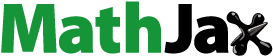
ABSTRACT
Border regions are often not very well connected to the national urban and economic centres and hence perform less well in terms of GDP per capita and unemployment. Cross-border commuting might be a way to improve the economic performance of border regions. This study explores the impact of a set of socio-economic, infrastructural or cultural explanatory variables that drive cross-border commuting in the Dutch-German-Belgium border regions for all outgoing commuters but also by gender, education and age. We found that cross-border commuting is a small-scale phenomenon, but the flows largely respond in the theoretically expected way to regional economic differences. Higher wages in the living region go together with lower cross-border outcommuting. More unemployment in the living region will make international outcommuting rise. Bordering regions with higher scores on the EU regional competitiveness index give lower international outcommuting. Quality of infrastructure does not show significant results. If the language on both sides of the border is the same, this gives more cross-border outcommuting. Males, medium educated and elderly workers show very similar outcomes as the model for all commuters, while cross border commuting of females and higher educates is hardly influenced by differences in the regional economic situation.
1. Introduction
According to EU-figures, EU border regions cover 40% of EU territory, account for 30% of the EU population and produce 30% of the EU GDP. In spite of this economic importance, border regions are within their countries often peripheral regions with lower economic growth in terms of GDP and employment and suffer from higher levels of unemployment (EC Citation2017). The study of cross-border labor markets and cross-border commuting becomes more and more important since increasing efforts are undertaken by the European Commission and the OECD to boost economic growth, integration and cohesion in border regions (OECD Citation2013; EC Citation2017).
The policy reasoning is clear. The economic performance of border regions can be improved by means of more cross-border interaction between regions on both sides of the border in order to generate more economies of scale and agglomeration benefits (Möller et al. Citation2018; Lundquist and Tripple Citation2013). In that respect a high degree of cross-border labor mobility is desirable to help employment adjust favorably to changing demand conditions, to boost innovation and economic growth and to stimulate regional integration (Haas and Osland Citation2014; OECD Citation2013). For achieving more cooperation and integration, economic growth and prosperity, cross-border cooperation has been the domain of EU Interreg funding since 1990. For the current period 2014–2020 the EU invests almost 6 billion euro in cross-border programmes. An inefficient allocation of labor resources may negatively affect the longer-term employment level and growth rate of potential economic output and, in the short run, limit the pace at which an economy grows. Therefore, the free movement of labor between countries still constitutes one of the central principles of the EU and is an important component of the completion of the single market (EC Citation2017).
Despite the efforts, cross-border commuting is still a small phenomenon. Some studies conclude that cross-border immobility is more common than cross-border mobility (Buch, Schmidt, and Niebuhr Citation2009; Nerb et al. Citation2009; Bouwens Citation2004; Van Houtum and van der Velde Citation2004). Borders are persistent obstacles that are affected by cross-border issues, such as language differences and accessibility, lack of information, differences in tax and social security systems and insufficient acceptance of qualifications (Edzes, van Dijk, and Venhorst Citation2018; EC Citation2017; Nerb et al. Citation2009). However, empirical evidence is scarce and little is known about the actual influence of cross-border obstacles on hindering commuting (Edzes, van Dijk, and Venhorst Citation2018). As the European Commission (EC Citation2017) concluded herself, collecting data and evidence on border obstacles is the first necessary step towards resolving this lack of evidence, but since then only limited resources have been invested in collecting and analyzing information on border difficulties and complexities.
In this study we use a unique data set of cross-border commuting flows from border regions for all workers, but also for specific groups of workers, like by gender, education and age. A model is constructed for cross-border commuting for Dutch, German and Belgium border regions over the period 2006–2013. Also this dataset has its limitations: we can only study outgoing commuting from the border region of living to the country of working. However, this dataset allows us to test whether or not cross-border commuting flows can be explained by economic factors like wages and unemployment or that other factors dominate. The main hypothesis is that competitive regions with higher wages and lower unemployment show lower cross-border out-commuting rates than less competitive regions with higher unemployment and lower wages, taking also into account other explanatory variables reflecting obstacles like language, accessibility and differences in institutions. We estimate this model for all commuters, but also for specific groups of commuters by gender, education and age.
In the next section we will first discuss the theoretical and empirical perspectives of cross-border commuting and in Section 3 we present the model we use to explain cross-border commuting. Section 4 describes the data on cross-border commuting that we have used and presents descriptive statistics of cross-border commuting flows between the three countries we analyse. Section 5 presents and discusses the estimation results. Cross-border commuting can be explained by (i) the regional wage rate, (ii) the regional unemployment rate, (iii) the level of competitiveness, (iv) the share of highways as accessibility measure, and (v) a common language dummy for border regions between different countries. Finally, Section 6 presents the conclusions and policy implications from our analysis.
2. Theoretical and Empirical Background
According to the official EU definition, a cross-border commuter is anyone who works in one member state, but lives in another member state from which he or she starts and returns in principle on a daily basis, but at least once a week.Footnote1 Cross-border labor market mobility differs from other forms of mobility, such as migration, because it takes place at regular (daily, at most weekly) intervals. At the beginning of such an interval the commuter moves towards his or her job in another country, while at the end of that interval the commuter returns to the home country. The area of living and working can be called a catchment area: the smallest possible area within which inhabitants have access to both living facilities and jobs (Gerber Citation2012; Hanson and Pratt Citation1988). Because of the coincidence of both a temporal and a spatial perspective, the notion of region and regional labor markets becomes important. The limited distance that someone can travel within a certain amount of time determines in essence the size of a regional labor market (Lundquist and Tripple Citation2013) and thus also the potential options for cross-border commuting. Due to lack of suitable data, cross-border commuting studies usually analyse cross-border commuter flows between a limited number of countries. These studies do show that these flows are usually small and there are only substantial flows for regions in a limited number of EU countries in the western and central part of Europe.
Boekema (Citation2000) and Van Dijk and Zanen (Citation1998, Citation2000) for the German-Dutch case, state that a cross-border labor market can only flourish in case of a structured, systematic way in which information is gathered and exchanged, which currently is still not the case. Bouwens (Citation2004) and Knotter (Citation2014a) describe the history of cross-border labor in the mining districts in the Dutch-Belgian-German borderland around Aachen and Liège that cross-border mobility varied considerably over time diminished sharply after the 1970s due to a conscious policy of regionalization of mining labor markets with the aim of binding the local labor force to the region.
When it comes to the functioning of cross-border labor markets and the determinants of cross-border commuting, we can distinguish different theoretical frameworks. (Edzes, van Dijk, and Venhorst Citation2018). In the simple neo-classical approaches cross-border commuting is driven by differences in economic factors like differences in wages and job opportunities on both sides of the border. More sophisticated theories take into account the multidimensional nature of borders, which lead to alternative explanations ranging from economic geographic models of uneven development between regions and the consequent development of push–pull factors in the labor market to post-structural observations of “mental thresholds” (Knotter Citation2014b). These models can include both rational and emotional explanatory frameworks (homo economicus versus homo socialis) (Jacob, Munford, and Rice Citation2019; Chilla and Heugel Citation2019; Huber and Nowotny Citation2013; Spierings and Van der Velde Citation2013, Citation2008; Van Houtum and van der Velde Citation2004). The homo economicus is driven by opportunities to maximize productivity and wages as postulated in rational choice approaches and the Human Capital Theory (Van Houtum and van der Velde Citation2004; Becker Citation1964). Spierings and Van der Velde (Citation2013, Citation2008) introduce the concept of “bandwidth of unfamiliarity” to understand the lack of mobility. Unlike rational choice approaches, barriers are considered endogenous: job seekers and entrepreneurs themselves raise the obstacles. Instead of wages and opportunity-driven job seekers, people might just focus on communities where they feel at home and that have common value patterns. See also Klatt (Citation2014).
