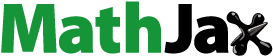
ABSTRACT
To address the problem of the classroom practice teaching of art and design, this paper proposes an interactive learning method in universities, followed by the use of AI technology to evaluate the quality of art and design teaching. The study aims to achieve the following objectives: 1) To introduce the current research status of art and design and interactive teaching methods used in other countries; 2) To discuss the essential ideas and mechanics of Back Propagation Neural Networks (BPNN) and other standard teaching approaches based on interactive learning; and 3) To input test data into the trained model and compare the obtained results with the evaluation results of experts. The findings of this study indicate that the model used to evaluate art and design instruction is accurate. The proposed interactive learning method is beneficial for art and design majors as it allows them to improve their practical skills and learn more engagingly and effectively. The use of AI technology for evaluation purposes can also improve the quality of art and design education in universities.
Introduction
Art and design education empowers students to develop their artistic and design skills. Hence the focus on students’ learning autonomy is becoming more prevalent. A high degree of self-motivation and self-management is also essential in the professional area to produce and maintain a creative practice. Thus, our primary educational objective in this discipline is to help our students become highly self-advocating (Sawyer Citation2017).
Art and design education learning is essentially a concept related to developing the learner’s awareness and applying knowledge to become a more effective learner. The interactive learning method can make it easier for students to identify the characteristics of the subject and their expectations for the teaching environment of art and design. Reflecting on the unique aspects of the art of design as a reflective process may help students become more adept in the ideas of the topic, which can help them further develop their conceptions of the subject. Students with this information may be able to make more informed decisions about how they approach learning, which might lead to more autonomy and productivity in the classroom (Shreeve, Sims, and Trowler Citation2010).
It may often be tempting for art and design educators to assume that the main characteristics of these particular disciplines are obvious and accessible to students. But moving from a highly organized high school teaching environment to a more autonomous, flexible, studio-based college environment can be daunting for most students and even negatively impact learning for a while. It is possible that the expectations and academic standards that underlie the studio-based teaching paradigm are new to students, or at the very least an important extension of what they have already learned in the past (Budge Citation2016a). These expectations and academic norms include the expectation that students will be active and autonomous, engaged in a stimulating learning situation, and expected to be forward-looking learners. Ability to manage one’s own learning process, design, monitor, and assess one’s own creative initiatives, as well as conduct one’s own independent research. These studio-based learning features at the third level assume that students can train themselves to take responsibility for their own learning and are committed to improving their learning to a higher level (Budge Citation2016b).
In addition, students’ prior knowledge of art and design will have an impact on how they approach their studies in the field of graphic design in general and graphic design in particular. Through some interviews with design students in interaction design teaching, it is noticed that the concept of design ontology is directly related to students’ notions of learning and methods, and students who have a relatively global concept of design ontology will develop their vision of what constitutes learning accordingly. In fact, the consideration of general teaching principles of art design is also a revelation for how we teach this subject (Sawyer Citation2018).
The fact that there is no single right answer, knowledge is often unpredictable or inconsistent with the various languages, perspectives and frameworks used by students and faculty to view works of art and the way they are reflected in other subjects. In studio teaching, by engaging in critical discussions of student experience, the nature of the subject is interpretable, but the meaning is never complete and unstable and fluid, because knowledge is inherently unstable and indeterminate (Wood Citation2004). In order to foster reflective learning, we use a combination of theme learning and core teaching ideas and practices in my classes. Students should be able to grasp the fundamentals of art and design, as well as the fundamentals of learning, by capturing the essence of the topic and the essence of the teaching method. Taking this approach to interactive learning recognizes how the learning of art and design is organized (Danvers Citation2003).
The interactive teaching of art design has many positive meanings. First of all, it helps to expand the teaching resources of art design and cultivate students’ artistic literacy and innovative thinking. At present, the excavation depth of interactive art in art design teaching is not enough, and the teaching content is single. Learning interactive art knowledge can change the current situation that students feel boring and jerky in learning, and make students feel fun in art and design learning. Interactive learning enriches teaching content and brings students a large amount of the latest materials. Through animation, video, music, etc., students’ senses of vision, touch, hearing and other aspects are mobilized. This enriches students’ perceptual experience and is conducive to the internalization of art and design knowledge (Kennewell et al. Citation2008).
