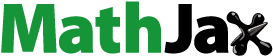
ABSTRACT
At a new historical crossroads, China’s Belt and Road Initiative (BRI) is a major measure to coordinate sustained economic growth at home, maintain a peaceful and stable environment in the surrounding region, and foster regional economic and commercial cooperation in the region. The BRI, a major initiative, can not only drive national economic growth but also has far-reaching significance for promoting world economic prosperity and peace. The national plan clearly pointed out that in the face of the new international pattern and situation, China should improve its new opening-up system and promote construction for the BRI in the future. In this context, research on the benefits and security issues for foreign investment under the BRI has become an important topic. This work combines it with artificial intelligence to propose a neural network (IPSO-DBN) for evaluating the effectiveness and safety of Chinese outbound investment. First, this work applies DBN to evaluate the benefits and security for outbound investment under the BRI. This work introduces a policy of linearly decreasing weights and an adaptive learning factor into the PSO algorithm to construct IPSO. Then, the IPSO is utilized to optimize initial weights for DBN, and the problem of initial weights are random and easy to fall local optimal solution is improved to construct IPSO-DBN. Second, this work puts forward a series of suggestions for Chinese enterprises to invest abroad in response to the current investment problems under the BRI Third, this work has carried out various experiments on IPSO-DBN and Chinese enterprises’ foreign investment proposals, and the experimental results have verified the feasibility of the method designed in this work.
1. Introduction
The Belt and Road development strategy was proposed in 2013 and has received extensive attention from the international community. In 2015, a document on promoting the joint construction of the Silk Road in the new century was jointly issued by multiple departments in the country, and the Belt and Road strategy was officially implemented. Many countries in the world have actively responded and participated in the Belt and Road Initiative (BRI) construction. With the help of multi-level, multi-channel, and extensive communication, consultation, and cooperation, the Belt and Road promotes further development for regional economy and actively strengthens exchanges with countries. This peacefully resolves frictions between countries in trade and investment activities and minimizes barriers to trade and investment. This creates a favorable environment for regional economic development and friendly exchanges between countries. This will strengthen cooperation in the financial field between countries, build a more stable Asian currency system, and improve the investment and financing financial system and credit system. This creates a favorable environment for investment activities between countries, eliminates investment obstacles, and fully protects the legitimate rights and interests of foreign investors (Ascensão et al. Citation2018; Hughes et al. Citation2020; Maçães Citation2021; Teo et al. Citation2019; Wang et al. Citation2021).
Driven by the Belt and Road strategy, foreign investment has ushered in new development opportunities. This has created a good opportunity for international economic cooperation and promoted the improvement of foreign investment level. The amount of China’s foreign investment has also continued to rise, ranking among the top countries in foreign investment output. China continues to deepen reform and open up, and the investment cooperation with countries is further deepened. The scope for cooperation was further extended, and the results of joint construction were shared in a wider area. Foreign investment projects are constantly increasing, and investment methods are constantly innovating with different investment projects. The number of overseas economic and trade cooperation has increased year by year, making investment more convenient, and foreign direct investment continues to grow rapidly. Under the guidance of the construction of an open economic system, especially the BRI, investment between countries will be more in-depth, and foreign investment will continue to develop steadily. Chinese enterprises are actively responding to complex as well as changing international market environment, accelerating pace of internationalization, and integrating into the process of economic globalization. Chinese enterprises seek mutual benefit and win–win results by means of foreign direct investment, international cooperation in labor services, and extensive establishment of international economic cooperation zones (Coenen et al. Citation2021; Cuiyun and Chazhong Citation2020; Han et al. Citation2020; Hurley, Morris, and Portelance Citation2019; Liu, Zhang, and Xiong Citation2020).
In recent years, the proportion of non-financial investment in China’s foreign investment has expanded, the industry structure of foreign investment has become increasingly reasonable, and the structure of investment entities has continued to be optimized. Looking at the domestic and international environment, China’s foreign investment and cooperation are still in a period of important strategic opportunities. At the critical moment when the traditional growth engine’s role in driving the economy is weakening and new economic growth drivers are being formed, there are still many risks of Chinese enterprises’ foreign investment, and security issues still need to be strengthened. In the environment of a strengthening trend of international economic integration, the foreign investment exchanges between countries have been strengthened, and the risks faced by foreign investment have become more prominent. Enterprises going out to invest abroad are affected by various security factors, which together affect the daily operation of enterprises. In the context of the in-depth implementation of the Belt and Road, the growth rate and scale of foreign investment have continued to increase. However, in recent years, the global political and economic environment has fluctuated, and the security risks faced by Chinese foreign investors in the process of foreign investment have become increasingly prominent. The main security risks faced by Chinese enterprises in the process of foreign investment include political risks, legal risks, social risks, and cultural risks (Hashemizadeh et al. Citation2021; Huang Citation2019; Hussain et al. Citation2020; Sun et al. Citation2020; Yuan et al. Citation2019).
In China’s foreign investment, investment income is also an important content. However, the feasibility study of foreign investment is insufficient, resulting in low investment returns. Enterprise capital operation is a huge multi-professional and interdisciplinary system engineering, and its operation process is complex, cumbersome, and highly specialized. Before some enterprises make foreign investment, the depth of feasibility study and demonstration is not enough, and they do not pay attention to the comparison and selection of multiple schemes and project risk analysis. Some enterprises even beautify the project investment feasibility report in order to promote a certain project investment, resulting in investment loss or invalid investment, which increases the opportunity cost of the enterprise. In addition, foreign investment lacks a unified development strategy and investment direction. Some enterprises have two situations in the selection of foreign investment projects. First, the investment direction of the project is not in line with the strategic development goals of the company, and it lacks overall, long-term, hierarchical, and synergistic properties. They cannot take into account the short-term and long-term interests of the company. Second, the investment direction of the project deviates from the core business of the enterprise, which is not conducive to the formation of economies of scale and the enhancement of market competitiveness. Finally, the internal control system for foreign investment is not perfect. Some enterprises have not yet established a comprehensive and complete foreign investment management system, and after the implementation of foreign investment projects, they are simply included in the financial accounting for investment accounting. They lack a set of scientific and operable investment project evaluation systems and methods. This is not conducive to the management of enterprises to grasp the benefits of each foreign investment project in a timely manner (Abban, Jiying, and Adjei Mensah Citation2020; Ahmad et al. Citation2020; Khan et al. Citation2020; Mahadevan and Sun Citation2020; Sun et al. Citation2020).
