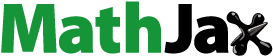
Abstract
Heart failure (HF) constitutes a major determinant of outcome in chronic kidney disease (CKD) patients. The main pattern of HF in CKD patients is preserved ejection fraction (HFpEF), and left ventricular diastolic dysfunction (LVDD) is a frequent pathophysiological mechanism and specific preclinical manifestation of HFpEF. Therefore, exploring and intervention of the factors associated with risk for LVDD is of great importance in reducing the morbidity and mortality of cardiovascular disease (CVD) complications in CKD patients. We designed this retrospective cross-sectional study to collect clinical and echocardiographic data from 339 nondialysis CKD patients without obvious symptoms of HF to analyze the proportion of asymptomatic left ventricular diastolic dysfunction (ALVDD) and its related factors associated with risk by multivariate logistic regression analysis. Among the 339 nondialysis CKD patients, 92.04% had ALVDD. With the progression of CKD stage, the proportion of ALVDD gradually increased. The multivariate logistic regression analysis revealed that increased age (OR 1.237; 95% confidence interval (CI) 1.108–1.381, per year), diabetic nephropathy (DN) and hypertensive nephropathy (HTN) (OR 25.000; 95% CI 1.355–48.645, DN and HTN vs chronic interstitial nephritis), progression of CKD stage (OR 2.785; 95% CI 1.228–6.315, per stage), increased mean arterial pressure (OR 1.154; 95% CI 1.051–1.268, per mmHg), increased urinary protein (OR 2.825; 95% CI 1.484–5.405, per g/24 h), and low blood calcium (OR 0.072; 95% CI 0.006–0.859, per mmol/L) were factors associated with risk for ALVDD in nondialysis CKD patients after adjusting for other confounding factors. Therefore, dynamic monitoring of these factors associated with risk, timely diagnosis and treatment of ALVDD can delay the progression to symptomatic HF, which is of great importance for reducing CVD mortality, and improving the prognosis and quality of life in CKD patients.
1. Introduction
Both the kidney and heart play extremely important roles in maintaining hemodynamics, water-electrolyte homeostasis, blood pressure (BP), and the balance of volume and circulation in the human body. Therefore, the presence of concurrent cardiac and kidney dysfunction is very common. Kidney dysfunction is a crucial factor in the development and prognosis of heart failure (HF), and HF constitutes a major determinant of outcome in patients with chronic kidney disease (CKD). The majority of CKD patients are at risk of accelerated cardiovascular disease (CVD) and mortality [Citation1]. Hospital mortality is significantly higher in CKD patients with HF than in those free of HF [Citation2], causing a heavy health and economic burdens.
Heart failure with preserved ejection fraction (HFpEF) accounts for up to approximately half of HF [Citation3–6], and will become the dominant form of HF with rising morbidity [Citation7,Citation8]. The main pattern of HF in CKD patients is HFpEF, and CKD has been considered as a phenotype of HFpEF [Citation9]. Although current HF guidelines of the European Society of Cardiology (ESC) proposed the HFA–PEFF algorithm to diagnose HFpEF [Citation10], HFpEF remains difficult to be detected and diagnosed early [Citation11–13]. Even more concerning, although the ejection fraction is retained in HFpEF, its prognosis is as poor as heart failure with reduced ejection fraction (HFrEF) [Citation14,Citation15], and it is associated with relatively poor health-related quality of life (HRQoL) compared with HFrEF [Citation16]. In contrast to HFrEF, when HFpEF progresses to obvious HF symptoms, no pharmacological therapy has been shown to improve the clinical symptoms and prognosis [Citation3,Citation10,Citation11]. Stages of HF include at-risk for HF (stage A), pre-HF (stage B), HF (stage C), and advanced HF (stage D) [Citation17]. The structural changes comprising stage B HF are relatively broad, including asymptomatic left ventricular systolic dysfunction (ALVSD) or asymptomatic left ventricular diastolic dysfunction (ALVDD) [Citation18]. Left ventricular diastolic dysfunction (LVDD) is the most common pathophysiological mechanism [Citation7,Citation8] and characteristics [Citation4,Citation7,Citation19] of HFpEF. LVDD may occur before ejection fraction drops or symptomatic HF occurs in CKD patients. Previous studies have mainly focused on the effect of LVDD on the prognosis of CKD patients [Citation20]. However, real-world evidence concerning the prevalence and related risk factors for LVDD in different causes of CKD has rarely been reported. Therefore, identification of factors associated with risk for ALVDD in the nondialysis CKD patients and intervention early before the onset of symptoms or entering the clinical stage of HF, can significantly reduce the prevalence and mortality of HFpEF in these patients.
Echocardiography can accurately assess heart function and identify patients at high risk for HF [Citation20]. Therefore, we collected clinical and echocardiographic data from nondialysis CKD patients without obvious symptoms of HF, and analyzed the proportion and factors associated with risk for ALVDD. Identifying and intervening in these factors associated with risk will reduce cardiovascular and all-cause mortality and improve the prognosis of CKD patients.