Area-based factors emphasize the characteristics of the region that stimulate or hinder, that is to say, push and pull cross-border movements. Besides economic factors like regional differences in wages and unemployment on both sides of the border, also factors like physical constraints, infrastructure and transport, rules and regulations, institutions and so on are of importance (Chilla and Heugel Citation2019; Lundquist and Tripple Citation2013; OECD Citation2013; Nerb et al. Citation2009). The better the conditions in terms of e.g. good accessibility and language similarity, the easier commuting and the more integrated regions will be. Topaloglou et al. (Citation2005) set up a typology of regions on the basis of which the level of integration is determined and found that simply on the bases of a common language the Dutch-Belgium border is more integrated than for instance the Dutch-German border.
For this study a unique data set of cross-border commuting flows between the Netherlands and the neighboring countries Belgium and Germany is available for which we know the NUTS-2 border-region where they live and the country where they are working. Besides aggregated flows for all workers we can also distinguish between specific groups of workers by gender, education and age. The data are available over the period 2006–2013. Because we only have aggregated data we will use an area-based approach in which the flows will be related to characteristics of the region. Based on the foregoing theoretical approaches we will use economic variables, like wages and unemployment, but also take into account the aspects of accessibility and familiarity.
Standard neo-classical theory predicts that the higher wages being paid in a region are, the lower the need to search for jobs across the border (Van Houtum and van der Velde Citation2004). Hence, more workers living close to the border between two countries will look for a job across the border if the wage in the own region is relatively low (Rupert and Wasmer Citation2009). Gottholmseder and Theurl (Citation2007) analyse commuting flows of the Austrian region of Vorarlberg to neighboring countries of Switzerland, Germany and Liechtenstein. Buch, Schmidt, and Niebuhr (Citation2009) study commuting between Danish and German border regions, while Mathä and Wintr (Citation2009) analyse commuting of Luxemburg to and from border regions in neighboring countries Germany, Belgium and France. Chilla and Heugel (Citation2019) study the driving forces in cross-border commuting in the Alpine Macro-region. Heinz and Ward-Warmedinger (Citation2006) and Slusarciuc (Citation2015) study and review studies on cross-border migration and commuting in a wider EU perspective. All these studies show that wages are an important explanatory variable for commuting. Also variables as partnership, age, children, employment sector, open mindedness, distance to nearest border, commuting time and language differences are sometimes mentioned as being relevant. Weterings (Citation2015) and Marlet, Oumer, and Van Woerkens (Citation2014) show for The Netherlands, Germany and Belgium that chances on a job greatly improve once barriers at the border would be lifted. Based on an analysis of commuting behavior between Belgium, France, Germany and Luxembourg between 1996 and 2003, Mathä and Wintr (Citation2009) indeed found that that higher (relative) wages attract incoming commuters while higher unemployment led to more outgoing commuting. Neo-classical and human capital theory also predict that when unemployment is high in the border region of a country, more job searchers will be looking for job opportunities across the border (Nerb et al. Citation2009).
Besides variables like wages and unemployment also the economic strength of a region, i.e. the more competitive a region is compared to neighboring regions, the more attractive this region will become for both workers and firms (OECD Citation2013). More competitiveness means more opportunities for firms to make profits and thus create jobs in that region for workers who earn higher wages (Lundquist and Tripple Citation2013).
Accessibility or connectivity is crucial for cross-border commuting (Lundquist and Tripple Citation2013) because better accessible regions are more attractive for commuters because it reduces travel time. As an indicator we take the length and quality of the infrastructure in border regions. More motorways in the border region make it easier for possible cross-border commuters to arrive at the border and move to their job in a neighboring country. A better local infrastructure implies more cross-border commuters (Nielsen and Hovgesen Citation2008).
Because communication between workers is important it is likely that a common language between bordering regions of two neighboring countries means that commuting will be higher than when the language is different (Bartz and Fuchs-Schündeln Citation2012; Mantegazzi, McCann, and Venhorst Citation2019). Even though different languages are less of a problem in border regions than in non-border regions, it does still mean a barrier for cross-border commuters. Therefore, we hypothesize that cross-border commuting will be higher in case of a common language (Bartz and Fuchs-Schündeln Citation2012; Mathä and Wintr Citation2009).
We also tried to get a hold of house prices in these border regions that could act as explanatory variable for cross-border commuting. Low house prices in a border region of one country may stimulate people living in a neighboring country, with higher house prices, to emigrate to this other country, where houses are cheaper, while they still hold their job in the original country. Unfortunately, we could not find similarly defined house prices for all border regions of our three countries, so this variable was discarded.
Based on the theoretical approaches and the empirical studies mentioned above and taking into account the specific nature of the aggregated commuting flows that are available for our analysis, we have limited choice with regard to the set of explanatory variables. Therefore, in our empirical model we test four area-based economic characteristics that are considered to be important in the literature on the explanation of cross-border commuting: wages, unemployment, competitiveness and infrastructure of the region. We use language similarity as important determinant reflecting familiarity. In addition to that we use a set of regional dummies to cover other relevant issues, like institutional differences etc.
Gerber (Citation2012) argues in a study about cross-border commuting in the Benelux that besides regional economic differences on both sides of the border, insight into the psychosocial barriers at the individual level are of importance for understanding cross-border commuting flows. Lin, Allan, and Cui (Citation2015) conclude in their review of the commuting literature that commuting patterns differ substantially by individual characteristics like gender, education and age. It is a general finding in the literature that women commute less and over a shorter distance than men (Jacob, Munford, and Rice Citation2019). One reason is that women are still the primary care giver for children and secondary income earners in a household. Psychological explanations seem relevant. Roberts, Hodgson, and Dolan (Citation2011) find for instance that commuting has an important detrimental effect on the psychological health of women, but not of men. Women's greater sensitivity to higher commuting time seems to be a result of their larger responsibility for day-to-day household tasks, including childcare and housework. Also women are more likely to use public transport and non-motorized transport, particularly walking (Miralles-Guasch, Martínez Melo, and Marquet Citation2016; Crane Citation2007), which of course limits their range to work further away. Therefore, women's willingness or ability to trade-off longer commutes against other aspects of job characteristics like higher wages, seems to be more restricted (Jacob, Munford, and Rice Citation2019). Cristaldi (Citation2005) concludes from her detailed study of gendered commuting patterns in Italy that Local Labor Systems for men and women greatly differ. Women are not only “constrained” within given physical spaces (smaller than men), but obliged to make the best of the limited work opportunities available in that limited space. Because it is likely that the average commuting distance for cross-border commuting is larger than for domestic commuting, we expect that the explanatory power of the economic variables will be much larger for males than for females.
