Abstract
Dermatomyositis (DM) is an autoimmune disease that primarily affects the skin and skeletal muscle. Virus infection and type I interferon-related signaling pathways play an important role in the pathogenesis of dermatomyositis. In this study, we found that the skin of patients with DM and the skin of patients with COVID-19 have similar transcriptional profiles, and identified key genes involved in dermatomyositis based on bioinformatics analysis. These hub-genes might be served as potential biomarkers for the early diagnosis and therapy of DM, including MX1, ISG15, IFIT3, IFIT1, RSAD2, IFIT2, IFI6, XAF1, IRF9, MX2.
Introduction
Dermatomyositis (DM) is a heterogeneous diffuse connective tissue disease, characterized by skin rash, chronic muscle weakness, and skeletal muscle mononuclear cell infiltration [Citation1]. DM is the most common subtype of idiopathic inflammatory myopathy (IIM). The incidence of IIM is low, and the annual incidence rate is about 1.16 ∼ 19/million people [Citation2]. The onset age has two peak periods, 10–15 years old and 45–60 years old, and the average age of onset is 40–60 years old [Citation3]. The most important clinical feature of DM is specific skin rash damage, such as Heliotrope rash, V-shaped shawl sign, and Gottron sign. Perivascular lymphocytic infiltration of activated T lymphocytes and macrophages is present in the skin of DM [Citation4].
The etiology of DM is still unclear. Both immune and non-immune mechanisms are involved in its pathogenesis [Citation5–8]. A dysregulated type I interferon response plays an important role in the development of DM [Citation9–11]. Type I IFN excess may be associated with disease severity and autoantibodies [Citation9]. In addition, some studies have suggested that the pathogenesis of dermatomyositis may be related to viral infection, especially EB virus infection [Citation12, Citation13]. The current epidemic of coronavirus disease 2019 (COVID-19) is caused by infection with SARS-CoV-2. Recent study indicates that COVID19 infection and DM may share some of the same pathogenesis, and both are associated with excessive activation of the type I interferon pathway [Citation14, Citation15]. And there are also studies suggesting that COVID-19-related myositis may be dermatomyositis [Citation16]. Therefore, it is necessary to re-analyze the differential genes between dermatomyositis and healthy control. This will provide useful information for the early diagnosis and treatment of dermatomyositis and is expected to provide new targets.
Specific skin lesions are the most important clinical manifestations of dermatomyositis and one of the diagnostic criteria. Therefore, the analysis of differential genes in dermatomyositis skin lesions is extremely important. In this study, we downloaded the GSE46239 mRNA expression profiles of DM’s skin and GSE193068 of COVID-19 patients’ skin from the website of Gene Expression Omnibus (GEO). Differentially expressed genes (DEGs) analyses were carried out by using the online analysis tool GEO2R. Gene ontology and pathway enrichment analysis were performed by R package clusterProfiler. Next, we constructed the protein-protein interaction (PPI) network of DEGs and identified these genes (module genes) that appeared in two or more analyses. Then, we selected the hub genes by cytoHubba. Finally, quantitative PCR-based detection of hub genes in muscle tissue and immunohistochemistry of skin tissue were performed. In this study, we have discovered some key genes and important signal pathways related to DM, hoping to provide new targets for the early diagnosis and treatment of DM.
Materials and methods
Microarray data analysis
The microarray expression profiling datasets GSE46239 and GSE193068 were downloaded from the Gene Expression Omnibus (GEO, https://www.ncbi.nlm.nih.gov/geo/). GSE46239 was based on the GPL570 Affymetrix Human Genome U133 Plus 2.0 Array, containing 52 samples consisting of 48 DM patients and 4 healthy controls. And the skin of patients with dermatomyositis come from the active part of the skin lesion. GSE193068 was based on GPL31171 Gene Expression Counter ® RCC – Human Immunology V2 panel, containing the skin expression of 10 COVID-19 patients and 4 healthy controls ().
Table 1. Basic information of the two microarrays.