2. Materials and methods
Ethical approval: This study was approved by the Ethics Committee of the First Affiliated Hospital of Xi’an Jiaotong University (approval number MC-KYLLSL-2023-003) and was conducted in accordance with the ethical principles of the Declaration of Helsinki. All patients have signed the informed consents.
2.1. Research subjects
The inclusion criteria were as follows: nondialysis CKD patients without signs or symptoms of HF aged 20–70 years at study entry, from the Department of Nephrology of Xi’an Jiaotong University, between January and December 2022, including CKD stages 1–5. CKD is defined as abnormalities of kidney structure or function, present for >3 months, with implications for health [Citation21]. CKD stage was classified according to the estimated glomerular filtration rate (eGFR) [Citation22] recommended by KDIGO guidelines [Citation21]. Referring to the glomerular filtration function examination recommended by professor Haiyan Wang [Citation23] and the clinical practical application, we chose the CKD-EPI formula for the eGFR based on creatinine: eGFR (mL/min/1.73 m2) = 141 × min (Scr/κ, 1)α × max (Scr/κ, 1)−1.209 × 0.993Age × 1.018 [if female] × 1.159 [if black], where Scr is serum creatinine, κ is 0.7 for females and 0.9 for males, α is −0.329 for females and −0.411 for males, min indicates the minimum of Scr/κ or 1, and max indicates the maximum of Scr/κ or 1 [Citation22].
The exclusion criteria were as follows: previous symptoms of HF or arrhythmia, or history of other cardiovascular or cerebrovascular diseases, pregnant and lactating women, acute or chronic infection in the last 3 months, trauma, surgery, organ transplantation, history of malignant tumor, familial hyperlipidemia, history of blood transfusion or active bleeding in the last 1 month.
This study was a retrospective cross-sectional analysis. A total of 339 nondialysis CKD patients without symptoms of HF were included in the study (252 males and 87 females, with an average age of 47.0 ± 12.0 years). Diagnosis of the causes of CKD was based on clinical diagnosis or pathological diagnosis by renal biopsy, and were confirmed by a nephrologist. Different causes of CKD were coded as 1–4 (1: secondary renal disease caused by metabolic diseases: diabetic nephropathy (DN) or hypertensive nephropathy (HTN); 2: primary glomerulonephritis: chronic glomerulonephritis (CGN) or nephrotic syndrome (NS) or IgA nephropathy (IgAN); 3: tubulointerstitial nephropathy: chronic interstitial nephritis (CIN); and 4: polycystic kidney disease (PKD) or other causes of CKD). There were 135 patients with DN, 18 with HTN, 117 with CGN, 18 with NS, 18 with IgAN, 12 with CIN, 12 with PKD, and nine with other causes of CKD. There were 57 patients in CKD stage 1, 33 in CKD stage 2, 42 in CKD stage 3, 15 in CKD stage 4, and 192 in CKD stage 5 ().
LVDD was defined based on an update from the American Society of Echocardiography and the European Association of Cardiovascular Imaging [Citation24], as briefly in . ALVDD was defined as LVDD but without signs or symptoms of HF [Citation25].
Figure 2. Algorithm for diagnosis of LVDD in subjects with normal LVEF [Citation24].
![Figure 2. Algorithm for diagnosis of LVDD in subjects with normal LVEF [Citation24].](/cms/asset/f93ab148-4c6a-4869-84af-983c25b79b6e/irnf_a_2353334_f0002_b.jpg)
2.2. Research methods
Medical history data of recruited participants were collected. Body mass index (BMI) = weight (kg)/height2 (m2). Current smokers were those who continuously or cumulatively smoked for 6 months or more and were active smoking. Ex-smokers were defined as those who had smoked for at least one month in the past but had stopped smoking for 2 or more consecutive years before the study. Never-smokers were those who had never smoked before the study. Pack-years were calculated as the number of packs of cigarettes smoked per day multiplied by the number of years.
Bp was measured 3 times from the right arm in a sitting position using a standard mercury sphygmomanometer at the same time in the morning by trained and certified nurses and then averaged. Smoking, drinking alcohol, coffee/tea, and strenuous exercise were not allowed for participants at least 30 min before BP measurement. Systolic BP (SBP) and diastolic BP (DBP) were recorded at the first and fifth phases of the Korotkoff sounds. The mean arterial pressure (MAP) was calculated as (systolic blood pressure + 2 × diastolic blood pressure)/3.
