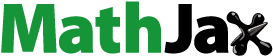
Abstract
Numerous studies have revealed a correlation between the risk of developing diabetic nephropathy (DN) and the gut microbiota (GM) composition. However, it remains uncertain whether the GM composition causes DN. We aimed to explore any potential causal links between the GM composition and the risk of developing DN. A meta-analysis conducted by the MiBioGen consortium of the largest genome-wide association study (GWAS) provided aggregated data on the GM. DN data were obtained from the IEU database. The inverse-variance weighting (IVW) method was employed as the primary analytical approach. The IVW analysis indicated that genus Dialister (OR = 0.51, 95% CI: 0.34–0.77, p = 0.00118) was protective against DN. In addition, class Gammaproteobacteria (OR = 0.47, 95% CI: 0.27–0.83, p = 0.0096), class Lentisphaeria (OR =0.76, 95% CI: 0.68–0.99, p = 0.04), order Victivallales (OR = 0.76, 95% CI: 0.58–0.99, p = 0.04), and phylum Proteobacteria (OR = 0.53, 95% CI: 0.33–0.85, p = 0.00872) were negatively associated with the risk of developing DN. Genus LachnospiraceaeUCG008 (OR =1.45, 95% CI: 1.08–1.95, p = 0.01), order Bacteroidales (OR = 1.59, 95% CI: 1.02–2.49, p = 0.04), and genus Terrisporobacter (OR = 1.98, 95% CI: 1.14–3.45, p = 0.015) were positively associated with the risk of developing DN. In this study, we established a causal relationship between the genus Dialister and the risk of developing DN. Further trials are required to confirm the protective effects of probiotics on DN and to elucidate the precise protective mechanisms involving genus Dialister and DN.
1. Background
One of the global causes of end-stage renal disease is DN, a microvascular complication of diabetes [Citation1–3]. Statistics indicate that 30%–40% of diabetic patients suffer from nephropathy, and the prevalence of DN constitutes one-third of the total number of chronic kidney disease (CKD) cases [Citation4]. Consequently, DN presents a significant health challenge for society. From a clinical perspective, proteinuria and a decline in renal function are the primary hallmarks of DN [Citation5,Citation6]. Furthermore, DN status is associated with a substantially increased risk of developing cardiovascular disease [Citation7]. Current treatment strategies for DN primarily focus on inhibiting the renin–angiotensin axis; however, the therapeutic effectiveness of these treatments remains inadequate [Citation8].
Numerous investigations have confirmed the altered composition of the GM in diabetes patients and the concurrent disruption of the intestinal barrier, underscoring the significant role that the GM composition plays in human metabolism [Citation9–11]. DN, a prevalent complication of diabetes mellitus, has also been extensively linked to the GM composition. Luo reported that an increase in the abundances of the genus Akkermansia and Candidatus Saccharimonas ameliorated glomerular injury and renal fibrosis [Citation12]. Li reported that dietary fiber supplementation can prevent DN by modulating the GM composition [Citation13]. Nevertheless, studies have also shown that GM depletion with antibiotics improves glomerular damage and insulin resistance [Citation14]. Therefore, to successfully ameliorate DN, it is imperative to elucidate the causal relationship between the GM composition and the risk of developing DN.
MR, which involves the use of genetic variation as an IV to examine causal associations between exposure and outcome [Citation15], is now widely accessible, particularly in the realms of metabolic [Citation16] and autoimmune [Citation17] diseases. The benefit lies in the fact that the causal relationship between genetic variation and prognosis is not influenced by confounding factors [Citation18]. In this study, we performed a two-sample MR analysis using GWAS summary statistics to investigate the causal connection between the GM composition and the risk of developing DN.
