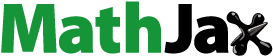
ABSTRACT
The number of molecules used in a typical Molecular Dynamics (MD) simulations is orders of magnitude lower than in the thermodynamic limit. It is therefore essential to correct diffusivities computed from Molecular Dynamics simulations for finite-size effects. We present a comprehensive review on finite-size effects of diffusion coefficients by considering self-, Maxwell–Stefan, and Fick diffusion coefficients in pure liquids, as well as binary, ternary, and quaternary mixtures. All finite-size corrections, both analytical and empirical, are discussed in detail. The finite-size effects of rotational and confined diffusion are also briefly discussed.
1. Introduction
Mass transport by diffusion plays a crucial role in a wide variety of processes in nature and engineering. Such processes are separation, drug and protein delivery in living organisms, fog formation, powder metallurgy, and many others [Citation1–5]. Diffusion is commonly described as the mass flux (i.e. transport) due to a gradient of concentration or chemical potential of a component [Citation6–8]. In liquids, diffusion is a relatively slow process and is often a limiting factor in engineering applications [Citation9–11]. Usually, the diffusion in engineering applications involves multicomponent liquid mixtures [Citation11,Citation12]. The accurate prediction of diffusivities of these mixtures is therefore very important [Citation13]. Self-diffusivity is the motion of individual molecules in a pure liquid or in a mixture due to random Brownian motion, i.e. in the absence of an external driving force [Citation14–16]. Technically, collective diffusivities are of greater interest in engineering applications. Collective diffusion describes the net transport of a component in a multicomponent mixture relative to a reference motion of another component. The mass flux in collective diffusion is described by a gradient in chemical potential due to differences in temperature, concentration, pressure and/or electric field [Citation11,Citation16,Citation17].
The framework for the systematic study of diffusion was established by the pioneering studies of Graham [Citation18] and Fick [Citation19] in the mid-19th century. Since then, experimental methods for measuring the diffusivity in liquids have significantly improved. Such methods include Nuclear Magnetic Resonance (NMR) [Citation20], Raman Micro-spectroscopy [Citation21], Dynamic Light Scattering (DLS) [Citation22,Citation23] and holographic interferometry [Citation24]. Despite this progress, experimental measurements are often challenging to perform. In many cases, experiments require special and costly equipment and can be very time consuming for mixtures with sluggish dynamics [Citation25–27]. Moreover, experimental measurements can be dangerous when poisonous or explosive compounds are studied, and when high temperatures and/or pressures are required [Citation28,Citation29]. The resolution of experiments is generally too low for obtaining insight at the molecular level [Citation30]. To this end, theoretical approaches based on the kinetic theory and hydrodynamics have been developed to predict diffusivities. Such approaches are the well-known Stokes–Einstein [Citation31] and Wilke–Chang [Citation32] models. Recently, Gross and co-workers [Citation33] proposed a model according to which the self-diffusion coefficients of pure liquids can be calculated from the scaling of the excess entropy, using the Perturbed-Chain Polar Statistical Associating Fluid Theory (PCP-SAFT) equation of state. Nevertheless, such semi-empirical correlations cannot be generalised for all fluids. A detailed discussion on theoretical approaches for obtaining diffusion can be found elsewhere [Citation3,Citation11,Citation12,Citation34,Citation35].
After more than half a century of practice, molecular simulation has been matured into a powerful tool for computing diffusion coefficients of pure fluids and mixtures, complementing experiments and models [Citation6,Citation10,Citation30,Citation36–55]. Diffusion coefficients can be computed by performing Molecular Dynamics (MD) or Kinetic Monte Carlo (KMC) simulations [Citation37,Citation38,Citation56]. The MD approach is by far the most widely used, and thus the focus of this review. In MD, diffusion coefficients can be computed either by using equilibrium (EMD) or non-equilibrium (NEMD) simulations [Citation57,Citation58]. In NEMD simulations, an external driving force is used to exert a net flux in the system from which the transport properties can be calculated [Citation36,Citation37,Citation59–62]. In EMD, diffusivities are computed in mixtures at equilibrium, i.e. in the absence of external forces. The Green-Kubo (GK) method and Einstein relations are two common approaches in EMD simulation. The GK method [Citation52,Citation53,Citation63–66] is based on the computation of integrals of velocity-time correlation functions, while the Einstein relations are based on the linear fit to mean-squared displacements as a function of time [Citation14,Citation67–69].
EMD simulations have been extensively used for computing self-diffusivities of fluid models, e.g. hard spheres and Lennard-Jones (LJ) particles [Citation70–74], as well of a wide variety of molecular fluids, e.g. water, carbon dioxide, hydrocarbons, ionic liquids, deep eutectic solvents, and biomolecules [Citation48,Citation75–85]. Many studies have focused on the computation of self-diffusivities in mixtures [Citation86–89]. EMD has also been widely used to predict collective diffusivity in binary, ternary, and multicomponent mixtures [Citation30,Citation49–52,Citation61,Citation90–98]. To describe collective diffusion in mixtures, the Maxwell–Stefan (MS) theory and Fick's law are the most commonly used approaches [Citation1,Citation11,Citation13,Citation17,Citation94]. According to the MS theory, a gradient in chemical potential is the driving force for mass transport [Citation94]. Since chemical potentials cannot be directly measured in experiments, the determination of MS diffusion coefficients is challenging [Citation13,Citation50,Citation94]. MS diffusivities can be computed in EMD simulations using so-called Onsager coefficients. Onsager coefficients allow for a phenomenological description of diffusion based on the Onsager theory of irreversible thermodynamics, which relates the velocity difference of the individual components to chemical potential gradients [Citation17,Citation94,Citation99,Citation100]. Contrary to MS diffusivities, Fick's diffusion [Citation17,Citation19] can be directly measured experimentally. Since MS and Fick describe the same physical phenomenon, these diffusivities are related. The conversion between Fick and MS diffusivities is enabled by the so-called matrix of thermodynamic factors, which is a measure of the non-ideality of the mixtures [Citation101,Citation102]. A way to obtain thermodynamic factors is via Kirkwood–Buff integrals (KBIs) [Citation103–107]. KBIs are expressed as either integrals over the radial distribution functions (RDFs) of a closed subvolume in canonical MD simulations, or as density fluctuations of an open and infinite system in grand-canonical simulations [Citation106,Citation108,Citation109]. For an in-depth discussion on KBIs as well as on self-, MS and Fick diffusivities, the reader is referred to the relevant literature [Citation6,Citation50,Citation94,Citation106,Citation107,Citation109–111].
