Abstract
The antineutrophil cytoplasmic antibody (ANCA)-associated vasculitis (AAV) is a systematic of relatively rare autoimmune diseases with unknown cause. Kidney involvement is one of the most common clinical manifestations, and the degree of renal damage is closely associated with the development and prognosis of AAV. In this study, we utilized the Robust Rank Aggreg (RRA) method in R to integrate GSE104948, GSE104954, GSE108109, GSE108112, and GSE108113 profile datasets loaded from Gene Expression Omnibus (GEO) database and identified a set of differentially expressed genes (DEGs) in kidney between AAV patients and living donors. Then, the results of gene ontology (GO) functional annotation showed that immunity and metabolism involved process of AAV both in glomerulus and tubulointerstitial. The Kyoto Encyclopedia of Genes and Genomes (KEGG) pathway analysis revealed that following pathways, such as complement and coagulation cascades pathway; Staphylococcus aureus infection; disease-COVID-19; and systemic lupus erythematosus (SLE) pathway play a crucial role in AAV. Next, the results analyzed by protein–protein interaction (PPI) network and Cytoscape software exhibited the hub genes ALB, TYROBP, and CYBB existed in both glomerular and tubulointerstitial compartments datasets. Finally, KEGG analysis using genes of two most important modules also further validated complement and coagulation cascades pathway and S. aureus infection existed both in glomerulus and tubulointerstitial compartments datasets. In conclusion, this study identified key genes and pathways involved in kidney of AAV, which was benefit to further uncover the mechanisms underlying the development and progress of AAV, biomarkers, and potential therapeutic targets as well.
Introduction
The antineutrophil cytoplasmic antibody (ANCA)-associated vasculitis (AAV) is a series of relatively rare autoimmune diseases with etiology unknown, mainly injury small vessels [Citation1]. AAV includes granulomatosis with polyangiitis (GPA), microscopic polyangiitis (MPA), and eosinophilic granulomatosis with polyangiitis (EGPA) [Citation2]. The serological marker for AAV is ANCA [Citation3]. There exist two major ANCA types, namely, directed against proteinase 3 (PR3) and myeloperoxidase (MPO). PR3-ANCA is mainly associated to GPA and MPO-ANCA to MPA. But substantial overlap exists and discordant ANCA type (e.g., MPO-positive GPA) is sometimes observed in certain populations, particularly in Asia [Citation4]. There is no different influence of AAV between males and females [Citation5–8]. The prevalence of AAV is estimated from dozens to hundreds per million [Citation9,Citation10]. Global figures of AAV prevalence display substantial geographic variations [Citation11]. During the past decades, both the incidence and prevalence of AAV have grown probably ascribe to improvements in disease classification, ANCA testing, and clinical recognition [Citation7,Citation12–14]. Although AAV affects small vessels in various organs, kidney involvement is one of the most common characteristics. Up to now, increasing studies point out that renal involvement is an important factor affecting the prognosis of patients with AAV both in adult and children. Before the employ of high-dose glucocorticoids (GC) and cyclophosphamide (CYC), the mortality rate of patients with severe AAV was up to 80% one year after diagnosis [Citation15]. Recently, the estimated five-year survival is 74–91% and 45–76% for GPA and MPA, respectively [Citation16]. However, infections accompanied by immunosuppressive therapy also attribute to rather high mortality rate [Citation15]. Thus, further revealing the pathogenesis of AAV and finding out the biomarkers and new therapeutic targets have become urgent emergency.
In this study, five microarray datasets download from the Gene Expression Omnibus (GEO) database were analyzed to further pick out the differentially expressed genes (DEGs) in AAV. After normalized all the datasets, the Robust Rank Aggreg (RRA) R package was applied to integrate DEGs. Subsequently, captured DEGs were analyzed by R package ‘clusterprofiler’ for the gene ontology (GO) functional annotation and Kyoto Encyclopedia of Genes and Genomes (KEGG) pathway analysis. Then, a protein–protein interaction (PPI) network of the DEGs was constructed using search tool for the retrieval of interacting genes/proteins (STRING) database, hub genes, and modules were identified by plug-in of Cytoscape software. Our research for analysis of AAV DEGs datasets may serve as reliable molecular markers and effective therapeutic targets, and also help to understand the pathophysiological mechanisms in AAV.
Materials and methods
Microarray data and kidney tissue processing
By searching in GEO database (http://www.ncbi.nlm.nih.gov/geo/), GSE104948, GSE104954, GSE108109, GSE108112 and GSE108113 profile datasets which met our requirements were downloaded. The datasets of GSE104948 and GSE104954 were based on a GeneChip Human Genome U133 Plus 2.0 Array platform (Affymetrix; Thermo Fisher Scientific, Inc., Waltham, MA), and datasets of GSE108109, GSE108112, and GSE108113 were based on a GeneChip Human Gene 2.1 ST Array platform (Affymetrix; Thermo Fisher Scientific, Inc., Waltham, MA). The samples of GSE104948 and GSE108109 came from glomerular compartments, samples of GSE104954 and GSE108112 from tubulointerstitial compartments. GSE108113 dataset was composed by GSE108109 and GSE108112. The detail of relevant datasets is shown in .
Table 1. Details of the GEO AAV data.