DEGs analysis
DEGs analysis was performed through using the online analysis tool GEO2R. The expression profiles of patients and healthy controls were compared to identify the DEGs. P-values and adjusted P-values were calculated using t-tests. Genes from each sample with the following criteria were retained: |log2 (fold-change)|≥1 and P value < 0.05. Venn diagram was used to analyze the intersection of DEGs from these two datasets.
Gene ontology and pathway enrichment analysis
The Gene ontology (GO) [Citation18] is a description of gene function according to biological process (BP), molecular function (MF) and cell composition (CC). Kyoto Encyclopedia of Genes and Genomes(KEGG) [Citation19] is a systematic analysis of the metabolic pathways of gene products in cells and the functions of these gene products, which helps to study genes and expression information as a whole network. We used R package clusterProfiler [Citation20] to view the GO and KEGG enrichment of DEGs of GSE46239. And a p value <0.05 was considered statistically significant.
Protein-protein interaction (PPI) and module analysis
The Search Tool for the Retrieval of Interacting Genes (STRING) database [Citation21] (http://string-db.org/cgi/input.pl) is to study the interaction network between proteins, which helps to mine the core regulatory genes. This database contains 24.6 million proteins and >2 billion interactions including 5090 organisms. We uploaded the DEGs of GSE46239 into the STRING database and set up the interaction score ≥ 0.900 (highest confidence) as the significant threshold with hiding disconnected nodes in the network. Then, we used the Cytoscape software [Citation22, Citation23] to construct the PPI network. The Molecular Complex Detection (MCODE) [Citation24] is a functional module for clustering construction in a huge gene (protein) network. The PPI network was imported into Cytoscape, and MCODE identified the most important two modules in the PPI network. The selection criteria were as follows: degree cutoff = 2, node score cutoff = 0.2, maximum depth = 100, k score = 2. Then, we used R package clusterProfiler to perform GO and KEGG analysis of the genes in the module.
Hub genes identification and analysis
Cytohubba [Citation25] is a plug-in used by Cytoscape software to identify hub nodes. CytoHubba ranks nodes according to their attributes in the network and provides 11 topological analysis methods. The hub genes were calculated based on the Maximal Chique Centrality (MCC) topological analysis methods by using cytoHubba.
Muscle and skin tissues collection
We collected muscle samples from 24 patients with IIM who were admitted to the Department of Rheumatology, Xiangya Hospital, Central South University (Supplementary Table S1). All of them met the diagnostic criteria of 1975 Bohan/Peter Diagnostic Standard for dermatomyositis and polymyositis [Citation26, Citation27]. Other autoimmune diseases, infections and tumors were excluded. The control group included 12 patients from Department of Neurology, Xiangya Hospital, Central South University. Skin samples from 4 patients with dermatomyositis and 3 controls were collected from the Department of Rheumatology, Xiangya Hospital, Central South University. Written informed consent has been obtained from each subject. And the study methodologies have been approved by the ethical standards of Xiangya Hospital of Central South University (No. 201212074). All experiments conform to the Declaration of Helsinki.
RNA extraction, cDNA synthesis and qRT-PCR detection
Trizol (Invitrogen/Life Technologies, Carlsbad, CA) was used to extract RNA from muscle tissue according to the instructions. It was then reversed into cDNA using the Takara reverse transcription reagent (Takara, Japan, Code No. RR036A). The mRNA level of hub genes was detected via qRT-PCR with TB Green mixture (Takara, Japan, Code No. RR420A) by using the ABI7500 system (Applied Biosystems, Foster City, CA, USA). The relative expression levels of genes in each sample were calculated by using the housekeeping gene GAPDH. The primers used in the study are shown in Supplementary Table S2.
Immunohistochemistry of skin tissue
Skin samples from patients were collected with 4% paraformaldehyde. The expression of RSAD2 was detected by immunohistochemistry.
Statistical methods
All data were analyzed by GraphPad Prism 7.0 software and expressed as mean ± S.D. Student’s unpaired T test was used to determine the statistical difference between the two groups. When the sample data were not normally distributed, Mann-Whitney test (two-tail test) was used for statistical analysis. And a p value <0.05 was considered statistically significant.
