Abstract
Critically ill patients with preexisting kidney dysfunction (PKD) are at high risk for acute kidney injury (AKI). Nevertheless, there is no criteria for screening and classifying AKI in patients with PKD. In this study, after assessing relationship between the change in SCr from baseline and in-hospital mortality, a new criteria, named APKD, for identifying AKI in PKD was proposed. APKD defined AKI in critically ill patients with PKD as an absolute increase of ≥ 0.2 mg/dL in SCr within 48 h or an increase in SCr ≥ 1.1 times over baseline within 7 d. APKD detected more AKI among PKD patients compared with the other criteria. Additionally, the AKI patients identified by APKD but missed by the other criteria had higher mortality than those without AKI. APKD shows higher sensitivities than KDIGO criteria in predicating in-hospital mortality. APKD, but not the KDIGO, is effective for staging the severity of AKI in patients with PKD. In conclusion, APKD is more effective in screening and classifying AKI in critically ill patients with PKD compared with the earlier criteria, and it may helpful in guiding clinical treatment and predicting prognosis.
Introduction
Acute kidney injury (AKI), characterized by sudden decline in kidney function, is a common disease in critically ill patients [Citation1–6]. In the past decades, many AKI diagnostic criteria have been issued to guide clinical work, such as Risk, Injury, Failure, Loss, End stage kidney disease (RIFLE) criteria, Acute Kidney Injury Network (AKIN) criteria and, more recently, Kidney Disease: Improving Global Outcomes (KDIGO) criteria [Citation7–10]. In addition to the volume of urine output (UO), the change in serum creatinine (SCr) from baseline is recommended for the identification and staging the severity of AKI [Citation7–10].
Baseline SCr (bSCr) used in these AKI criteria is to reflect the baseline renal function of patients before kidney injury [Citation11–14]. Patients with bSCr within the past 12 months yielding an estimated glomerular filtration rate (eGFR) <60 mL/min/1.73 m2 were considered having chronic kidney disease (CKD) [Citation15–19]. Some studies have paid attention to the particularity of CKD patients with subsequent AKI. Compared with AKI patients without CKD, patients with acute on CKD have significantly lower long-term survival rate and higher dialysis risk [Citation13,Citation20]. Nevertheless, the usefulness of KDIGO AKI definition and staging criteria in patients with prior kidney dysfunction (PKD) is not fully assessed.
A recent study found that the criteria based on the reference change value of SCr were more efficiency than KDIGO criteria in predicting the outcomes of AKI patients with previous CKD [21]. In intensive care unit (ICU) clinical practice, preadmission bSCr, especially the SCr levels during 7–365 d before admission, is usually unavailable [Citation11,Citation12]. Moreover, as a prerequisite for the diagnosis of CKD, the accurate duration of kidney dysfunction is also difficult to determine. In other words, a patient with decreased eGFR may have CKD, acute kidney dysfunction or both [Citation4]. Thus, how to identify AKI in these patients is an important clinical issue worth of research.
In the real world, the missing values of bSCr, and relatively few patients with PKD make it difficult for clinicians to explore the above issues. The public-access ICU database based on real-world electronic medical records allows us to investigate various questions that arise in ICU clinical practice [Citation22–24]. In this study, the critically ill subjects of Multiparameter Intelligent Monitoring in Intensive Care (MIMIC) III/IV databases and the eICU Collaborative Research Database (eICU-CRD) were enrolled to explore whether the KDIGO AKI criteria is applicable to patients with preexisting kidney dysfunction (PKD). If not, a new criterion will be designed to identify and classify acute on preexisting kidney dysfunction (APKD).
Methods
Data sources
This retrospective observational study was conducted using the data stored in the MIMIC-III version 1.4, MIMIC-IV version 1.0 (Boston, MA, USA), and eICU databases version 2.0 (NY, USA). MIMIC-III and MIMIC-IV databases are large, single-center databases containing high-quality data on ICU inpatients of Beth Israel Deaconess Medical Center (BIDMC) [Citation22,Citation23]. eICU-CRD is a multicenter database that records the health data of critically ill patients admitted to 335 units at 208 hospitals located throughout the United States [Citation24].
Study population
Patients who have the data of bSCr were enrolled in this study. For patients with multiple hospitalizations, only the information of their first ICU admission was analyzed. The exclusion criteria are as follows: (1) Age <18 years; (2) ICU length of stay < 24-h; (3) History of renal transplant; (4) SCr measurement less than 2 times during ICU stay. The flow chart of included patients was shown in Supplemental Figure 1.