Human kidney tissue was treated as previously illustrated [Citation17]. In brief, obtained kidney tissue was stored in RNAlater (ThermoFisher) and microdissected into tubulointerstitial and glomerular compartments [Citation18]. Transcriptional data were employed to evaluate reliability of microdissection by reaching to 16–64 folds enrichment of glomerular selective or tubulointerstitial selective transcripts in each independent compartment [Citation18].
Identification of DEGs
The R package ‘limma’ was applied to normalize each expression matrix respectively, and then further screen DEGs in each dataset. These genes met the requirement, which were with p-value < 0.05 and logFC >1 were considered upregulated DEGs in each dataset. On the contrary, these genes, which were with p-value <0.05 and logFC <−1 were identified as downregulated DEGs in each dataset. The Venn diagram which exhibited the relationship among these DEGs was visualized applying VennDiagram R package.
Integration of expression datasets
The captured DEGs were further integrated applying the RRA R package according to glomerular compartments DEGs, tubulointerstitial compartments DEGs, glomerular and tubulointerstitial compartments DEGs. Then, the R package ‘VennDiagram’ was employed to exhibit the relationship among these integrated DEGs.
GO and KEGG pathway enrichment analysis of integrated DEGs
GO enrichment and KEGG pathway analysis was achieved by the R package ‘clusterprofiler’ [Citation19]. Data with adjusted p < 0.05 were considered statistically significant.
Construction of PPI network, capture of hub genes, and analysis of modules
The online database, STRING (https://string-db.org/), was employed to get the interactions between different proteins [Citation20]. Then the obtained data from STRING were further imported into Cytoscape software to acquire PPI network [Citation21]. The cytoHubba plug-in of Cytoscape software was used to pick out the top 10 hub genes based on the value of degree. The MCODE plug-in of Cytoscape software was applied to analyze PPI network and acquire the top two modules.
Results
Recognition of DEGs in ANCA-associated vasculitis
The ANCA-associated vasculitis datasets GSE104948, GSE104954, GSE108109, and GSE108112 were normalized, and the results are displayed in Supplementary Figure 1. The DEGs were screened using the R package ‘limma’ (adjusted p < 0.05 and |logFC| > 1). Firstly, 392 differential genes were contained in the GSE104948 dataset, including 165 upregulated and 227 downregulated genes. Next, there were 241 differential genes in the GSE104954 dataset, including 76 upregulated genes and 165 downregulated genes. In addition, 1519 differential genes, 941 upregulated genes, and 578 downregulated genes were contained in the GSE108109 dataset. Finally, the GSE108112 dataset contained 438 differential genes, including 271 upregulated genes and 167 downregulated genes. The DEGs of four datasets were exhibited in , and the top 75 genes’ heatmap in each dataset is shown in . The correlation matrix heatmap of top 75 genes in each dataset is displayed in Supplementary Figure 2. Venn diagram among each dataset is displayed in Supplementary Figure 3(A).
Figure 1. Differentially expressed genes between the two groups of samples in each dataset (A) GSE104948, (B) GSE104954, (C) GSE108109, (D) GSE108112. The red dots represent the upregulated genes based on an adjusted p < 0.05 and |log fold change| > 1; the blue dots represent the downregulated genes based on an adjusted p < 0.05 and |log fold change| > 1; the black spots represent genes with no significant difference in expression.
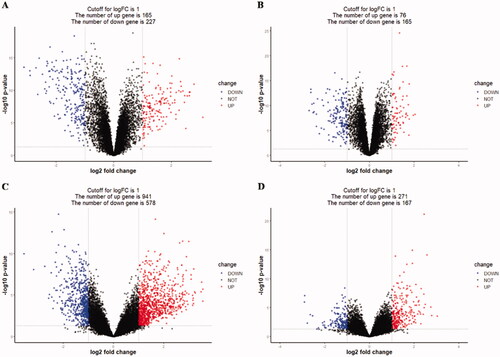
Identification of integrated DEGs in ANCA-associated vasculitis by RRA
The integrated DEGs were screened utilizing the RRA package (corrected p < 0.05 and |logFC |>1). About 143 integrated DEGs from glomerular compartments datasets (GSE104948 and GSE108109), consisting of 73 upregulated genes and 70 downregulated genes, were identified by the RRA method. What’s more, 81 DEGs from integrated tubulointerstitial compartments datasets (GSE104954 and GSE108112) contained 22 upregulated genes and 59 downregulated genes. In addition, 273 integrated DEGs from glomerular and tubulointerstitial compartments datasets (GSE104948, GSE104954, GSE108109, GSE108112) were consisted by 146 upregulated genes and 127 downregulated genes. As shown in , the top 20 upregulated and downregulated genes in each integrated DEGs were showed on heatmap, respectively. Venn diagram of each integrated DEGs is exhibited in Supplementary Figure 3(B).
Figure 3. LogFC heatmap of integrated datasets (A) Glomerular compartments DEGs, (B) Tubulointerstitial compartments DEGs, (C) Glomerular and tubulointerstitial compartments DEGs. The abscissa represents the GEO IDs, the ordinate represents the gene name, the red represents logFC > 0, the green represents logFC < 0 and the value in the box represents the logFC value.