Results
Identification of DEGs in GSE46239 and GSE193068
A total of 380 DEGs were identified in GSE46239 of DM patients, and 271 genes were up-regulated and other 109 genes were down-regulated (, ). Besides, a total of 159 up-regulated genes and 12 down-regulated genes were found in the GSE193068 dataset of COVID-19 patients (). And it was found that there were co-expressed differential genes in these two datasets (). The most significant pathways of these common genes are NOD-like receptor signaling way, viral protein interaction with cytokine and cytokine receptor, and coronavirus disease-COVID-19.
Figure 1. Volcano plots of datasets. (A) Volcano plots of GSE46239 in DM. The ordinate represents log2 (fold change), the abscissa represents log10 (p value), each point represents a gene, |log2 FC| ≥1and p < 0.05 as thresholds. (B) Volcano plots of GSE193068 in COVID-19. (C) The intersection of the two data sets. (D) KEGG pathway of these common genes.
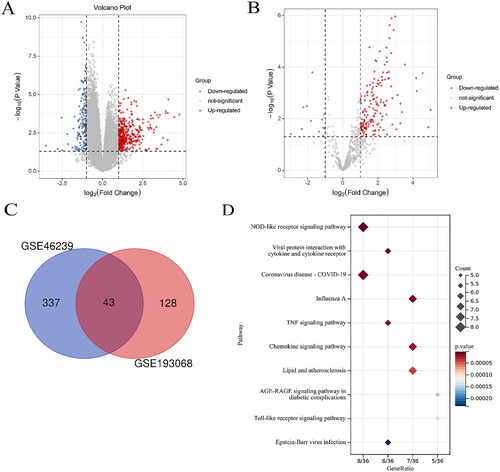
Gene term enrichment analysis recognized similar a signature of COVID-19 infection in patients with DM
To further explore potential functions of these DEGs in DM, we performed GO and pathway analyses on DM by using the criterion of p value <0.05. As shown in , it shows the top 10 significant terms for the BP, CC, MF and KEGG of DEGs in GSE46239. The DEGs were enriched for genes in the BP term involved in response to virus, response to interferon-gamma and type I interferon signaling pathway. In the MF term, the DEGs were enriched in receptor ligand activity, signaling receptor activator activity and glycosaminoglycan binding. In the CC term, the DEGs were enriched in collagen-containing extracellular matrix, external side of plasma membrane, and early endosome. The KEGG enrichment analysis showed that the most significant pathways were Coronavirus disease-COVID-19, influenza A and NOD-like receptor signaling pathway.
PPI networks and module analysis of 380 DEGs in GSE46239
All of the 380 DEGs (271 up-regulated genes and 109 down-regulated genes) were uploaded onto the online website STRING (http://string-db.org) and then they were analyzed by using the Cytoscape software. The PPI network contained 173 nodes and 829 edges, and 207 genes did not fall into the PPI network (). Then we selected the two significant modules by using the plug-in MCODE. Module 1 consisted of 25 nodes/genes and 296 edges (), which are mainly associated with cellular response to type I interferon signaling pathway (BP), double-stranded RNA binding (MF), postsynaptic endocytic zone (CC), and hepatitis C(KEGG) (, module1). Module 2 consisted of 23 nodes/genes and 117 edges (), which are mainly associated with cellular response to interferon-gamma (BP), immune receptor activity (MF), external side of plasma membrane (CC), and viral protein interaction with cytokine and cytokine receptor (KEGG) (, module 2).
Figure 3. Protein–protein interaction (PPI) network of differentially expressed genes (DEGs) in DM. A. Based on the STRING online database, 173 genes were filtered into the DEG PPI network. B. The most significant module 1 (25 genes) from the PPI network. C. The most significant module 2 (23 genes) from the PPI network.