Data collection
Data extraction was performed in Postgre SQL database management system using Structured Query Language (SQL). Clinical data at ICU admission, including age, gender, ethnicity, comorbidities, Simplified Acute Physiology Score II (SAPS-II) score, sequential organ failure assessment (SOFA) score, and the use of vasopressors, were recorded, respectively. eGFR was estimated based on bSCr levels and was calculated by using (CKD epidemiology collaboration) CKD-EPI creatinine equation [Citation25–28]. All SCr measurements taken during the patient’s ICU stay, within 7-d prior to ICU stay and within the 12 months prior to hospital stay were collected. Death occurred during the hospital stay was recorded.
Definitions
In this study, preadmission baseline SCr (pbSCr) was defined as the mean SCr during 7–365 d before admission [Citation11,Citation12], and the dynamic SCr within 48-h or 7-d window was referred as dynamic baseline SCr (dbSCr) [Citation10]. We defined PKD as eGFR <60 mL/min per 1.73 m2 calculated based on dbSCr or pbSCr [Citation15–19].
Three criteria were used to screen AKI in this study, including KDIGO criteria, cROCK criteria, and APKD criteria. the KDIGO criteria defined AKI as an increase in SCr by ≥0.3 mg/dL within 48 h, or an increase in SCr up to 1.5 times within the previous 7 d [Citation10]. cROCK criteria ares a criterion which is proposed based on the reference change value of SCr. cROCK criteria defined an AKI in CKD as a ≥ 25% increase in SCr in 7 d [Citation21]. APKD criteria are the criteria proposed by this study which defined AKI as absolute increase in SCr of ≥ 0.2 mg/dL within 48 h or an increase in SCr ≥1.1 times over the bSCr value within 7 d. According to KDIGO criteria, AKI Stage 2 was defined as a peak to baseline ratio of 2.0–2.9 within 7 d. Increasing in SCr to 3 times baseline, or SCr value more than 4.0 mg/dL, or receiving renal replacement treatment were classified as KDIGO Stage 3 [10]. The APKD criteria define AKI Stages 2 and 3 as SCr increases of ≥1.3 times and ≥1.9 times, respectively.
Sepsis in this study was defined as suspected or documented infection together with SOFA score ≥ 2 according to Sepsis-3 criteria [Citation29,Citation30].
Statistical analysis
Among the three databases, the data of MIMIC-IV database was initially used to explore the applicability of AKI criteria in patients with or without PKD. MIMIC-III and eICU databases were used as external verification databases.
Continuous variables are presented as the means [standard deviations] or medians [interquartile range (IQR)]. Categorical variables were expressed as the number and percentage. Comparisons between groups were made by using Mann–Whitney test for continuous variables, and for categorical variables, the Chi-square test or Fishers’ exact test were performed, as appropriate.
The performance of the three AKI definitions was compared in predicting the risk of in-hospital mortality using logistic regression models. Four models were fitted to determine the potential impact of con-founders on the relationship between AKI and the outcomes. Model 1: unadjusted for any con-founders. Model 2: adjusted for age and gender. Model 3: adjusted for age, gender, and bSCr. Model 4: multivariate con-founders were adjusted, including admission age, gender, bSCr, comorbidities (myocardial infarct, congestive heart failure, liver disease, cancer, and tumor), sepsis, hypertension, vasopressors use, RRT, and SAPSII score. We determined the optimal threshold for prediction of inhospital mortality by using the Youden-Index. Based on the cutoff point, the areas under the receiver operating characteristic curve (AUCs), and the sensitivities of the models were computed and compared [Citation31–33].
Three sets of sensitivity analyses were performed to further verify the usefulness of the three criteria in patients with PKD. First, Models 2 and 3 were used to assess the applicability of the three criteria in screening AKI and staging AKI severity. Second, the patients with sepsis-induced AKI were analyzed as an independent cohort [Citation34–37]. Finally, in addition to dbSCr, pbSCr was used to calculate eGFR. Then, the differences between the three criteria in screening AKI and staging AKI severity were compared.
Statistical analyses were performed with the SPSS software version 26.0 (SPSS Inc., Chicago, IL) and the R software version R4.1.2 for windows. In this study, two-sided p value p < 0.05 was considered statistically significant.