Besides for males we expect also that higher educated will show higher cross border commuting rates. It is well-known that lower educated and elderly may face higher levels of unemployment. This may make their need higher to search for a job on the other side of the border. On the other hand, lower educated and elderly workers may find it more difficult to find work in another country, because they are less flexible than young and higher educated workers. Schwanen, Dieleman, and Dijst (Citation2001) show that the relationship between age and distance traveled is weak. Levinson (Citation1998) found that middle-aged commuters have longer commutes than younger and older commuters. The foregoing suggests that the response to the explanatory variables we use in our model might differ by gender, education and age. Therefore, besides estimating the model for the total flow of commuters, we will also estimate the model for cross-border commuting flows by gender, education and age-group of the commuters.
3. A Model of Cross-Border Commuting
Cross-border commuter flows comprise of workers living in one country (living country), but work in another, neighboring, country (working country). We focus on commuting between border regions, but our data set only contains information of outgoing commuting form the border-region where the commuter lives. With regard to the work destination we only know the country of working but not the region in which one works. So, our analysis is limited to outgoing commuting from the border region of living to the country of working. This lack of information about the working regions also limits the choice of explanatory variables. This implies that in our empirical model we explain the flow of cross-boundary commuters who live in a NUTS-2 border region during period t, i.e. from point in time t-1 through t, but work somewhere in a neighboring country. This commuter flow is taken as share of the total number of workers living in this border region at time t-1. In this way the size of the region will not matter when we look at cross-border commuting. The same approach will be used when the model is estimated for separate groups by gender, education and age. Male commuters in a border region will then be divided by the (lagged) male employed labor force living in that region. Other types of commuting rates are defined accordingly. The dependent variable of our model will hence be:(1)
(1) where Yijkt is the flow of commuters living in a NUTS-2 border region i of country j, but working in the neighboring country k during period t, i.e. between points in time t-1 and t. This flow Yijkt is taken relative to Eijt−1, which is the stock of the total employed labor force living in region i of country j at the start of period t, which effectively is the end of time t-1. This share of commuter flows is available for all bordering NUTS-2 regions of the Netherlands, Germany and Belgium for 2006–2013.
As far as explanatory variables for (1) are concerned, we will only use state variables referring to the situation at the start of period t, i.e. ultimo t-1. The theoretically relevant variables are based on the various commuting studies mentioned earlier. Due to lack of suitable regional data, our empirical analysis can only be based on a limited number of variables. Therefore, in our empirical model we employ four area-based economic characteristics to explain cross-border commuting: wages, unemployment, competitiveness and infrastructure of the region and use language similarity as important determinant reflecting familiarity. In addition to that we use a set of regional dummies to cover other relevant issues, like institutional differences.
We use the real wage rate, based on the standard neo-classical theory that the higher wages are being paid in a region and the larger the difference with the wages on the other side of the border, the lower the need to search for jobs across the border.
Regional data on real wages are only available at a NUTS-1 level and not at the NUTS-2 level for which we have commuting flows. We therefore have no other option than to use the real hourly wage rate, w/h, per NUTS-1 region m of country j as this was unavailable at NUTS-2 levels. Here w represents real wages, i.e. nominal wages corrected for inflation, and h refers to the number of hours worked. These wage rates refer to time t-1. As a consequence, for all NUTS-2 border regions i that build a NUTS-1 area m, we assume the same wage rate as in the whole NUTS-1 area. In our analysis, (real) wages per hour worked per NUTS-2 border region are then:(2)
(2) The variable defined in (2) reflects differences in the real wage between the regions where the commuters live. This variable captures differences in the wage level between regions, but also partly reflects institutional differences between countries.
Second, a related variable makes a comparison between wage rates in the directly bordering regions on both sides of the border, thus reflecting more directly the wage differentials between these border regions. This variable consists of the wage in a NUTS-1 region m of a home country j on one side of the border and the wage rate in a directly bordering NUTS-1 region m of neighboring country k, where j≠k. Again, all NUTS-2 border regions i that build a NUTS-1 area m, have a similar wage rate as the one in the NUTS-1 area. The larger the difference between the wage rates in two neighboring regions will be, the larger the cross-border commuting between these two regions is. This second wage variable is defined as:(3)
(3) Third, neo-classical and human capital theory also predict that when unemployment is high in the border region of a country, more job searchers will be looking for job opportunities across the border. Therefore, we take unemployed job searchers living in border region i of country j, as a share of the total labor force living in region i of country j, as our explanatory variable for the cross-border commuting rate:
(4)
(4) where u/lf is the share of unemployed living in NUTS-2 border region i of country j in the NUTS-2 labor force living in NUTS-2 region i of country j both at t-1. We assume that a higher unemployment rate in the living region will increase the likelihood of commuting across the border for work.
Fourth, we include a variable reflecting the competitiveness of a region. We expect that higher competitiveness of a region lowers cross-border commuting from that region. The competitiveness index, compit, is defined between 0 and 100, where 0 refers to no and 100 to complete competitiveness in period t of region i, where regions refer to all NUTS-2 regions in the EU. The competitiveness index is a composite index based on eleven pillars with the objective of describing different dimensions of the level of competitiveness. The pillars are designed to capture short- as well as long-term capabilities of the region. They are classified into three major groups: (1) key basic drivers of an economy are measured by the pillars Institutions, Macro-economic stability, Infrastructure, Health and Quality of Primary & Secondary Education (2) factors for the advancement of competitiveness: Higher Education/ Training and Lifelong Learning, Labor Market Efficiency and Market Size (3) key drivers for regional improvement: Technological Readiness, Business Sophistication and Innovation. (for further details see: Annoni and Kozovska Citation2010). The first year of regional competitiveness, compit refers to the year 2010, whereas the first observation of our other variables refers to 2006. We approximate these missing years, 2006–2009, for each region by the average competitiveness index over all available years 2010–2013 for that region.
Fifth, accessibility or connectivity is crucial for cross-border commuting. As an indicator we take the length and quality of the infrastructure in border regions, i.e. the share of highways in the border region land surface. More highways in the border region make it easier to work in a neighboring country. Of course, accessibility on both sides of the border are of importance. As mentioned before, we know the region where the commuter lives, but we do not know the working region, so we only know the working country. However, we do know that most cross-border commuters work in the region just across their living, border, region. Therefore, we use the quality of infrastructure in the border region that lies exactly opposite to this living region as indicator for the quality of the infrastructure in the working region. Accessibility, i.e. the quality of the regional infrastructure in cross-border regions is operationalized as the share of highways the land surface of the border region, or:(5)
(5) where hw refers to the length of highways in NUTS-2 region i of respectively the living country j and the opposite working region in country k. We assume a width (in both directions, including idle stretches of land on both sides of the highway) of 1 kilometre. The variable land refers to the total land surface of the NUTS-2 border region under consideration. So hw/land is the share of the NUTS-2 land surface that is used for highways. Again, i refers to the border region in country j or country k and the timing is at the end of time t-1. Several other variables have been considered, like travel time to the nearest border. However, that variable turned out to perform less well, because of the interaction with the language variable.