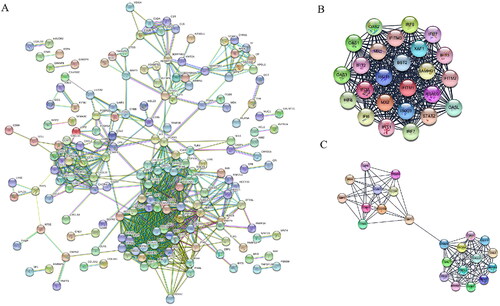
The identified hub genes in patients with DM participates in COVID-19 infection
In this part, we used the cytoscape plug-in cytoHubba to confirm the hub gene according to the latest analysis method MCC analysis method which is strongly recommended. The top 10 genes with the highest MCC scores are considered hub genes (, ). And the pathways enrichment of hub genes were associated with Coronavirus disease-COVID-19, influenza A and hepatitis C (, ).
Figure 5. Hub gene and KEGG pathway analysis in DM. A. Top 10 hub genes with highest MCC score in cytoHubba. B. KEGG pathway analysis of hub genes.
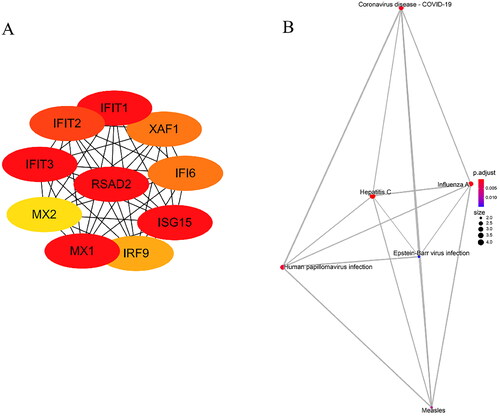
Table 2. 380 DEGs were identified in GSE46239, including 271 up-regulated genes and 109 down-regulated genes.
Table 3. Functional roles of top 10 hub genes with highest MCC score.
Hub gene expression in the muscle tissue of patients with IIM
24 muscle tissue samples of IIM were obtained. 10 hub genes were selected for analysis by qPCR in IIM muscle tissues. The expression of these hub genes in IIM muscle tissues was significantly higher than that in the NC group (p < 0.05, ).
RSAD2 is elevated in the skin of patients with DM
As we can see in , RSAD2 is at the core condition. Therefore, RSAD2 was selected for immunohistochemistry for validation in skin tissues of patients with dermatomyositis. It showed that RSAD2 expression was significantly increased in patients with dermatomyositis compared with normal controls (). It was mainly expressed in skin fibroblasts.
Discussions
Dermatomyositis is an inflammatory autoimmune disease, affecting skin, muscle, and blood vessels. The pathogenesis is complicated, involved in multiple factors, including genetic, epigenetic, and environmental factors [Citation28]. This study was expected to find key genes and signal pathway in the skin of DM. A total of 380 DEGs of GSE46239 were identified, consisting of 271 up-regulated genes and 109 down-regulated genes. And we found that the skin of DM patients and the skin of COVID-19 patients shared a common transcriptional profile. Then, we used R package cluster Profiler to perform GO and KEGG analysis for the DEGs of GSE46239. A PPI network of DEGs in GSE46239 was constructed and 173 genes and 829 edges, and two significant modules were chosen from the PPI network. Next, 10 hub genes were identified by using plug-in cytoHubba, namely MX1, ISG15, IFIT3, IFIT1, RSAD2, IFIT2, IFI6, XAF1, IRF9, MX2. Finally, we verified these genes in the muscle and skin tissue of patients with DM.
Interferon is a cytokine produced by monocytes and lymphocytes, which has the effects of anti-viral, anti-tumor, anti-infection and immune regulation. Interferon (IFN) family can be divided into three classes, consisting of type I IFN, type II IFN, and type III IFN [Citation29]. The etiology of DM is still unclear. However, type I IFN signal pathway is strongly associated with the pathogenesis of DM [Citation30–32]. DM patients showed an increased expression of the type I IFN-inducible genes in blood, muscle and skin [Citation33–36]. In addition, type I IFN-inducible genes were positively correlated with the disease activity of DM [Citation33, Citation35]. Type I IFN mainly includes IFNαand β, produced by macrophages and plasmacytoid dendritic cells (pDCs). IFN can activate JAK-STAT pathway by phosphorylation of STAT 1 and 2 via binding with the receptors [Citation29].
