollenstein, N., J. Rotsztejn, M. Troendle, A. Pedroni, C. Zhang, and N. Langer. 2018. ZuCo, a simultaneous EEG and eye-tracking resource for natural sentence reading. Scientific Data 5 (1):1–13. doi:10.1038/sdata.2018.291.
- Khasawneh, M. A. S. 2021. An electronic training program on developing the written expression skills among a sample of foreign language learners EFL who are at-risk for learning disabilities during the emerging covid-19. Academy of Social Science Journal 7 (10):1974–82.
- Liu, S., J. Wang, and V. Saravanan. 2021. Ice and snow talent training based on construction and analysis of artificial intelligence education informatization teaching model. Journal of Intelligent & Fuzzy Systems 40 (2):3421–31. doi:10.3233/JIFS-189380.
- Meng-Yue, C., L. Dan, and W. Jun. 2020. A study of college English culture intelligence-aided teaching system and teaching pattern. English Language Teaching 13 (3):77–83. doi:10.5539/elt.v13n3p77.
- Pérez-Paredes, P., C. Ordoñana Guillamón, and P. Aguado Jiménez. 2018. Language teachers’ perceptions on the use of OER language processing technologies in MALL. Computer Assisted Language Learning 31 (5–6):522–45. doi:10.1080/09588221.2017.1418754.
- Rinantanti, Y., S. Z. Bin-Tahir, and A. Suriaman. 2019. The impact of EFL senior high school teachers’ performance in papua. Indonesia Toward the Students’ English Learning Achievement, Asian EFL Journal 23 (3.3):431–47.
- Shadiev, R., and M. Yang. 2020. Review of studies on technology-enhanced language learning and teaching. Sustainability 12 (2):524–32. doi:10.3390/su12020524.
- Todd, R. W. 2020. Teachers’ perceptions of the shift from the classroom to online teaching. International Journal of TESOL Studies 2 (2):4–16.
- Toto, G. A., and P. Limone. 2021. Motivation, stress and impact of online teaching on Italian teachers during COVID-19. Computers 10 (6):75–94. doi:10.3390/computers10060075.
- Xiao-Dong, L., and C. Hong-Hui. 2020. Research on VR-Supported flipped classroom based on blended learning—a case study in “learning English through news. International Journal of Information and Education Technology 10 (2):104–09. doi:10.18178/ijiet.2020.10.2.1347.
- Xu, Z., and Y. Shi. 2018. Application of constructivist theory in flipped classroom-take college English teaching as a case study. Theory and Practice in Language Studies 8 (7):880–87. doi:10.17507/tpls.0807.21.
- Yuliansyah, A., and M. Ayu. 2021. The implementation of project-based assignment in online learning during covid-19. Journal of English Language Teaching and Learning 2 (1):32–38. doi:10.33365/jeltl.v2i1.851.