Venous blood was drawn from all subjects after a fasting period of at least 8 h. Automatic biochemical analyzers in the clinical laboratory were used to measure total cholesterol (TC), triacylglycerol (TG), low-density lipoprotein cholesterol (LDL-C), high-density lipoprotein cholesterol (HDL-C), blood urea nitrogen (BUN), Scr, cystatin C (Cys-C), blood calcium and phosphorus, hemoglobin A1c (HbA1c), hemoglobin (Hb), lipoprotein(a) (Lp(a)), C-reactive protein (CRP), and erythrocyte sedimentation rate (ESR). Twenty-four-hour urine samples were collected, and 24-h urinary protein (PRO) and urine microalbumin (UMALB) were measured.
All echocardiography measurements were performed by trained, experienced sonographer using an Philips Epic 7 Color Doppler ultrasonic diagnostic apparatus (New Bedford, MA) (S5-1 heart probe, frequency range 2–5 MHz).
The left ventricular ejection fraction (LVEF) of the patient was measured for three consecutive cardiac cycles, and the mean value was taken to evaluate ventricular systolic function. The ratio (E/A) of early mitral valve blood flow velocity (E) and the atrial contraction mitral valve with the peak speed (A) and the ratio (E/e′) of early diastolic blood flow velocity of mitral valve and the early diastolic motion velocity of the lateral wall and septum of the mitral annulus (e′) were recorded to evaluate cardiac diastolic function. According to Stevenson standard formula, body surface area (BSA, m2) = 0.0061 × height (cm) + 0.0128 × weight (kg) − 0.1529. Left ventricular mass index (LVMI) = LVM/BSA. According to the 2018 ESH/ESC guidelines [Citation26], LVMI >115 g/m2 (male) or >95 g/m2 (female) or ventricular septum or left ventricular posterior wall thickness ≥10 mm is defined as left ventricular hypertrophy, and relative wall thickness ≥0.43 is defined as the relative increased thickness of the left ventricular wall.
Dummy variables were created for unordered categorical variables. Univariate logistic regression was used to calculate the odds ratio (OR) and 95% confidence interval (CI) between the LVDD and normal LVDD (nLVDD) groups. Several variables (p ≤ .2 in our univariate analysis or clinically believed or confirmed in previous studies to be associated with LVDD [Citation27]; there was no multicollinearity among these variables based on a correlation coefficient |r| >0.5 being considered indicative of collinearity [Citation28]) were included in the multivariate logistic regression analysis. The multivariate logistic regression model was constructed using backward logistic regression (included variables with p < .1 and excluded those with p > .05) [Citation27] to screen out the factors associated with risk for LVDD, and the ORs and 95% CIs were calculated. Blood calcium was divided into a normal blood calcium group (2.25–2.75 mmol/L, code: 0) and a hypocalcemia group (<2.25 mmol/L, code: 1). Blood phosphorus was divided into the hypophosphatemia group (<0.96 mmol/L, code 0), the normal blood phosphorus group (0.96–1.61 mmol/L, code 1), and the hyperphosphatemia group (>1.61 mmol/L, code 2).
2.3. Statistical analysis
SPSS statistical software package version 24.0 produced by International Business Machines Corporation (Armonk, NY) was used for data analysis. ALVDD in nondialysis CKD patients was recorded, and the proportion of ALVDD in nondialysis CKD stages 1–5 was calculated. LVDD was used as a dichotomous outcome variable (LVDD or nLVDD) for data analysis. Baseline data were compared between the LVDD and nLVDD groups.
Measurement data with a normal distribution were expressed as the mean ± standard deviation, and a t-test was performed to compare the means of the two groups. Measurement data with nonnormal distribution were presented as (median, quartile) (M,Q), and the Kruskal–Wallis rank sum test was used to compare medians between the groups. Categorical data were presented as numbers (n) of patients, and the Chi-square test was used to determine differences. The Kruskal–Wallis rank sum test was performed for intergroup comparisons of ranked data. All p values were two-sided, and p ≤ .05 was considered indicative of statistical significance.
GraphPad Prism 8.0 software produced by GraphPad Software (La Jolla, CA) was used to illustrate the figures. MedCalc 19.0 software produced by MedCalc Software Ltd. (Ostend, Belgium) was used to illustrate the receiver operating characteristic (ROC) curves to evaluate the prediction accuracy of the model [Citation29,Citation30].
3. Results
3.1. The proportion of ALVDD in nondialysis CKD patients
Among the 339 nondialysis CKD patients without signs or symptoms of HF, LVDD was present in 315 patients (92.04%) (89.47% in CKD stage 1, 90.91% in stage 2, 92.86% in stage 3, 93.33% in stage 4, and 94.27% in stage 5), and 24 patients (7.08%) had no diastolic dysfunction in echocardiography. The differences between CKD stages were statistically significant (p < 0.001). The proportion of ALVDD in nondialysis CKD patients was higher, and with the progression of CKD stage, the proportion of ALVDD gradually increased (). The proportion of ALVDD was 96.08% for disease 1, 92.16% for disease 2, 75.00% for disease 3, and 85.71% for disease 4, respectively. The proportion in subjects with disease 1 (secondary renal disease caused by metabolic diseases) was significantly higher than in those with disease 2, 3, and 4 (p < 0.01) ().