The involvement of RSAD2 in the pathogenesis of DM has not been reported. As an IFN-inducible gene, RSAD2 played an important role in other autoimmune diseases, such as systemic lupus erythematosus (SLE) [Citation37–39], systemic sclerosis (SSc) [Citation40, Citation41], and primary Sjogren syndrome (pSS) [Citation42, Citation43]. RSAD2 was overexpressed in CD19+ B cell of pSS and knockdown of RSAD2 can attenuate B cell hyperactivity through suppressing NF-κb signaling pathway [Citation42]. Besides, the expression of RSAD2 in fibroblasts was significantly increased after virus stimulation [Citation44]. In this study, we found that skin lesions in DM were closely related to the viral infection pathway and that RSAD2 was upregulated mainly in fibroblasts of DM skin. Taken together, RSAD2 may participate in the pathogenesis of DM by affecting fibroblasts in the skin of DM. In the peripheral circulation, RSAD2 may enhance the immune response and the production of autoantibodies by enhancing the reactivity of immune cells such as B cells in DM patients. This finding was interesting and the specific mechanism needs to be further investigated.
Recent studies suggest that the type I interferon pathway plays an important role in COVID-19 [Citation45–47]. Activation of the cGAS-STING pathway associated with type I interferon is involved in lung damage in COVID-19 patients [Citation17]. And type I interferon exacerbates inflammation in patients with severe COVID-19 [Citation48]. Our results suggest that the skin of patients with dermatomyositis and the skin of patients with COVID-19 have similar transcriptional profiles. And the DEGs in the skin of patients with dermatomyositis are closely related to type I interferon signaling pathway and COVID-19 signaling pathway. It shows that DM is as unfathomable as COVID-19, and the pathogenesis is similar but complex.
In summary, we found that patients with DM shared a common transcriptional profile with COVID-19. And we identified 380 DEGs and 10 hub-genes in DM, which were associated with type I IFN signaling pathway and COVID-19 closely. In-depth research on these hub-genes will help us better understand the pathogenesis of DM. These hub-genes might also become new targets for the diagnosis and treatment of DM.
Authors’ contributions
Yiying Yang conceived and designed the study, performed the experiments, analyzed the data and drafted this manuscript, as the first author. Hongjun Zhao, and Jie Song performed the experiments. Muyao Guo and Huali Zhang contributed to analysis of the data, and revising of the manuscript. All authors have read and approved the final manuscript.
Supplemental Material
Download Zip (28.1 KB)Data availability
The data used to support the findings of this study is available from GSE46239 and GSE193068.
Disclosure statement
No potential conflict of interest was reported by the authors.
Additional information
Funding
References
- Bottai M, Tjarnlund A, Santoni G, et al. EULAR/ACR classification criteria for adult and juvenile idiopathic inflammatory myopathies and their major subgroups: a methodology report. Rmd Open. 2017;3(2):1.
- Meyer A, Meyer N, Schaeffer M, et al. Incidence and prevalence of inflammatory myopathies: a systematic review. Rheumatology (Oxford). 2015;54(1):50–9.
- Furst DE, Amato AA, Iorga SR, et al. Epidemiology of adult idiopathic inflammatory myopathies in a U.S. managed care plan. Muscle Nerve. 2012;45(5):676–683.
- Muro Y, Sugiura K, Akiyama M. Cutaneous manifestations in dermatomyositis: key clinical and serological features-a comprehensive review. Clin Rev Allergy Immunol. 2016;51(3):293–302.
- Kao L, Chung L, Fiorentino DF. Pathogenesis of dermatomyositis: role of cytokines and interferon. Curr Rheumatol Rep. 2011;13(3):225–232.
- Gao S, Luo H, Zhang H, et al. Using multi-omics methods to understand dermatomyositis/polymyositis. Autoimmun Rev. 2017;16(10):1044–1048.