2. Methods
2.1. Data sources
The research concept is depicted in . In this investigation, human GM data were acquired from a genome-wide meta-analysis conducted by the MiBioGen consortium, encompassing 18,340 subjects from 24 cohorts [Citation19,Citation20]. The V4, V3-V4, and V1-V2 regions of 16S rRNA were the focus of this study. We identified genetic variants associated with the abundance of bacterial taxa through microbiota quantitative trait loci (mbQTL) mapping. A total of 211 bacterial traits were identified, and after removing 15 unknown bacterial traits, 196 were screened, including 9 phyla, 16 classes, 20 orders, 32 families and 119 genera. DN GWAS data, comprising data from 1,032 patients and 451,248 control participants, were obtained from the IEU, which was published in 2022 (PMID: 34594039) (https://gwas.mrcieu.ac.uk/datasets/ebi-a-GCST90018832/) [Citation21].
2.2. Selection of instrumental variables (IVs)
Initially, the genome-wide significance threshold for single nucleotide polymorphisms (SNPs) was set at 1.0 × 10−5, thereby excluding SNPs with P ≥ 1.0 × 10−5. To assess the linkage disequilibrium (LD) between SNPs, the European population of the Thousand Genomes Project was utilized as a reference panel, with R2 < 0.001 (clumping window size =10,000 kb). We gauged the strength of the IVs by calculating the F statistic, expressed as ,
, with N representing the number of participants, EAF denoting the effect allele frequency, and β indicating the estimated effect of the SNPs to assess their ability to uniquely predict the outcome [Citation22–24].
2.3. Statistical analysis
In this MR study, we utilized MR–Egger regression, weighted median, and IVW methods to explore the causal relationship between the GM composition and the risk of developing DN. The specifics of these methods have been extensively described in other publications [Citation25–28]. IVW is the most crucial and commonly used methodology. IVW is used to estimate the average effect of genetic variants on causality by means of a weighted linear regression model, and the results of IVW are unbiased if there is no horizontal pleiotropy [Citation26,Citation29]. Cochrane’s Q test was then employed to evaluate the heterogeneity of the results [Citation30], and if heterogeneity was present (p < 0.05), then the IVW results would be more reliable with the use of a random-effects model. MR-PRESSO analysis was used to identify outliers, which need to be removed if present, otherwise horizontal pleiotropy may be present [Citation31]. In addition, we conducted a horizontal pleiotropy analysis, and MR–Egger regression analysis suggested that if horizontal pleiotropy was present (p < 0.05), the assumption of MR analysis was violated. Finally, a sensitivity analysis was conducted. Leave-one-out analysis, a popular technique for sensitivity analysis, involves evaluating the reliability of the remaining SNPs by progressively excluding each SNP.
R version 4.3.2 was utilized to conduct all the statistical analyses. Two sample MR and MR- PRESSO packages were used.
3. Results
3.1. Causal association between the GM composition and the risk of developing DN
A total of 2645 SNPs were selected as IVs for 196 bacterial traits. In this analysis, we used IVW as the primary method. We discovered that eight bacterial traits exhibited a causal effect on the risk of developing DN (): class Gammaproteobacteria (OR = 0.47, 95% CI: 0.27–0.83, p = 0.0096), class Lentisphaeria (OR = 0.76, 95% CI: 0.68–0.99, p = 0.04), genus Dialister (OR = 0.51, 95% CI: 0.34–0.77, p = 0.00118), order Victivallales (OR = 0.76, 95% CI: 0.58–0.99, p = 0.04), and phylum Proteobacteria (OR = 0.53, 95% CI: 0.33–0.85, p = 0.00872). These bacterial traits exhibited positive effects, implying that they may be protective against the risk of developing DN. Genus LachnospiraceaeUCG008 (OR = 1.45, 95% CI: 1.08–1.95, p = 0.01) and order Bacteroidales (OR = 1.59, 95% CI: 1.02–2.49, p = 0.04) had a negative effect on the risk of developing DN. In addition, the weighted median (WM) method indicated that genus Terrisporobacter (OR =1.98, 95% CI: 1.14–3.45, p = 0.015) increased the risk of developing DN, but the WM analysis did not demonstrate a relationship between several other bacteria and the risk of developing DN (details are provided in the Supplementary Tables S1 and S2). Unfortunately, none of the aforementioned causal effects remained significant after correction for the false discovery rate (FDR) (p > 0.05).