Results
Usefulness of KDIGO AKI definition and staging criteria in patients with and without prior kidney dysfunction
After reviewing 76,540 ICU admissions in the MIMIC-IV cohort, 32,794 patients were included in this study. There were 9795 patients had PKD, and AKI was identified in 6108 (62.4%) patients: 33.4% with Stage 1, 3.8% with Stage 2, and 25.1% with Stage 3. The detailed clinical characteristics and demographics of the present cohort are presented in .
Table 1. Patient demographics and clinical characteristics.
Crude in-hospital mortality increased with the increase of the stages of AKI among patients without PKD (10.9% vs. 22.6% vs. 37.5%, p < 0.001). However, among patients with PKD, the mortality rates of patients with KDIGO Stage 2 was higher than those with stage 3(37.8% vs. 30.2%, p = 0.003) (Supplemental Figure 2). The results of logistic regression analysis showed that PKD patients with AKI Stage 2 presented higher OR compared with those with AKI Stage 3 (Unadjusted OR, 4.82; 95% CI, 3.82–6.08 vs. OR, 3.45; 95% CI, 3.02–3.94) (Supplemental Figure 3). The results were validated in MIMIC-III and e-ICU cohorts (Supplemental Figure 4).
A new criterion for the definition and classification of APKD
Generalized additive models showed that the SCr ratio (pSCr/bSCr) and the absolute rising values of SCr (pSCr-bSCr) had nonlinear and asymmetric relationships with mortality ()). Then, patients were divided into 16 groups and 21 groups on the basis of the SCr ratio and the absolute change values of SCr, respectively.
Figure 1. Association of the ratio of pSCr/bSCr and the absolute increase in SCr (pSCr-bSCr) with in-hospital mortality in patients with PKD. PKD: preexisting kidney dysfunction. pSCr: peak serum creatinine; bSCr: baseline serum creatinine.
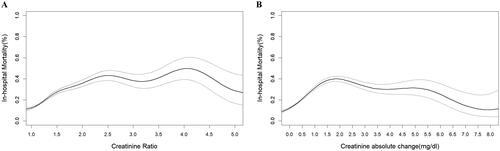
As shown in Supplemental Figure 5, in MIMIC-IV cohorts, the hospital mortality of patients with absolute increase in SCr of 0.1–0.19 mg/dL was comparable to those with 0.0–0.1 mg/dL (9.1% vs. 11.2%, p = 0.151), but was lower than those with absolute change in SCr ≥ 0.2 mg/dL (11.2% vs. 17.0%, p < 0.001). Additionally, patients with the SCr ratio of 1.1–1.19 had higher hospital mortality compared those with the SCr ratio of 1.0–1.09 (11.9% vs. 15.9%, p < 0.001), but the mortality was similar between 1.1–1.19 group and 1.2–1.29 group (15.9% vs. 17.5%, p = 0.213). There is a significant difference in mortality between 1.2–1.29 group and 1.3–1.39 group (17.5% vs. 21.9%, p = 0.012). According to these results, an absolute increase in SCr of ≥ 0.2 mg/dL within 48 h or an increase in SCr ≥1.1 times over baseline within 7 d was considered for the diagnosis of AKI among patients with PKD. An increase in SCr ≥1.3 times and ≥1.9 times baseline were defined as APKD Stages 2 and 3, respectively.
Comparison of the incidence and outcomes of AKI defined by different criteria among patients with PKD
In MIMIC-IV cohort, the incidence of AKI in patients with PKD based on KDIGO, cROCK, and APKD were 62.4%, 46.3%, and 78.3%, respectively. Compared with KDIGO and cROCK criteria, APKD identified 1563 (15.9%) and 3138 (32.0%) more AKI patients. In MIMIC-III and e-ICU cohort, APKD also detected more AKI among PKD patients compared with KDIGO or cROCK.
The crude in-hospital mortality of AKI patients diagnosed based on the three criteria was significantly higher than that of non-AKI patients (all p < 0.001). Nevertheless, as shown in , the patients identified by AKPD but missed by KDIGO or cROCK had higher mortality than those with no AKI based on APKD in MIMIC-III/IV and e-ICU cohorts (all p < 0.01).
Table 2. Comparison of the incidence and outcomes of AKI defined by different criteria among patients with PKD.