A remaining challenge of MD simulations is the limited system size. The system sizes used in a typical MD simulation vary from a few hundred to several thousands of molecules. Periodic boundary conditions are traditionally imposed in all directions to mimic the bulk fluid phase and eliminate surface effects [Citation36–39]. Due to the long-range nature of hydrodynamic and electrostatic interactions between molecules in the simulation box and with the periodic images (because of periodic boundary conditions), dynamical properties may suffer from the finite-size effects [Citation37,Citation112,Citation113]. In particular, the computation of diffusivities [Citation74,Citation114–118], activity coefficients [Citation119], thermal conductivities [Citation120], ionic conductivities [Citation121], and KBIs [Citation122,Citation123] have been shown to depend on the system size. Other properties, such as the shear viscosity are not affected by the system size [Citation78,Citation124–126]. As Dünweg and Kremer [Citation114] explicitly state in their pioneering work on the dynamics of polymer chains, to avoid such finite-size effects one may consider performing simulations without periodic boundary conditions, e.g. using hard walls. Nevertheless, such a choice would simply introduce different effects which are possibly more difficult to control, e.g. unwanted fluid-wall interactions. It is important to note that finite-size effects are present in the computation of both self- [Citation78,Citation127] and collective diffusivities [Citation91,Citation117,Citation128], both in pure liquids and multicomponent mixtures. To mitigate finite-size effects, researchers have used relatively large system sizes, large cut-off radii in the interaction potentials, or analytic corrections [Citation51,Citation77,Citation115,Citation129,Citation130].
In this review paper, we present a comprehensive discussion of the finite-size dependence of self- and collective diffusion coefficients computed with EMD simulations for various molecular and LJ systems. Our study covers pure fluids, binary, ternary and quaternary mixtures. Finite-size effects for diffusivity computations in confined systems, and for rotational diffusion are also briefly discussed. The rest of the manuscript is organised as follows: In Section 2, the available MD codes for computing diffusion coefficients are presented. The finite-size dependence and available correction terms for self, rotational and collective diffusivities are discussed in Sections 3, 4 and 5, respectively. Finite-size effects of diffusion of confined liquids is also discussed in Section 3. Our main findings are summarised in Section 6.
2. Computational details and available software
The open-source MD packages LAMMPS [Citation131] and GROMACS [Citation132] are widely used for computing transport properties of liquids. One of the reasons for the popularity of these packages is the default built-in features and tools which can be used to calculate diffusion coefficients based on the Green-Kubo and Einstein methods [Citation36,Citation133]. The source codes of both LAMMPS and GROMACS are designed in a modular way which promotes the development of new features by the simulation community. Recently, a new open-source plugin for LAMMPS, called OCTP (On-the-fly Calculation of Transport Properties), has been developed by Jamali et al. [Citation134,Citation135]. OCTP uses Einstein relations combined with the order-n algorithm [Citation36,Citation136] to accurately compute the following properties: shear and bulk viscosities, radial distribution functions (RDFs), thermal conductivities, and self- and MS diffusivities. In addition, Humbert et al. [Citation137] has recently developed the open-source post-processing tool PyLAT (Python Analysis Tools) for LAMMPS which enables the computation of several transport properties such as viscosities, self-diffusivities, ionic conductivities, dielectric constants, and RDFs. Deublein et al. [Citation138] developed a molecular simulation program named ms2, to compute thermodynamic and transport properties of pure liquids and multicomponent mixtures based on classical MD and MC simulations. In ms2, static properties such as thermal and caloric properties, chemical potentials, vapour-liquid equilibria, Henry's law constant, and second virial coefficients, and dynamic properties such as self-diffusion coefficients, MS diffusivities, shear and bulk viscosities can be computed in different ensembles.
All diffusivities presented in this study are computed using the OCTP plugin in LAMMPS. A schematic representation of the workflow for computing self- and collective diffusivities is shown in Figure . The exact simulation scheme and force field details can be found in our previous studies [Citation117,Citation128].
3. Finite-size dependence of self-diffusivities
The study by Dünweg and Kremer [Citation114] was one of the first focusing on finite-size effects of dynamic properties computed in MD. The authors carried out MD simulations of a polymer chain diluted in a good solvent for different system sizes. It was shown that the computed diffusion coefficient of the chain scales linearly with the inverse of the simulation box length, L, which is proportional to (where N is the number of molecules). This means that the self-diffusivities of a molecule in the thermodynamic limit,
, can be obtained by linear extrapolating to
. Almost a decade later, Yeh and Hummer [Citation115] validated the findings of Dünweg and Kremer by performing MD simulations of LJ particles and TIP3P [Citation139] water. To obtain
, Yeh and Hummer [Citation115] derived an analytic finite-size correction term based on hydrodynamics [Citation115]. Although the same term has been already derived by Dünweg and Kremer [Citation114], it is commonly referred to as the Yeh–Hummer (YH) correction:
(1)
(1) where
is the finite self-diffusion coefficient computed in MD simulations, (
) is the Boltzmann constant, T is the absolute temperature,
is the shear viscosity computed in MD simulations, and
is a dimensionless constant equal to 2.837298 for periodic (cubic) lattices [Citation140,Citation141].
and
can be directly computed in EMD simulations using the Einstein relations [Citation36,Citation37,Citation134]:
(2)
(2) and
(3)
(3) where
is the position of the j-th molecule of species i at time t, and
is the number of molecules of species i in the system.
are the off-diagonal components of the stress tensor (i.e.