In this context, research on the benefits and security issues of China’s foreign investment in the context of the BRI has become an important topic. This work combines it with artificial intelligence to propose IPSO-DBN for evaluating the effectiveness and safety of Chinese outbound investment. First, this work applies DBN to evaluate the benefits and security for outbound investment under the BRI. This work introduces a policy of linearly decreasing weights and an adaptive learning factor into the PSO algorithm to construct IPSO. Then, the IPSO is utilized to optimize initial weights for DBN, and the problem of initial weights are random and easy to fall local optimal solution is improved to construct IPSO-DBN. Second, this work puts forward a series of suggestions for Chinese enterprises to invest abroad in response to the current investment problems under the BRI. Third, this work has carried out various experiments on IPSO-DBN and Chinese enterprises’ foreign investment proposals, and the experimental results have verified the feasibility of the method designed in this work.
2. Related Work
Huang, Fischer, and Xu (Citation2017) conducted research on the social responsibility of multinational enterprises’ overseas investment in the BRI. It believed that enterprises should not only focus on their own benefits but also focus on common development with countries along the route. This was a practical method to reduce overseas investment risks and enhance the corporate image of overseas investment enterprises. It could promote orderly economic development and rational allocation of resources. This technique, according to the literature (TANG et al. Citation2015), was critical to China’s international direct investment pattern, industrial structure improvement, and economic development. Large markets and plentiful resources along the Belt and Road had created enormous opportunities for economic and commercial cooperation between countries. This provided objective conditions for the redeployment of China’s foreign direct investment pattern, which could break the long-standing situation of unbalanced investment development and serious loss of efficiency.
Chan (Citation2017) analyzed the motivation for direct investment in regions along the route. This believed that the demographic dividend and resource advantages of the countries made cost of production factors cheap, and they showed obvious market-seeking and natural-resource-seeking motives in the investment process. This promoted China’s economic development while improving the efficiency of resource allocation in the host country. This proved that the Belt and Road was a win–win economic model for both investors and even neighboring countries. Qian and Fulton (Citation2017) divided the countries along the route into different regions from the perspective of geospatial. It conducted an in-depth analysis from the aspects of total foreign investment, growth rate, flow, and stock. It is believed that the key factor affecting direct investment was market size of host country.
ZiroMwatela and Changfeng (Citation2016) analyzed direct investment flows in various regions. The development for the Belt and Road had achieved steady development from speeding up planning to promoting international cooperation. China’s direct investment had achieved breakthrough development from high-speed growth to focusing on structure, but the focus of investment was still unstable. In the future, direct investment along the route should focus on balanced development, and focus on the optimization of investment structure while maintaining the growth of investment scale. As China’s industrial structure improved and its economy grows, Fardella and Prodi (Citation2017) had dialectically discussed the current direct investment conditions in nations along the Belt and Road. In addition, as economic and commercial cooperation along the Belt and Road route has progressed, the investment climate in many developing nations had improved. This provided the possibility to further expand investment, which in turn was conducive to promoting domestic enterprises to enter the international market.
Zhang and Doreen (Citation2017) believed that the rapid growth of Chinese enterprises’ foreign investment in recent years largely stemmed from the actual needs of the domestic situation and benefited from government policies and strategic deployments. At the same time, Chinese enterprises’ foreign investment was mainly distributed in countries and regions with relatively good economic development and social and political stability, and the scale of investment in countries along the route was relatively small. In the future, the investment direction could choose labor-intensive industries as a breakthrough.
Gabusi (Citation2017) studied the current situation and challenges of overseas investment. The direct investment of Chinese multinational enterprises was developing rapidly, and private enterprises were becoming more and more active in cross-border investment. However, due to lack of experience, unreasonable investment layout, and high potential risks, enterprises might face new bottlenecks in overseas investment. Under the new normal, enterprises should pay attention to the diversification of industries and the innovation of investment methods when making foreign direct investment.
Pencea (Citation2017) analyzed the international risks faced by direct investment along the Belt and Road. Political stability and level for government intervention in the market might exacerbate OFDI risks. The degree of market freedom and financial market openness created uncertainty. Yu (Citation2017) analyzed the stock of direct investment and studied the influencing factors and investment efficiency of direct investment. It made targeted recommendations on characteristics, such as overall performance, efficiency gaps, and changing trends.
Cheng, Song, and Huang (Citation2017) took the production capacity investment cooperation efficiency of developing countries and emerging countries. It analyzed the efficiency of China’s capacity cooperation with OFDI in emerging and developing countries. The study found that reverse efficiency spillovers had typical single-threshold nonlinear characteristics and significant positive effects, and China as a whole has entered the high-efficiency spillover stage of cross-border production capacity cooperation.
Mitić (Citation2017) introduced a stochastic frontier gravity model when studying the efficiency of foreign direct investment. It longitudinally analyzed changing trend of direct investment efficiency since the BRI was proposed and horizontally compared regional differences in investment efficiency. It believed that China’s foreign direct investment had problems, such as serious efficiency loss and uneven development. Jianping and Chenlin (Citation2017) explored the efficiency of China’s manufacturing overseas direct investment with global value-chain background.
Since the BRI was put forward, the technical efficiency of manufacturing foreign investment was continuously improved. There are obvious differences in the efficiency of investment in different countries, and at the same time, there were obvious differences in the investment efficiency of foreign investment enterprises of different ownerships.
This work makes a significant contribution by introducing a novel approach to evaluating the effectiveness and safety of Chinese outbound investment under the BRI through the integration of artificial intelligence techniques. Specifically, a neural network called IPSO-DBN is proposed, which combines the PSO algorithm with a deep belief network (DBN).
To address the issue of random initial weights and the tendency to fall into local optimal solutions, this work incorporates a policy of linearly decreasing weights and an adaptive learning factor into the PSO algorithm, resulting in the creation of IPSO. By utilizing IPSO, the initial weights for the DBN are optimized, effectively improving the problem of suboptimal solutions and enhancing the accuracy of the evaluation process. This unique integration of IPSO and DBN forms the foundation of the IPSO-DBN framework.
In addition to the evaluation aspect, this work also contributes by providing a series of valuable suggestions for Chinese enterprises to navigate the current investment problems encountered under the BRI. These recommendations are derived from the insights gained through the evaluation process using IPSO-DBN and aim to enhance the decision-making process for Chinese enterprises investing abroad.