Comparing the performance of in-hospital mortality predictive models between APKD and KDIGO staging criteria
As shown in , AKI defined by APKD and KDIGO criteria showed similar risk of death (OR, 2.40; 95% CI, 2.05–2.81 and OR, 2.43; 95% CI, 2.15–2.75). Apart from this, no significant differences in the AUC between the APKD and KDIGO predictive models were observed in MIMICIV cohorts (AUC = 0.730 vs. 0.731, p = 0.868). Nevertheless, using the maximum value of Youden index (Sensitivity + Specificity − 1) as a criterion for selecting the optimum cutoff value. APKD staging criteria show higher sensitivities than KDIGO criteria in predicating in-hospital mortality (0.669 vs. 0.638, p < 0.001).
Table 3. Performance of in-hospital mortality predictive models between APKD and KDIGO in different databases.
APKD criteria in staging the severity of AKI in patients with prior kidney dysfunction
According to APKD criteria, there were 3737(38.1%) patients with AKI Stage 1, 2750 (20.1%) with Stage 2 and 1184 (12.1%) with Stage 3. The mortality of no-APKD and APKD stage 1–3 were 10.0%, 16.8%, 25.1%, and 38.4%, respectively. Crude in-hospital mortality of AKI patients diagnosed based on APKD criteria was higher than that with non-AKI, and it increased with the increase of the stages of APKD (). Using the logistic regression model, the risk of death was also increased with the increase of the stages of APKD (OR [95% CI]; 1.81 [1.54–2.14], OR [95% CI]; 3.00 [2.55–3.55], and OR [95% CI]; 5.60 [4.67–6.74]) (). The applicability of the APKD criteria in staging the severity of APKD patients was also confirmed in MIMIC-III and e-ICU cohorts (Supplemental Figures 6 and 7).
Sensitivity analysis
Three sets of sensitivity analyses were performed to evaluate the usefulness of APKD criteria.
First, three multivariate logistic regression models (Models 2–4) were used to analysis, the associations between AKI stages according to APKD and KDIGO and in-hospital mortality. Similar results were obtained from the unadjusted and adjusted models (Supplemental Figure 8).
Sepsis is one of the main causes of AKI among critically illness patients [Citation34–37]. Compared with KDIGO and cROCK, APKD detected more AKI in septic patients. The results also showed that, the crude mortality and unadjusted and adjusted ORs for death were similar between KDIGO AKI Stages 2 and 3 among patients with PKD (Supplemental Figures S9(A) and S10(A)). Nevertheless, the APKD staging criteria applied to this study population showed a good separation between non-AKI and different stages of AKI (Supplemental Figure S11).
Finally, the results were validated when eGFR were calculated based on the values of pbSCr. When using pbSCr-based eGFR, there were 2549 (44.6%), 2241 (39.2%), and 3142 (54.9%) AKI subjects met KDIGO, cROCK, and APKD criteria, respectively. APKD AKI staging criteria, but not the KDIGO staging criteria, is effective for staging the severity of patients with eGFR less than 60 mL/min/1.73 m2 (Supplemental Figure S9(B) and Figure S10(B), and Figure S12).
Discussion
Accurate identification of AKI and staging of its severity are crucial for guiding clinical treatment and predicting prognosis. According to KDIGO criteria, the severity of AKI was classified according to the increased levels of SCr and decreased urinary volume [Citation10]. In many studies, including randomized controlled trials, SCr-based criteria were used to enroll patients with AKI and classify their severity [Citation20,Citation38,Citation39]. Nevertheless, the efficiency of SCr-based criteria in screening AKI may be influenced by the baseline kidney function. In this study, a new criterion was proposed to define AKI in patients with prior kidney dysfunction (APKD criteria). The results showed that APKD could detect about 15% more AKI events in patients with PKD than KDIGO. According to cROCK, criteria for acute on CKD, more than one half of patients with PKD are defined as non-AKI, and APKD could identify about 30% more AKI events than it. Moreover, the mortality of patients with AKI identified by APKD, but not KDIGO and cROCK, was significantly higher than non-AKI patients.