Annotations: disease 1, DN or HTN; 2, CGN or NS or IgAN; 3, CIN; 4, PKD or other causes of CKD.
3.2. The difference test and univariate logistic regression of baseline data between the LVDD group and the nLVDD group of CKD patients
The differences between the LVDD group and the nLVDD group of nondialysis CKD patients in age, subtype of primary kidney disease, blood phosphorus stratification, Lp(a), UMALB, E, A, e′, and E/A were statistically significant (p < 0.05). Increased age (OR = 1.144), hyperphosphatemia (OR = 2.220), DN and HTN (OR = 4.082), and increased Lp(a) (OR = 1.002) were factors associated with risk for ALVDD according to the OR values derived from univariate logistic regression ().
Table 1. Comparison of baseline data between LVDD and nLVDD in CKD patients.
3.3. Multicollinearity analysis of the independent variables
In the correlation coefficient matrix, the correlation coefficients between the eGFR and Scr, the eGFR and Cys-C, the eGFR and Hb, Cys-C and Scr, TC and LDL-C, serum total protein and serum albumin, serum total protein and urinary PRO, and serum albumin and urinary PRO were −0.682, −0.692, 0.597, 0.876, 0.923, 0.737, −0.515, and −0.599, respectively. To avoid multicollinearity, indicators of Scr, Cys-C, Hb, LDL-C, serum total protein, and serum albumin were excluded from the multivariate logistic regression model.
3.4. Multivariate logistic regression analysis and screening of factors associated with risk for ALVDD in nondialysis CKD patients
Several variables (p ≤ 0.2 in our univariate analysis or clinically believed or confirmed in previous studies to be associated with LVDD; there was no multicollinearity among these variables) were included in the multivariate logistic regression analysis. The results indicated that increased age (OR = 1.237, per year), DN and HTN (OR = 25.000, DN + HTN vs. CIN), CKD stage (OR = 2.785, per stage), increased MAP (OR = 1.154, per mmHg), urinary protein (OR = 2.825, per g/24 h), and low blood calcium (OR = 0.072, per mmol/L) were factors associated with risk for ALVDD in nondialysis CKD patients after adjusting for confounding factors (p < .05), and the ORs and 95% CIs are shown in .
Annotations: Blood calcium stratification 0, normal blood calcium; 1, hypocalcemia. Blood phosphorus stratification 0, hypophosphatemia; 1, normal blood phosphorus; 2, hyperphosphatemia. Disease 1, DN or HTN; 2, CGN or NS or IgAN; 3, CIN; 4, PKD or other causes of CKD.
3.5. Evaluation of the multivariate logistic regression fitting model
A p value of <.001 in the omnibus test indicated that the fitting model was statistically significant. The Hosmer and Lemeshow test (p = .09), Nagelkerke R2 coefficient (0.584) and likelihood ratio test: −2Log Likelihood value (55.029) indicated that the multivariate logistic model had an excellent goodness-of-fit. The prediction accuracy of the fitted model was as high as 96.90%.
3.6. The ROC curve of the probability value predicted by the fitted multivariate logistic regression model
The area under the ROC curve (AUC) was 0.995, and its 95% CI was 0.985–1.000 (Z statistic = 93.169, p < .0001). The sensitivity, specificity, and Youden’s index of the fitted model were 96.09%, 99.99%, and 0.9608, respectively ().
4. Discussion
Abnormalities in left ventricular structure and function are common and often precede the onset of clinical CVD in patients with CKD, including HF, especially HFpEF, which is the most prevalent HF subtype of CKD. Even without obvious clinical HF symptoms, LVDD has been shown to be a risk factor for cardiovascular and all-cause mortality in CKD [Citation31,Citation32]. Among the 339 nondialysis CKD patients we enrolled in our study, the proportion of ALVDD in CKD stage 1 was already high (89.47%). With the progression of the CKD stage, the proportion of ALVDD gradually increased, reaching as high as 94.27% in CKD stage 5, indicating that ALVDD was prevalent in CKD patients and may occur before left ventricular hypertrophy and systolic dysfunction. Therefore, exploring the factors associated with risk for ALVDD is of great clinical importance in reducing the morbidity and mortality of CVD complications in nondialysis CKD patients.