To validate the proposed method, various experiments have been conducted on IPSO-DBN using Chinese enterprises’ foreign investment proposals. The experimental results affirm the feasibility and effectiveness of the developed method. This empirical validation serves as a significant contribution, providing evidence of the utility and reliability of the IPSO-DBN approach in evaluating and improving Chinese outbound investments within the BRI.
In summary, this work introduces the innovative IPSO-DBN framework for evaluating the effectiveness and safety of Chinese outbound investment under the BRI. Additionally, it offers practical suggestions to address investment problems specific to the BRI. The conducted experiments provide empirical evidence supporting the feasibility and effectiveness of the proposed method. Together, these contributions advance the understanding and implementation of successful outbound investments within the BRI.
The unique contribution of this work lies in the integration of artificial intelligence techniques, specifically, the IPSO-DBN neural network, to evaluate the effectiveness and safety of Chinese outbound investment under the BRI. This combination of algorithms and methodologies is novel and has the potential to provide valuable insights and improvements in evaluating the benefits and security issues associated with BRI investments.
By utilizing the IPSO algorithm to optimize the initial weights of the DBN, we aim to overcome the limitations of random initial weights and local optimal solutions commonly encountered in traditional DBN models. This innovative approach can lead to more accurate evaluations of outbound investments, assisting Chinese enterprises in making informed decisions and mitigating risks.
Additionally, your proposal includes the development of specific recommendations for Chinese enterprises to address investment problems under the BRI. These suggestions, derived from the insights gained through the evaluation process using IPSO-DBN, offer practical guidance to enhance the effectiveness and success of Chinese investments abroad.
Furthermore, the experimental results obtained from applying IPSO-DBN to Chinese enterprises’ foreign investment proposals serve as empirical evidence, validating the feasibility and efficacy of the proposed method. This empirical validation contributes to the body of knowledge on evaluating BRI investments and demonstrates the potential of AI-driven approaches in addressing complex economic and security issues.
In summary, the unique contribution of our work lies in the novel integration of IPSO and DBN, the development of recommendations for investment problems under the BRI and the empirical validation of the proposed method. These elements collectively advance the understanding and implementation of effective and safe outbound investments within the framework of the BRI.
3. Method
First, this work applies DBN to evaluate the benefits and security for outbound investment under the BRI. This work introduces a policy of linearly decreasing weights and an adaptive learning factor into the PSO algorithm to construct IPSO. Then, the IPSO is utilized to optimize initial weights for DBN, and the problem that initial weights are random and easy to fall local optimal solution is improved to construct IPSO-DBN. Second, this work puts forward a series of suggestions for Chinese enterprises to invest abroad in response to the current investment problems under the BRI.
3.1. DBN Algorithm
Deep belief network is an efficient deep learning algorithm, which can extract and mine the deep features of data, and is suitable for solving nonlinear and complex data problems. The schematic structure is illustrated in .
Visible, hidden, and multiple buried levels comprise a deep belief network. It is possible to think of the DBN network as a stack of RBMs, where the output of the previous RBM is utilized as the input to the following RBM. RBM is a random neural network that can learn probability distributions by analyzing input data. The model structure is demonstrated in .
It has a visible and a buried layer of neurons. The hidden layer and the visible layer are linked symmetrically in two directions, although the adjacent neurons in the layer are not coupled.
RBM is a probability model based on energy function. It is necessary to define the energy function of RBM first and then introduce the corresponding distribution function.
where and
are bias.
The joint probability distribution is:
where is energy.
The probability function of the neurons in the visible layer and the hidden layer is:
where is sigmoid function.
DBN training can be separated into two stages: the pre-training stage, followed by the reverse fine-tuning step. Layer-by-layer greedy learning is used to train each RBM in the network in the pre-training step. An RBM’s hidden layer output is fed into the RBM that follows it as an input. The network’s startup is made possible by the layer-by-layer transmission of data feature information. After the pre-training is completed, the reverse fine-tuning stage is entered because each weight cannot be guaranteed to be optimal. This process is similar to the BP algorithm, adjusting until the error is less than a pre-set value or the highest number of iterations reached.
Choosing the number of neurons in the hidden layers is an important decision that can impact the model’s performance. While there is no definitive rule for determining the exact number of neurons, here are some common approaches and considerations:
Rule of Thumb: A commonly used rule of thumb is to set the number of neurons in the hidden layer(s) to be between the number of input neurons and the number of output neurons. For example, if you have 10 input neurons and 5 output neurons, you might choose to have a hidden layer with a number of neurons ranging from 5 to 10. However, this rule is not strict, and the optimal number may vary depending on the specific problem and dataset.
Model Complexity: The complexity of the problem and the amount of available data can also guide your decision. More complex problems may require larger hidden layers with a higher number of neurons to capture intricate patterns and relationships. Conversely, simpler problems may benefit from smaller hidden layers with fewer neurons to avoid overfitting.
Overfitting and Underfitting: It is important to strike a balance between model complexity and generalization. If the model has too few neurons in the hidden layer(s), it may underfit the data and have limited capacity to learn complex patterns. On the other hand, if the model has too many neurons, it may overfit the data, capturing noise and irrelevant patterns. Regularization techniques like dropout and weight decay can help mitigate overfitting.
Experimentation and Validation: It is often necessary to experiment with different configurations and assess their performance using validation techniques. You can start with a small number of neurons and gradually increase the complexity until the model’s performance plateaus or starts to degrade on a validation set. This process helps determine an optimal number of neurons that achieves good generalization without overfitting.
Domain Knowledge: Knowledge about the specific problem domain can guide the selection of the number of neurons. For instance, if you have prior knowledge about the complexity of the relationships in the data or known characteristics of the problem, you can use that information to inform your decision.
Selecting the number of neurons is not a strict science, and it often involves an element of trial and error. It considers multiple factors, experiments with different configurations, and evaluates the model’s performance to arrive at an appropriate number of neurons in the hidden layers for the specific problem.
On the other hand, the optimal network topology depends on various factors, including the problem’s complexity, the dataset’s size and nature, the availability of computational resources, and other specific requirements of your task. Finding the best architecture for a given problem often requires experimentation, validation, and fine-tuning to find the best architecture for a given problem.