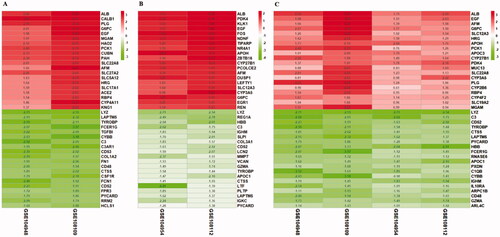
GO enrichment analysis of integrated DEGs
As it is well accepted now, the GO terms would be divided into three parts: cell component (CC), molecular function (MF), and biological process (BP). The GO results of each integrated DEGs are exhibited in and Supplementary Figure 4. These significant genes from glomerular compartments were mainly enriched in neutrophil degranulation (gene ontology: BP), apical part of cell (gene ontology: CC), and organic acid blinding (gene ontology: MF). These significant genes from tubulointerstitial compartments were mainly enriched in humoral immune response (gene ontology: BP), secretory granule lumen (gene ontology: CC), and endopeptidase activity (gene ontology: MF). These significant genes from glomerular and tubulointerstitial were mainly enriched in neutrophil degranulation (gene ontology: BP), collagen-containing (gene ontology: CC), and glycosaminoglycan binding (gene ontology: MF).
KEGG pathway analysis of integrated DEGs
KEGG pathway annotation of the integrated DEGs was using the clusterProfile R package, and the results of the analysis are displayed in . The upregulated DEGs from glomerular compartments datasets (GSE104948 and GSE108109) were mainly enriched in these pathways, such as phenylalanine metabolism; tyrosine metabolism and glycine, serine, and threonine metabolism (). The downregulated DEGs from glomerular compartments datasets were mainly enriched in Staphylococcus aureus infection; complement and coagulation cascades; pertussis (). The upregulated DEGs from tubulointerstitial compartments datasets (GSE104954 and GSE108112) were enriched in renin-angiotensin system; parathyroid hormone synthesis, secretion, and action; MAPK signaling pathway, etc. (). The downregulated DEGs from tubulointerstitial compartments datasets were mainly enriched in S. aureus infection; pertussis; complement and coagulation cascades (). The upregulated DEGs from glomerular and tubulointerstitial compartments datasets (GSE104948, GSE104954, GSE108109, GSE108112), were mainly enriched in these pathways, for example, phenylalanine metabolism; glycine, serine and threonine metabolism; glycolysis/gluconeogenesis (). The downregulated DEGs from glomerular and tubulointerstitial compartments datasets were enriched in pertussis; complement and coagulation cascades; viral protein interaction with cytokine and cytokine receptor, etc. ().
Figure 5. KEGG pathway enrichment analysis of the integrated DEGs (A) Glomerular compartments DEGs, (B) Tubulointerstitial compartments DEGs, (C) Glomerular and tubulointerstitial compartments DEGs. The red bars represent top 15 pathways from upregulated DEGs in each integrated dataset. The blue bars represent top 15 pathways from downregulated DEGs in each integrated dataset.
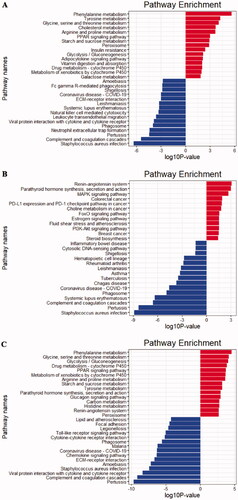
Construction of PPI network, hub gene and module analysis
The PPI networks from glomerular compartments DEGs, tubulointerstitial compartments DEGs, glomerulular and tubulointerstitial compartments DEGs were separately displayed in . The top 10 essential hub genes screened according value of degree are exhibited in . And the top two crucial modules are displayed in .
Figure 6. PPI network of different expression genes (A) Glomerular compartments DEGs, (B) Tubulointerstitial compartments DEGs, (C) Glomerular and tubulointerstitial compartments DEGs.
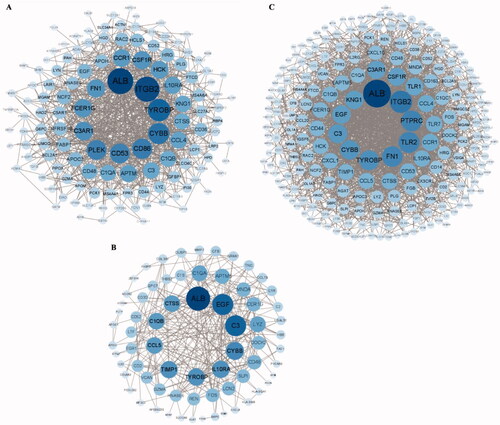
Figure 7. Hub genes of different expression genes (A, D) Glomerular compartments DEGs, (B, E) Tubulointerstitial compartments DEGs, (C, F) Glomerular and tubulointerstitial compartments DEGs. The dark of yellow is positively associated with the degree values.