,
, and
), and V is the volume of the system. The angular brackets denote an ensemble average. It is important to note that
computed from EMD does not exhibit finite-size effects. [Citation78,Citation115,Citation124–126]
In addition to LJ fluids and water, the validity and applicability of the YH correction has been shown for various components, e.g. carbon dioxide, n-alkanes, and deep eutectic solvents [Citation30,Citation78,Citation130,Citation142]. The magnitude of the finite-size effects may significantly vary, depending on the system type and the thermodynamic conditions [Citation127,Citation128]. For example, as shown by Moultos et al. [Citation78], the YH correction needed to compensate for the finite-size effects of the computed diffusivity of CO at 323.15 K and 200 bar using 250 molecules is ca. 8%, a value which is comparable with the error in the calculation of the diffusivity of a finite-size system. In sharp contrast, for systems in which the solute size is significantly larger than the size of the solvent molecules, the YH correction can be even larger than the computed diffusivity. In particular, recently Erdös et al. [Citation143] computed the diffusivity of cyclodextrins in water and showed that the YH correction is ca. 30% to 75% of the final (corrected) self-diffusion coefficients (depending on T and P). Thus, in such systems, the use of finite-size corrections is imperative, even if relatively large system-sizes are used in MD simulations (i.e. 6000 molecules [Citation143]). Based on the YH correction (Equation (Equation1
(1)
(1) )), and by assuming that the self-diffusivity of a molecule, with effective hydrodynamic radius R, can be described using the Stokes–Einstein equation [Citation14,Citation31,Citation144]
(4)
(4) one can derive the following:
(5)
(5) Equation (Equation4
(4)
(4) ) shows that the finite-size effects of self-diffusivity depend on the size of the diffusing molecule with respect to the size of the simulation box. Hence, it is not surprising that finite-size effects in diffusivity computations were initially observed for polymers [Citation115].
In Figure , finite-size effects are shown for the self-diffusivity of several pure fluids and mixtures. Figure (a) shows the self-diffusion coefficient of SPC/E water as a function of the system size. The simulations were carried out at 298.15 K and 1.0 atm, considering six system sizes in the range of 512–8000 molecules. The self-diffusivity of SPC/E water in the thermodynamic limit deviates by 1.3% from the corrected diffusivity using the YH expression (Equation (Equation1(1)
(1) )). These results are in agreement with the self-diffusivity of SPC/E water reported in literature [Citation127,Citation142,Citation145]. The finite-size effects of the self-diffusivity in a binary LJ system are shown in Figure (b) and (c). For this system, dimensionless reduced units were considered where
,
and m are the LJ parameters, and the mass, respectively. The first component (LJ
) is used as the basis with
,
and
. The parameters of the second component (i.e. LJ
) are
,
and
. Equimolar binary mixtures for four system sizes (500, 1000, 2000 and 4000 particles) were considered. Simulations were performed at a reduced temperature of 0.65 and a reduced pressure of 0.05, corresponding to an average reduced density of 0.34. As in pure liquids, self-diffusion coefficients in binary mixtures vary proportionally with the inverse of the simulation box length. The difference between the corrected self-diffusion coefficients using the YH expression and extrapolated values to the thermodynamic limit is less than 1%. To test the validity of the YH correction for the self-diffusion coefficients in ternary molecular mixtures, MD simulations of the toluene/acetone/water mixture were carried out at 298.15 K and 1 atm. Results are shown in Figure (d–f). Four different system sizes consisting of 400–1500 molecules (in total) were simulated. The mole fractions of toluene, acetone and water are 0.10 0.65 and 0.25, respectively. The density of the system is 835 kg/m
, which agrees with the experimental density [Citation146]. The OPLS-AA force field was used for Toluene [Citation147] and Acetone [Citation148], and the SPC/E force field [Citation149] was used for water. As shown clearly in Figure , for both LJ fluids and molecular systems, the YH correction (Equation (Equation1
(1)
(1) )) can accurately predict the finite-size effects of self-diffusivity.
Figure 2. (Colour online) (a) Self-diffusion coefficients of pure water at 298.15 K and 1 atm for six system sizes consisting of 512–8000 molecules. (b) Self-diffusion coefficients of binary LJ mixtures at a reduced temperature of 0.65 and a reduced pressure of 0.05 for LJ, and (c) LJ
for four system sizes consisting of 500, 1000, 2000, and 4000 particles. (d) Self-diffusion coefficients of toluene, (e) acetone and (f) water in a ternary mixture of toluene-acetone-water at 298.15 K and 1 atm (
,
, and
) for 5 system sizes consisting of 400–1500 molecules. The uncorrected MD results are shown with red circles. Grey diamonds show the corrected self-diffusion coefficients using Equation (Equation1
(1)
(1) ). Blue dashed lines are the linear extrapolation of MD results to the thermodynamic limit and black dashed lines are the extrapolated values. The axes of subfigures scales differently.
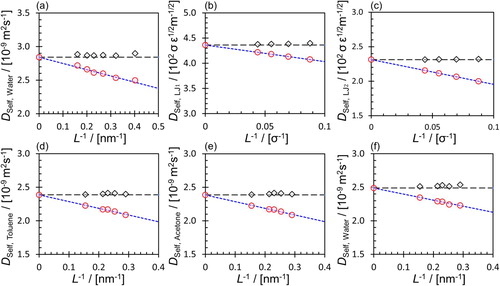
Recently, Jamali et al. [Citation126] proposed a method (called D-based) for computing shear viscosities of pure and multi-component fluids by exploiting the finite-size effects of self-diffusivities. In this method, a linear regression is performed to self-diffusivities computed for at least two different system sizes. The slope of the linear fit is proportional to the inverse of the shear viscosity because of the YH correction term (Equation (Equation1(1)
(1) )). Jamali et al. [Citation126] tested this method using 250 binary and 26 ternary LJ systems, pure water, and ionic liquid Bmim [Tf
N] ionic liquid. The obtained viscosities were shown to be in good agreement with the ones computed directly in EMD using the conventional methods (e.g. Einstein relations). For the exact details on the D-based method and guidelines for using it in the most efficient way, the reader is referred to the original paper [Citation126].