Students may learn the fundamentals of computer manipulation in a more nuanced manner thanks to the inclusion of interactive technology in the classroom. This is not only beneficial to students’ overall development, but also to the demands of society as a whole.” Students will discover fresh and distinctive interactive teaching techniques, cultivate their multi-perspective thinking, and alter their limited viewpoints. Because interactive art integrates science, philosophy, life and other categories, it reflects the organic combination of various disciplines and multiple values. Learn new forms of artistic expression and stimulate the artistic potential and creative thinking of junior high school students (Senthamarai Citation2018).
Secondly, the interactive teaching of art design is realized through interactive technology, and the education and teaching methods are updated. The use of active learning strategies in the classroom has long been a focus of educational scientists. A dearth of research exists, however, on the subject of interaction between instructors and students in the classroom while teaching interactive art design. In this paper, multi-dimensional interaction is formed through interactive technology, which helps students’ bold participation and realizes efficient classroom (Yakovleva and Yakovlev Citation2014). In the teaching of art design, through real-time interactive games, each student can fully experience and feel, which is more conducive to the burst of inspiration and helps students express their ideas.
At the same time, students’ ability to collect information and organize materials is cultivated in interactive teaching. Students see with their eyes and feel with their hearts, mobilize various senses to enter the artist, and experience the core of the artwork. Each student creatively understands artworks from their own perspective, interacts and dialogs with artworks, enriches personal aesthetic experience, and exercises aesthetic judgment ability. Since the students’ individuality and creativity are expressed in the art works, this determines that the appearance of the art works is different for everyone. Art teachers sympathetically read and evaluate students’ works to achieve emotional interaction with students (Amiri Citation2011).
This paper proposes an interactive learning method for art and design majors in universities, followed by the use of AI technology to evaluate the quality of art and design teaching. There are several reasons why AI technology, specifically the BPNN, can be useful for evaluating the quality of art and design education in universities:
Objective evaluation: AI technology can provide an objective evaluation of the quality of art and design instruction, which may be challenging to achieve with traditional evaluation methods. AI models can analyze large amounts of data quickly and accurately, leading to more precise and reliable results.
Efficiency: Evaluating the quality of art and design instruction manually can be time-consuming and expensive. By using AI technology, the evaluation process can be automated, saving time and resources.
Scalability: With the increasing number of students enrolled in art and design programs, it may not be feasible to evaluate each student’s work manually. AI models can handle a vast amount of data, making it possible to scale the evaluation process to meet the needs of large student populations.
Feedback: The BPNN model used in this study can provide feedback to both students and instructors on the strengths and weaknesses of their work and teaching methods, respectively. This feedback can help students improve their practical skills and help instructors refine their teaching methods.
Standardization: AI models can apply standard criteria and metrics consistently when evaluating student work, reducing subjectivity and ensuring that all students are evaluated using the same criteria.
Overall, using AI technology, particularly the BPNN model, for evaluating the quality of art and design education can provide a more objective, efficient, and scalable method of evaluation, while also providing valuable feedback to students and instructors.
The motivation for conducting this research is to address the issues with the traditional classroom practice teaching of art and design majors in universities. While classroom teaching is an effective way to develop theoretical knowledge, it may not always be the best way to develop practical skills. Therefore, this research proposes an interactive learning method for art and design majors in universities, followed by the use of AI technology to evaluate the quality of art and design teaching. The study aims to contribute to the field of art and design education in the following ways:
Providing a new interactive learning method for art and design majors in universities that promotes the development of practical skills in addition to theoretical knowledge.
Evaluating the effectiveness of AI technology for assessing the quality of art and design instruction, which can provide valuable feedback to educators and students.
Contributing to the ongoing conversation about the role of AI technology in education, specifically in the context of art and design education.
Overall, the research contributes to the field of art and design education by proposing a new interactive learning method and evaluating the potential of AI technology to enhance the quality of instruction. By doing so, this research aims to improve the educational experience and outcomes for art and design students in higher institutions.
The article is structured into four main sections. Section 2, Related Work, provides a review of the current research status of art and design education and the interactive teaching methods used in other countries. This section aims to contextualize the proposed interactive learning method and provide a foundation for the study.