To determine the optimal network topology for prediction, the following steps were implemented:
Understand the problem: Gain a thorough understanding of the prediction problem, the input features, and the expected output. It identifies any specific characteristics or patterns that may influence the choice of network topology.
Start with simple architectures: Begin with simpler network topologies, such as a single hidden layer with a few neurons, and gradually increase the complexity as needed. This allows for establishing baseline performance and assessing the impact of additional layers or neurons.
Experiment with different topologies: Explore a range of network topologies by varying the number of hidden layers, neurons per layer, and activation functions. Were made systematically tested in different configurations and evaluated the performance using appropriate validation techniques (e.g., cross-validation).
Regularization techniques: Incorporated regularization techniques, such as dropout, weight decay, or early stopping, to prevent overfitting and improve generalization performance. These techniques help control the complexity of the model and avoid excessive memorization of the training data.
Validation and evaluation: Used appropriate validation metrics and techniques to assess the performance of different network topologies. This includes evaluating metrics such as accuracy, precision, recall, or mean squared error, depending on the nature of the specific prediction task.
Iterative refinement: Based on the evaluation results, iterated and refined the best network topology by adjusting the number of layers, neurons, and other hyperparameters. Continuously monitored the performance of validation data and made adjustments accordingly.
It is important to note that the optimal network topology depends on the specific problem and the data at hand. Therefore, several experiments with different topologies were made to achieve the best predictive performance for the specific task.
3.2. Improved PSO Algorithm
When DBN is initialized, the initial weights are determined by a random assignment. This will make the model training time longer and the prediction accuracy not enough. Aiming at this issue, this work chooses an improved PSO algorithm to optimize the initial value for DBN. The PSO algorithm is an abstraction of the predation behavior of birds. A flock of birds is looking for a certain piece of food at different speeds and directions at different positions within a certain area. The information in the search process is shared among the birds, and the transmission of information and the cooperation show the characteristics of swarm intelligence. In the whole search area, particles have position and flight speed. And it can be based on the individual’s own information and the information shared by the group. It iteratively tracks the optimal position currently known by the individual and the optimal position that can be searched in the current group. It continuously updates its position to obtain global optimal solution.
The change of particle state is mainly affected by three factors: the original speed of the particle, the past individual optimal state, and the group optimal state. In the process of continuous iteration, the individual particle not only absorbs its own experience but also adjusts its own speed and position adaptively based on the experience of the group. The PSO pipeline is demonstrated in .
PSO first initializes various parameters in the PSO algorithm and then determines the fitness of the individual. Then, update velocity and position in the group and finally determine whether the optimization process is terminated. The iteration terminates when the change of the extremum of the population meets the minimum limit or reaches the preset maximum iterations. The output for global optimal position of the current round is the obtained optimal solution. Otherwise, return to the previous step to continue the iteration.
How well the algorithm performs is directly influenced by how well the parameters are chosen. The population size is the total number of particles in the population. When the population size is large, particles have a larger search area. The problem is that it will increase the time it takes to optimize, and it may even cause the algorithm to fail. The smaller the population, the more likely it is that a local solution will be found. The inertia weight has a significant impact on the PSO algorithm’s global and local search capabilities and should be optimized. How efficiently a particle retains its original speed depends on its inertia weight. Having a high inertia weight weakens the local search ability, but it increases the global search ability and the convergence rate. Local optimal solutions are not easy to find, but it is difficult to find a more exact one. With a tiny weight, local and global search abilities are strong; nevertheless, convergence speeds are slow and it is simple to slip into a local optimal solution.
However, the found solution is more accurate with a smaller weight. Each particle in a group is affected by the learning factor in the optimization process since each particle shares knowledge with the others. Both the individual and global ideal positions of a particle are represented by two learning variables, which are degree coefficients. Choosing the right learning factors can help prevent the algorithm from falling into a local optimal solution and shorten the time it takes to optimize the method. The maximum particle velocity should be set during algorithm optimization. The greater the search parameter, the more powerful the global search. If have a strong local search ability, it will have a better chance of finding an accurate answer than if do not have a strong local search capacity at all.
The parameter improvement of PSO algorithm can be started from three aspects: adjustment of inertia weight, improvement of limiting learning factor and other parameters. The inertia weight value is relatively large at the beginning of the loop. The inertia weight value should be kept to a minimum when we approach the global ideal solution later in the process. This work uses a linear weight decay (LWD) strategy.
where is current iteration,
is maximum iteration,
is the maximum weight,
is the minimum weight.
Decreasing trend of the weight value is relatively slow in early stage, global search ability is strong, which is conducive to finding an approximate range for optimal solution. At a later stage, the weight reduction trend is accelerated, which is conducive to finding a more accurate solution. This reduces the limitations of the typical linear decreasing method to a certain extent, and the performance is improved.
In addition, the PSO algorithm’s ability to optimize itself is directly influenced by the component of learning. First, the particle is primarily influenced by the individual optimal value, and then by the group optimal value at the end of the process of optimization. This work adopts an adaptive learning factor (ALF) strategy, which adjusts its value as the number of iteration changes.
where is the maximum learning factor,
is the minimum learning factor.
To improve global optimization and prevent falling local optimal solution, this paper introduces a random disturbance factor (RDF). In the continuous iteration process of particles, when the global optimal position does not change for several consecutive generations, the particles are forced to be influenced by random disturbance factors.
where is random number and
is the position of the particle with the worst fitness.
3.3. IPSO-DBN Algorithm
DBN is able to mine and learn about data at a granular level. As a result, it is possible to make the training process fall into a local optimal solution or extend the convergence period of a DBN network. Using the IPSO-DBN model developed in this paper, the DBN network’s starting weights can be optimized to increase prediction accuracy.
The essence for the optimization based on the IPSO algorithm is to map connection weights to particles in the IPSO algorithm. Through group cooperation and information sharing, it continuously changes the speed and position of particles for optimization until the best initial weight is selected. The flowchart of optimizing DBN network weights using IPSO is demonstrated in .
The first step is to initialize DBN network and particle swarm. Determine the number of DBN network layers and neurons, and determine the dimension for a particle according to the number of connection weights of the neural network. Determine the weight range, and randomly initialize velocity and position. In the second step, the fitness is calculated; individual optimal position and global optimal position are updated. The third step is to calculate that if the current fitness of the particle is greater than the individual optimal value, this value is assigned to the individual extreme value, otherwise it remains unchanged. If there is an individual optimal value in the whole group that is better than the group optimal value, it will be assigned to the group optimal value, otherwise it will remain unchanged. The fourth step is to update velocity and position. The fifth step is to judge whether the iterative process is terminated according to the conditions.