3.1. Finite-size dependence of self-diffusivities of fluids in confinement
The self-diffusivity of a liquid in a nanochannel may significantly deviate from the self-diffusivity in the bulk phase due to both the actual confinement effect (fluid-wall interactions) and the use of periodic boundary conditions [Citation116,Citation150]. It has been shown that the physical properties of liquids in confinement such as the pressure, density, viscosity and diffusivity can spatially vary (i.e. evidence of localised phenomena) [Citation151–161]. These local effects typically subside within a couple of molecular diameters away from the walls [Citation162–165]. This means that, as channel diameter increases, dynamic properties (i.e. viscosity, diffusivity) eventually become equal to the respective quantity in the bulk phase [Citation165–167]. Recently, Simonnin et al. [Citation116] investigated how the self-diffusion coefficient of a nanoconfined fluid is affected by the confining distance (H) and the simulation box length parallel to the wall (L). Simonnin et al. [Citation116] showed that the self-diffusion coefficient decreases as the simulation box length parallel to the wall (L) increases. This effect is evident due to the use of periodic boundary conditions. Furthermore, the diffusion coefficient increase with increasing confining distance. This is mainly due to the receding effect of wall-liquid interactions, as also discussed earlier in other studies [Citation168–171]. An analytic correction term for finite-size dependence in nanoconfined fluids was derived by Simonnin et al. [Citation116] using continuum hydrodynamics. This term accounts for the contribution of both L and H. The model was developed for an LJ fluid in a slit-like pore with no-slip condition, and the finite-size correction of such system was validated using MD simulations:
(6)
(6)
(7)
(7) where
and
are the self-diffusion coefficients for nanoconfined liquid in thermodynamic limit and at finite-size, respectively. These finite-size correction terms (Equations (Equation6
(6)
(6) ) and (Equation7
(7)
(7) )) are in good agreement with MD simulation results, except for very narrow channels [Citation116]. In narrow channels, the assumptions based on classical constitutive equations break down, e.g. Newton's law of viscosity. Consequently, continuum variables such as viscosity, diffusivity, thermal conductivity obtained from constitutive equations become inaccurate [Citation152,Citation161,Citation172].
4. Finite-size dependence of rotational diffusivities
Finite-size effects are also present in the computation of rotational diffusion [Citation173] in EMD. The accurate prediction of rotational diffusivity is mainly important for the design of bio-engineering applications, which involves relatively large and rather asymmetric molecules (e.g. DNA, RNA, carbohydrates). To this purpose, studies have investigated the scaling of the rotational diffusivity with the system size [Citation174–176]. Linke et al. [Citation177] performed MD simulations of B-DNA dodecamer and horse-heart myoglobin using various system sizes. Linke et al. [Citation177] developed an analytic correction term to account for the finite-size dependence of the computed rotational diffusion coefficients in three-dimensions (3-D):
(8)
(8) where
and
is the rotational diffusion coefficient in the thermodynamic limit and the one computed in MD simulations, respectively.
is the shear viscosity and V is the volume of the simulation box. A similar correction term for the finite-size effects of the rotational diffusivity of biological membrane proteins and carbon nanotubes was derived by Vögele et al. [Citation178] based on a two-dimensional (2-D) periodic Stokes flow:
(9)
(9) The difference between Equations (Equation8
(8)
(8) ) and (Equation9
(9)
(9) ) is the use of 2-D and 3-D flows for the correction of rotational diffusivities. Vögele et al. [Citation178] suggested that mean square displacement expressions for rotational diffusion in 2-D are much simpler compared to the same expressions in 3-D (i.e. use of quaternions). As can be seen from Equations (Equation1
(1)
(1) ), (Equation8
(8)
(8) ) and (Equation9
(9)
(9) ), the correction term for the translational diffusion contains the inverse of the simulation box length, while the finite-size correction term for rotational diffusion contains the inverse box volume. This indicates that the required correction of rotational diffusion is much smaller than the one for translational diffusion.
5. Collective diffusivities
Fick diffusion coefficients relate liquid fluxes to concentration gradients. The generalised Fick's law in an n-component system with respect to a molar reference frame equals [Citation11,Citation19]:
(10)
(10) where
is the diffusion flux,
is the total molar concentration,
is the mole fraction of component j, and
are the Fick diffusion coefficients. For an n-component system, the Fick diffusion matrix consists of
components.
The MS theory relates the gradient in chemical potentials to the liquid friction at a constant driving force [Citation17,Citation94]:
(11)
(11) where R is the universal gas constant,
is the chemical potential gradient of component i at constant pressure and temperature. (
) is the difference in average velocities between components i and j, which is proportional to the friction force at a constant driving force. Đ
ij are the MS diffusion coefficients, which are (unlike Fick diffusivities of
) symmetric, i.e. Đ
ij = Đ
ji. For an n-component system, there exist
MS diffusion coefficients. In a molar reference frame, the relation between Fick and MS diffusivities can be expressed by using the matrix of thermodynamic factors [
] and the phenomenological diffusivity matrix [
] [Citation17,Citation94]:
(12)
(12) where [Citation179]
(13)
(13) where
is the kronicker delta,
is the activity coefficient of component i. The
symbol is used to point out that partial differentiation of
with respect to
is performed at constant mole fractions of all other components except for the
-th component [Citation10].
The elements of can be obtained as follows:
(14)
(14) where
are the Onsager coefficients computed using EMD simulations (here shown using the Einstein formulation) [Citation90,Citation94,Citation99,Citation100]:
(15)
(15) where
and
are the number of molecules of species i and j, respectively.
is the total number of molecules in the system, and
is the position of i-th molecule of species j at time t. Onsager coefficients can be transformed into MS diffusivities using the [
] matrix. The [
] matrix is the inverse of [
]:
(16)
(16) MS diffusion coefficients is related to the elements of [
] matrix by
(17)
(17)
(18)
(18) The resulting expressions for binary, ternary, quaternary systems can be found in [Citation6,Citation49,Citation90]. For ideal diffusing systems, MS diffusivities can be related to self-diffusivities [Citation84], but in general this is not the case due to velocity cross-correlations.