Finally, we have a variable that indicates a common language between bordering regions of two neighboring countries. We hypothesize that cross-border commuting will be higher in case of a common language. The similarity of the Dutch and Flemish languages gives rise to a dummy variable, Dlang,i, that is unity for all NUTS-2 regions of the Netherlands that border Belgium and all Dutch speaking NUTS-2 regions in Belgium that border the Netherlands. For all other border regions between our countries the languages differ so in that case this dummy is zero.
Because not all potentially relevant explanatory variables will be captured by these six variables, we will also include a set of dummy variables for each separate NUTS-2 border region in each of our three countries. We get: (i) five dummies for each Dutch NUTS-2 border region with Germany, (ii) four dummies for each German NUTS-2 border region with the Netherlands, (iii) three dummies for each Dutch NUTS-2 border region with Belgium, (iv) five dummies for each Belgian NUTS-2 border region with the Netherlands. These four sets of dummies might also pick up institutional differences at the country-level if the estimates within regions are similar and systematically differ from the other groups. In total we have 19 regions. We will use the Dutch province of Noord-Brabant as reference region in our estimation of cross-border commuting. Furthermore, the Dutch province of Groningen had so many missing data that its dummy could effectively be left out. So, we end up with 17 regional dummy variables. Finally, year dummies were added to our model, for each year of the period 2006–2013.
Summarizing, our dependent variable is the flow of cross-border commuters living in a NUTS-2 border region i of country j and working in neighboring country k during period t, i.e. between points in time t-1 and t, divided by the employed labor force living in this border region at the end of time t-1. Apart from the overall commuting rate, we can also estimate the model for commuting rates by gender (male/female), education (low/medium/high) and age group (15-44/45+) for each region. This means that we can identify both numerator (commuting) and denominator (employment) in (1) for each of these types. The six explanatory variables refer to states instead of flows, so they are all based on the situation at the end of time t-1. Since real wage rates as explanation for cross-border commuting are only available at NUTS-1 regions, we assume that this wage rate also holds for all NUTS-2 regions that together build this NUTS-1 region. The other explanatory variables are available at NUTS-2 levels. Finally, our model has dummy variables for each NUTS-2 bordering region in each of our three countries and a dummy giving unity when a common language in the border regions of different countries is spoken. One might criticize the above choice for explanatory variable as being crude. The thing is that we need variables for all three countries’ NUTS-2 regions for at least 2006–2013. That limits our choice of suitable explanatory variables to those presented so far. Our explanatory model of cross-border commuting will therefore be:(6)
(6) In log-linear form our model becomes
(7)
(7)
4. Data and Description of Cross-Border Commuting
The dependent variable in our model is cross-border commuting flows at the NUTS-2 level for the bordering regions shown in . shows the numbers of cross-border commuters according to our data. It shows that for each of our three countries most commuters do indeed live in the NUTS-2 border regions. shows that between 2006 and 2013, there were on average 19 thousand workers, living anywhere in the Netherlands, who commuted to Germany and 14 thousand to Belgium. In NUTS-2 border regions with Germany there were 16 thousand commuters to Germany and in NUTS-2 border regions with Belgium there were 12 thousand. So, considering only border regions does indeed capture most of the cross-border commuters.
Figure 1. NUTS-2 Border Regions for the Netherlands and Germany and for the Netherlands and Belgium (Dutch NUTS-2 Region Limburg Borders Both). Source: Eurostat.
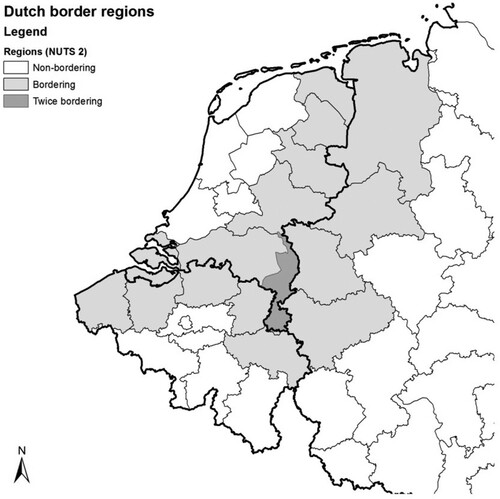
Table 1. Average Annual Cross-Border Commuters Between the Netherlands and its Two Neighboring Countries for 2006–2013 from Official Statistics (× 1000).
The average number of cross-border commuters towards the Netherlands from Germany between 2006 and 2013 was 44 thousand. Of these 44 thousand some 33 thousand lived in Germany's NUTS-2 border regions with the Netherlands. Cross-border commuters living in Belgium who worked in the Netherlands in that period amounted to 37 thousand, of whom 32 thousand lived in Belgian NUTS-2 border regions with the Netherlands. So, most cross-border commuters do indeed live near the border. also points to another typical phenomenon, which is that the number of commuters living in border regions of both neighboring countries of the Netherlands, but working in the Netherlands, are two to three times larger than commuters living in Dutch border regions who work in either Germany or Belgium.
shows commuting from the living NUTS-2 border regions to the neighboring working country for the Netherlands and Germany. Again, these figures show that commuting flows from Dutch NUTS-2 border regions towards Germany are substantially lower than the opposite flow. The Dutch NUTS-2 border regions with Germany are, from North to South: Groningen, Drenthe, Overijssel, Gelderland and Limburg. The NUTS-2 border regions in Germany with the Netherlands are from North to South: Weser-Ems, Münster, Düsseldorf and Köln. See for the location of the regions. Only the Netherlands’ most southern province of Limburg has relatively many commuters to Germany, reaching almost 10 thousand persons, or 1.7% of the average employed labor force living in Limburg between 2006 and 2013. Cross-border commuting shares of other Dutch border-provinces are much lower, around 0.3–0.4% of the respective employed labor forces. The share of commuters from German NUTS-2 regions towards the Netherlands is only slightly larger.
Table 2. Cross-Border Commuting of Dutch and German Border Regions, Averages 2006–2013.
Next we move to Dutch commuters towards Belgium and vice versa. shows commuters living in Dutch NUTS-2 border regions with Belgium, i.e. from West to East, in Zeeland, Noord-Brabant and Limburg. This latter province also borders Germany and there we saw that commuters going from Limburg to a job in Germany was relatively high (). However, the flow from Limburg to Belgium is much lower. The reverse commuter flow is about persons living in Belgian NUTS-2 border regions with the Netherlands, i.e. from West to East, living in West-Vlaanderen, Oost-Vlaanderen, Antwerpen, Belgian Limburg and Liège, but holding a job in the Netherlands. See for the location of the regions. Also note that many Belgian border regions have Dutch as language. This makes it easier for Belgian and Dutch workers to work at the other side of the border, except for Liège, where the official language is French.