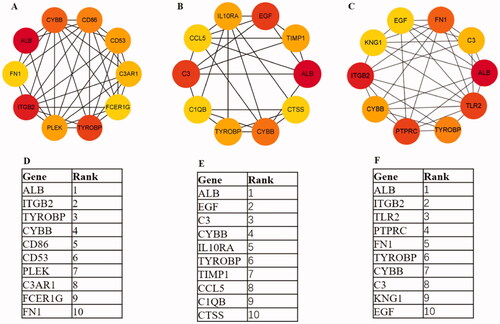
Figure 8. The module1 and module2 of different expression genes (A, B) Module1 and module2 in glomerular compartments DEGs, (C, D) Module1 and module2 in tubulointerstitial compartments DEGs, (E, F) Module1 and module2 in glomerular and tubulointerstitial compartments DEGs. The genes involved in module1 or module2 labeled in yellow.
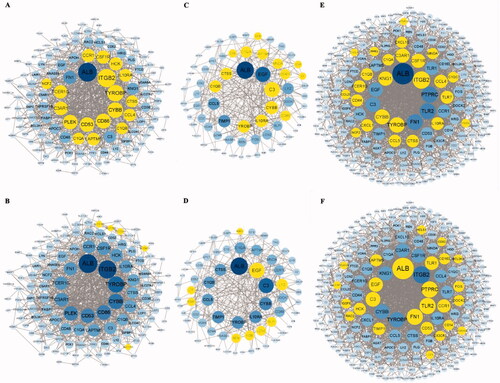
The genes from top two modules of glomerular compartments mainly enriched in S. aureus infection; complement and coagulation cascades; phagosome. Genes from top two modules of tubulointerstitial compartments enriched in S. aureus infection; complement and coagulation cascades; pertussis. The genes from top two modules of glomerular and tubulointerstitial compartments enriched in following pathways, listing as chemokine signaling pathway; cytokine–cytokine receptor interaction; complement and coagulation cascades, etc. All these results further exhibited that infection and immunity played very vital role in AAV.
Discussion
The occurrence of AAV is relatively low and the disease is recognized as a comparatively rare autoimmune disease. However, it is not rare to nephrologists, rheumatologists, clinical immunologists, as well as other physicians [Citation22]. AAV can attack people in all ages, including children, but the elderly at 65–74 years are more susceptible [Citation23]. In spite of adequate immunosuppressive, the prognosis of AAV patient is still poor [Citation24]. Previous researches revealed that the five-year survival rate of patient with AAV was about 70%, and infection was the main cause of death [Citation25]. Recently, with increasing knowledge of AAV pathogenesis, the management and diagnosis of AAV have greatly improved; however, challenges still yet to be totally resolved. Therefore, further exploring the potential mechanisms related to the malignant biological process of AAV would help to detect reliable biomarkers for the early diagnosis, predicting prognosis, early monitoring recurrence, and new therapeutic intervention targets as well.
AAV can undermine small vessels in various systems and organs, such as lungs, skin, joints, and kidneys [Citation26]. Kidney involvement is one of the most common clinical manifestation of AAV that could happen over half of the AAV patients, and dialysis-dependency at disease onset is high [Citation27,Citation28]. A cohort study included 502 patients with AAV diagnosed between 1985 and 2007 in America detected kidney involvement reached to 97%, ESKD was 32% and 27% died [Citation29]. Consistently, a descriptive study recruited renal-limited AAV patients(N = 16) between 2005 and 2016 from 8 centers worldwide, detected 38% progressing to ESRD, seven deaths, five within 6 months of diagnosis and two another at 16 and 21 months of follow-up [Citation30]. In China, a review from Zhao et.al systematically summarized the progress of AAV in Chinese patients [Citation31]. To some degree different from western countries, it showed AAV was not uncommon in China, the MPA was the compelling preponderance, MPO-ANCA was much more often than PR3-ANCA, and secondary infection (rather than AAV activity)was the main cause of death [Citation31]. What’s more, research in children of AAV in China found AAV usually occurred in teenage girls, the most commonly affected organ was also the kidney, and 58.8% of them progressed to ESRD with a median duration of three months [Citation32]. Thus, exploring the pathophysiological mechanism of kidney in AAV will not only benefit to raise awareness occurrence and development of AAV, but also contribute to improve the treatment and prognosis. Considering function of glomerulus focused on filtration and tubular mainly on reabsorption, we analyzed datasets according to the sample collected from glomerular or/and tubulointerstitial, respectively.
In this study, the GSE104948, GSE104954, GSE108109, GSE108112, and GSE108113 datasets were analyzed employing RRA method. The captured integrated DEGs were then experienced GO analysis. The genes from glomerular compartment were mainly enriched in neutrophil degranulation (gene ontology: BP), apical part of cell (gene ontology: CC), and organic acid binding (ontology: MF). The genes from tubulointerstitial compartment were mainly enriched in humoral immune response (gene ontology: BP), secretory granule lumen (gene ontology: CC), and endopeptidase activity (ontology: MF). And genes from glomerular and tubulointerstitial were mainly enriched in neutrophil degranulation (gene ontology: BP), collagen-containing (gene ontology: CC), and glycosaminoglycan binding (gene ontology: MF). These results suggested that immunity and metabolism involved in process of AAV both in glomerulus and tubulointerstitial.