Section 3, Method, outlines the proposed interactive learning method and the use of AI technology for evaluating art and design instruction. This section provides a detailed description of the backpropagation neural network (BPNN) and other standard teaching approaches used in the study. It also describes the data collection process and the methodology used to evaluate the effectiveness of the proposed interactive learning method.
Section 4, Experiment and Analysis, presents the results of the study. This section describes the input test data into the trained model and compares the obtained results with the evaluation results of experts. It also provides an analysis of the data and discusses the implications of the findings.
Finally, Section 5, Conclusion, summarizes the main findings of the study and discusses their implications for art and design education. This section also highlights the limitations of the study and suggests areas for future research. The conclusion provides an overview of the article and emphasizes the value of the proposed interactive learning method and the potential of AI technology for enhancing art and design education in universities.
Related Work
The art and design education at CAU in my nation needs to catch up to the fast growth of China’s design sector, notably in teaching practical courses. CAU is a major factor in developing my country’s artistic and design skills. To raise the general level of artistic and design talent at CAU and the quality of education and teaching at CAU, it is critical to improving the teaching of practical courses in art and design classrooms. The teaching theory of art design at CAU could be better, and the practical teaching link still needs to be stronger. Classroom practice teaching is most CAUs’ main form of art design education (Mason and Steers Citation2006).
The conventional classroom technique and substance of teaching are increasingly out of touch with society and incommensurate as the market economy develops. Many art design professors at CAU have conducted extensive theoretical research into practical teaching and innovative teaching methods based on their disciplines, professional characteristics, student conditions, and social needs against the country’s vigorous education and teaching reform promotion. The interactive teaching mode, the “three-stage” practical teaching of computer art design major, and thematic teaching and other practical teaching modes and theories provide important theoretical references and practical basis for the teaching research of art design majors in classroom practice courses. These excellent teaching theories and models have important reference significance for reforming and developing classroom practice teaching of art design majors in CAU China (Hall Citation2010).
The term “interaction” refers to how people, their surroundings, and their actions interact in a given circumstance. There are several ways to think about interaction in educational psychology. This kind of contact is defined by feedback in classical educational psychology. As a result, feedback significantly impacts learning outcomes, and the timing and accuracy of input play a critical role. It is widely accepted in cognitive psychology that interaction is at the heart of learning in the present age of learning psychology (Giorgdze and Dgebuadze Citation2017). Individuals and their environments are intertwined in learning, according to Reference (Murray and Brightman Citation1996).
The learner’s cognitive structure interacts with learning materials. The interaction became more nuanced and broadened with the rise of constructivism. Many theories claim that learning occurs when new and old ideas are combined. Subject construction theory is one of these theories. Situational epistemology believes learning occurs when the learner and the learning environment interact. Learning activity theory holds that learning results from the interplay between the many activity components. Learning objects and learners interact in various ways, which we call interaction (Ridley Citation2007 The economic and technical shifts that gave birth to interactive learning as a criticism of the neoclassical market model initially arose in economics research on corporate innovation.
According to reference (Tuma Citation2021), the notion of interactive learning was introduced, and it was claimed that many essential learning and innovation processes are interactive. Interactions between divisions inside a company, enterprises, information providers, financial institutions, training, and governmental administrations are just a few examples of this interaction in action. Institutional influences on innovation and technology policy agenda-making were the topic of a debate about interactive learning began by reference (Xhemajli Citation2016).
Consequently, an introduction to interactive learning in innovation is provided, but a coherent and specific description needs to be included. While it is true that interactive learning involves a connection between components, it may also be used to refer to a process in which elements engage together in knowledge formation, dissemination, and application that is influenced by institutional norms and social practices (Meguid and Collins Citation2017).
Humanistic psychology and psycholinguistics, which arose in the late 1960s and early 1970s, formed the basis of interactive teaching in the 1970s – methods of educating students to improve their capacity to learn. In interactive teaching, the idea is that professors and students are no longer merely passively receiving professional information from one another. Teachers and students should engage in various interactions, as well as between students and students (S). Language instruction should be student-centered, and instructors should supply students with relevant language resources, establish a natural language environment, and allow students to learn meaningfully.