Table 3. Cross-Border Commuting of Dutch and Belgian Border Regions, Averages 2006–2013.
shows for each region the annual commuting rates for each border region over the period 2006–2013. Although there is some variation in the time pattern over regions, for most regions the pattern is ratter similar with the highest commuting region in the beginning, lower rates in the middle of the period and raising afterwards. Like in cross-border commuters have been particularly large for the Belgian NUTS-2 region of Limburg (BE22) to the Netherlands for all years. More than 5% of the employed labor force living in Belgian Limburg in those years held a job in the Netherlands. The reverse flow of commuters from Dutch border regions towards Belgium (NL34, NL33, NL42B) is much smaller. Only the share of employed persons living in the Dutch NUTS-2 region of Zeeland (NL34) that commute towards a job in Belgium (most likely to Antwerp, with its harbor activities) is large, with 2% of the employed labor force living in Zeeland. The share of persons living in Dutch Limburg, holding a job in Belgium is now relatively small with only 0.6% (NL42B), while this share was almost 2% for commuters living in Dutch Limburg and holding a job in Germany (NL42D). So, people living in Dutch Limburg preferably work in Germany, probably because of the abundance of jobs in the nearby Ruhr area. There are much less jobs in the Belgian region of Limburg (BE33) that lies on the other side of the Dutch province of Limburg. This may also explain the high commuting rate from this Belgian Limburg (BE22) towards the Netherlands and causes more competition in the Dutch province of Limburg that might explain the high commuting in this province towards Germany. The relatively low commuting rates from the Belgian region of Liège (BE33) might be due to the French language that is spoken there, which is an additional barrier that makes commuting to and from the Netherlands more difficult.
Figure 2. Annual Rates of Cross-Border Commuting Between NUTS-2 Border Regions in the Netherlands and its Two Neighboring Countries for 2006–2013 (%).
BE25=West-Vlaanderen, BE23=Oost-Vlaanderen, BE21=Antwerpen, BE22=Belgian Limburg, BE33=Liège, DE94=Weser-Ems, DEA3=Münster, DEA1=Düsseldorf, DEA2=Köln, NL11=Groningen, NL13=Drenthe, NL21=Overijssel, NL22=Gelderland, NL=42D = Limburg, bordering Germany, NL34=Zeeland, NL33=Noord-Brabant, NL42B = Limburg, bordering Belgium. Source: Eurostat and Statistics Netherlands.
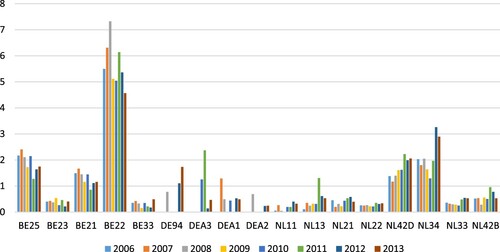
As a final descriptive statistics we present detailed statistics of the commuter rates by gender, education and age for each border region in Table A1 in the Appendix. It is clear that commuting rates for males are substantially higher than for females for all regions. The difference is most outspoken for the outflow from the Dutch border regions. The commuting rates by education level show a lot of variety. Overall, the commuting rates of higher educated seem to be a bit higher, while medium educated show lower commuting rates. Also by age groups there is substantial variation by region, but less than by gender and education. Within regions the variation between age groups is limited.
5. Estimation Results
This section presents and discusses the estimation results of the model explaining the rate of cross-border commuting in (6) between 2006 and 2013. presents the OLS estimation results of the models of the commuting share for all workers and of the commuting shares by gender, education and age group.
Table 4. Estimation Results (OLS) of Model (7) for Cross-Border Outcommuting from NUTS-2 Border Regions of Living to the Country of Working, 2006–2013.
For the overall cross-border commuting rate, in the first column, we find that a 1%-point rise in the log of real hourly wages in the living region (NUTS-1) will lead to a fall in the log of the cross-border outcommuting rate of some 26%-points. So, in line with expectations a higher wage rate in the living region gives that less workers living there want to go abroad for work. In addition to the wage level in the living region, we included also a wage variable reflecting the wage differentials between the living border region in one country and the opposite border region in the neighboring country. The results show that a 1%-point rise in wage differences gives a 13%-point higher outflow of cross-border commuters moving from the low wage to the high wage border region.
The estimation results in also show that a 1%-point rise in the regional unemployment rate will raise regional outcommuting by 0.6%-points. This implies that in line with our expectations, more unemployed in a border region will raise cross-border outcommuting, since a rise in unemployment will enlarge the search-for-work area to regions with more prosperous job opportunities.
A 1%-point rise in the region's index of competitiveness will make cross-border outcommuting from that region fall by 6%-points. As expected, the more competitive a (border) region is, the more attractive this region will become for both firms and workers to operate in. More competitiveness thus means, first, more opportunities for firms to make profits and create jobs and, second, for workers to find a job and to earn higher wages and there are hence less incentives to look for work across the border. Theoretically we expected that better accessibility between border regions will go together with higher outcommuting flows. However, although the quality of infrastructure, i.e. the share of highways in either the border regions of the living country or in the opposite border regions of the working country, show the expected positive sign, the coefficients are not significant at conventional levels. Several other variables have been considered to reflect accessibility, like travel time to the nearest border. We first used the distance between (the centre of) a NUTS-2 border region in the living country and (the centre of) the opposite working country. Notice that we do not know in which working region the cross-border commuter works, so we take the entire country. Second, we used the travel time in minutes (by car) to get from (the centre of) a border region in the living country and (the centre of) the opposite working country. Third, assuming most cross-border commuters will probably hold jobs close to the border, we assume these commuter jobs are (mostly) located in the NUTS-2 border region of the working country opposite to the living NUTS-2 region. Finally, we made a similar assumption for the travel time, i.e. the time needed to move between (the centre of) the NUTS-2 border region in the living country and the opposite NUTS-2 border region in the working country. All in all, these variables either had no effect at all or even had the wrong sign. So, in the end we used the variable the share of highways, although this variable has also its limitations. For example, along the German – Dutch border there is a highway A31 running parallel to the border. However, if there are enough exits, this still might increase accessibility. For instance, the trip from Groningen to Enschede is now quicker via the A31 in Germany than following shorter routes within the Netherlands. As expected, a common language on both sides of the border makes it easier for commuters to work abroad and hence, shows a highly significant positive effect. The results we find for the relation between cross-border commuting and differences in wages, unemployment and a similar language or in line with other studies, like e.g. the very similar approach by Mathä and Wintr (Citation2009).
Finally, we discuss the regional dummy variables for each of the NUTS-2 border regions of our three countries. The dummy variables take up unobserved regional differences and specific regional characteristics. The group of dummy variables for regions in each country can also account for institutional differences between the countries. The results show that the latter maybe at hand, because the coefficients for the regions of a particular country are rather similar. Therefore, we discuss the results in four groups: (i) Dutch regions bordering Germany, (ii) German regions bordering the Netherlands, (iii) Dutch regions bordering Belgium, (iv) Belgian regions bordering The Netherlands. The numeraire will be the Dutch province of Noord-Brabant bordering Belgium. The other Dutch regions bordering Belgium, Zeeland and Limburg, show a negative significant coefficient so compared to the numeraire of Noord-Brabant, so there is less cross-border outcommuting.
Most Belgian regions bordering The Netherlands have a positive, but not necessarily significant, coefficient. Nevertheless, compared to the opposite commuting, there is higher commuting from Belgium towards The Netherlands than the other way around. The strong positive effect of the dummy on Limburg-Germany compared to the dummy on Limburg-Belgium indicates that more commuters go from Dutch Limburg towards Germany, most likely the Ruhr area, than from this region towards Belgium. Also the Dutch regions Overijssel and Gelderland that border Germany show significant positive coefficients implying higher commuting rates from the Netherlands to Germany than to Belgium. The highest positive and significant dummies are found for the four regions in Germany bordering the Netherlands, indicating that higher commuting rates are found in these German border regions with The Netherlands than from the Netherlands towards Germany.