- Venalis P, Lundberg IE. Immune mechanisms in polymyositis and dermatomyositis and potential targets for therapy. Rheumatology (Oxford). 2014;53(3):397–405.
- Mammen AL. Dermatomyositis and polymyositis: clinical presentation, autoantibodies, and pathogenesis. Ann N Y Acad Sci. 2010;1184:134–153.
- Fernandez-Ruiz R, Niewold TB. Type I interferons in autoimmunity. J Invest Dermatol. 2022;142(3 Pt B):793–803.
- Bolko L, Jiang W, Tawara N, et al. The role of interferons type I, II and III in myositis: a review. Brain Pathol. 2021;31(3):e12955.
- Arshanapalli A, Shah M, Veerula V, et al. The role of type I interferons and other cytokines in dermatomyositis. Cytokine. 2015;73(2):319–325.
- Behan WM, Behan PO. The role of viral infection in polymyositis, dermatomyositis and chronic fatigue syndrome. Baillieres Clin Neurol. 1993;2(3):637–657.
- Peravali R, Acharya S, Raza SH, et al. Dermatomyositis developed after exposure to Epstein-Barr virus infection and antibiotics use. Am J Med Sci. 2020;360(4):402–405.
- Qian J, Xu H. COVID-19 disease and dermatomyositis: a mini-review. Front Immunol. 2021;12:747116.
- Gupta L, Lilleker JB, Agarwal V, et al. COVID-19 and myositis – unique challenges for patients. Rheumatology (Oxford). 2021;60(2):907–910.
- Tanboon J, Nishino I. COVID-19-associated myositis may be dermatomyositis. Muscle Nerve. 2021;63(1):E9–E10.
- Domizio JD, Gulen MF, Saidoune F, et al. The cGAS-STING pathway drives type I IFN immunopathology in COVID-19. Nature. 2022;603(7899):145–151.
- Ashburner M, Ball CA, Blake JA, et al. Gene ontology: tool for the unification of biology. The gene ontology consortium. Nat Genet. 2000;25(1):25–29.
- Ogata H, Goto S, Sato K, et al. KEGG: kyoto encyclopedia of genes and genomes. Nucleic Acids Res. 1999;27(1):29–34.
- Yu GC, Wang LG, Han YY, et al. clusterProfiler: an R package for comparing biological themes among gene clusters. OMICS. 2012;16(5):284–287.
- Franceschini A, Szklarczyk D, Frankild S, et al. STRING v9.1: protein-protein interaction networks, with increased coverage and integration. Nucleic Acids Res. 2013;41(Database issue):d808–15.
- Kohl M, Wiese S, Warscheid B. Cytoscape: software for visualization and analysis of biological networks. Methods Mol Biol. 2011;696:291–303.
- Smoot ME, Ono K, Ruscheinski J, et al. Cytoscape 2.8: new features for data integration and network visualization. Bioinformatics. 2011;27(3):431–432.
- Bader GD, Hogue CW. An automated method for finding molecular complexes in large protein interaction networks. BMC Bioinf. 2003;4:2.
- Chin CH, Chen SH, Wu HH, et al. cytoHubba: identifying hub objects and Sub-networks from complex interactome. BMC Syst Biol. 2014;8(Suppl 4):S11.
- Bohan A, Peter JB. Polymyositis and dermatomyositis (first of two parts). N Engl J Med. 1975;292(7):344–347.
- Bohan A, Peter JB. Polymyositis and dermatomyositis (second of two parts). N Engl J Med. 1975;292(8):403–407.
- Silva R-E, Pinto AP, Pirmez R, et al. Dermatomyositis–part 1: definition, epidemiology, etiology and pathogenesis, and clinics. Skinmed. 2016;14(4):273–279.
- Luo S, Wang Y, Zhao M, et al. The important roles of type I interferon and interferon-inducible genes in systemic lupus erythematosus. Int Immunopharmacol. 2016;40:542–549.