The levels of bSCr or reference SCr vary from patient to patient. Thus, instead of the peak of SCr, increasing SCr from baseline is used as the marker to diagnosis AKI in all AKI criteria [Citation7–10]. Nevertheless, the normal variability of it should also be considered. The reference change value of SCr is within the range of 14–25% in healthy adults, and it was estimated at 20–30% in patients with eGFR< 60 mL/min/1.73 m2 [21]. Accordingly, a criterion based on the reference change value of SCr was proposed to define AKI in patients with CKD [21]. However, only using the distribution of reference change value to determine the cutoff value of it for the diagnosis of AKI maybe not reasonable for critically ill patients, because they are at high risk for AKI. For them, the normal variability of reference change value estimated by the distribution of it may be not ‘normal’. In this study, we analyzed the relationship between reference change value and in-hospital mortality, and the differences in mortality between different ranges of reference change value were carefully compared. The results showed that an absolute change values ≥ 0.2 mg/dL within 48 h or an increase in SCr ≥1.1 times over baseline within 7 d are associated with increased mortality in critically ill patients. Interestingly, a recent study found that a very small variation of SCr (>0.05 mg/dL) was associated with increased in-hospital mortality in the novel coronavirus disease 2019 (COVID-19) patients [Citation40].
Currently, the variability of bSCr definition in different studies may lead to heterogeneity in reported AKI frequency and prognosis. dbSCr and pbSCr are widely used as reference SCr for diagnosing and staging AKI [Citation11,Citation20,Citation39,Citation41]. While both dbSCr and pbSCr are unavailable, the equations, including CKD-EPI and MDRD, are recommended to estimating the value of bSCr (estimated bSCr) [Citation8,Citation42–45]. Nevertheless, this back-estimation method defaults that the basic renal function of the patient is normal, which undoubtedly leads to an erroneous estimation of the bSCr of some patients [Citation25,Citation27,Citation28,Citation46]. Thus, only patients with actual measured SCr values were included in this study. We found that, although the values of dbSCr and pbSCr are different in some patients (data not shown), APKD criteria is effective in screening and classifying AKI in patients with PKD. Moreover, the usefulness of APKD criteria was also validated in different populations and in different databases.
There are some limitations of this study. First, as an observational study using the data of electronic databases, the results might be affected by residual confounding factors that are not adjusted or cannot be adjusted. Second, UO-based criteria are not used for diagnosing AKI in this study. Some studies found that diuresis is a more sensitive marker of AKI than SCr [Citation47–49]. Nevertheless, in the present cohort, using KDIGO UO criteria, there is no significantly difference in in-hospital mortality between PKD patients with AKI Stage 1 and those without AKI (10.2% vs. 8%, p = 0.058). The mortality of the patients with AKI Stages 1 and 2 is also similar, although there is a statistical difference (10.2% vs. 13.4%, p = 0.024). Therefore, how to identify AKI in patients with PKD using UO-based criteria deserves further study. Thirdly, although in-hospital mortality was recorded as the endpoint of AKI, economic and long-term mortality and quality-of-life of the patients were not recorded. Finally, the APKD criteria need to be further validated in non-ICU patients.
Conclusions
APKD is more effective in screening and classifying AKI in critically ill patients with PKD compared with earlier criteria. The results need to be verified in the future by multicenter randomized controlled trials.
Ethical approval
MIMIC III/IV database used in this study was approved by the Institutional Review Boards (IRB) of the Massachusetts Institute of Technology and does not contain protected health information. Details of the ethical activities of the eICU database are available on the official website (https://eicu-crd.mit.edu).
Author contributions
Conception and design: G Zhao; (II) Administrative support: Z Lu, Y Yao; (III) Provision of study materials or patients: G Zhao, D Hong; (IV)Collection and assembly of data: D Hong, J Zhang, F Dong, S Chen, W Dong, X Chen, L Chen; (V) Data analysis and interpretation: G Zhao, D Hong; (VI) Manuscript writing: All authors; (VII) Final approval of manuscript: All authors.
Supplemental Material
Download PDF (743.1 KB)Disclosure statement
No potential conflict of interest was reported by the author(s).
Data availability statement
Source data for information presented in this study are available from the corresponding authors on reasonable request.
Additional information
Funding
References
- Ronco C, Bellomo R, Kellum JA. Acute kidney injury. Lancet. 2019;394(10212):1949–1964.
- Hoste EA, Bagshaw SM, Bellomo R, et al. Epidemiology of acute kidney injury in critically ill patients: the multinational AKI-EPI study. Intens Care Med. 2015;41(8):1411–1423.
- Soliman IW, Frencken JF, Peelen LM, et al. The predictive value of early acute kidney injury for long-term survival and quality of life of critically ill patients. Crit Care. 2016;20(1):242.