Our study indicated that the risk of ALVDD in the DN and HTN groups was significantly higher than that of CIN, PKD, and other CKD causes in the univariate analysis. DN and HTN were still independent factors associated with risk for ALVDD in nondialysis CKD patients after adjusting for the influence of confounding factors in multivariate logistic analysis. Meanwhile, we found that MAP was also a factor associated with risk for ALVDD in multivariate logistic analysis, which further confirmed the important pathophysiological mechanism of increased BP and its associated HTN in LVDD of CKD patients. Because cardiac structural abnormalities can also lead to impaired myocardial strain force, we also collected cardiac structural data and found that 315 nondialysis CKD patients with ALVDD had increased left ventricular wall thickness, accounting for up to 94.27% of the sample population. The increased thickness of the ventricular wall leads to decreased left ventricular compliance [Citation33], and the abnormal structure of the ventricular wall leads to changes in cardiac geometry [Citation34], which may also be one of the reasons for the high proportion of ALVDD in nondialysis CKD patients. One of the most common causes of increased ventricular wall thickness is hypertension [Citation35], and increased BP or its associated HTN can directly give rise to LVDD or lead to LVDD by causing structural abnormalities in the heart. Our results lend credence to the important concept that BP control is the cornerstone for the prevention and therapy of CVD complications in the management of CKD [Citation1]. HFpEF is common in diabetes and is mainly caused by LVDD [Citation36]. One possible explanation is that insulin resistance and/or hyperinsulinemia lead to endothelial dysfunction [Citation37]. Another possible explanation is that the activation of the renin–angiotensin–aldosterone system in diabetes leads to sodium and water retention and vascular tension changes, playing an important role in the pathogenesis of LVDD [Citation38], and the nonsteroidal mineralocorticoid receptor antagonist finerenone can alleviate LVDD [Citation39]. Special attention should be given to the monitoring of LVDD in CKD patients with DN and HTN.
We also found that increased 24-h urinary protein was an independent factor associated with risk for ALVDD in nondialysis CKD patients. It is well known that urinary protein is an indicator and a key determinant for renal dysfunction, and with an increase in the urinary PRO level, the degree of renal function damage also increases. These results further confirmed that the severity of kidney damage and the progression of CKD were closely associated with the onset, development, and progression of ALVDD in nondialysis CKD patients. Therefore, we should control urinary protein actively and monitor dynamic changes of urinary PRO levels closely in the management of nondialysis CKD, whether to assess the therapeutic effects or to monitor the occurrence and development of ALVDD.
It has been found that with the CKD stage progression, a decrease in eGFR [Citation1,Citation40] and calcium metabolism disorder [Citation41] are the main risk factors for LVDD in CKD patients. We also found that the progression of the CKD stage was an independent factor associated with risk for ALVDD in nondialysis CKD patients. It was reported that serum calcium levels in patients with LVDD were lower than those in patients without LVDD [Citation42,Citation43]. We also found that in the multivariate analysis, hypocalcemia was a factor associated with risk for ALVDD in nondialysis CKD patients after adjusting for other confounding factors, while in univariate analysis, hyperphosphatemia was a factor associated with risk, further confirming that even in the absence of obvious symptoms of HF, metabolic disorder of calcium and phosphorus is an important factor associated with risk for the development of ALVDD in the nondialysis CKD population. This may be because calcium ions are essential elements for the action potential and excitation–contraction coupling of cardiomyocytes. Physiologically, the diastolic and systolic activities of cardiomyocytes and the extension of their functional potentials are dependent on calcium flow [Citation44], especially the active relaxation of the heart, which requires adenosine triphosphate (ATP) to initiate the dissociation of calcium from troponin C and reuptake back into the sarcoplasmic reticulum [Citation45], causing the uncoupling of actin and myosin to allow myofibrils to return to their precontracted length [Citation46]. Hypocalcemia in CKD patients leads to delayed inactivation of myocardial excitation–contraction coupling [Citation47], disturbance of sarcoplasmic reticulum transfer of calcium ions [Citation48], increased affinity between calcium ions and troponin, and decreased inactivation of the cross-bridge-actin interaction. All of these mechanisms delay the process of calcium reuptake back to the sarcoplasmic reticulum [Citation46], resulting in LVDD.
Our study found that age was also an independent factor associated with risk for ALVDD in nondialysis CKD patients. It is known that aging is a risk factor for both HF and CKD, and the correlation between age and LVDD is even stronger than that of systolic dysfunction [Citation6]. Although the age-specific prevalence of HF is decreasing, but this trend is less dramatic for HFpEF than for HFrEF [Citation6]. With the aging of the world population, HFpEF may become a very important cause of death in CKD [Citation19]. Therefore, we should pay more attention to monitoring LVDD in elderly nondialysis CKD patients to find any ALVDD, which has been largely overlooked.
To validate the results and evaluate the accuracy of the multifactor model, we tested the fitted multivariate logistic regression model. We found that the overall fit of the model was meaningful and quite good. It is important to evaluate the prediction accuracy of the fitted model, which was as high as 96.90% in our study, suggesting that this model is applicable in the prediction of ALVDD in nondialysis CKD patients. Meanwhile, according to the ROC curve drawn from the prediction probability values of the model, the prediction ability and accuracy of the model are very high. All of these results indicate the satisfactory goodness-of-fit, robustness, and predictive ability of the predictive model to assess ALVDD in nondialysis CKD patients.