5.1. Finite-size dependence of collective diffusivities
5.1.1. Binary mixtures
Jamali et al. [Citation128] performed an in-depth investigation of finite-size effects of MS diffusivities in binary mixtures. These authors performed EMD simulations of 200 LJ and 9 molecular mixtures and showed that, similarly to self-diffusivities, MS-diffusivities scale linearly with the system size. On the basis of the YH-correction (Equation (Equation1(1)
(1) )), and by taking into account the non-ideality of the mixtures, Jamali et al. [Citation128] developed a phenomenological correction term which should be added to the computed MS diffusivity,
, to obtain the property in the thermodynamic limit, Đ
:
(19)
(19) where
is the thermodynamic factor (which can be obtained from MD simulations using KBIs [Citation103,Citation106]). As can be seen from Equation (Equation19
(19)
(19) ), the magnitude of the required finite-size correction for MS diffusivities depends on the thermodynamic factor. This means that for non-ideal mixtures close to demixing (
) the correction term becomes very large.
For binary mixtures, there is single MS and Fick diffusion coefficient defined. They are related to each other by
(20)
(20) As shown in the work by Jamali et al. [Citation128], the finite-size correction for Fick diffusivities in binary mixtures can be derived by combining Equations (Equation19
(19)
(19) ) and (Equation20
(20)
(20) ) [Citation128]:
(21)
(21) Thus, the YH correction applies to the Fick diffusivities. This finding seems reasonable since the YH correction is a hydrodynamic effect and the Fick diffusivities appear in the hydrodynamic equations.
In Figure , the finite-size effects of the MS and Fick diffusivities are shown for the binary mixture methanol/methylamine and a binary LJ system. MD simulations were performed for equimolar methanol/methylamine mixtures consisting of four different system sizes (i.e. 250–2000 molecules) at 298 K and 1 atm. Force field parameters for methanol [Citation180] and methylamine [Citation181,Citation182] were obtained from the Transferable Potential for Phase Equilibria (TraPPE) force field. For the LJ system, simulations were performed at a reduced temperature of 0.65 and a reduced pressure of 0.05. The mole fractions of species 1 and 2 were chosen as 0.3 and 0.7, respectively. This LJ system corresponds to an average reduced density of 0.22. The LJ system involves prominent dissimilarities in size ( equal to 1.6), interaction energy (
equal to 0.6), and mass (
equal to 4.096). For all other simulation and force field details, the reader is referred to Ref. [Citation128]. Analytic finite-size corrections for MS and Fick diffusion coefficients are computed using Equations (Equation19
(19)
(19) ) and (Equation21
(21)
(21) ), respectively. The analytic corrections are compared with the linear regression of the MD results to the thermodynamic limit. As can be seen in Figure , the proposed correction accurately accounts for the finite-size effects of MS and Fick diffusion coefficients in binary molecular and LJ systems. Maximum deviations of 2.1% and 3.3% were observed from the extrapolated values in binary molecular and LJ systems, respectively.
Figure 3. (Colour online) (a) MS and (b) Fick diffusion coefficients of a binary equimolar methanol–methylamine mixture at 298 K and 1 atm as a function of the simulation box length (L). (c) MS and (d) Fick diffusion coefficients of binary LJ mixtures ( and
) at a reduced temperature of 0.65 and a reduced pressure of 0.05 as a function of the simulation box length (L). The uncorrected MD results are shown with red circles. Blue dashed lines are the linear extrapolation of the MD results to the thermodynamic limit. Grey diamonds show the corrected MS and Fick diffusion coefficients using Equations (Equation19
(19)
(19) ) and (Equation21
(21)
(21) ), respectively. Black dashed lines are the corrected diffusion coefficients computed from the linear extrapolation of the Onsager coefficients of the smallest sizes (
and
) to the thermodynamic limit. For LJ systems, simulations were performed for four system sizes consisting of 250, 500, 1000, and 2000 particles. For the molecular mixture, four system sizes consisting of 500, 1000, 2000, and 4000 particles were considered. The results are based on the study in [Citation128]. The axes of subfigures scales differently.
![Figure 3. (Colour online) (a) MS and (b) Fick diffusion coefficients of a binary equimolar methanol–methylamine mixture at 298 K and 1 atm as a function of the simulation box length (L). (c) MS and (d) Fick diffusion coefficients of binary LJ mixtures (x1=0.3 and x2=0.7) at a reduced temperature of 0.65 and a reduced pressure of 0.05 as a function of the simulation box length (L). The uncorrected MD results are shown with red circles. Blue dashed lines are the linear extrapolation of the MD results to the thermodynamic limit. Grey diamonds show the corrected MS and Fick diffusion coefficients using Equations (Equation19(19) ð∞=ðMD+1ΓkBTξ6πηL=ðMD+1ΓDYH(19) ) and (Equation21(21) D∞=DMD+DYH(21) ), respectively. Black dashed lines are the corrected diffusion coefficients computed from the linear extrapolation of the Onsager coefficients of the smallest sizes (NMolecular=250 and NLJ=500) to the thermodynamic limit. For LJ systems, simulations were performed for four system sizes consisting of 250, 500, 1000, and 2000 particles. For the molecular mixture, four system sizes consisting of 500, 1000, 2000, and 4000 particles were considered. The results are based on the study in [Citation128]. The axes of subfigures scales differently.](/cms/asset/2abf98e0-a1b9-4f66-87d0-79d580c3d767/gmos_a_1810685_f0003_oc.jpg)
5.1.2. Ternary mixtures
Very recently, Jamali et al. [Citation117] derived the generalised form for finite-size corrections of collective diffusion of multicomponent mixtures. By analysing the eigenvalues and eigenvectors of the Fick diffusion matrix, it was shown that only eigenvalues, which describe the speed of diffusion, are system-size dependent. The eigenvectors, which describe the direction of the diffusion process, do not depend on the size of the simulation box. Thus, the off-diagonal components of the Fick diffusion matrix exhibit no finite-size effects, while the diagonal components are system size dependent and can be corrected using the YH correction term. The generalised expression for finite-size corrections of the Fick diffusivity matrix is [Citation117]:
(22)
(22) where [I] is the identity matrix. By combining Equations (Equation12
(12)
(12) ) and (Equation22
(22)
(22) ), one can obtain the generalised expression for finite-size corrections of the
matrix:
(23)
(23) in which MS diffusivities are computed using Equations (Equation16
(16)
(16) )–(Equation18
(18)
(18) ).