When the number of iterations reaches the maximum value or the change of the optimal value of the population is small, the iteration is terminated and the next step is entered. Otherwise, go back to the second step. In the sixth step, each dimension value of the global optimal vector finally obtained after the optimization of the PSO algorithm is used as the connection weight of the DBN network. Afterward, the pre-training and reverse fine-tuning of DBN are performed until DBN training requirements are met.
The steps involved in training the initial model and how the weights are updated using the algorithm:
Initialize the Model: Start by initializing the neural network model, including the number of layers, the number of neurons in each layer, and the initial weights and biases. The initial weights are often set randomly.
Define the Loss Function: Choose a suitable loss function that quantifies the error between the model’s predictions and the ground truth. The choice of loss function depends on the specific problem and the nature of the data (e.g., mean squared error for regression, categorical cross-entropy for classification).
Forward Propagation: Perform forward propagation, where the input data are fed into the network, and the activations and outputs are computed layer by layer. This process propagates the input through the network, producing a prediction.
Calculate Loss: Compare the predicted output with the actual target output using the chosen loss function. This calculates the loss/error value, which represents how well the model is currently performing.
Backpropagation: The backpropagation algorithm is used to calculate the gradients of the weights and biases with respect to the loss function. These gradients indicate the direction and magnitude of weight adjustments needed to minimize the loss.
Weight Update: With the gradients calculated, update the weights and biases of the model using an optimization algorithm. This algorithm determines how the weights should be adjusted to minimize the loss. Examples of optimization algorithms include stochastic gradient descent (SGD), Adam, or RMSprop.
Repeat the Process: Iterate through steps 3 to 6, feeding new batches of training data into the network, calculating the loss, performing backpropagation, and updating the weights. This iterative process, known as an epoch, helps the model learn patterns and improve its predictions.
Training Termination: Determine a stopping criterion for training, such as a maximum number of epochs, reaching a certain level of accuracy, or observing diminishing improvements in the loss. Once the termination criterion is met, the training process stops, and the final weights are obtained.
It is important to note that the specific algorithm for weight update, such as IPSO-DBN in your case, should be applied during the weight update step (Step 6). This algorithm, which integrates particle swarm optimization (PSO) with a deep belief network (DBN), optimizes the initial weights and biases of the network. The IPSO-DBN algorithm determines how the weights should be adjusted based on the defined objectives and constraints, considering factors like the linearly decreasing weights and adaptive learning factors mentioned in your work.
Randomly, initialize the weights using Gaussian distribution. This involves assigning random values to each weight parameter in the network.
Overall, the training process involves iteratively adjusting the weights and biases of the network based on the calculated gradients and the chosen optimization algorithm, until the model reaches a satisfactory level of performance.
3.4. Foreign Investment Strategy
Affected by the double survival pressure brought by the intensified pressure of international market competition and the rising cost of domestic factors, most enterprises will face many unfavorable factors in the process of development. This work proposes a series of foreign investment strategies for the benefit and safety of China’s foreign investment under the BRI.
First, further promote digital brand building and support enterprises to actively participate in the process of digital industrialization and industrial digitization. Use digital means to enhance the value of traditional brands and vigorously cultivate personalized cross-border e-commerce enterprises. Through the building of market reputation and brand image, the core competitiveness will be established, thereby reducing marketing costs and gradually expanding international market channels. By establishing independent stations, cross-border e-commerce companies can effectively circumvent the regulatory barriers of third-party cross-border service platforms. This can be modified and adjusted according to its own development requirements, and differentiated management can be implemented, which is less restricted by background data operations.
On the other hand, the independent station provides a highly free and personalized platform channel for cross-border e-commerce enterprises. Through precise delivery, enterprises can better convey brand values, brand advantages, corporate culture, and so on to customers, and the brand premium effect is obvious. In addition, cross-border e-commerce enterprises can also use independent stations to realize the accumulation of private domain traffic and the autonomy of customer data. And use big data to analyze and promote marketing to potential customers to improve customer stickiness, so as to achieve a comprehensive improvement in channel control capabilities.
Second, improve the level of trade facilitation. Thanks to development for digital technology and improvement of data infrastructure construction, the digital trade industry chain has developed rapidly. However, as a brand-new industrial form, there is still huge room for growth at each end of the digital trade industry chain. It needs to seize the development opportunity and notice the leading role of system. Empowered by digital technology, data are the foundation of the digital trade industry chain. All parties involved can be united to jointly promote the standardization of digital trade and establish a data repository with a unified surveying and mapping caliber. This provides the basis for economic research, industry analysis, and policy advice in related fields.
Third, actively promote green and sustainable development. Carbon neutrality is an important issue on the sustainable development agenda. More and more Internet platforms and data centers have also begun to provide green certificate transactions, market-based procurement, and renewable energy applications to implement carbon neutral policies. Green and sustainable development may become an important component of Chinese corporate brands going overseas in the future. Data analysis shows that in recent years, more consumers are willing to pay more premiums for green products, and concept of green consumption has been continuously strengthened. In this context, cross-border e-commerce companies can take this opportunity to explore a resource-saving and environment-friendly industrial-chain development model in the process of branding overseas.
Fourth, speed up the exploration of the talent introduction mechanism. In the context of the shortage of talents, it is very necessary for the digital transformation of enterprises and the digital transformation of employees to resonate at the same frequency. On the one hand, it is necessary to promote and strengthen the application ability training of existing employees. This enables them to master more cross-border e-commerce platform operation skills and e-commerce business knowledge on the basis of using traditional foreign trade work experience. At the same time, it must also fit the development characteristics of the industrial chain. Colleges and universities are also an important part of talent training. It is necessary to further implement the integration of production and education in the Internet field and improve the training of corresponding talents in higher education.
4. Experiment
This study uses neural networks:
Nonlinear Modeling: Neural networks excel at capturing complex nonlinear relationships in data. They are capable of learning intricate patterns and representations, making them suitable for various tasks where linear models may be insufficient.
Feature Learning: Neural networks have the ability to automatically learn and extract relevant features from raw data. Instead of manually engineering features, the network learns to identify important representations during the training process. This can be particularly useful when dealing with high-dimensional or unstructured data, such as images, text, or time series.