3.2. Sensitivity analysis
The SNPs used for MR analysis all had F-statistics greater than 10, eliminating the biasing effect of weak IVs. For the leave-one-out approach, the reliability of the remaining SNPs was assessed by systematically removing one SNP at a time. The results clearly indicated that upon the removal of any SNP, the effects of the other SNPs remained substantially unchanged (). Cochrane’s Q test indicated the absence of heterogeneity (), while MR–Egger regression analysis revealed no evidence of horizontal pleiotropy (). Furthermore, MR-PRESSO did not detect any outliers. Consequently, based on the results of the aforementioned analyses, the IVW results were deemed plausible in the absence of heterogeneity and horizontal pleiotropy. Genus Dialister, class Gammaproteobacteria, class Lentisphaeria, order Victivallales, phylum Proteobacteria, genus LachnospiraceaeUCG008, order Bacteroidales, and genus Terrisporobacter abundances were associated with the risk of developing DN.
Table 1. The heterogeneity of gut microbiota instrumental variables.
Table 2. Directional horizontal pleiotropy assessed by intercept term in MR Egger regression of the association between gut microbiota and DN.
4. Discussion
The GM summary data from the GWAS meta-analysis of the MiBioGen consortium and the DN summary statistics from the IEU were utilized in two-sample MR analysis. Our study identified several microbial taxa with positive effects on the risk of developing DN, especially the genus Dialister. Class Gammaproteobacteria, class Lentisphaeria, order Victivallales and phylum Proteobacteria may represent potentially helpful probiotics, while genus LachnospiraceaeUCG008, order Bacteroidales and genus Terrisporobacter were associated with a greater risk of developing DN.
To date, numerous studies have documented the association between the risk of developing DN and the GM composition [Citation9,Citation13,Citation32,Citation33]. Although no research has established a causal link between the risk of developing DN and genus Dialister, several studies have suggested that the genus Dialister may be beneficial for patients with chronic kidney disease [Citation34,Citation35], indicating that we are moving in the right direction. Probiotics such as genus Dialister have been shown to have preventive effects against neurological and digestive disorders [Citation36,Citation37]. Class Victivallales and order Lentisphaeria have received relatively little research attention. Nonetheless, current research indicates that they are closely related to immunomodulation. For example, individuals with rosacea have lower levels of order Lentisphaerae [Citation38]. Additionally, there was a negative correlation between the severity of symptoms and order Lentisphaerae in patients with posttraumatic stress disorder [Citation39]. Interestingly, in a randomized clinical trial, patients with type I diabetes who received placebos exhibited significantly greater abundances of genus Terrisporobacter than patients receiving probiotics did [Citation40]. A MR analysis also revealed that the abundance of order Bacteroidales was positively associated with the incidence of diabetes (OR = 1.24, 95% CI = 1.01–1.53, p = 0.044), which was consistent with our findings [Citation41].
Short-chain fatty acids (SCFAs), which primarily includes butyric, propionic, and acetic acids, are one of the end products of GM metabolism. We identified several microorganisms that produce SCFAs. Li found that SCFAs inhibited the proliferation of glomerular thylakoid cells induced by high glucose or lipopolysaccharide (LPS) and reduced the generation of reactive oxygen species and the expression of inflammatory factors [Citation13]. Additionally, SCFAs enhance glucose tolerance in mice through the AMPK, PPY, and GLP-1 pathways [Citation42–44]. Furthermore, SCFAs significantly impact insulin resistance and obesity [Citation45]. In Wong’s study, individuals with CKD had increased serum levels of urease, p-cresol, and other chemicals as butyric acid decreased [Citation46]. However, there is disagreement regarding the role of acetic acid in CKD. In a rat model of diabetic kidney disease, acetic acid was found to upregulate the expression of G protein-coupled receptor 43 (GPR43), leading to the accumulation of cholesterol in renal epithelial cells and ultimately to renal damage [Citation47]. Nevertheless, other researchers have proposed that the GPR109A and GPR43-mediated benefits of acetic acid might be observed in the kidney [Citation48].