Next we will discuss the most relevant outcomes for cross-border commuting rates by gender, education and age, which are also presented in . The outcomes for the language dummy are very similar in all models, indicating that the same language leads to higher commuting rates for all distinguished groups of commuters.
5.1. Gender
We start with discussing the results for cross-border outcommuting by gender in the second and third columns of . For males, the outcomes compare well to the outcomes of the overall commuting model of the first column, although the significance of the wage differences between regions on both sides of the border and the share of highways becomes insignificant. However, for females all coefficients are insignificant. This outcome confirms the theoretical expectations formulated in Section 2. Women commute less and over a shorter distance than men because they perform other tasks in the household, including childcare, that restricts their choice set with regard to the work location. Personal and household factors are dominant for this choice above economic variables related to job opportunities. As Cristaldi (Citation2005) concludes from her detailed study of gendered commuting patterns in Italy that a Local Labor System for men and women greatly differ. Our results also suggest that women are not only “constrained” within given physical spaces (smaller than men), but obliged to make the best of the limited work opportunities available in that limited space and cross-border commuting falls outside the Local Labour System of most women.
As far as regional dummies for both male and female commuting are concerned, we find that for Dutch-Belgian commuter flows in both directions most dummies become insignificant, while for the Dutch-German flows and vice versa the results are pretty much the same as for the overall model.
5.2. Education
In columns 4, 5 and 6 of the results are presented for cross-border outcommuting by the level of education of the commuter, as share of the total employed labor force by that education, living in the border region. For those with a medium level of education, which is the largest group, the results are very similar to the overall model. A 1%-point higher wage rate in the home region lowers medium level educated cross-border commuters with 20%-points. However, wage differentials between the home and opposite foreign regions are no longer significant. The latter also holds for the other two education levels. Accessibility becomes a significant factor for medium educated: a 1%-point rise in the share of highways of the living region will raise the regional outcommuting rate of medium educated workers by 1.7%-points. A similar rise in the share of highways in the regions that lie in the bordering country exactly opposite to the original living region makes cross-border outcommuting from this original region fall by 0.5%-points. Do note that these effects are only weakly significant at 10%. There is a similarly weak effect of unemployment on outcommuting of medium educated workers of 0.4%-point.
Cross-border outcommuting of low educated workers living in border regions is not sensitive for the wage level, but positively affected by the unemployment rate in that region. A 1%-point increase in the unemployment rate will rise cross-border out-commuting of low educated by 1.5%-points. In addition, a rise in the competitiveness index of 1%-point makes cross-border outcommuting of low educated fall by 11%-points. Both effects for unemployment and competitiveness for low educated are twice as high as for the overall group. Also, a similar language stimulates outcommuting, but the share of highways is insignificant. The effects of the various regional dummies in the model for low and medium level educated commuters largely correspond to the ones of the general model, except for the Belgian regions.
Finally, we move to the results for high educated cross-border commuters. In this case all the economic variables become insignificant, only a similar language has a significant positive effect on commuting. The regional dummy variables for high educated commuters are again largely the same as we found for low and medium level educated. It might be that high educated commuters are not very sensitive to general regional economic variables like wage level and unemployment, because they are, like females, mainly driven by more personal motives to work across the border.
5.3. Age
The results for the models of commuting by age group are in the final two columns of . The estimated models for both age groups compare well to the outcome of the general model specification, although significance levels are sometimes a bit lower. Most striking is that for the group of elderly commuters there is a high and significant (positive) effect of wage differentials between the home and foreign regions on cross-border outcommuting like for the overall group, while this coefficient is not significant for the younger group. A 1%-point rise in these wage differentials rises cross-border outcommuting by 19%-points. The effect of the unemployment rate on commuting is two times larger for the older group, with 0.9%-points, than for the younger group, with 0.4%-points.
6. Concluding remarks
We found that cross-border commuting is a relatively small-scale phenomenon, which involves generally less than 1% of the employed labor force living in NUTS-2 border regions of the Netherlands, Germany and Belgium. This implies there is potential for policies to stimulate cross-border commuting, particularly when the labor market situation on different sides of the border may also be differing. Looking at total numbers of cross-border commuters, we find that on average between 2006 and 2013, the number of workers on Dutch jobs that live in German or Belgian border regions with the Netherlands are two to three times larger than the number of workers on German or Belgian jobs that live in Dutch border regions. There were almost 16 thousand workers who lived on the Dutch side along the Dutch-German border, but held a job in Germany, against 33 thousand who lived on the German side along the Dutch-German border but held a job in the Netherlands. For the Dutch-Belgian border these numbers were almost 12 thousand workers living at the Dutch side along the Dutch-Belgian border but working in Belgium, against almost 33 thousand workers living at the Belgian side along the Dutch-Belgian border, but holding a job in the Netherlands. In other words, there are two to three times as many cross-border commuters that live in German or Belgian border regions with the Netherlands but hold a job in the Netherlands than vice versa.
To get more insight into the response of commuters to economic factors, we estimated an explanatory model for cross-border commuters living in border regions of the Netherlands, Germany and Belgium towards any one of these neighboring countries for work. So, our analysis is restricted to outgoing cross-border commuting from the border-region of living to the country of working. We found that cross-border outcommuting is lower when the wage rate of workers in the living region is higher and the wage differences between regions on both sides of the border are smaller. This is particularly so for male and medium level educated commuters and holds for all ages. Female cross-border commuters make other assessments in the trade-off between work and private and are driven by other motives and preferences than regional economic factors like wages, unemployment and competitiveness. The same holds for high educated commuters. They are also driven by other motives then the ones suggested by our variables. The share of highways in border regions in which one lives, has only a minor effect on cross-border outcommuting. More highways in a region are expected to lead to more cross-border passages, certainly in the current EU-area with open borders, but the effect in our models is often not significant. Only medium level educated commuters seem to benefit from more highways. Higher unemployment in the living border region will particularly lead to more cross-border commuting for males, lower educated and elderly. Finally, we found that the more competitive a region is, the lower cross-border coutommuting will be for most commuters. Obviously a more competitive region offers enough job opportunities for the workers who live there, so cross-border commuting is then less necessary. The other way around, a less competitive region leads to more cross-border outcommuting, like the Belgian region of Limburg.
A common language has a positive effect on international outcommuting, which in our case refers to Dutch border regions and Dutch-speaking Belgian border regions. This holds for all different types of commuters. When the regional dummy variables are combined to yield some kind of country-level effect, we find that, indeed, there are systematic differences in the coefficient values between countries.
Dutch commuting flows to Belgium are smaller than to Germany, but at the same time German commuting to the Netherlands is larger than vice versa. Because the value and significance of the coefficients for the dummies are very similar if grouped by country, it seems very likely that the dummies also reflect differences in institutions between the three countries. It is well-known that economy-wide wage negotiations in the Netherlands are part of intensive consults between trade unions and employer organizations that have resulted in moderate wage developments in the past forty years. Belgium and Germany have their own wage development settings, giving different wage developments than in the Netherlands. Furthermore, house prices and fiscal rules for, e.g. tax deductions for mortgages and social security differ substantially between countries.