Classroom indoctrination has been the primary form of college education in many countries, including art and design majors. However, the traditional classroom teaching methods may not be sufficient to develop practical skills among art and design students. To address this problem, this study proposes an interactive learning method for art and design majors in universities, and uses AI technology, specifically the Back Propagation Neural Network (BPNN), to evaluate the quality of art and design instruction.
The contributions of this study are as follows:
Introducing an interactive learning method for art and design majors that emphasizes practical skill development and engagement.
Applying AI technology, specifically the BPNN model, for the evaluation of art and design instruction, which provides an objective, efficient, and scalable method of evaluation.
Providing valuable feedback to both students and instructors on the strengths and weaknesses of their work and teaching methods, respectively.
Contributing to the improvement of the quality of art and design education in universities.
The findings of the study indicate that the proposed interactive learning method and AI-based evaluation can enhance the quality of art and design education and provide valuable feedback to students and instructors.
Method
Teaching Method Based on Interactive Learning
Investigating interactive teaching approaches, a kind of interactive learning, is the primary focus of current research into applying this topic to the classroom. According to the available research, it is required to outline the teaching techniques of interactive learning to guide the teaching and increase the learning impact of interactive learning.
Here are some research questions that investigated in this study:
Can an interactive learning method enhance the practical skills of art and design majors in universities?
How accurate and reliable is the Back Propagation Neural Network (BPNN) model for evaluating the quality of art and design instruction?
How does the feedback provided by the BPNN model compare to that provided by expert evaluation and peer review?
Can the combination of an interactive learning method and AI-based evaluation improve the overall quality of art and design education in universities?
These research questions reflect the main aims and contributions of the study, which are to propose an interactive learning method for art and design majors in universities, apply AI technology, specifically the BPNN model, for the evaluation of art and design instruction, and provide valuable feedback to students and instructors on the strengths and weaknesses of their work and teaching methods, respectively.
The following are the three main components of an interactive lesson plan.
1) Classroom engagement is at the heart of interactive teaching. “interactive teaching” refers to activities in which students and instructors work together to achieve a common goal. Students are the recipients of information and the producers of knowledge, as the core body of educational activities. To effectively activate students’ subjective initiative, teachers must shift from being information imparters to knowledge guides and instructors. Classroom seating arrangements, for example, fall under the “hard environment” category, but student-to-student dialogue and interaction fall under the “soft environment.” Both in problem-oriented interactive teaching and in group-led interactive teaching, Problem-based interactive teaching cultivates students’ pioneering and inventive attitude toward self-directed learning. It may help pupils become more aware of the importance of collaboration and problem-solving.
Developing students’ ability to question is the major goal of problem-oriented interactive education. Students should be taught to approach issues scientifically and to base their allegations and critiques on evidence. Students should not only have the courage to ask questions but also become adept at doing so, as this will help them develop their capacity for creative thinking in the long run. Students are randomly divided into multiple homogenous groups according to the needs of the learning subject to implement group interactive teaching. Regarding teaching, the most important connection is group activities, which require students to work together to accomplish learning objectives and evaluate and reward the group’s overall performance. Make students the driving force behind their education, and the initiative will follow suit. Group talks, assessment, feedback, encouragement, mutual support and reciprocal learning, mutual instructors and students, and other cooperative and interactive activities enable pupils to develop a feeling of collaboration. Finally, it benefits students’ open-mindedness and enhances the interaction between professors and students and between students and their peers.
2) Interactive education delivered over the internet. The “human-computer-human” interaction is implemented using network communication technology in the context of interactive education in the network environment. There are two basic types of network-based interactive instruction. For example, with real-time interaction, instructors and students are involved in the online teaching process at the same time. Non-real-time engagement, conversely, implies that students contact professors or other students through network communication channels, such as forums, blogs, etc.
BP Neural Network
The BP learning algorithm is one of the most widely used supervised learning algorithms in feedforward neural networks. Scientists have verified the BP learning algorithm through detailed mathematical derivation. Multi-layer feedforward neural network BPNN is based on signal monitoring and inverse error technique. It employs the gradient descent approach to continuously learn from mistakes and alter the network’s weights and thresholds to reduce the network’s total squared error sum. Its network structure is relatively simple, the algorithm is easy to understand, the error is small after adjustment, and the accuracy of the entire network is high. It has been widely used in many fields.