- Kellum JA, Romagnani P, Ashuntantang G, et al. Acute kidney injury. Nat Rev Dis Primers. 2021;7(1):52.
- Pickkers P, Darmon M, Hoste E, et al. Acute kidney injury in the critically ill: an updated review on pathophysiology and management. Intensive Care Med. 2021;47(8):835–850.
- Bellomo R, Kellum JA, Ronco C. Acute kidney injury. Lancet. 2012;380(9843):756–766.
- Mehta RL, Kellum JA, Shah SV, et al. Acute Kidney injury network: report of an initiative to improve outcomes in acute kidney injury. Crit Care. 2007;11(2):R31.
- Bellomo R, Ronco C, Kellum JA, et al. Acute renal failure - definition, outcome measures, animal models, fluid therapy and information technology needs: the second international consensus conference of the acute dialysis quality initiative (ADQI) group. Crit Care. 2004;8(4):R204–12.
- Stevens PE, Levin A, Kidney Disease: Improving Global Outcomes Chronic Kidney Disease Guideline Development Work Group Members. Evaluation and management of chronic kidney disease: synopsis of the kidney disease: improving global outcomes 2012 clinical practice guideline. Ann Intern Med. 2013;158(11):825–830.
- Disease KJKI. Kidney Disease: improving global outcomes (KDIGO). Acute Kidney Injury Work Group. 2012;2:1–138.
- Thomas ME, Blaine C, Dawnay A, et al. The definition of acute kidney injury and its use in practice. Kidney Int. 2015;87(1):62–73.
- Siew ED, Ikizler TA, Matheny ME, et al. Estimating baseline kidney function in hospitalized patients with impaired kidney function. Clin J Am Soc Nephrol. 2012;7(5):712–719.
- Acosta-Ochoa I, Bustamante-Munguira J, Mendiluce-Herrero A, et al. Impact on outcomes across KDIGO-2012 AKI criteria according to baseline renal function. JCM. 2019;8(9):1323.
- Warnock DG, Neyra JA, Macedo E, et al. Comparison of static and dynamic baseline creatinine surrogates for defining acute kidney injury. Nephron. 2021;145(6):664–674.
- Drawz P, Rahman M. Chronic kidney disease. Ann Intern Med. 2015;162(11):Itc1–16.
- Foundation NK K/DOQI clinical practice guidelines for chronic kidney disease: evaluation, classification, and stratification. J Am J Kidney Dis. 2002;39(2):S1–S266.
- Levin A, Stevens PE, Bilous RW, et al. Kidney disease: improving global outcomes (KDIGO) CKD work group. Nat Rev Nephrol. 2013;3(1):1–150.
- Liu P, Quinn RR, Lam NN, et al. Accounting for age in the definition of chronic kidney disease. JAMA Intern Med. 2021;181(10):1359–1366.
- KDIGO 2020 clinical practice guideline for diabetes management in chronic kidney disease. Kidney Int. 2020;98(4s):S1–s115.
- Wu VC, Huang TM, Lai CF, et al. Acute-on-chronic kidney injury at hospital discharge is associated with long-term dialysis and mortality. Kidney Int. 2011;80(11):1222–1230.
- Xu X, Wang L, Xiong M, et al. New Criterion to evaluate acute-on-chronic kidney injury based on the creatinine reference change. Am J Nephrol. 2020;51(6):453–462.
- Johnson AE, Pollard TJ, Shen L, et al. MIMIC-III, a freely accessible critical care database. Sci Data. 2016;3:160035.
- Johnson AEW, Bulgarelli L, Shen L, et al. MIMIC-IV, a freely accessible electronic health record dataset. Sci Data. 2023;10(1):1.
- Pollard TJ, Johnson AEW, Raffa JD, et al. The eICU collaborative research database, a freely available multi-center database for critical care research. Sci Data. 2018;5:180178.
- Michels WM, Grootendorst DC, Verduijn M, et al. Performance of the Cockcroft-Gault, MDRD, and new CKD-EPI formulas in relation to GFR, age, and body size. Clin J Am Soc Nephrol. 2010;5(6):1003–1009.
- Meeusen JW, Kasozi RN, Larson TS, et al. Clinical impact of the refit CKD-EPI 2021 creatinine-based eGFR equation. Clin Chem. 2022;68(4):534–539.
- Levey AS, Inker LA, Coresh J. GFR estimation: from physiology to public health. Am J Kidney Dis. 2014;63(5):820–834.