In conclusion, our study revealed a high proportion of LVDD in nondialysis CKD patients even without significant HF symptoms. Patients with secondary renal disease caused by metabolic diseases are at greater risk of ALVDD than those with other causes of CKD. Simple and accurate tools for identification of factors associated with risk for LVDD is important for early diagnosis of the high-risk asymptomatic HF population among nondialysis CKD patients. Intervention of these underlying factors associated with risk early before the onset of clinical HF symptoms would delay the progression to symptomatic HF. The identification of these factors associated with risk is of great importance for reducing CVD mortality and improving the quality of life and prognosis in CKD patients.
5. Limitations
The present study exhibits some limitations. Our single-center study was retrospective observational in nature and the sample size was relatively limited. Therefore, prospective studies with a larger sample should be conducted to further verify our findings.
6. Conclusions
The proportion of ALVDD is high in nondialysis CKD patients. Increased age, the causes of CKD (DN or HTN), CKD stage, increased MAP, proteinuria, and low blood calcium are independent factors associated with risk for ALVDD in nondialysis CKD patients.
Disclosure statement
No potential conflict of interest was reported by the author(s).
Additional information
Funding
References
- Matsushita K, Ballew SH, Wang AY, et al. Epidemiology and risk of cardiovascular disease in populations with chronic kidney disease. Nat Rev Nephrol. 2022;18(11):1–12. doi: 10.1038/s41581-022-00616-6.
- Taherkhani A, Farrokhi YR, Mohseni M, et al. Chronic kidney disease: a review of proteomic and metabolomic approaches to membranous glomerulonephritis, focal segmental glomerulosclerosis, and IgA nephropathy biomarkers. Proteome Sci. 2019;17(1):7. doi: 10.1186/s12953-019-0155-y.
- Chioncel O, Lainscak M, Seferovic PM, et al. Epidemiology and one-year outcomes in patients with chronic heart failure and preserved, mid-range and reduced ejection fraction: an analysis of the ESC Heart Failure Long-Term Registry. Eur J Heart Fail. 2017;19(12):1574–1585. doi: 10.1002/ejhf.813.
- Redfield MM. Heart failure with preserved ejection fraction. N Engl J Med. 2016;375(19):1868–1877. doi: 10.1056/NEJMcp1511175.
- Shiga T, Suzuki A, Haruta S, et al. Clinical characteristics of hospitalized heart failure patients with preserved, mid-range, and reduced ejection fractions in Japan. ESC Heart Fail. 2019;6(3):475–486. doi: 10.1002/ehf2.12418.
- Dunlay SM, Roger VL, Redfield MM. Epidemiology of heart failure with preserved ejection fraction. Nat Rev Cardiol. 2017;14(10):591–602. doi: 10.1038/nrcardio.2017.65.
- Reddy YN, Borlaug BA. Heart failure with preserved ejection fraction. Curr Probl Cardiol. 2016;41(4):145–188. doi: 10.1016/j.cpcardiol.2015.12.002.
- Yu AS, Pak KJ, Zhou H, et al. All-cause and cardiovascular-related mortality in CKD patients with and without heart failure: a population-based cohort study in Kaiser Permanente Southern California. Kidney Med. 2023;5(5):100624. doi: 10.1016/j.xkme.2023.100624.
- Tang M, Long Y, Liu S, et al. Prevalence of cardiovascular events and their risk factors in patients with chronic obstructive pulmonary disease and obstructive sleep apnea overlap syndrome. Front Cardiovasc Med. 2021;8:694806. doi: 10.3389/fcvm.2021.694806.
- Pieske B, Tschöpe C, de Boer RA, et al. How to diagnose heart failure with preserved ejection fraction: the HFA-PEFF diagnostic algorithm: a consensus recommendation from the Heart Failure Association (HFA) of the European Society of Cardiology (ESC). Eur J Heart Fail. 2020;22(3):391–412. doi: 10.1002/ejhf.1741.
- Kitzman DW, Little WC, Brubaker PH, et al. Pathophysiological characterization of isolated diastolic heart failure in comparison to systolic heart failure. JAMA. 2002;288(17):2144–2150. doi: 10.1001/jama.288.17.2144.
- Park M, Hsu CY, Li Y, et al. Associations between kidney function and subclinical cardiac abnormalities in CKD. J Am Soc Nephrol. 2012;23(10):1725–1734. doi: 10.1681/ASN.2012020145.
- Heidenreich PA, Bozkurt B, Aguilar D, et al. 2022 AHA/ACC/HFSA guideline for the management of heart failure: a report of the American College of Cardiology/American Heart Association Joint Committee on Clinical Practice Guidelines. Circulation. 2022;145(18):e895–e1032. doi: 10.1161/CIR.0000000000001063.