The BPNN is eventually selected as the AI technology for evaluating the quality of art and design instruction in this study for several reasons:
BPNN is a widely used and well-established machine learning technique for supervised learning problems, particularly in pattern recognition and classification tasks. Its popularity and effectiveness have been demonstrated in many fields, including image and speech recognition, and it has been used for evaluating the quality of education in other disciplines as well.
BPNN is particularly suitable for evaluating the quality of art and design instruction because it can learn from a large dataset of labeled images, such as student artwork, and then classify new images according to their quality. It is well-suited for tasks that involve recognizing patterns in complex and noisy data, which is often the case with artwork.
BPNN is a feed-forward neural network, which means that it can be trained to approximate any function, given sufficient data and computational resources. This makes it a flexible and powerful tool for evaluating the quality of art and design instruction, as it can adapt to different types of images and evaluate them based on a wide range of features.
BPNN is relatively easy to implement and train compared to other deep learning techniques, such as Convolutional Neural Networks (CNNs), which require more specialized knowledge and computational resources. This makes it a practical choice for evaluating the quality of art and design instruction, particularly in situations where resources are limited.
In summary, BPNN is selected as the AI technology for evaluating the quality of art and design instruction in this study because it is a well-established and effective machine learning technique, suitable for evaluating complex and noisy data, flexible and adaptable to different types of images, and relatively easy to implement and train.
BP Neural Network Structure
BPNN can be divided into three or multi-layer neural networks according to the number of layers of the network, including the input layer, hidden layer, and output. The hidden layer can be set to one or more layers, and its topology is shown in .
Mathematical Derivation of BPNN Algorithm
The BPNN learning algorithm is also known as a tutored learning algorithm. Its learning process consists of two stages. It may be summarized as follows: It begins with the input layer sending information to the hidden layer’s neurons; next, the hidden layer’s neurons process the information, and ultimately the output layer outputs the information. There is no backward propagation while using forward propagation since the weights and thresholds of the connections between each neuron remain the same. Using backpropagation, the neural network adjusts each layer’s weights and thresholds until the ultimate result is achieved when the output layer’s error is too great to match the criteria. It is safe to say that the output results match the specified error limits. An example of a three-layer BPNN structure may be used to show how the system learns. Three-layer BPNNs have an input layer, a hidden layer, and a final output layer, all working together. Neurons in different layers communicate with neurons in the same layer, but they do not communicate with each other. In a three-layer feedforward BPNN, it is assumed that the input vector is X, and the output vector of the hidden layer is Y. The output layer output vector is O. The expected output vector is d. Let represent the connection weight between the input layer node
and the hidden layer nodes dicey,
represent the connection weight between the hidden layer node
and the output layer
,
represents the threshold of the hidden layer node, Theta
is the output threshold for layer nodes.
1) Forward propagation process. First, randomly assign a small value ,
,
, and
, generally between (0, 1). The calculation formulas of the output signal
of the kth neuron node in the hidden layer and the output signal
of the jth neuron node in the output layer are:
2) Error backpropagation process. The back-propagation process of the error starts from the output layer, then calculates the error of each layer by layer in the reverse direction. Each layer’s connection weights and thresholds are updated according to the gradient descent method so that the network’s actual output is as close as possible to the desired output. If the actual output of the neural network is different from the expected output, the output error E is defined, and the calculation formula is:
Expand the above output error definition to the hidden layer; the calculation formula is:
And then further extended to the input layer, the calculation formula is:
According to formula (4) and formula (5), it can be seen that the output error of the neural network can be regarded as a function of the weight and threshold, so the error can be reduced by adjusting the weight and threshold.
Advantages and Disadvantages of BPNN Algorithm
The BPNN is similar to the structure of the human brain and has strong adaptability when processing data. After training and learning, various mappings from input to output can be well completed. Combining this mapping with nonlinear characteristics can solve various nonlinear problems well. The process is clear and concise, and the results are highly credible. It can also effectively reflect the relationship between input and output data and has a strong generalization ability for unfamiliar data. But neural networks also have some drawbacks.