Recent advancements in the study of DN have indicated that harmful bacterial metabolites are among the factors contributing to and exacerbating DN, in addition to hyperglycemia and chronic inflammation. The secondary metabolite phenol is created when Tyrosine Phenol-lyase (TPL) enzymatically cleaves tyrosine. Phenyl sulfate is subsequently obtained by sulfurating phenol [Citation49], which causes podocyte damage and proteinuria. Some high-risk bacteria that we found may be responsible for the development of DN by causing long-term inflammation and producing toxic compounds such as phenyl sulfate. However, additional clinical studies are required to validate this hypothesis.
The strengths of this study are as follows: For the first time, we utilized two-sample MR to investigate the causal relationship between the GM composition and the risk of developing DN in European populations using the largest GWAS GM summary statistics from the MiBioGen project. We carefully selected independent SNPs and conducted a series of sensitivity analyses to mitigate the influence of confounders on causal effects. Additionally, SNPs with F-statistics < 10 were excluded to minimize bias from weak IVs. In contrast to other MR studies [Citation50], our research incorporated multiple levels of the gut microbiota, including phylum, class, order, family, and genus, leading to more robust and compelling findings.
However, our study has several limitations. First, it is uncertain whether the results of our research, which were obtained from European populations, are applicable to other ethnicities, thus necessitating future MR studies to confirm the reliability of the findings, particularly in non-European populations, to determine the causal effect between the GM composition and the risk of developing DN. Second, to conduct sensitivity and horizontal pleiotropy analyses, more SNPs needed to be included as IVs, so instead of the traditional significance threshold (P < 1 × 10−8), we chose 1.0 × 10−5. Third, in this study, we used statistical data rather than raw data, which resulted in our inability to accurately localize to DN subtypes. Hence, additional investigations are required when data on subtypes become available. Fourth, although we chose five levels of bacterial traits for the exposure data, the sample size of GM was still relatively small, and a larger range of GM could be included in future studies.
5. Conclusion
We concluded that there is a causal link between the abundance of genus Dialister and the risk of developing DN. However, further clinical trials are necessary to validate this finding, as the results of MR analysis alone are not conclusive.
Authors’ contributions
W.D, Y.H, L.J contributed to the research design, statistical analysis, Q.L, L.C, H.T, K.T, L.L contributed to the drafting and revision of the article. The final manuscript has been read and approved by all the listed authors.
Abbreviations | ||
CI | = | Confidence interval |
DN | = | diabetic nephropathy |
FDR | = | False discovery rate |
GM | = | gut microbiota |
GWAS | = | Genome-wide association study |
IV | = | Instrumental variable |
IVW | = | Inverse variance weighted |
LD | = | Linkage disequilibrium |
MAF | = | Minor allele frequency |
mbQTL | = | Microbiota quantitative trait loci |
MR | = | Mendelian randomization |
OR | = | Odds ratio |
SNP | = | Single nucleotide polymorphism |
TPL | = | Tyrosine Phenol-lyase |
SCFA | = | short-chain fatty acids |
LPS | = | Lipopolysaccharide. |
Supplemental Material
Download MS Excel (23 KB)Acknowledgements
We thank those who provided public data and those who acted in this study.
Disclosure statement
No potential conflict of interest was reported by the author(s).
Data availability statement
The source of the GM data is the MiBioGen consortium (https://mibiogen.gcc.rug.nl/) and the source of the diabetic nephropathy data is the IEU public database (https://gwas.mrcieu.ac.uk/).
Additional information
Funding
References
- Umanath K, Lewis JB. Update on diabetic nephropathy: core curriculum 2018. Am J Kidney Dis. 2018;71(6):884–895. doi:10.1053/j.ajkd.2017.10.026.