- Kitai T, Miyakoshi C, Morimoto T, et al. Mode of death among Japanese adults with heart failure with preserved, midrange, and reduced ejection fraction. JAMA Netw Open. 2020;3(5):e204296. doi: 10.1001/jamanetworkopen.2020.4296.
- Kapłon-Cieślicka A, Benson L, Chioncel O, et al. A comprehensive characterization of acute heart failure with preserved versus mildly reduced versus reduced ejection fraction – insights from the ESC-HFA EORP Heart Failure Long-Term Registry. Eur J Heart Fail. 2022;24(2):335–350. doi: 10.1002/ejhf.2408.
- Boxuan PU, Guangda HE, Runqing LI, et al. Differences and trends in health-related quality of life in hospitalized patients for heart failure with preserved, mildly reduced and reduced left ventricular ejection fraction. Chin Circ J. 2023;38(06):656–662.
- Bozkurt B, Coats AJ, Tsutsui H, et al. Universal definition and classification of heart failure: a report of the Heart Failure Society of America, Heart Failure Association of the European Society of Cardiology, Japanese Heart Failure Society and Writing Committee of the Universal Definition of Heart Failure. J Card Fail. 2021. doi: 10.1016/j.cardfail.2021.01.022.
- Echouffo-Tcheugui JB, Erqou S, Butler J, et al. Assessing the risk of progression from asymptomatic left ventricular dysfunction to overt heart failure: a systematic overview and meta-analysis. JACC Heart Fail. 2016;4(4):237–248. doi: 10.1016/j.jchf.2015.09.015.
- Zhang N, Feng B, Ma X, et al. Dapagliflozin improves left ventricular remodeling and aorta sympathetic tone in a pig model of heart failure with preserved ejection fraction. Cardiovasc Diabetol. 2019;18(1):107. doi: 10.1186/s12933-019-0914-1.
- Kang E, Lee SW, Ryu H, et al. Left ventricular diastolic dysfunction and progression of chronic kidney disease: analysis of KNOW-CKD data. J Am Heart Assoc. 2022;11(13):e25554. doi: 10.1161/JAHA.122.025554.
- Stevens PE, Levin A, Kidney Disease: Improving Global Outcomes Chronic Kidney Disease Guideline Development Work Group Members. Evaluation and management of chronic kidney disease: synopsis of the kidney disease: improving global outcomes 2012 clinical practice guideline. Ann Intern Med. 2013;158(11):825–830. doi: 10.7326/0003-4819-158-11-201306040-00007.
- Levey AS, Stevens LA, Schmid CH, et al. A new equation to estimate glomerular filtration rate. Ann Intern Med. 2009;150(9):604–612. doi: 10.7326/0003-4819-150-9-200905050-00006.
- Ma YC, Zuo L, Chen JH, et al. Improved GFR estimation by combined creatinine and cystatin C measurements. Kidney Int. 2007;72(12):1535–1542. doi: 10.1038/sj.ki.5002566.
- Nagueh SF, Smiseth OA, Appleton CP, et al. Recommendations for the evaluation of left ventricular diastolic function by echocardiography: an update from the American Society of Echocardiography and the European Association of Cardiovascular Imaging. Eur Heart J Cardiovasc Imaging. 2016;17(12):1321–1360. doi: 10.1093/ehjci/jew082.
- Hunt SA, Abraham WT, Chin MH, et al. ACC/AHA 2005 guideline update for the diagnosis and management of chronic heart failure in the adult: a report of the American College of Cardiology/American Heart Association Task Force on Practice Guidelines (Writing Committee to update the 2001 guidelines for the evaluation and management of heart failure): developed in collaboration with the American College of Chest Physicians and the International Society for Heart and Lung Transplantation: endorsed by the Heart Rhythm Society. Circulation. 2005;112(12):e154–e235. doi: 10.1161/CIRCULATIONAHA.105.167586.
- Williams B, Mancia G, Spiering W, et al. 2018 ESC/ESH guidelines for the management of arterial hypertension. Eur Heart J. 2018;39(33):3021–3104. doi: 10.1093/eurheartj/ehy339.
- Bi Y, Wang L, Xu Y, et al. Diabetes-related metabolic risk factors in internal migrant workers in China: a national surveillance study. Lancet Diabetes Endocrinol. 2016;4(2):125–135. doi: 10.1016/S2213-8587(15)00366-6.
- Vatcheva KP, Lee M, McCormick JB, et al. Multicollinearity in regression analyses conducted in epidemiologic studies. Epidemiology. 2016;6(2):227. doi: 10.4172/2161-1165.1000227.
- Stoltzfus JC. Logistic regression: a brief primer. Acad Emerg Med. 2011;18(10):1099–1104. doi: 10.1111/j.1553-2712.2011.01185.x.
- Meurer WJ, Tolles J. Logistic regression diagnostics: understanding how well a model predicts outcomes. JAMA. 2017;317(10):1068–1069. doi: 10.1001/jama.2016.20441.