The KEGG pathway analysis revealed that the integrated upregulated genes in glomerular compartment were mainly enriched in the following five pathways: phenylalanine metabolism; tyrosine metabolism; glycine, serine and threonine metabolism; cholesterol metabolism; arginine and proline metabolism. The downregulated genes in glomerular compartment focused on S. aureus infection; complement and coagulation cascades; pertussis; neutrophil extracellular trap formation and phagosome. The integrated upregulated genes in tubulointerstitial compartment were enriched in these pathways, such as renin-angiotensin system; parathyroid hormone secretion and action; MAPK signaling pathway; colorectal cancer and PD-L1 expression and PD-1 checkpoint pathway in cancer and so on. The downregulated genes in tubulointerstitial compartment were mainly in following pathways: S. aureus infection; pertussis; complement and coagulation cascades; systemic lupus erythematosus; and phagosome, etc. The upregulated genes from glomerular and tubulointerstitial were enriched in phenylalanine metabolism; glycine, serine and threonine metabolism; glycolysis/gluconeogenesis, etc. The downregulated genes from glomerular and tubulointerstitial compartment focused on pertussis; complement and coagulation cascades; viral protein interaction with cytokine and cytokine receptor; S. aureus infection; amebiasis; ECM-receptor interaction, etc.
It was important to discover complement and coagulation cascades pathway played a crucial role in AAV for the reason that it existed both in glomerular compartment and tubulointerstitial compartment datasets. The complement system, which included dozens of membrane-bound and circulating proteins, had a crucial role both in humoral and innate immunity. Activation of the complement system was mainly comprised by three pathways: the classical, the alternative pathways, and the lectin. Activation of any of three pathways resulted in formation of C3 convertase, and followed by activation of C5, finally resulted in initiating the assembly of the membrane attack complex (MAC), also called C5b-9 [Citation33]. The complement system was used to being ignored by AAV pathophysiology [Citation34], for lacking indirect signs of complement consumption in plasma of patients with AAV by routine tests. Until recently, the significance of complement had attracted growing attention [Citation35,Citation36]. A meta-analysis found plasma MAC, C5a, and factor B in patients with active AAV were increased compared with controls and patients in remission (excluding factor B) [Citation37]. Another study also demonstrated AAV patients with hypo-complementemic and low serum C3 were independent predictors for ESRD [Citation38,Citation39]. Among these patients with obtainable kidney biopsy, 56% had C3 deposition by immunofluorescence and exhibited more commonly a mixed histological type, low level of serum C3, serious infection [Citation38]. Avacopan, a new oral competitive inhibitor that interfered the binding of C5a to C5aR1 (CD88), revealed to have least similar efficacy contrasted to high-dose corticosteroids in patients with renal involved active AAV [Citation40].
S. aureus infection was also a crucial pathway in AAV. Study had found an increased nasal staphylococci carriage, which associated with relapse of Wegener's granulomatosis [Citation41]. A systematic review exhibited median time between infection and vasculitis development was three months’ cases of infection-induced MPO-ANCA, 21.7% patients expired during follow-up, 52.2% regressed after the resolution of infection in 12/23 [Citation42]. It suggested that sufficient anti-infective therapy was very important in MPO-AAV before immunosuppressive treatment, or else might lead to catastrophic consequence [Citation43].
Meanwhile, our data analysis showed coronavirus disease-COVID-19 and systemic lupus erythematosus (SLE) pathway also involved in AAV, which was consistent with previous reports. Up to now, growing studies had reported AAV after COVID-19 infection, which not only included adult [Citation44–46], but also children [Citation47]. Meanwhile, some studies also reported there was an overlap between AAV and SLE [Citation48,Citation49]. In light of these above reports, testing ANCA was vital for patients with COVID-19 infection or SLE.
Then, we separately constructed PPI network with integrated genes from different renal part. Ten hub genes from glomerular compartment were ALB, ITGB2, TYROBP, CYBB, CD86, CD53, PLEK, C3AR1, FCER1G, FN1. The top 10 hub genes from tubulointerstitial compartment listed as ALB, EGF, C3, CYBB, IL10RA, TYROBP, TIMP1, CCL5, C1QB, CTSS. The top 10 hub genes from glomerular and tubulointerstitial were ALB, ITGB2, TLR2, PTPRC, FN1, TYROBP, CYBB, C3, KNG1, EGF.
Obviously, the hub genes ALB, TYROBP, and CYBB existed in both the hub genes of glomerular or/and tubulointerstitial datasets. Highly consistent with our research, the research from Jae-Seung Moon et al. found that C-reactive protein (CRP) to serum albumin (ALB) ratio identified as an independent predictor of all-cause of mortality in patients with AAV [Citation50]. Thus, ALB might be as a biomarker in AAV. TYROBP was an adapter protein which non-covalently associated with activating receptors found on the surface of a variety of immune cells to mediate signaling and cell activation following ligand binding by the receptors [Citation51], mediate immune cell activation [Citation52,Citation53]. Despite the relationship between TYROBP and AAV was not yet reported. TYROBP might be associate with pathophysiological process of AAV for the reason that immunity imbalance was an essential factor in AAV. CYBB was a critical component of the membrane-bound oxidase of phagocytes that generated superoxide, as it was the beta chain of Cytochrome b (-245). CYBB deficiency was one of five described biochemical defects associated with chronic granulomatous disease (CGD) [Citation54]. But the relationship between CYBB and AAV was still not yet reported. Considering these, exploring the relationship between hub genes and AAV may help to elaborate the occurrence and development mechanism of AAV. Meanwhile, some hub genes only significantly different expressed in glomerulus or tubulointerstitial compartment dataset. Thus, it hinted the change expression of these genes may be beneficial to predict damage existed in glomerulus or tubulointerstitial. But it needed more deepen research.