- Valencia WM, Florez H. How to prevent the microvascular complications of type 2 diabetes beyond glucose control. BMJ. 2017;356:i6505. doi:10.1136/bmj.i6505.
- Li PK, Ma TK. Global impact of nephropathies. Nephrology (Carlton). 2017;22(Suppl 4):9–13. doi:10.1111/nep.13146.
- Deng Y, Li N, Wu Y, et al. Global, regional, and national burden of diabetes-related chronic kidney disease from 1990 to 2019. Front Endocrinol (Lausanne). 2021;12:672350. doi:10.3389/fendo.2021.672350.
- Chiarelli F, Trotta D, Verrotti A, et al. Kidney involvement and disease in patients with diabetes. Panminerva Med. 2003;45(1):23–41.
- Sun YM, Su Y, Li J, et al. Recent advances in understanding the biochemical and molecular mechanism of diabetic nephropathy. Biochem Biophys Res Commun. 2013;433(4):359–361. doi:10.1016/j.bbrc.2013.02.120.
- Tervaert TWC, Mooyaart AL, Amann K, et al. Pathologic classification of diabetic nephropathy. J Am Soc Nephrol. 2010;21(4):556–563. doi:10.1681/asn.2010010010.
- Hu Q, Jiang L, Yan Q, et al. A natural products solution to diabetic nephropathy therapy. Pharmacol Ther. 2023;241:108314. doi:10.1016/j.pharmthera.2022.108314.
- Iatcu CO, Steen A, Covasa M. Gut microbiota and complications of type-2 diabetes. Nutrients. 2021;14(1):166. doi:10.3390/nu14010166.
- Yang G, Wei J, Liu P, et al. Role of the gut microbiota in type 2 diabetes and related diseases. Metabolism. 2021;117:154712. doi:10.1016/j.metabol.2021.154712.
- Zhang L, Wang Z, Zhang X, et al. Alterations of the gut microbiota in patients with diabetic nephropathy. Microbiol Spectr. 2022;10(4):e0032422. doi:10.1128/spectrum.00324-22.
- Luo L, Luo J, Cai Y, et al. Inulin-type fructans change the gut microbiota and prevent the development of diabetic nephropathy. Pharmacol Res. 2022;183:106367. doi:10.1016/j.phrs.2022.106367.
- Li YJ, Chen X, Kwan TK, et al. Dietary fiber protects against diabetic nephropathy through short-chain fatty acid-mediated activation of G protein-coupled receptors GPR43 and GPR109A. J Am Soc Nephrol. 2020;31(6):1267–1281. doi:10.1681/asn.2019101029.
- Lu J, Chen PP, Zhang JX, et al. GPR43 deficiency protects against podocyte insulin resistance in diabetic nephropathy through the restoration of AMPKα activity. Theranostics. 2021;11(10):4728–4742. doi:10.7150/thno.56598.
- Emdin CA, Khera AV, Kathiresan S. Mendelian randomization. JAMA. 2017;318(19):1925–1926. doi:10.1001/jama.2017.17219.
- Sanna S, van Zuydam NR, Mahajan A, et al. Causal relationships among the gut microbiome, short-chain fatty acids and metabolic diseases. Nat Genet. 2019;51(4):600–605. doi:10.1038/s41588-019-0350-x.
- Xu Q, Ni J-J, Han B-X, et al. Causal relationship between gut microbiota and autoimmune diseases: a two-sample mendelian randomization study. Front Immunol. 2021;12:746998. doi:10.3389/fimmu.2021.746998.
- Sekula P, Del Greco MF, Pattaro C, et al. Mendelian randomization as an approach to assess causality using observational data. J Am Soc Nephrol. 2016;27(11):3253–3265. doi:10.1681/asn.2016010098.
- MiBioGen consortium. https://mibiogen.gcc.rug.nl/.