Flexibility and Adaptability: Neural networks offer flexibility in terms of architecture and model design. They can be customized to handle different types of data and problem domains. With various types of layers, activation functions, and network topologies, neural networks can be tailored to specific requirements and adapt to different types of data.
Large-Scale Data Analysis: Neural networks are well suited for handling large-scale datasets. They are capable of parallel processing and distributed computing, allowing efficient training on massive amounts of data. This makes them suitable for tasks like image classification, natural language processing, and recommendation systems.
Prediction Accuracy: Neural networks have demonstrated state-of-the-art performance on a wide range of tasks, including image and speech recognition, natural language processing, and time-series forecasting. Their ability to capture complex relationships and learn from data makes them powerful tools for achieving high prediction accuracy.
Continual Improvement: The field of neural networks is continuously evolving, with ongoing research and advancements. New architectures, optimization algorithms, regularization techniques, and training strategies are constantly being developed, improving the performance and capabilities of neural networks.
It is worth noting that the choice to use neural networks depends on the specific problem, available data, computational resources, and other considerations.
Here are some explanations of why we have selected the IPSO-DBN method to evaluate the safety and effectiveness of Chinese outbound investment in the BRI context:
Enhanced Evaluation Capability: The integration of IPSO-DBN combines the strengths of two powerful techniques: the PSO algorithm and the deep belief network (DBN). The PSO algorithm is known for its optimization capabilities, while DBN is a type of deep learning model that excels in extracting complex patterns and features from data. By combining these techniques, IPSO-DBN can potentially provide a more comprehensive and accurate evaluation of the benefits and security issues associated with BRI investments.
Addressing Limitations of Traditional Approaches: The choice of IPSO-DBN may stem from the limitations observed in previous studies using traditional evaluation methods. Traditional approaches may suffer from issues, such as random initialization and getting trapped in local optimal solutions. The introduction of IPSO-DBN with its policy of linearly decreasing weights and adaptive learning factor in the PSO algorithm aims to overcome these limitations by improving the accuracy and reliability of the evaluation process.
Relevance to the BRI: The IPSO-DBN method may have been chosen specifically for its applicability to the BRI. By tailoring the evaluation framework to the unique challenges and objectives of the BRI, IPSO-DBN can provide more contextually relevant results. This method allows for a comprehensive analysis of the effectiveness and safety of Chinese outbound investments within the BRI’s framework, facilitating better decision-making for Chinese enterprises operating in the initiative’s regions.
Potential for Innovation and Advancement: The choice of IPSO-DBN may be driven by a desire to contribute something novel and innovative to the field. By combining two advanced techniques, IPSO-DBN offers a new approach that has the potential to advance the understanding and practice of evaluating investments under the BRI. The method’s integration of artificial intelligence and optimization algorithms demonstrates a forward-thinking approach to address the complexities and challenges associated with evaluating large-scale investment projects.
Ultimately, the selection of IPSO-DBN as the proposed method likely stems from a combination of its enhanced evaluation capabilities, ability to address limitations in traditional approaches, relevance to the BRI, and potential for innovation and advancement in the field of investment evaluation.
4.2. Dataset
This work is based on the web crawler technology to collect the corresponding data from the Internet to construct the data resources required for network training and testing. The data includes two characteristics of foreign investment income and foreign investment security risk. The specific characteristic indicators are demonstrated in . The evaluation metrics in this work are accuracy and recall.
Table 1. The characteristic indicators of foreign investment income.
Table 2. The characteristic indicators of foreign investment security risk.
The process of normalizing datasets before developing models is a common practice in machine learning, including neural network models. Normalization is performed to ensure that the input data has consistent scales and distributions, which can help improve model training and performance. Normalization is particularly important when working with features that have different ranges or units.
Normalization techniques typically involve transforming the data to have a mean of zero and a standard deviation of one (zero mean and unit variance) or scaling the data to a specific range, such as [0, 1]. The specific normalization method used depends on the characteristics of the data and the requirements of the model.
Here are a few commonly used normalization techniques:
Standardization (Z-score normalization): This involves subtracting the mean of the feature from each data point and then dividing it by the standard deviation of the feature. This ensures that the feature has a mean of zero and a standard deviation of one.
Min-max scaling: This method scales the data to a specific range, typically [0, 1]. The values of the feature are linearly transformed based on the minimum and maximum values observed in the dataset.
Logarithmic scaling: In some cases, it may be appropriate to apply a logarithmic transformation to the data. This can help in handling skewed distributions or compressing large ranges.
The decision to normalize the dataset depends on the nature of the data and the requirements of the specific modeling task. However, it is generally recommended to normalize the data, especially when using neural networks. Normalization can help the model converge faster during training, prevent certain features from dominating the learning process due to their larger scales, and ensure that the model’s predictions are not biased toward specific features.
It is important to note that normalization should be performed on the training data and then applied consistently to the validation and test data using the same normalization parameters (mean and standard deviation or min-max values) obtained from the training data. This ensures that the normalization process is consistent across all datasets used in the model development and evaluation.
4.3. Training Loss Experiment
In the IPSO-DBN algorithm, training the network is essential. This work first analyzes the training loss of IPSO-DBN, and the experimental data is demonstrated in .
As the training epoch increases, the loss of IPSO-DBN decreases rapidly at first and then stabilizes. When reaching 60 epochs, IPSO-DBN has reached convergence.
4.4. Comparison of IPSO-DBN with Others
To further verify the superiority of IPSO-DBN network, this work compares it with other artificial intelligence methods, and comparison results are demonstrated in .
The IPSO-DBN proposed in this work can achieve the highest accuracy and recall. Compared with any other method, IPSO-DBN can achieve corresponding improvements in both indicators.
4.5. Linear Weight Decay Experiment
IPSO-DBN uses a linear weight decay strategy. To verify the superiority of this measure, this work compares the accuracy and recall rates when using linear weight decay and traditional fixed weight, respectively, as demonstrated in .
Compared with the traditional fixed weight strategy, after using the linear decay weight strategy, the accuracy and recall rate of IPSO-DBN are improved by 1.8% and 2.3%. This confirms the superiority of the linear decay weight strategy.
4.6. Adaptive Learning Factor Experiment
IPSO-DBN uses an adaptive learning factor strategy. To verify the superiority of this measure, this work compares the accuracy and recall rates when using adaptive learning factor and fixed learning factor, respectively, as demonstrated in .