The validity of the generalised correction terms shown in Equations (Equation22(22)
(22) ) and (Equation23
(23)
(23) ) was tested in the study by Jamali et al. [Citation117] using a ternary mixture of chloroform/acetone/methanol, and 28 different ternary LJ liquids. In Figures and , we show the computed Fick and MS diffusion coefficients of the ternary molecular mixture of chloroform (
), acetone (
) and methanol (
). MD simulations were carried out at 298 K and 1 atm, which correspond to a system with an average density of 1025 kg/m
. Diffusion coefficients were computed for four system sizes containing 500, 1000, 2000 and 4000 molecules. The OPLS force field was used to model chloroform, acetone and methanol [Citation183]. All simulation details and the force field parameters can be found in [Citation117]. As postulated in Equation (Equation22
(22)
(22) ), the off-diagonal components of the Fick diffusivity matrix do not depend on the system size (see Figure ). In sharp contrast, the diagonal components have a pronounced finite-size dependence, which can be accurately corrected using the YH correction (Equation (Equation1
(1)
(1) )). As shown in Figure , unlike Fick diffusivities, all MS diffusion coefficients depend on the system size and can be corrected using Equation (Equation23
(23)
(23) ). The maximum difference observed between the diffusion coefficients obtained via the analytic correction and the linear extrapolation to the infinite system size was 1.5%. This difference is rather small considering that the error in the computed diffusivities is not higher than 5%.
Figure 4. (Colour online) Fick diffusion coefficients of a ternary molecular mixture (molar reference frame) of (1) chloroform (2) acetone, and (3) methanol ( and
) at 298 K and 1 atm as a function of the simulation box length (L). (a) Diagonal component
, (b) Off-diagonal component
, (c) Off-diagonal component
, and (d) Diagonal component
. The uncorrected MD results are shown with red circles. Blue dashed lines are the linear extrapolation of the MD results to the thermodynamic limit. Grey diamonds show the corrected Fick diffusion coefficients using Equation (Equation22
(22)
(22) ). Black dashed lines are the corrected Fick diffusion coefficients computed from the linear extrapolation of the Onsager coefficients of the smallest size (N=500) to the thermodynamic limit. Simulations were performed for four system sizes consisting of 500, 1000, 2000, and 4000 particles. The results are based on the study in [Citation128]. The axes of subfigures scales differently.
![Figure 4. (Colour online) Fick diffusion coefficients of a ternary molecular mixture (molar reference frame) of (1) chloroform (2) acetone, and (3) methanol (xchloroform=xacetone=0.3 and xmethanol=0.4) at 298 K and 1 atm as a function of the simulation box length (L). (a) Diagonal component D1,1, (b) Off-diagonal component D1,2, (c) Off-diagonal component D2,1, and (d) Diagonal component D2,2. The uncorrected MD results are shown with red circles. Blue dashed lines are the linear extrapolation of the MD results to the thermodynamic limit. Grey diamonds show the corrected Fick diffusion coefficients using Equation (Equation22(22) [D∞]=[DMD]+DYH[I](22) ). Black dashed lines are the corrected Fick diffusion coefficients computed from the linear extrapolation of the Onsager coefficients of the smallest size (N=500) to the thermodynamic limit. Simulations were performed for four system sizes consisting of 500, 1000, 2000, and 4000 particles. The results are based on the study in [Citation128]. The axes of subfigures scales differently.](/cms/asset/b4e03ece-2453-4332-8f84-4b1417d1f2e5/gmos_a_1810685_f0004_oc.jpg)
Figure 5. (Colour online) MS diffusion coefficients of a ternary molecular mixture of (1) chloroform (2) acetone, and (3) methanol (, and
) at 298 K and 1 atm as a function of the simulation box length (L). (a)
, (b)
, and (c)
. The uncorrected MD results are shown with red circles. Blue dashed lines are the linear extrapolation of the MD results to the thermodynamic limit. Grey diamonds show the corrected MS diffusion coefficients based on Equation (Equation23
(23)
(23) ). Black dashed lines are the corrected MS diffusion coefficients computed from the linear extrapolation of the Onsager coefficients of the smallest size (N=500) to the thermodynamic limit. Simulations were performed for four system sizes consisting of 500, 1000, 2000, and 4000 particles. The results are based on the study in [Citation117]. The axes of subfigures scales differently.
![Figure 5. (Colour online) MS diffusion coefficients of a ternary molecular mixture of (1) chloroform (2) acetone, and (3) methanol (xchloroform=xacetone=0.3, and xmethanol=0.4) at 298 K and 1 atm as a function of the simulation box length (L). (a) ð1,2, (b) ð1,3, and (c) ð2,3. The uncorrected MD results are shown with red circles. Blue dashed lines are the linear extrapolation of the MD results to the thermodynamic limit. Grey diamonds show the corrected MS diffusion coefficients based on Equation (Equation23(23) [Δ∞]=[ΔMD]+DYH[Γ]−1(23) ). Black dashed lines are the corrected MS diffusion coefficients computed from the linear extrapolation of the Onsager coefficients of the smallest size (N=500) to the thermodynamic limit. Simulations were performed for four system sizes consisting of 500, 1000, 2000, and 4000 particles. The results are based on the study in [Citation117]. The axes of subfigures scales differently.](/cms/asset/2dfbf1dc-3e02-4390-85a6-84367677c595/gmos_a_1810685_f0005_oc.jpg)
To further investigate the finite-size effects of collective diffusivities in ternary mixtures, an LJ system at a reduced temperature of 0.65 and reduced pressure of 0.05 was simulated. The σ and m parameters for all species are chosen to be equal. To induce dissimilarities in the system, the ε parameters were varied (i.e. ,
, and
), and adjustable parameters (
) were applied to the Lorentz–Berthelot mixing rules (i.e.