- Kurilshikov A, Medina-Gomez C, Bacigalupe R, et al. Large-scale association analyses identify host factors influencing human gut microbiome composition. Nat Genet. 2021;53(2):156–165. doi:10.1038/s41588-020-00763-1.
- Sakaue S, Kanai M, Tanigawa Y, et al. A cross-population atlas of genetic associations for 220 human phenotypes. Nat Genet. 2021;53(10):1415–1424. doi:10.1038/s41588-021-00931-x.
- Palmer TM, Lawlor DA, Harbord RM, et al. Using multiple genetic variants as instrumental variables for modifiable risk factors. Stat Methods Med Res. 2012;21(3):223–242. doi:10.1177/0962280210394459.
- Levin MG, Judy R, Gill D, et al. Genetics of height and risk of atrial fibrillation: a Mendelian randomization study. PLoS Med. 2020;17(10):e1003288. doi:10.1371/journal.pmed.1003288.
- Gill D, Efstathiadou A, Cawood K, et al. Education protects against coronary heart disease and stroke independently of cognitive function: evidence from Mendelian randomization. Int J Epidemiol. 2019;48(5):1468–1477. doi:10.1093/ije/dyz200.
- Bowden J, Davey Smith G, Burgess S. Mendelian randomization with invalid instruments: effect estimation and bias detection through Egger regression. Int J Epidemiol. 2015;44(2):512–525. doi:10.1093/ije/dyv080.
- Burgess S, Scott RA, Timpson NJ, et al. Using published data in Mendelian randomization: a blueprint for efficient identification of causal risk factors. Eur J Epidemiol. 2015;30(7):543–552. doi:10.1007/s10654-015-0011-z.
- Bowden J, Davey Smith G, Haycock PC, et al. Consistent estimation in mendelian randomization with some invalid instruments using a weighted median estimator. Genet Epidemiol. 2016;40(4):304–314. doi:10.1002/gepi.21965.
- Hartwig FP, Davey Smith G, Bowden J. Robust inference in summary data Mendelian randomization via the zero modal pleiotropy assumption. Int J Epidemiol. 2017;46(6):1985–1998. doi:10.1093/ije/dyx102.
- Burgess S, Dudbridge F, Thompson SG. Combining information on multiple instrumental variables in Mendelian randomization: comparison of allele score and summarized data methods. Stat Med. 2016;35(11):1880–1906. doi:10.1002/sim.6835.
- Burgess S, Butterworth A, Thompson SG. Mendelian randomization analysis with multiple genetic variants using summarized data. Genet Epidemiol. 2013;37(7):658–665. doi:10.1002/gepi.21758.
- Verbanck M, Chen CY, Neale B, et al. Detection of widespread horizontal pleiotropy in causal relationships inferred from Mendelian randomization between complex traits and diseases. Nat Genet. 2018;50(5):693–698. doi:10.1038/s41588-018-0099-7.
- Deng L, Yang Y, Xu G. Empagliflozin ameliorates type 2 diabetes mellitus-related diabetic nephropathy via altering the gut microbiota. Biochim Biophys Acta Mol Cell Biol Lipids. 2022;1867(12):159234. doi:10.1016/j.bbalip.2022.159234.
- Kim JE, Nam H, Park JI, et al. Gut microbial genes and metabolism for methionine and branched-chain amino acids in diabetic nephropathy. Microbiol Spectr. 2023;11(2):e0234422. doi:10.1128/spectrum.02344-22.
- Wu I-W, Lin C-Y, Chang L-C, et al. Gut microbiota as diagnostic tools for mirroring disease progression and circulating nephrotoxin levels in chronic kidney disease: discovery and validation study. Int J Biol Sci. 2020;16(3):420–434. doi:10.7150/ijbs.37421.
- He J-W, Zhou X-J, Li Y-F, et al. Associations of genetic variants contributing to gut microbiota composition in immunoglobin a nephropathy. mSystems. 2021;6(1):e00819-20 doi:10.1128/mSystems.00819-20.