These conclusions lead to important, but also rather obvious, policy implications. Although, cross border commuter flows are still small, we clearly find that cross-border commuters respond in the theoretically expected way to cross-border differences in unemployment and wages. Cross-border commuting flows are lower from regions with relatively high wages and low unemployment. More competitive regions also show lower commuting. In addition, we find that infrastructure hardly matters for cross-border commuting. Having a common language is also important implying that policy programs that stimulate language training in border regions without such a common language, i.e. the Dutch-German border regions and the Limburg-Liège border region, may stimulate cross-border commuting.
In the end, cross-border commuting is the result of push and pull factors that seem to work out differently for different groups. None of the explanatory variables works for female and high educated commuters, apart from language, so for them other factors, such as childcare facilities, job perspectives and so on, mainly determine cross-border movements. For low educated males, the unemployment in their living region is an important push factor and for medium educated males the wage rate in the foreign country acts as pull factor and also accessibility becomes important.
We provide an empirical analysis of cross-border outcommuting of the Netherlands with its two neighboring countries Germany and Belgium, based on NUTS-2 border regions. This regional aspect may be more adequate than a national approach would be, since most cross-border commuting is from (NUTS-2) border regions. At the same time this regional aspect limits the availability of adequate explanatory variables for all border regions of our three countries. In that sense our analysis is no more than a first step towards a more thorough analysis of cross-border commuting. A potential way to improve the economic performance of border regions is to stimulate more cross-border interaction by policies removing barriers in order to generate more economies of scale and agglomerations benefits. There is also a need for developing a better conceptual theoretical framework for the analysis of cross-border commuting. Sohn and Licheron (Citation2018) use an innovative multidimensional conceptualization of border effects into four factors (separation, contact, differentiation and affirmation) to examine the effects of borders on the performance of metropolitan areas in Europe. They find that economic differentials display positive effects, while cultural differences have negative effects on metropolitan performance. Other factors show more ambivalent outcomes. This approach might also be a promising attempt for the analysis of cross-border commuting but requires also that better data sets become available that allow the analyses of linked employer and employee information in combination with regional characteristics.
Disclosure statement
No potential conflict of interest was reported by the author(s).
Notes
1 Regulation (EC) No 883/2004 of the European Parliament and of the Council of 29 April 2004 on the coordination of social security systems (Text with relevance for the EEA and for Switzerland) https://eur-lex.europa.eu/legal-content/GA/TXT/?uri=CELEX:02004R0883-20140101. Accessed at September 2 2019.
References
- Annoni, P., and K. Kozovska. 2010. EU Regional Competitiveness Index 2010. Luxemburg: Publications Office of the European Union.
- Bartz, K., and N. Fuchs-Schündeln. 2012. The Role of Borders, Language and Currencies as Obstacles to Labor Market Integration. European Economic Review 56, no. 6: 1148–63. doi: https://doi.org/10.1016/j.euroecorev.2012.05.008
- Becker, G.S. 1964. Human Capital Theory. New York: Columbia.
- Boekema, F., ed. 2000. Grensregiós en arbeidsmarkten. Theoretische en empirische perspectieven [Border Regions and Labour Markets. Theoretical and Empirical Perspectives]. Assen: Van Gorcum. [in Dutch].
- Bouwens, S. 2004. The Dynamics of Cross-Border Labor: Commuting From the Dutch to the German Part of the Euregio Meuse-Rhine, 1960–2000. Journal of Borderlands Studies 19, no. 2: 135–53. doi:https://doi.org/10.1080/08865655.2004.9695630.
- Buch, T., T.D. Schmidt, and A. Niebuhr. 2009. Cross-border Commuting in the Danish-German Border Region – Integration, Institutions and Cross-Border Interaction. Journal of Borderlands Studies 24, no. 2: 38–54. doi: https://doi.org/10.1080/08865655.2009.9695726
- Chilla, T., and A. Heugel. 2019. Cross-border Commuting Dynamics: Patterns and Driving Forces in the Alpine Macro-Region. Journal of Borderlands Studies, doi:https://doi.org/10.1080/08865655.2019.1700822.
- Crane, Randall. 2007. Is There a Quiet Revolution in Women’s Travel? Revisiting the Gender Gap in Commuting. Journal of the American Planning Association 73, no. 3: 298–316. doi:https://doi.org/10.1080/01944360708977979.
- Cristaldi, F. 2005. Commuting and Gender in Italy: A Methodological Issue. The Professional Geographer 57, no. 2: 268–84. doi:https://doi.org/10.1111/j.0033-0124.2005.00477.x.
- Edzes, A., J. van Dijk, and V. Venhorst. 2018. Der grenzüberschreitende Arbeitsmarkt der Niederlande mit Deutschland und Belgien: Jenseits von Romantik [The Cross-Boundary Labour Market of The Netherlands with Germany and Belgium: The Other Side of Romances]. In: Grenzen: Theoretische, konzeptionelle und praxisbezogene Fragestellungen zu Grenzen und deren Überschreitungen, ed. M. Heintel, R. Musil and N. Weixlbaumer, 379–400. Wiesbaden: Springer Fachmedien Wiesbaden GmbH [in German].
- European Commission. 2017. Boosting Growth and Cohesion in Border Regions. Brussels. http://ec.europa.eu/regional_policy/en/information/publications/communications/2017/boosting-growth-and-cohesion-in-eu-border-regions (accessed May 3, 2018).
- Gerber, P. 2012. Advancement in Conceptualizing Cross-Border Daily Mobility: the Benelux Context in the European Union. Eurpean Journal of Transport Infrastructure Research 12, no. 2: 178–97.
- Gottholmseder, G., and E. Theurl. 2007. Determinants of Cross-Border Commuting: Do Cross-Border Commuters Within the Household Matter? Journal of Borderlands Studies 22, no. 2: 97–112. doi: https://doi.org/10.1080/08865655.2007.9695679
- Haas, A., and L. Osland. 2014. Commuting, Migration, Housing and Labour Markets: Complex Interactions. Urban Studies 51, no. 3: 463–76. doi: https://doi.org/10.1177/0042098013498285
- Hanson, S., and G. Pratt. 1988. Reconceptualizing the Links Between Home and Work in Urban Geography. Economic Geography 64, no. 4: 299–321. doi: https://doi.org/10.2307/144230
- Heinz, F.F., and M. Ward-Warmedinger. 2006. Cross-Border Labour Mobility within an Enlarged EU. ECB Occasional Papers No. 52, European Central Bank (ECB), Frankfurt am Main.
- Huber, P., and K. Nowotny. 2013. Moving Across Borders: Who is Willing to Migrate or to Commute? Regional Studies 47, no. 9: 1462–81. doi: https://doi.org/10.1080/00343404.2011.624509
- Jacob, N., L. Munford, and N. Rice. 2019. The Disutility of Commuting? The Effect of Gender and Local Labor Markets. Regional Science and Urban Economics 77: 264–75. doi: https://doi.org/10.1016/j.regsciurbeco.2019.06.001
- Klatt, M. 2014. (Un)Familiarity? Labor Related Cross-Border Mobility in Sønderjylland /Schleswig Since Denmark Joined the EC in 1973. Journal of Borderlands Studies 29, no. 3: 353–73. doi:https://doi.org/10.1080/08865655.2014.938968.