There is no theoretical basis for determining the network structure. It is initially important to establish the topology of neural networks to develop a BPNN mathematical model. The number of input vectors may be used to identify the number of neuron nodes in the input layer, and the number of output vectors can be used to calculate the number of neuron nodes in the output layer. The number of hidden layers and the number of neuron nodes in each hidden layer have yet to be determined consistently. If the number of hidden layers is too small, the final output error will be large, and the prediction accuracy will be low. If there are fewer settings, it will prevent the neural network from learning slowly and efficiently.
The convergence rate is slow. Three parameters affect the adjustment rate of BPNN: weight w, threshold θ, and learning rate η. BPNN uses the gradient descent method to correct the connection weight w and the threshold θ, but the calculation process of the gradient descent method has limitations. For example, when the number of training reaches a certain number, only a small error can be reduced each time. In other words, if a predetermined error is to be reached, the number of iterations will be increased accordingly. The learning rate was introduced to speed up the network’s convergence speed. However, its determination is a highly difficult task. An artificially inflated sum of squared error rates is used to gauge a model’s accuracy. Because of this, the network’s learning process might become sluggish if its learning rate is too low. However, if the learning rate is too high, the network will fluctuate while attempting to reduce error, impeding the convergence of the whole learning process.
Experiment and Analysis
Data Samples and Preprocessing
To verify the validity of the model proposed in this paper, 1750 groups of data were collected from the Academic Affairs Office of a university for training and testing.
Parameter Selection of the Model
1) Selection of activation function: In this paper, three functions are selected for comparison, and the specific experimental results are shown in . It can be seen that the effect of the Relu function is better. Therefore, the activation function selected in this paper is the Relu function.
2) For the number of neurons in the hidden layer, this paper verifies the algorithm’s performance by setting the number of neurons from 2 to 20 different. Calculate its accuracy to get the optimal number of neurons. The experimental results are shown in . It can be seen that the accuracy rate is the highest when the number of hidden layer nodes is 14, so the number of hidden layer nodes is selected as 14.
3) Selection of learning rate: The learning rate is also an important factor affecting the training effect of the neural network. This paper selects the learning rate in the [0.1, 1] range for testing. The results are shown in . The model’s training effect is best when the learning rate is 0.6, so the selected learning rate is 0.6.
Performance Test of the Optimal Model
After selecting the optimal parameters of the RBF model through the above experiments, 100 groups of samples are input into BPNN to obtain the accuracy rate of its sample training, as shown in . It can be seen that the model quickly achieves convergence and the accuracy meets the preset requirements.
While comparing the proposed solution with other existing solutions can provide valuable insights into the strengths and weaknesses of different approaches to art and design instruction, it is not included as part of the current study. Instead, the current study focuses on proposing an interactive learning method for art and design majors in universities and applying AI technology, specifically the Back Propagation Neural Network (BPNN) model, for the evaluation of art and design instruction.
However, a comparison table between the proposed solution and other existing solutions is a potential future direction for this research. This table could include a comparison of the strengths and weaknesses of the proposed interactive learning method and AI-based evaluation with other approaches, such as traditional lecture-based teaching and manual evaluation by experts.
By comparing the proposed solution with other existing solutions, this future work could provide valuable insights into the relative effectiveness of different approaches to art and design instruction and help identify areas for improvement in the proposed method. Additionally, it could help inform future research directions and contribute to the ongoing development of art and design education in universities.
The proposed interactive learning method for art and design majors in universities, followed by the use of AI technology to evaluate the quality of art and design teaching, offers several advantages over traditional classroom practice teaching.
Firstly, the interactive learning method provides students with an opportunity to develop practical skills in addition to theoretical knowledge. This method allows for hands-on, experiential learning that can enhance students’ abilities to apply theoretical concepts in practice. By actively engaging with the subject matter, students can improve their problem-solving skills and develop a deeper understanding of the concepts.
Secondly, the use of AI technology for evaluation purposes can provide valuable feedback to educators and students. By analyzing large amounts of data, the AI system can identify patterns and provide insights into the strengths and weaknesses of teaching methods. This feedback can inform future teaching practices, allowing educators to tailor their instruction to meet the needs of individual students.
Thirdly, the proposed method can help bridge the gap between industry and academia. By providing students with practical skills and experience, the method can prepare them for real-world applications and increase their employability. The AI system can also identify areas where teaching methods may need to be updated to reflect industry trends and demands, allowing for a more relevant and up-to-date curriculum.