To identify tissue specific of hub genes, we searched hub genes expression of normal tissues in the GTEx database (https://www.gtexportal.org/). In hub genes from glomerular datasets, ALB expressed relatively high in kidney–cortex compared with other tissues (Supplementary Figure 5(A)). In hub genes from tubulointerstitial datasets, ALB expressed relatively high in kidney–cortex compared with other tissues and EGF expressed relatively high in kidney–medulia (Supplementary Figure 5(B)). In hub genes from glomerular and tubulointerstitial datasets, it exhibited ALB and KNG1 expressed relatively high in kidney–cortex, EGF and KNG1 expressed relatively high in kidney–medulia (Supplementary Figure 5(C)). These results showed that ALB, EGF and KNG1 might be potential crucial genes participated in the pathophysiological process in kidney of AAV.
There is increasing evidence that represented the importance of genetic contribution to AAV. A genome-wide association study (GWAS) about AAV from European revealed both major-histocompatibility-complex (MHC) and non-MHC associated with AAV and also that GPA and MPO were genetically distinct [Citation55]. It is reported that MPO-ANCA was associated with HLA-DQ, while PR3-ANCA was associated with HLA-DP, the genes encoding α1-antitrypsin (SERPINA1) and proteinase 3(PRTN3) [Citation55]. In addition, eosinophilic granulomatosis with polyangiitis (EGPA) patients with MPO-ANCA positive was associated with HLA-DQ, while there was no association between HLA-DQ and ANCA-negative EGPA [Citation56]. Variants at the GPA33 and IL5/IRF1 loci were only associated with the ANCA-negative EGPA patients [Citation56]. In Chinese patients, studies had also suggested the relationship between AAV and different HLA loci. HLA-DRB1*1454 was less prevalent in AAV than normal controls, DRB1*1101 was more frequent in MPA patients, DRB1*1202 was more prevalent in PR3-ANCA-positive granulomatosis patients than normal controls [Citation57]. HLA-DPB1 variant rs3117242 was found to be associated with GPA in the Han Chinese population [Citation58]. All of these variants might affect expression of certain genes. Due to downloaded datasets not including clinic details and GWAS results, these integrated DEGs were specific to AAV patients or not, and which variants of genes existed in these samples, were still yet to be known. To solve these puzzles, we have gathered samples and clinic information of AAV patients and other autoimmune diseases in our center, intending to unveil this tricky puzzle by combining methods, such as microarray analysis, GWAS, expression quantitative trait loci (eQTL) analysis and bidirectional Mendelian randomization study, etc.
Conclusions
In summary, this research systematically explored potential mechanism underlying the kidney of AAV via integrated bioinformatics analysis. Meanwhile, this study also screened certain crucial genes and significant pathways which might benefit to hunt new biomarkers or therapeutic targets in kidney of AAV. Additionally, considering the different location and function of glomerular and tubulointerstitial in kidney, we analyzed glomerular and tubulointerstitial datasets separately. It would be more specifical to explore the pathophysiological mechanism of kidney in AAV. In the future, animal and clinic molecular biology experiments will be further required to validate the findings of this research.
Supplemental Material
Download PDF (1.6 MB)Disclosure statement
No potential conflict of interest was reported by the author(s)
Additional information
Funding
References
- Furuta S, Jayne DR. Antineutrophil cytoplasm antibody-associated vasculitis: recent developments. Kidney Int. 2013;84(2):244–249.
- Cornec D, Cornec-Le Gall E, Fervenza FC, et al. ANCA-associated vasculitis - clinical utility of using ANCA specificity to classify patients. Nat Rev Rheumatol. 2016;12(10):570–579.
- Falk RJ, Jennette JC. Anti-neutrophil cytoplasmic autoantibodies with specificity for myeloperoxidase in patients with systemic vasculitis and idiopathic necrotizing and crescentic glomerulonephritis. N Engl J Med. 1988;318(25):1651–1657.
- Chen M, Yu F, Zhang Y, et al. Characteristics of chinese patients with wegener's granulomatosis with anti-myeloperoxidase autoantibodies. Kidney Int. 2005;68(5):2225–2229.
- Mohammad AJ, Jacobsson LT, Mahr AD, et al. Prevalence of wegener's granulomatosis, microscopic polyangiitis, polyarteritis nodosa and Churg-Strauss syndrome within a defined population in Southern Sweden. Rheumatology. 2007;46(8):1329–1337.
- Watts RA, Mahr A, Mohammad AJ, et al. Classification, epidemiology and clinical subgrouping of antineutrophil cytoplasmic antibody (ANCA)-associated vasculitis. Nephrol Dial Transplant. 2015;30 Suppl 1:i14–22.
- Berti A, Cornec D, Crowson CS, et al. The epidemiology of antineutrophil cytoplasmic autoantibody-associated vasculitis in Olmsted county, Minnesota: a twenty-year US population-based study. Arthritis Rheumatol. 2017;69(12):2338–2350.