Compared with traditional fixed learning factor, after using adaptive learning factor strategy, the accuracy and recall of IPSO-DBN are improved by 1.4% and 1.6%. This confirms the superiority of the adaptive learning factor strategy designed in this work.
4.7. Random Disturbance Factor Experiment
IPSO-DBN uses a random disturbance factor strategy. To verify the superiority of this measure, this work compares the accuracy and recall rates when using random disturbance factor and not using random disturbance factor, respectively, as demonstrated in .
Compared with not using random disturbance factor strategy, after using the random disturbance factor strategy, the accuracy and recall of IPSO-DBN are improved by 1.2% and 0.9%. This confirms the superiority of the random disturbance factor strategy designed in this work.
4.8. DBN Optimization with IPSO Experiment
This work uses the improved IPSO to optimize the DBN. To verify the feasibility of this measure, this work compares the network performance of DBN, PSO-DBN, and IPSO-DBN, respectively, as demonstrated in .
Compared with traditional DBN, after using PSO to optimize DBN, the accuracy and recall rate of the network can be improved accordingly. After the improvement of PSO, the optimization of DBN can obtain the highest accuracy and recall rate.
4.9. Foreign Investment Strategy Experiment
This work proposes a series of measures for China’s foreign investment in the context of the BRI. In order to verify the effectiveness of these measures, the foreign investment returns and foreign investment security risks before and after using these measures were compared. The compared indicators are consistent with the contents in , and the comparison is carried out using a percentage system score. The comparative data are demonstrated in .
Table 3. Foreign investment income comparison.
Table 4. Foreign investment security risk comparison.
From the data shown in the two tables, it can be seen that after using the foreign investment strategy proposed in this work, investment returns can be improved, and investment security risks can be reduced. This verifies the superiority of the foreign investment strategy proposed in this work.
4.10. Discussion
The present study offers several features and improvements that contribute to the production of more accurate results compared to previous studies in the field.
Integration of IPSO-DBN: The utilization of IPSO-DBN, which combines the PSO algorithm with a deep belief network, is a unique aspect of this study. By incorporating the IPSO algorithm to optimize the initial weights of the DBN, the problem of random initial weights and the tendency to fall into local optimal solutions are effectively addressed. This integration enhances the accuracy and robustness of the evaluation process, leading to more accurate results.
Enhanced Evaluation Methodology: The proposed IPSO-DBN framework provides a more advanced and sophisticated methodology for evaluating the benefits and security issues of Chinese outbound investments. By leveraging the power of deep learning techniques and optimization algorithms, IPSO-DBN offers a more comprehensive and nuanced analysis compared to traditional evaluation methods. This enhanced methodology enables a more accurate assessment of the effectiveness and safety of investments under the BRI.
Specificity to BRI: Unlike previous studies that may have focused on general investment evaluation or specific regions, this study specifically targets the BRI. By tailoring the evaluation framework to the unique characteristics and challenges associated with BRI investments, the present study provides more contextually relevant and accurate results. The suggested recommendations for Chinese enterprises investing abroad under the BRI further strengthen the specificity and relevance of the study, ensuring that the results are better aligned with the objectives and requirements of the initiative.
Experimental Validation: The present study incorporates various experiments using IPSO-DBN and Chinese enterprises’ foreign investment proposals. These experiments serve as empirical validation and provide concrete evidence of the feasibility and accuracy of the proposed method. By showcasing the effectiveness of IPSO-DBN through rigorous experimentation, the study establishes a higher level of confidence in the accuracy of its results compared to previous studies that may have lacked empirical validation.
In summary, the present study stands out in terms of its integration of IPSO-DBN, enhanced evaluation methodology, specificity to the BRI, and experimental validation. These factors collectively contribute to producing more accurate results compared to previous studies in the field, ultimately enhancing our understanding and decision-making regarding Chinese outbound investments under the BRI.
5. Conclusion
Since the major BRI was proposed in 2013, many countries along the route have invested in the construction boom. Although many of these countries along the route are not at a high level of development, because of this, their development potential is huge, providing a good opportunity and platform for Chinese enterprises to invest overseas. In this context, more and more Chinese enterprises go out to the countries along the route to conduct investment and business activities. Although the scale and investment efficiency of China’s direct investment has continued to increase, the proportion of foreign direct investment attracted has been increasing year by year.
However, under deteriorating global trade, how to realize the upgrading and adjustment of its own industry and improve the investment efficiency has become a problem that urgently needs in-depth research. In this context, research on the benefits and security issues of China’s foreign investment under the BRI has become an important topic. This work combines it with artificial intelligence to propose a neural network for evaluating the effectiveness and safety of Chinese outbound investment.
First, this work applies DBN to evaluate the benefits and security for outbound investment under the BRI. This work introduces a policy of linearly decreasing weights and an adaptive learning factor into the PSO algorithm to construct IPSO. Then, the IPSO is utilized to optimize initial weights for DBN, and the problem that initial weights are random and easy to fall local optimal solution is improved to construct IPSO-DBN. Second, this work puts forward a series of suggestions for Chinese enterprises to invest abroad in response to the current investment problems under the BRI. Third, this work has carried out various experiments on IPSO-DBN and Chinese enterprises’ foreign investment proposals, and the experimental results have verified the feasibility of the method designed in this work.
Disclosure statement
No potential conflict of interest was reported by the author.
Additional information
Funding
References
- Abban, O. J., W. Jiying, and I. Adjei Mensah. 2020. Analysis on the nexus amid CO 2 emissions, energy intensity, economic growth, and foreign direct investment in belt and road economies: Does the level of income matter? Environmental Science & Pollution Research 27:11387–1883.
- Ahmad, M., P. Jiang, A. Majeed, and M. Yousaf Raza. 2020. Does financial development and foreign direct investment improve environmental quality? Evidence from belt and road countries. Environmental Science & Pollution Research 27 (19):23586–601.
- Ascensão, F., L. Fahrig, A. P. Clevenger, R. T. Corlett, J. A. Jaeger, W. F. Laurance, and H. M. Pereira. 2018. Environmental challenges for the belt and road initiative. Nature Sustainability 1 (5):206–09.
- Chan, S. 2017. The belt and road initiative: Implications for China and East Asian economies. The Copenhagen Journal of Asian Studies 35 (2):52–78.