- Jiang J, Zhao M, Chang C, et al. Type I interferons in the pathogenesis and treatment of autoimmune diseases. Clin Rev Allergy Immunol. 2020;59(2):248–272.
- Gallay L, Mouchiroud G, Chazaud B. Interferon-signature in idiopathic inflammatory myopathies. Curr Opin Rheumatol. 2019;31(6):634–642.
- Pinal-Fernandez I, Casal-Dominguez M, Derfoul A, et al. Identification of distinctive interferon gene signatures in different types of myositis. Neurology. 2019;93(12):e1193–e204.
- Walsh RJ, Kong SW, Yao Y, et al. Type I interferon-inducible gene expression in blood is present and reflects disease activity in dermatomyositis and polymyositis. Arthritis Rheum. 2007;56(11):3784–3792.
- Greenberg SA, Pinkus JL, Pinkus GS, et al. Interferon-alpha/beta-mediated innate immune mechanisms in dermatomyositis. Ann Neurol. 2005;57(5):664–678.
- Baechler EC, Bauer JW, Slattery CA, et al. An interferon signature in the peripheral blood of dermatomyositis patients is associated with disease activity. Mol Med. 2007;13(1–2):59–68.
- Wong D, Kea B, Pesich R, et al. Interferon and biologic signatures in dermatomyositis skin: specificity and heterogeneity across diseases. PLoS One. 2012;7(1):e29161.
- Shen M, Duan C, Xie C, et al. Identification of key interferon-stimulated genes for indicating the condition of patients with systemic lupus erythematosus. Front Immunol. 2022;13:962393.
- Fang Q, Li T, Chen P, et al. Comparative analysis on abnormal methylome of differentially expressed genes and disease pathways in the immune cells of RA and SLE. Front Immunol. 2021;12:668007.
- Fan H, Zhao G, Ren D, et al. Gender differences of B cell signature related to estrogen-induced IFI44L/BAFF in systemic lupus erythematosus. Immunol Lett. 2017;181:71–78.
- Zheng JN, Li Y, Yan YM, et al. Identification and validation of key genes associated with systemic Sclerosis-Related pulmonary hypertension. Front Genet. 2020;11:816.
- Piera-Velazquez S, Mendoza FA, Addya S, et al. Increased expression of interferon regulated and antiviral response genes in CD31+/CD102+ lung microvascular endothelial cells from systemic sclerosis patients with end-stage interstitial lung disease. Clin Exp Rheumatol. 2021;39(6):1298–1306.
- Zhu H, Zheng J, Zhou Y, et al. Knockdown of RSAD2 attenuates B cell hyperactivity in patients with primary sjogren’s syndrome (pSS) via suppressing NF-kappab signaling pathway. Mol Cell Biochem. 2021;476(5):2029–2037.
- Maria NI, Steenwijk EC, IJpma AS, et al. Contrasting expression pattern of RNA-sensing receptors TLR7, RIG-I and MDA5 in interferon-positive and interferon-negative patients with primary Sjogren’s syndrome. Ann Rheum Dis. 2017;76(4):721–730.
- Tang J, Tang A, Du H, et al. Peste des petits ruminants virus exhibits Cell-Dependent interferon active response. Front Cell Infect Microbiol. 2022;12:874936.
- Acharya D, Liu G, Gack MU. Dysregulation of type I interferon responses in COVID-19. Nat Rev Immunol. 2020;20(7):397–398.
- Trouillet-Assant S, Viel S, Gaymard A, et al. Type I IFN immunoprofiling in COVID-19 patients. J Allergy Clin Immunol. 2020;146(1):206–208 e2.
- Zhang C, Feng YG, Tam C, et al. Transcriptional profiling and machine learning unveil a concordant biosignature of type I Interferon-Inducible host response across nasal swab and pulmonary tissue for COVID-19 diagnosis. Front Immunol. 2021;12:733171.
- Lee JS, Park S, Jeong HW, et al. Immunophenotyping of COVID-19 and influenza highlights the role of type I interferons in development of severe COVID-19. Sci. Immunol. 2020;5(49):eabd1554.