- Li J, Cui Z, Long JY, et al. The frequency of ANCA-associated vasculitis in a national database of hospitalized patients in China. Arthritis Res Ther. 2018;20(1):226.
- Ormerod AS, Cook MC. Epidemiology of primary systemic vasculitis in the Australian Capital Territory and South-Eastern New South Wales. Intern Med J. 2008;38(11):816–823.
- Reinhold-Keller E, Zeidler A, Gutfleisch J, et al. Giant cell arteritis is more prevalent in urban than in rural populations: results of an epidemiological study of primary systemic vasculitides in Germany. Rheumatology. 2000;39(12):1396–1402.
- Kobayashi S, Fujimoto S. Epidemiology of vasculitides: differences between Japan, Europe and North America. Clin Exp Nephrol. 2013;17(5):611–614.
- Knight A, Ekbom A, Brandt L, et al. Increasing incidence of Wegener's granulomatosis in Sweden, 1975-2001. J Rheumatol. 2006;33(10):2060–2063.
- Pearce FA, Lanyon PC, Grainge MJ, et al. Incidence of ANCA-associated vasculitis in a UK mixed ethnicity population. Rheumatology. 2016;55(9):1656–1663.
- Mohammad AJ. An update on the epidemiology of ANCA-associated vasculitis. Rheumatology. 2020;59(Suppl 3):iii42–iii50.
- Shi L. Anti-neutrophil cytoplasmic antibody-associated vasculitis: prevalence, treatment, and outcomes. Rheumatol Int. 2017;37(11):1779–1788.
- Robson J, Doll H, Suppiah R, et al. Damage in the anca-associated vasculitides: long-term data from the European Vasculitis study group (EUVAS) therapeutic trials. Ann Rheum Dis. 2015;74(1):177–184.
- Cohen CD, Frach K, Schlondorff D, et al. Quantitative gene expression analysis in renal biopsies: a novel protocol for a high-throughput multicenter application. Kidney Int. 2002;61(1):133–140.
- Grayson PC, Eddy S, Taroni JN, et al. Metabolic pathways and immunometabolism in rare kidney diseases. Ann Rheum Dis. 2018;77(8):1266–1233.
- Yu G, Wang LG, Han Y, et al. clusterProfiler: an R package for comparing biological themes among gene clusters. OMICS. 2012;16(5):284–287.
- Szklarczyk D, Gable AL, Nastou KC, et al. The STRING database in 2021: customizable protein-protein networks, and functional characterization of user-uploaded gene/measurement sets. Nucleic Acids Res. 2021;49(D1):D605–D612.
- Shannon P, Markiel A, Ozier O, et al. Cytoscape: a software environment for integrated models of biomolecular interaction networks. Genome Res. 2003;13(11):2498–2504.
- ANCA-associated vasculitis. Nat Rev Dis Primers. 2020;6(1):72.
- Watts RA, Lane SE, Bentham G, et al. Epidemiology of systemic vasculitis: a ten-year study in the United Kingdom. Arthritis Rheum. 2000;43(2):414–419.
- Chen YX, Chen N. Pathogenesis of rapidly progressive glomerulonephritis: what do we learn? Contrib Nephrol. 2013;181:207–215.
- Chen YX, Yu HJ, Zhang W, et al. Analyzing fatal cases of chinese patients with primary antineutrophil cytoplasmic antibodies-associated renal vasculitis: a 10-year retrospective study. Kidney Blood Press Res. 2008;31(5):343–349.
- Jariwala M, Laxer RM. Childhood GPA, EGPA, and MPA. Clin Immunol. 2020;211:108325.
- Booth AD, Almond MK, Burns A, et al. Outcome of ANCA-associated renal vasculitis: a 5-year retrospective study. Am J Kidney Dis. 2003;41(4):776–784.
- Chen YX, Zhang W, Chen XN, et al. Clinical analysis of ANCA-associated renal vasculitis patients with chronic dialysis. Clin Exp Rheumatol. 2014;32(3 Suppl 82):S5–S10.
- Lionaki S, Blyth ER, Hogan SL, et al. Classification of antineutrophil cytoplasmic autoantibody vasculitides: the role of antineutrophil cytoplasmic autoantibody specificity for myeloperoxidase or proteinase 3 in disease recognition and prognosis. Arthritis Rheum. 2012;64(10):3452–3462.
- Novick TK, Chen M, Scott J, et al. Patient outcomes in renal-limited antineutrophil cytoplasmic antibody vasculitis with inactive histology. Kidney Int Rep. 2018;3(3):671–676.
- Li ZY, Ma TT, Chen M, et al. The prevalence and management of anti-neutrophil cytoplasmic antibody-associated vasculitis in China. Kidney Dis. 2016;1(4):216–223.
- Wu J, Pei Y, Rong L, et al. Clinicopathological analysis of 34 cases of primary antineutrophil cytoplasmic antibody-associated vasculitis in Chinese children. Front Pediatr. 2021;9:656307.
- Mathern DR, Heeger PS. Molecules great and small: the complement system. Clin J Am Soc Nephrol. 2015;10(9):1636–1650.