Overall, the proposed interactive learning method and the use of AI technology for evaluation purposes offer several advantages that can enhance the quality of art and design education in universities. By providing students with practical skills and experience, offering valuable feedback to educators and students, and bridging the gap between industry and academia, this method can contribute to the ongoing improvement of art and design education.
Conclusion
People’s demands for spirituality and aesthetics are rising in tandem with the rise of the social economy and the consequent rise in the quality of life. For my country’s design sector to continue growing rapidly, the need for art and design skills also grows. The art and design major has become popular for college admissions, and the number of art students is increasing. At present, many CAU has added this major. To increase the number of students and demonstrate the school’s strength, the popular art and design major is one of the key majors for school enrollment. The expansion of social needs, the rapid development of CAU, and the expansion of enrollment have made the number of students majoring in art and design at CAU reach an unprecedented scale.
The teaching quality of art and design majors in general higher education directly affects the comprehensive quality and professional level of future art and design talents in China. It affects the development and core competitiveness of my country’s design industry. The term “interactive learning” encompasses a wide range of activities that include students in learning. It is frequently employed in many different domains, including business innovation, effective communication skills development, and classroom instruction, with positive outcomes.
The current study on interactive learning focuses mostly on the consequences and teaching techniques of interactive learning in many academic fields. Enterprise innovation research includes creating based learning systems, models, and websites incorporating interactive learning models. The proposed method contributes to interactive learning by providing a more engaging and effective way for art and design majors to learn. Interactive learning is a student-centered approach to teaching that emphasizes active participation and collaboration between students and educators. It is an approach that can enhance students’ learning outcomes and improve their problem-solving skills.
The proposed method is based on interactive learning principles and allows students to actively engage with the subject matter through hands-on, experiential learning. By using AI technology to evaluate teaching methods, the proposed method can provide immediate feedback to students, allowing them to monitor their progress and adjust their approach as needed. This feedback can also inform educators about the effectiveness of their teaching methods, allowing them to tailor their instruction to meet the needs of individual students.
Moreover, the proposed method uses a backpropagation neural network (BPNN) and other standard teaching approaches based on interactive learning to improve students’ practical skills in art and design. This approach can help students develop a deeper understanding of the subject matter and improve their ability to apply theoretical concepts in practice.
Overall, the proposed method contributes to interactive learning by providing a more engaging and effective way for art and design majors to learn. It allows students to actively engage with the subject matter and receive immediate feedback, contributing to their overall learning outcomes and problem-solving skills.
Based on the above background, this paper proposes an interactive learning method for art design majors in CAU, and then uses AI technology to evaluate the quality of art design teaching and completes the following work:
Introduce the research status of the art design and interactive teaching methods at home and abroad.
The essential ideas and mechanics of BPNN and other standard teaching approaches based on interactive learning sed.
The use of AI-based approaches in art and design teaching has the potential to bring significant value and implications to the field of education. Here are a few potential benefits and considerations:
Personalized Learning: One of the significant benefits of incorporating AI into art and design education is that it can help personalize learning for students. AI algorithms can analyze student data, such as their performance on assignments or assessments, and provide personalized recommendations for further study or practice. This approach can help students learn at their own pace and focus on areas where they need the most support.
Objective Assessment: AI algorithms can also provide objective assessments of student work, which can be particularly valuable in subjective fields like art and design. For example, an AI system could analyze a student’s artwork and provide feedback on elements like composition, color, and style. This approach can help ensure that assessments are fair and consistent across different students and teachers.
Improved Feedback: AI-based approaches can also provide more detailed and actionable feedback than traditional teaching models. For example, an AI system could analyze a student’s artwork and provide feedback on specific brush strokes or color choices. This feedback can help students identify areas where they need to improve and develop more nuanced skills.
Ethical Considerations: However, there are also ethical considerations that need to be taken into account when using AI-based approaches in education. For example, some critics have raised concerns about privacy and data protection, as AI systems require access to large amounts of student data. Additionally, there is a risk that AI-based approaches could reinforce biases and perpetuate inequalities if not designed and implemented carefully.