,
, and
) [Citation37]. The mole fractions are 0.4, 0.3 and 0.3 for LJ species 1, 2 and 3, respectively. Four system sizes, ranging from 500 to 4000 molecules, were considered. All other simulation details and force field parameters can be found in [Citation117]. Figures and illustrate Fick and MS diffusivities, respectively, for this LJ system. In-line with the results of the molecular mixture, corrected diffusion coefficients are in a good agreement with the diffusivities obtained from the linear extrapolation to the infinite system size. The deviation in finite-size corrected diffusivities between two approaches is up to 2.5%.
Figure 6. (Colour online) Fick diffusion coefficients of a ternary LJ mixture (molar reference frame) ( and
) at a reduced temperature of 0.65 and a reduced pressure of 0.05 as a function of the simulation box length (L). (a) Diagonal component
, (b) Off-diagonal component
, (c) Off-diagonal component
, and (d) Diagonal component
. The uncorrected MD results are shown with red circles. Blue dashed lines are the linear extrapolation of the MD results to the thermodynamic limit. Grey diamonds show the corrected Fick diffusion coefficients using Equation (Equation22
(22)
(22) ). Black dashed lines are the corrected Fick diffusion coefficients computed from the linear extrapolation of the Onsager coefficients of the smallest size (N=500) to the thermodynamic limit. Simulations were performed for four system sizes consisting of 500, 1000, 2000, and 4000 particles. The results are based on the study in [Citation117]. The axes of subfigures scale differently.
![Figure 6. (Colour online) Fick diffusion coefficients of a ternary LJ mixture (molar reference frame) (x1=0.4 and x2=x3=0.3) at a reduced temperature of 0.65 and a reduced pressure of 0.05 as a function of the simulation box length (L). (a) Diagonal component D1,1, (b) Off-diagonal component D1,2, (c) Off-diagonal component D2,1, and (d) Diagonal component D2,2. The uncorrected MD results are shown with red circles. Blue dashed lines are the linear extrapolation of the MD results to the thermodynamic limit. Grey diamonds show the corrected Fick diffusion coefficients using Equation (Equation22(22) [D∞]=[DMD]+DYH[I](22) ). Black dashed lines are the corrected Fick diffusion coefficients computed from the linear extrapolation of the Onsager coefficients of the smallest size (N=500) to the thermodynamic limit. Simulations were performed for four system sizes consisting of 500, 1000, 2000, and 4000 particles. The results are based on the study in [Citation117]. The axes of subfigures scale differently.](/cms/asset/d0e98222-bd4f-4773-bed0-dc2a85678c4c/gmos_a_1810685_f0006_oc.jpg)
Figure 7. (Colour online) MS diffusion coefficients of a ternary LJ mixture ( and
) at a reduced temperature of 0.65 and a reduced pressure of 0.05 as a function of the simulation box length (L). (a)
, (b)
, and (c)
. The uncorrected MD results are shown with red circles. Blue dashed lines are the linear extrapolation of the MD results to the thermodynamic limit. Grey diamonds show the corrected MS diffusion coefficients based on Equation (Equation23
(23)
(23) ). Black dashed lines are the corrected MS diffusion coefficients computed from the linear extrapolation of the Onsager coefficients of the smallest size (N=500) to the thermodynamic limit. Simulations were performed for four system sizes consisting of 500, 1000, 2000, and 4000 particles. The results are based on the study in [Citation117]. The axes of subfigures scales differently.
![Figure 7. (Colour online) MS diffusion coefficients of a ternary LJ mixture (x1=0.4 and x2=x3=0.3) at a reduced temperature of 0.65 and a reduced pressure of 0.05 as a function of the simulation box length (L). (a) ð1,2, (b) ð1,3, and (c) ð2,3. The uncorrected MD results are shown with red circles. Blue dashed lines are the linear extrapolation of the MD results to the thermodynamic limit. Grey diamonds show the corrected MS diffusion coefficients based on Equation (Equation23(23) [Δ∞]=[ΔMD]+DYH[Γ]−1(23) ). Black dashed lines are the corrected MS diffusion coefficients computed from the linear extrapolation of the Onsager coefficients of the smallest size (N=500) to the thermodynamic limit. Simulations were performed for four system sizes consisting of 500, 1000, 2000, and 4000 particles. The results are based on the study in [Citation117]. The axes of subfigures scales differently.](/cms/asset/8b22f683-b90f-45e8-a867-29658fb13214/gmos_a_1810685_f0007_oc.jpg)
As proposed by Vrabec and co-workers [Citation91], the finite-size corrected MS and Fick diffusivities can also be obtained by extrapolating the Onsager coefficients to the thermodynamic limit, i.e. by linear regression in a plot with 1/L. Figure shows the Onsager coefficients for the ternary LJ system computed in this study ( and
). It is important to note that this approach requires multiple simulations at various system sizes and that a linear extrapolation typically introduces uncertainties. Recently, Vrabec and co-workers [Citation91] investigated the finite-size effects of collective diffusion coefficients of water/methanol/ethanol/2-propanol mixture. The required phenomenological coefficient (
) and thermodynamic factor (
) matrices for Fick diffusivities were obtained from EMD simulations. A finite-size correction for Fick diffusion coefficients was proposed based on the linear extrapolation of the diagonal elements of the Onsager coefficient matrix to the thermodynamic limit. Vrabec and co-workers [Citation91] suggested that off-diagonal Onsager coefficients do not depend on the system size for their relatively large systems (6000 molecules). In the binary, ternary, and quaternary systems studied here, both diagonal and non-diagonal elements of the computed Onsager coefficients show finite-size effects. In Figure , an example of the finite-size dependency of the Onsager coefficients for the ternary LJ system (
and
) is shown. MS and Fick diffusion coefficients computed from the extrapolation of Onsager coefficients in thermodynamic limit show a good agreement with the analytic corrections, and also with the linear extrapolation of the Fick and MS diffusion coefficients (dashed lines in Figures ). Using both the diagonal and off-diagonal elements of Onsager coefficients is therefore crucial, particularly when relatively small systems are considered. For large systems, the influence of the off-diagonal elements can be relatively small if the required finite-size correction is small.