- Kettritz R. With complements from ANCA mice. J Am Soc Nephrol. 2014;25(2):207–209.
- Brilland B, Garnier AS, Chevailler A, et al. Complement alternative pathway in ANCA-associated vasculitis: Two decades from bench to bedside. Autoimmun Rev. 2020;19(1):102424.
- Trivioli G, Vaglio A. The rise of complement in ANCA-associated vasculitis: from marginal player to target of modern therapy. Clin Exp Immunol. 2020;202(3):403–406.
- Moiseev S, Lee JM, Zykova A, et al. The alternative complement pathway in ANCA-associated vasculitis: further evidence and a meta-analysis. Clin Exp Immunol. 2020;202(3):394–402.
- Chalkia A, Thomas K, Giannou P, et al. Hypocomplementemia is associated with more severe renal disease and worse renal outcomes in patients with ANCA-associated vasculitis: a retrospective cohort study. Ren Fail. 2020;42(1):845–852.
- Chen Z, Lin L, Yang W, et al. Clinical characteristics and prognostic risk factors of anti-neutrophil cytoplasmic antibody (ANCA)-associated vasculitides (AAV). Int Immunopharmacol. 2020;87:106819.
- Tesar V, Hruskova Z. Avacopan in the treatment of ANCA-associated vasculitis. Expert Opin Investig Drugs. 2018;27(5):491–496.
- Popa ER, Tervaert JW. The relation between Staphylococcus aureus and Wegener's granulomatosis: current knowledge and future directions. Intern Med. 2003;42(9):771–780.
- Kakoullis L, Parperis K, Papachristodoulou E, et al. Infection-induced myeloperoxidase specific antineutrophil cytoplasmic antibody (MPO-ANCA) associated vasculitis: a systematic review. Clin Immunol. 2020;220:108595.
- Azar MM, Muse VV, Villalba JA, Turbett SE. Case 2-2020: a 64-year-old man with fever and respiratory failure. N Engl J Med. 2020;382(3):276–285.
- Izci Duran T, Turkmen E, Dilek M, et al. ANCA-associated vasculitis after COVID-19. Rheumatol Int. 2021;41(8):1523–1529.
- Sekar A, Campbell R, Tabbara J, et al. ANCA glomerulonephritis post moderna COVID-19 vaccination. Kidney Int. 2021;100(2):473–474.
- Uppal NN, Kello N, Shah HH, et al. De novo ANCA-associated vasculitis with glomerulonephritis in COVID-19. Kidney Int Rep. 2020;5(11):2079–2083.
- Powell WT, Campbell JA, Ross F, et al. Acute ANCA vasculitis and asymptomatic COVID-19. Pediatrics. 2021;147(4):e2020033092.
- Xu ZG, Li WL, Wang X, et al. Systemic lupus erythematosus and antineutrophil cytoplasmic antibody-associated vasculitis overlap syndrome in a 77-year-old man: a case report. World J Clin Cases. 2021;9(3):707–713.
- Kato K, Kawamura T, Terashima R, et al. A case of systemic lupus erythematosus/antineutrophil cytoplasmic antibody-associated vasculitis overlap syndrome with dissociated pathological and immunological findings. Case Rep Nephrol. 2020;2020(5698708):5698708.
- Moon JS, Ahn SS, Park YB, et al. C-Reactive protein to serum albumin ratio is an independent predictor of all-cause mortality in patients with ANCA-Associated vasculitis. Yonsei Med J. 2018;59(7):865–871.
- Dietrich J, Cella M, Seiffert M, et al. Cutting edge: signal-regulatory protein beta 1 is a DAP12-associated activating receptor expressed in myeloid cells. J Immunol. 2000;164(1):9–12.
- Mulrooney TJ, Posch PE, Hurley CK. DAP12 impacts trafficking and surface stability of killer immunoglobulin-like receptors on natural killer cells. J Leukoc Biol. 2013;94(2):301–313.
- Bouchon A, Dietrich J, Colonna M. Cutting edge: inflammatory responses can be triggered by TREM-1, a novel receptor expressed on neutrophils and monocytes. J Immunol. 2000;164(10):4991–4995.
- Bustamante J, Arias AA, Vogt G, et al. Germline CYBB mutations that selectively affect macrophages in kindreds with X-linked predisposition to tuberculous mycobacterial disease. Nat Immunol. 2011;12(3):213–221.
- Lyons PA, Rayner TF, Trivedi S, et al. Genetically distinct subsets within ANCA-associated vasculitis. N Engl J Med. 2012;367(3):214–223.
- Lyons PA, Peters JE, Alberici F, et al. Genome-wide association study of eosinophilic granulomatosis with polyangiitis reveals genomic loci stratified by ANCA status. Nat Commun. 2019;10(1):5120.
- Luo H, Chen M, Yang R, et al. The association of HLA-DRB1 alleles with antineutrophil cytoplasmic antibody-associated systemic vasculitis in Chinese patients. Hum Immunol. 2011;72(5):422–425.
- Wu Z, Wu Q, Xu J, et al. HLA-DPB1 variant rs3117242 is associated with anti-neutrophil cytoplasmic antibody-associated vasculitides in a Han Chinese population. Int J Rheum Dis. 2017;20(8):1009–1015.