- Cheng, Y., L. Song, and L. Huang. 2017. The belt & road initiative in the global arena: Chinese and European perspectives. New York: Springer.
- Coenen, J., S. Bager, P. Meyfroidt, J. Newig, and E. Challies. 2021. Environmental governance of China’s belt and road initiative. Environmental Policy & Governance 31 (1):3–17.
- Cuiyun, C., and G. Chazhong. 2020. Green development assessment for countries along the belt and road. Journal of Environmental Management 263:110344. doi:10.1016/j.jenvman.2020.110344.
- Fardella, E., and G. Prodi. 2017. The belt and road initiative impact on Europe: An Italian perspective. China & World Economy 25 (5):125–38. doi:10.1111/cwe.12217.
- Gabusi, G. 2017. “Crossing the river by feeling the gold”: The Asian infrastructure investment bank and the financial support to the belt and road initiative. China & World Economy 25 (5):23–45.
- Han, M., J. Lao, Q. Yao, B. Zhang, and J. Meng. 2020. Carbon inequality and economic development across the belt and road regions. Journal of Environmental Management 262:110250. doi:10.1016/j.jenvman.2020.110250.
- Hashemizadeh, A., Y. Ju, S. Mojtaba Hosseini Bamakan, and H. P. Le. 2021. Renewable energy investment risk assessment in belt and road initiative countries under uncertainty conditions. Energy 214:118923.
- Huang, Y. 2019. Environmental risks and opportunities for countries along the belt and road: Location choice of China’s investment. Journal of Cleaner Production 211:14–26.
- Huang, Y., T. B. Fischer, and H. Xu. 2017. The stakeholder analysis for SEA of Chinese foreign direct investment: The case of ‘one belt, one road’initiative in Pakistan. Impact Assessment & Project Appraisal 35 (2):158–71. doi:10.1080/14615517.2016.1251698.
- Hughes, A. C., A. M. Lechner, A. Chitov, A. Horstmann, A. Hinsley, A. Tritto, A. Chariton, B. V. Li, D. Ganapin, E. Simonov, et al. 2020. Horizon scan of the belt and road initiative. Trends in Ecology & Evolution 35 (7):583–93.
- Hurley, J., S. Morris, and G. Portelance. 2019. Examining the debt implications of the belt and road initiative from a policy perspective. Journal of Infrastructure, Policy and Development 3 (1):139–75. doi:10.24294/jipd.v3i1.1123.
- Hussain, J., K. Zhou, S. Guo, and A. Khan. 2020. Investment risk and natural resource potential in “belt & road initiative” countries: A multi-criteria decision-making approach. The Science of the Total Environment 723:137981.
- Jianping, D., and F. Chenlin. 2017. The study on religious risks in the belt and road initiative. Journal of Finance and Economics 43 (9):134.
- Khan, A., Y. Chenggang, S. Bano, and J. Hussain. 2020. The empirical relationship between environmental degradation, economic growth, and social well-being in belt and road initiative countries. Environmental Science & Pollution Research 27 (24):30800–14.
- Liu, W., Y. Zhang, and W. Xiong. 2020. Financing the belt and road initiative. Eurasian Geography and Economics 61 (2):137–45. doi:10.1080/15387216.2020.1716822.
- Maçães, B. 2021. Belt and road: A Chinese world order. Oxford: Oxford University Press.
- Mahadevan, R., and Y. Sun. 2020. Effects of foreign direct investment on carbon emissions: Evidence from China and its belt and road countries. Journal of Environmental Management 276:111321. doi:10.1016/j.jenvman.2020.111321.
- Mitić, A. 2017. Belt and road strategic communication resilience: Lessons from the SERBIA-BRI frame analysis. China-CEE Institute Working Paper 19:391.
- Pencea, S. 2017. Romania-China trade and investment relations against the backdrop of” one belt, one road“strategy. Romanian Economic and Business Review 12 (2):17–28.
- Qian, X., and J. Fulton. 2017. China-gulf economic relationship under the “belt and road” initiative. Asian Journal of Middle Eastern and Islamic Studies 11 (3):12–21.
- Sun, Y., L. Chen, H. Sun, and F. Taghizadeh-Hesary. 2020. Low-carbon financial risk factor correlation in the belt and road PPP project. Finance Research Letters 35:101491. doi:10.1016/j.frl.2020.101491.
- Sun, H., B. Kofi Edziah, X. Song, A. Kwaku Kporsu, and F. Taghizadeh-Hesary. 2020. Estimating persistent and transient energy efficiency in belt and road countries: A stochastic frontier analysis. Energies 13 (15):3837. doi:10.3390/en13153837.
- TANG, J., T. ZHANG, P. ZHOU, and R. ZHENG. 2015. An analysis of mineral resources distribution and investment climate in the ‘one belt, one road’Countries. Geological Bulletin of China 34 (10):1918–28.
- Teo, H. C., A. Mark Lechner, G. W. Walton, F. Ka Shun Chan, A. Cheshmehzangi, M. Tan-Mullins, H. Kai Chan, T. Sternberg, and A. Campos-Arceiz. 2019. Environmental impacts of infrastructure development under the belt and road initiative. Environments 6 (6):72. doi:10.3390/environments6060072.
- Wang, C., Z. Miao, X. Chen, and Y. Cheng. 2021. Factors affecting changes of greenhouse gas emissions in belt and road countries. Renewable & Sustainable Energy Reviews 147:111220. doi:10.1016/j.rser.2021.111220.
- Yu, H. 2017. Motivation behind China’s ‘one belt, one road’initiatives and establishment of the Asian infrastructure investment bank. Journal of Contemporary China 26 (105):353–68. doi:10.1080/10670564.2016.1245894.
- Yuan, J., X. Li, C. Xu, C. Zhao, and Y. Liu. 2019. Investment risk assessment of coal-fired power plants in countries along the belt and road initiative based on ANP-entropy-TODIM method. Energy 176:623–40. doi:10.1016/j.energy.2019.04.038.
- Zhang, L., and W. Doreen. 2017. Media representations of China: A comparison of China daily and financial times in reporting on the belt and road initiative. Critical Arts 31 (6):29–43.
- ZiroMwatela, R., and Z. Changfeng. 2016. Africa in China’s ‘one belt, one road’Initiative: A critical analysis. IOSR Journal of Humanities and Social Science 21 (12):10–21.