- Valles-Colomer M, Falony G, Darzi Y, et al. The neuroactive potential of the human gut microbiota in quality of life and depression. Nat Microbiol. 2019;4(4):623–632. doi:10.1038/s41564-018-0337-x.
- Joossens M, Huys G, Cnockaert M, et al. Dysbiosis of the faecal microbiota in patients with Crohn’s disease and their unaffected relatives. Gut. 2011;60(5):631–637. doi:10.1136/gut.2010.223263.
- Chen YJ, Lee WH, Ho HJ, et al. An altered fecal microbial profiling in rosacea patients compared to matched controls. J Formos Med Assoc. 2021;120(1 Pt 1):256–264. doi:10.1016/j.jfma.2020.04.034.
- Hemmings SMJ, Malan-Müller S, van den Heuvel LL, et al. The microbiome in posttraumatic stress disorder and trauma-exposed controls: an exploratory study. Psychosom Med. 2017;79(8):936–946. doi:10.1097/psy.0000000000000512.
- Ho J, Nicolucci AC, Virtanen H, et al. Effect of prebiotic on microbiota, intestinal permeability, and glycemic control in children with type 1 diabetes. J Clin Endocrinol Metab. 2019;104(10):4427–4440. doi:10.1210/jc.2019-00481.
- Luo M, Sun M, Wang T, et al. Gut microbiota and type 1 diabetes: a two-sample bidirectional Mendelian randomization study. Front Cell Infect Microbiol. 2023;13:1163898. doi:10.3389/fcimb.2023.1163898.
- Sakakibara S, Yamauchi T, Oshima Y, et al. Acetic acid activates hepatic AMPK and reduces hyperglycemia in diabetic KK-A(y) mice. Biochem Biophys Res Commun. 2006;344(2):597–604. doi:10.1016/j.bbrc.2006.03.176.
- Zhou J, Martin RJ, Tulley RT, et al. Dietary resistant starch upregulates total GLP-1 and PYY in a sustained day-long manner through fermentation in rodents. Am J Physiol Endocrinol Metab. 2008;295(5):E1160–1166. doi:10.1152/ajpendo.90637.2008.
- Tolhurst G, Heffron H, Lam YS, et al. Short-chain fatty acids stimulate glucagon-like peptide-1 secretion via the G-protein-coupled receptor FFAR2. Diabetes. 2012;61(2):364–371. doi:10.2337/db11-1019.
- Canfora EE, Jocken JW, Blaak EE. Short-chain fatty acids in control of body weight and insulin sensitivity. Nat Rev Endocrinol. 2015;11(10):577–591. doi:10.1038/nrendo.2015.128.
- Wong J, Piceno YM, DeSantis TZ, et al. Expansion of urease- and uricase-containing, indole- and p-cresol-forming and contraction of short-chain fatty acid-producing intestinal microbiota in ESRD. Am J Nephrol. 2014;39(3):230–237. doi:10.1159/000360010.
- Hu ZB, Lu J, Chen PP, et al. Dysbiosis of intestinal microbiota mediates tubulointerstitial injury in diabetic nephropathy via the disruption of cholesterol homeostasis. Theranostics. 2020;10(6):2803–2816. doi:10.7150/thno.40571.
- Kimura I, Inoue D, Hirano K, et al. The SCFA receptor GPR43 and energy metabolism. Front Endocrinol (Lausanne). 2014;5:85. doi:10.3389/fendo.2014.00085.
- Kikuchi K, Saigusa D, Kanemitsu Y, et al. Gut microbiome-derived phenyl sulfate contributes to albuminuria in diabetic kidney disease. Nat Commun. 2019;10(1):1835. doi:10.1038/s41467-019-09735-4.
- Li P, Wang H, Guo L, et al. Association between gut microbiota and preeclampsia-eclampsia: a two-sample Mendelian randomization study. BMC Med. 2022;20(1):443. doi:10.1186/s12916-022-02657-x.