- Knotter, A. 2014a. Changing Border Regimes, Mining, and Cross-Border Labor in the Dutch–Belgian–German Borderlands, 1900–1973. Journal of Borderlands Studies 29, no. 3: 375–84. doi:https://doi.org/10.1080/08865655.2014.938969.
- Knotter, A. 2014b. Perspectives on Cross-Border Labor in Europe: “(Un)Familiarity” or “Push-and-Pull”? Journal of Borderlands Studies 29, no. 3: 319–26. doi:https://doi.org/10.1080/08865655.2014.938972.
- Levinson, D. 1998. Accessibility and the Journey to Work. Journal of Transport Geography 6, no. 1: 11–21. doi: https://doi.org/10.1016/S0966-6923(97)00036-7
- Lin, D., A. Allan, and J. Cui. 2015. The Impacts of Urban Spatial Structure and Socio-Economic Factors on Patterns of Commuting: a Review. International Journal of Urban Sciences 19, no. 2: 238–55. doi:https://doi.org/10.1080/12265934.2015.1016092.
- Lundquist, K.J., and M. Tripple. 2013. Distance, Proximity and Types of Cross-Border Innovation Systems: A Conceptual Analysis. Regional Studies 47, no. 3: 450–60. doi: https://doi.org/10.1080/00343404.2011.560933
- Marlet, G., A. Oumer, and R. Ponds en C. Van Woerkens. 2014. Groeien aan de Grens. VOC uitgevers: Nijmegen. [in Dutch].
- Mantegazzi, D., Ph. McCann, and V. Venhorst. 2019. The Impact of Language Borders on the Spatial Decay of Agglomeration and Competition Spillovers. Journal of Regional Science, Accepted for Publication August 28: 2019. doi:https://doi.org/10.1111/jors.12464.
- Mathä, T., and L. Wintr. 2009. Commuting Flows Across Bordering Regions: a Note. Applied Economic Letters 16, no. 7: 735–8. doi: https://doi.org/10.1080/13504850701221857
- Miralles-Guasch, C., M. Martínez Melo, and O. Marquet. 2016. A Gender Analysis of Everyday Mobility in Urban and Rural Territories: From Challenges to Sustainability. Gender,Place&Culture 23, no. 3: 398–417. doi:https://doi.org/10.1080/0966369X.2015.1013448.
- Möller, C., E. Alfredsson-Olsson, B. Ericsson, and K. Overvåg. 2018. The Border as an Engine for Mobility and Spatial Integration: A Study of Commuting in a Swedish-Norwegian Context. Norwegian Journal of Geography 72, no. 4: 217–33.
- Nerb, G., F. Hitzelsberger, A. Woidich, S. Pommer, S. Hemmer, and P. Heczko. 2009. Scientific Report on the Mobility of Cross-Border Workers within the EU-27/EEA/EFTA Countries. Final Report, MKW Wirtschatfsforschung GmbH Munich and Empirica Kft Sopron, January 2009. https://ec.europa.eu/futurium/en/system/files/ged/mkw_workers_mobility.pdf.
- Nielsen, T.A.S., and H.H. Hovgesen. 2008. Exploratory Mapping of Commuter Flows in England and Wales. Journal of Transport Geography 16, no. 2: 90–9. doi: https://doi.org/10.1016/j.jtrangeo.2007.04.005
- OECD. 2013. Regions and Innovation: Collaborating Across Borders. Paris: OECD.
- Roberts, J., R. Hodgson, and P. Dolan. 2011. “It’s Driving her mad”: Gender Differences in the Effects of Commuting on Psychological Health. Journal of Health Economics 30, no. 5: 1064–76. doi: https://doi.org/10.1016/j.jhealeco.2011.07.006
- Rupert, P., and E. Wasmer. 2009. Housing and the Labour Market: Time to Move and Aggregate Unemployment. IZA Discussion Paper No. 4172.
- Schwanen, T., F.M. Dieleman, and M. Dijst. 2001. Travel Behaviour in Dutch Monocentric and Policentric Urban Systems. Journal of Transport Geography 9, no. 3: 173–86. doi: https://doi.org/10.1016/S0966-6923(01)00009-6
- Slusarciuc, M. 2015. Development of Cross-Border Areas: Study Cases Review. USV Annals of Economics and Public Administration 15, no. 1: 142–51.
- Sohn, C., and J. Licheron. 2018. The Multiple Effects of Borders on Metropolitan Functions in Europe. Regional Studies 52, no. 11: 1512–24. doi:https://doi.org/10.1080/00343404.2017.1410537.
- Spierings, B., and M. Van der Velde. 2008. Shopping, Borders and Unfamiliarity: Consumer Mobility in Europe. Tijdschrift Voor Economische en Sociale Geografie 99, no. 4: 497–505. doi: https://doi.org/10.1111/j.1467-9663.2008.00484.x
- Spierings, B., and M. Van der Velde. 2013. Cross-Border Differences and Unfamiliarity: Shopping Mobility in the Dutch-German Rhine-Waal Euroregion. European Planning Studies 21, no. 1: 5–23. doi: https://doi.org/10.1080/09654313.2012.716236
- Topaloglou, L., D. Kallioras, P. Manetos, and G. Petrakos. 2005. A Border Regions Typology in the Enlarged European Union. Journal of Borderlands Studies 20, no. 2: 67–89. doi: https://doi.org/10.1080/08865655.2005.9695644
- Van Dijk, J., and T.-J. Zanen. 1998. Grenzüberschreitende Zusammenarbeit und Arbeitsmarkt in der Ems-Dollart-Region [Cross-Border Co-Operation and Labour Markets in the Ems-Dollard Region]. In: Mobilität und Kooperation auf Grenzüberschreitenden Arbeidsmärkten: Deutschland – Niederlande, ed. Peter de Gijsel und Hans-Joachim Wenzel, 63–76. Osnabrück: IMIS [in German].
- Van Dijk, J., and T.-J. Zanen. 2000. Grensoverschrijdende samenwerking en de arbeidsmarkt in de Eems-Dollard regio [Cross-Border Cooperation on the Labour Market of the Eems-Dollard Region]. Chapter 6. In Grensregio’s en arbeidsmarkten – Theoretische en empirische perspectieven [Border Regions and Labour Markets. Theoretical and Empirical Perspectives], ed. F. Boekema, 59–82. Assen: Van Gorcum. [In Dutch].
- Van Houtum, H., and M. van der Velde. 2004. The Power of Cross-Border Labour Market Immobility. Tijdschrift Voor Economische en Sociale Geografie 95, no. 1: 100–7. doi: https://doi.org/10.1111/j.0040-747X.2004.00296.x
- Weterings, A. en G. Van Gessel-Dabekaussen. 2015. Arbeidsmarkt zonder grenzen [Labour Market without Borders]. Planbureau voor de Leefomgeving en Centraal Bureau voor de Statistiek: Den Haag. [in Dutch].