- Schwandt A, Denkinger M, Fasching P, et al. Comparison of MDRD, CKD-EPI, and Cockcroft-Gault equation in relation to measured glomerular filtration rate among a large cohort with diabetes. J Diabet Complicat. 2017;31(9):1376–1383.
- Singer M, Deutschman CS, Seymour CW, et al. The Third international consensus definitions for sepsis and septic shock (sepsis-3). JAMA. 2016;315(8):801–810.
- Shankar-Hari M, Phillips GS, Levy ML, et al. Developing a new definition and assessing new clinical criteria for septic shock: for the third international consensus definitions for sepsis and septic shock (sepsis-3). JAMA. 2016;315(8):775–787.
- Wang Q, Tang Y, Zhou J, et al. A prospective study of acute kidney injury in the intensive care unit: development and validation of a risk prediction model. J Transl Med. 2019;17(1):359.
- Sun SQ, Zhang T, Ding D, et al. Circulating microRNA-188, -30a, and -30e as early biomarkers for contrast-induced acute kidney injury. JAHA. 2016;5(8):e004138.
- Kwak S, Kim JY, Cho H. Vancomycin-induced nephrotoxicity in non-intensive care unit pediatric patients. Sci Rep. 2021;11(1):20681.
- Bellomo R, Kellum JA, Ronco C, et al. Acute kidney injury in sepsis. Intensive Care Med. 2017;43(6):816–828.
- Poston JT, Koyner JL. Sepsis associated acute kidney injury. BMJ. 2019;364:k4891.
- Peerapornratana S, Manrique-Caballero CL, Gómez H, et al. Acute kidney injury from sepsis: current concepts, epidemiology, pathophysiology, prevention and treatment. Kidney Int. 2019;96(5):1083–1099.
- Zarjou A, Agarwal A. Sepsis and acute kidney injury. J Am Soc Nephrol. 2011;22(6):999–1006.
- Xu X, Nie S, Zhang A, et al. A New criterion for pediatric AKI based on the reference change value of serum creatinine. J Am Soc Nephrol. 2018;29(9):2432–2442.
- Sparrow HG, Swan JT, Moore LW, et al. Disparate outcomes observed within kidney disease: improving global outcomes (KDIGO) acute kidney injury stage 1. Kidney Int. 2019;95(4):905–913.
- Alfano G, Ferrari A, Fontana F, et al. Twenty-four-hour serum creatinine variation is associated with poor outcome in the novel coronavirus disease 2019 (COVID-19) patients. Kidney Res Clin Pract. 2021;40(2):231–240.
- Wahl TS, Graham LA, Morris MS, et al. Association between preoperative proteinuria and postoperative acute kidney injury and readmission. JAMA Surg. 2018;153(9):e182009.
- Levey AS, Coresh J, Greene T, et al. Using standardized serum creatinine values in the modification of diet in renal disease study equation for estimating glomerular filtration rate. Ann Intern Med. 2006;145(4):247–254.
- Levey AS, Bosch JP, Lewis JB, et al. A more accurate method to estimate glomerular filtration rate from serum creatinine: a new prediction equation. Modification of diet in renal disease study group. Ann Intern Med. 1999;130(6):461–470.
- Imai E, Horio M, Nitta K, et al. Modification of the modification of diet in renal disease (MDRD) study equation for Japan. Am J Kidney Dis. 2007;50(6):927–937.
- Závada J, Hoste E, Cartin-Ceba R, et al. A comparison of three methods to estimate baseline creatinine for RIFLE classification. Nephrol Dial Transplant. 2010;25(12):3911–3918.
- Bagshaw SM, Uchino S, Cruz D, et al. A comparison of observed versus estimated baseline creatinine for determination of RIFLE class in patients with acute kidney injury. Nephrol Dial Transplant. 2009;24(9):2739–2744.
- Macedo E, Malhotra R, Bouchard J, et al. Oliguria is an early predictor of higher mortality in critically ill patients. Kidney Int. 2011;80(7):760–767.
- Prowle JR, Liu YL, Licari E, et al. Oliguria as predictive biomarker of acute kidney injury in critically ill patients. Crit Care. 2011;15(4):R172.
- Wlodzimirow KA, Abu-Hanna A, Slabbekoorn M, et al. A comparison of RIFLE with and without urine output criteria for acute kidney injury in critically ill patients. Crit Care. 2012;16(5):R200.