Overall, the use of AI-based approaches in art and design teaching has the potential to complement and enhance traditional pedagogies and teaching models. However, educators must also consider the ethical implications of using AI in education and ensure that these approaches are implemented in a way that benefits all students equally.
Disclosure statement
No potential conflict of interest was reported by the author(s).
Additional information
Funding
References
- Amiri, F. 2011. Programming as design: The role of programming in interactive media curriculum in art and design [J]. International Journal of Art & Design Education 30 (2):200–2230. doi:10.1111/j.1476-8070.2011.01680.x.
- Budge, K. 2016a. Learning tobe: The modelling modeling design practice in university art and design teaching [J]. International Journal of Art & Design Education 35 (2):243–58. doi:10.1111/jade.12060.
- Budge, K. 2016b. Teaching art and design: communicating creative practice through embodied and tacit knowledge [J]. Arts and Humanities in Higher Education 15 (3–4):432–45. doi:10.1177/1474022215592247.
- Danvers, J. 2003. Towards a radical pedagogy: provisional notes on learning and teaching in art & design [J]. International Journal of Art & Design Education 22 (1):47–57. doi:10.1111/1468-5949.00338.
- Giorgdze, M., and M. Dgebuadze. 2017. Interactive teaching methods: Challenges and perspectives [J]. IJAEDU- International E-Journal of Advances in Education 3 (9):544–48. doi:10.18768/ijaedu.370419.
- Hall, J. 2010. Making art, teaching art, learning art: Exploring the concept of the artist teacher [J]. International Journal of Art & Design Education 29 (2):103–10. doi:10.1111/j.1476-8070.2010.01636.x.
- Kennewell, S., H. Tanner, S. Jones, and G. Beauchamp. 2008. Analysing the use of interactive technology to implement interactive teaching [J]. Journal of Computer-Assisted Learning 24 (1):61–73. doi:10.1111/j.1365-2729.2007.00244.x.
- Mason, R., and J. Steers. 2006. The impact of formal assessment procedures on teaching and learning in art and design in secondary schools [J]. International Journal of Art & Design Education 25 (2):119–33. doi:10.1111/j.1476-8070.2006.00477.x.
- Meguid, E. A., and M. Collins. 2017. Students’ perceptions of lecturing approaches: Traditional versus interactive teaching [J]. Advances in Medical Education and Practice 8:229. doi:10.2147/AMEP.S131851.
- Murray, R., and J. R. Brightman. 1996. Interactive teaching [J]. European Journal of Engineering Education 21 (3):295–308. doi:10.1080/03043799608923415.
- Ridley, R. T. 2007. Interactive teaching: A concept analysis [J]. The Journal of Nursing Education 46 (5):203.
- Sawyer, R. K. 2017. Teaching creativity in art and design studio classes: A systematic literature review [J]. Educational Review 22:99–113. doi:10.1016/j.edurev.2017.07.002.
- Sawyer, R. K. 2018. Teaching and learning how to create in art and design schools [J]. Journal of the Learning Sciences 27 (1):137–81. doi:10.1080/10508406.2017.1381963.
- Senthamarai, S. 2018. Interactive teaching strategies [J]. Journal of Applied and Advanced Research 3 (1):36–38. doi:10.21839/jaar.2018.v3iS1.166.
- Shreeve, A., E. Sims, and P. Trowler. 2010. A kind of exchange’: Learning from art and design teaching [J]. Higher Education Research & Development 29 (2):125–38. doi:10.1080/07294360903384269.
- Tuma, F. 2021. The use of educational technology for interactive teaching in lectures [J]. Annals of Medicine & Surgery 62:231–35. doi:10.1016/j.amsu.2021.01.051.
- Wood, J. 2004. Open minds and a sense of adventure: How teachers of art & design approach technology [J]. International Journal of Art & Design Education 23 (2):179–91. doi:10.1111/j.1476-8070.2004.00396.x.
- Xhemajli, A. 2016. The role of the teacher in interactive teaching [J]. International Journal of Cognitive Research in Science, Engineering and Education 4 (1):31. doi:10.5937/IJCRSEE1601031X.
- Yakovleva, N. O., and E. V. Yakovlev. 2014. Interactive teaching methods in contemporary higher education [J]. Pacific Science Review 16 (2):75–80. doi:10.1016/j.pscr.2014.08.016.