- Bansal N, Roy J, Chen HY, et al. Evolution of echocardiographic measures of cardiac disease from CKD to ESRD and risk of all-cause mortality: findings from the CRIC study. Am J Kidney Dis. 2018;72(3):390–399. doi: 10.1053/j.ajkd.2018.02.363.
- Liang HY, Hsiao YL, Yeh HC, et al. Associations between myocardial diastolic dysfunction and cardiovascular mortality in chronic kidney disease: a large single-center cohort study. J Am Soc Echocardiogr. 2022;35(4):395–407. doi: 10.1016/j.echo.2021.12.003.
- Antlanger M, Aschauer S, Kopecky C, et al. Heart failure with preserved and reduced ejection fraction in hemodialysis patients: prevalence, disease prediction and prognosis. Kidney Blood Press Res. 2017;42(1):165–176. doi: 10.1159/000473868.
- Winterberg PD, Robertson JM, Kelleman MS, et al. T cells play a causal role in diastolic dysfunction during uremic cardiomyopathy. J Am Soc Nephrol. 2019;30(3):407–420. doi: 10.1681/ASN.2017101138.
- Li X, Zhu S, Song G, et al. Retinol-binding protein 4 is closely correlated to blood pressure level and E/A in untreated essential hypertension patients. Ann Palliat Med. 2019;8(5):645–650. doi: 10.21037/apm.2019.11.07.
- Lawson CA, Tay WT, Bernhardt L, et al. Association between diabetes, chronic kidney disease, and outcomes in people with heart failure from Asia. JACC Asia. 2023;3(4):611–621. doi: 10.1016/j.jacasi.2023.03.005.
- Wang M, Li Y, Li S, et al. Endothelial dysfunction and diabetic cardiomyopathy. Front Endocrinol. 2022;13:851941. doi: 10.3389/fendo.2022.851941.
- Jia G, Jia Y, Sowers JR. Role of mineralocorticoid receptor activation in cardiac diastolic dysfunction. Biochim Biophys Acta Mol Basis Dis. 2017;1863(8):2012–2018. doi: 10.1016/j.bbadis.2016.10.025.
- Lima-Posada I, Stephan Y, Soulié M, et al. Benefits of the non-steroidal mineralocorticoid receptor antagonist finerenone in metabolic syndrome-related heart failure with preserved ejection fraction. Int J Mol Sci. 2023;24(3):2536. doi: 10.3390/ijms24032536.
- Ter Maaten JM, Damman K, Verhaar MC, et al. Connecting heart failure with preserved ejection fraction and renal dysfunction: the role of endothelial dysfunction and inflammation. Eur J Heart Fail. 2016;18(6):588–598. doi: 10.1002/ejhf.497.
- Nardi E, Mulè G, Nardi C, et al. Is echocardiography mandatory for patients with chronic kidney disease? Intern Emerg Med. 2019;14(6):923–929. doi: 10.1007/s11739-019-02028-0.
- Gromadziński L, Januszko-Giergielewicz B, Pruszczyk P. Hypocalcemia is related to left ventricular diastolic dysfunction in patients with chronic kidney disease. J Cardiol. 2014;63(3):198–204. doi: 10.1016/j.jjcc.2013.08.003.
- Ramadan SM, Hadeel AM, Nashwa A, et al. Left ventricular mass and functions in Egyptian children with chronic kidney disease in comparison to normal subjects. Saudi J Kidney Dis Transpl. 2022;33(2):296–306. doi: 10.4103/1319-2442.379028.
- Ayaz O, Banga S, Heinze-Milne S, et al. Long-term testosterone deficiency modifies myofilament and calcium-handling proteins and promotes diastolic dysfunction in the aging mouse heart. Am J Physiol Heart Circ Physiol. 2019;316(4):H768–H780. doi: 10.1152/ajpheart.00471.2018.
- Pabel S, Wagner S, Bollenberg H, et al. Empagliflozin directly improves diastolic function in human heart failure. Eur J Heart Fail. 2018;20(12):1690–1700. doi: 10.1002/ejhf.1328.
- Bers DM. Cardiac sarcoplasmic reticulum calcium leak: basis and roles in cardiac dysfunction. Annu Rev Physiol. 2014;76(1):107–127. doi: 10.1146/annurev-physiol-020911-153308.
- Zhang L, Nan C, Chen Y, et al. Calcium desensitizer catechin reverses diastolic dysfunction in mice with restrictive cardiomyopathy. Arch Biochem Biophys. 2015;573:69–76. doi: 10.1016/j.abb.2015.03.015.
- Mcdonald KS, Emter CA. Exploring new concepts in the management of heart failure with preserved ejection fraction: is exercise the key for improving treatment? J Appl Physiol (1985). 2015;119(6):724–725. doi: 10.1152/japplphysiol.00570.2015.