Figure 8. (Colour online) Onsager coefficients of a ternary LJ mixture ( and
) at a reduced temperature of 0.65 and a reduced pressure of 0.05 as a function of the simulation box length (L). (a)
, (b)
, (c)
, (d)
, (e)
, and (f)
. The finite-size MD results are shown with red circles. Blue dashed lines are the linear fits to the MD results. Simulations were performed for four system sizes consisting of 500, 1000, 2000, and 4000 particles. The results are based on the study in [Citation117]. The axes of subfigures scale differently.
![Figure 8. (Colour online) Onsager coefficients of a ternary LJ mixture (x1=0.4 and x2=x3=0.3) at a reduced temperature of 0.65 and a reduced pressure of 0.05 as a function of the simulation box length (L). (a) Λ11, (b) Λ22, (c) Λ33, (d) Λ12, (e) Λ13, and (f) Λ23. The finite-size MD results are shown with red circles. Blue dashed lines are the linear fits to the MD results. Simulations were performed for four system sizes consisting of 500, 1000, 2000, and 4000 particles. The results are based on the study in [Citation117]. The axes of subfigures scale differently.](/cms/asset/56ffaad5-bc38-4d56-be2e-926ad8a0ee20/gmos_a_1810685_f0008_oc.jpg)
5.1.3. Quaternary mixtures
The generalised finite-size correction for collective diffusion (Equations (Equation22(22)
(22) ) and (Equation23
(23)
(23) )) derived by Jamali et al. [Citation117] has not yet been explicitly verified for systems containing more than three components, e.g. quaternary mixtures. Here, we performed MD simulations for an equimolar 4-component LJ colour mixture at a reduced temperature of 2.0 and a reduced pressure of 3.4, which corresponds to a reduced density of 0.72. Six different system sizes were simulated, i.e. 400, 800, 1200, 1600, 3200 and 4800 particles. The σ and ε LJ parameters for all species are chosen to be equal, so the mixture is ideal, i.e. [
and
. This means that the matrix of thermodynamic factor reduces to the identity matrix. To induce a physical dissimilarity, the masses of the particles are varied as follows:
,
,
, and
. In Figures and , the Fick and MS diffusivities of the quaternary system are shown as a function of the system size, respectively. Figure shows that the off-diagonal elements of the Fick diffusion matrix are size-independent, while the diagonal elements vary proportionally with the inverse of the simulation box length. In-line with our findings for the ternary mixtures, finite-size effects of the diagonal elements of the Fick diffusion matrix can be accurately corrected using the YH expression (Equation (Equation1
(1)
(1) )). Figure shows that all MS diffusion coefficients are size-dependent. The finite-size correction for MS diffusivities is only a function of the YH term because the matrix of thermodynamic factors is equal to the identity matrix (see Equation (Equation23
(23)
(23) )). Deviations between MS diffusivities corrected using the analytic correction and the ones obtained by linear extrapolation to the thermodynamic limit are in the range of 0 to 4.5%.
Figure 9. (Colour online) Fick diffusion coefficients of an equimolar quaternary LJ mixture (molar reference frame) at a reduced temperature of 2 and a reduced pressure of 3.4 as a function of the simulation box length (L). (a) Diagonal component , (b) Off-diagonal component
, (c) Off-diagonal component
, (d) Off-diagonal component
, (e) Diagonal component
, (f) Off-diagonal component
, (g) Off-diagonal component
, (h) Off-diagonal component
, and (i) Diagonal component
. The uncorrected MD results are shown with red circles. Grey diamonds show the corrected Fick diffusion coefficients using Equation (Equation22
(22)
(22) ). Blue dashed lines are the linear extrapolation of the MD results to the thermodynamic limit and Black dashed lines are the extrapolated values. Simulations were performed for six system sizes consisting of 400, 800, 1200, 1600, 3200, and 4800 particles. The axes of subfigures scales differently.
![Figure 9. (Colour online) Fick diffusion coefficients of an equimolar quaternary LJ mixture (molar reference frame) at a reduced temperature of 2 and a reduced pressure of 3.4 as a function of the simulation box length (L). (a) Diagonal component D1,1, (b) Off-diagonal component D1,2, (c) Off-diagonal component D1,3, (d) Off-diagonal component D2,1, (e) Diagonal component D2,2, (f) Off-diagonal component D2,3, (g) Off-diagonal component D3,1, (h) Off-diagonal component D3,2, and (i) Diagonal component D3,3. The uncorrected MD results are shown with red circles. Grey diamonds show the corrected Fick diffusion coefficients using Equation (Equation22(22) [D∞]=[DMD]+DYH[I](22) ). Blue dashed lines are the linear extrapolation of the MD results to the thermodynamic limit and Black dashed lines are the extrapolated values. Simulations were performed for six system sizes consisting of 400, 800, 1200, 1600, 3200, and 4800 particles. The axes of subfigures scales differently.](/cms/asset/0625d46d-d70b-4d21-a2f2-8c6cccdbdcd9/gmos_a_1810685_f0009_oc.jpg)
Figure 10. (Colour online) MS diffusion coefficients of an equimolar quaternary LJ mixture at a reduced temperature of 2 and a reduced pressure of 3.4 as a function of the simulation box length (L).(a) Ð1,2, (b) Ð1,3, (c) Ð1,4, (d) Ð2,3, (e) Ð2,4, and (f) Ð3,4. The uncorrected MD results are shown with red circles. Grey diamonds show the corrected MS diffusion coefficients using Equation (Equation22(22)
(22) ). Blue dashed lines are the linear extrapolation of the MD results to the thermodynamic limit and black dashed lines are the extrapolated values. Simulations were performed for six system sizes consisting of 400, 800, 1200, 1600, 3200, and 4800 particles. The axes of subfigures scales differently.
![Figure 10. (Colour online) MS diffusion coefficients of an equimolar quaternary LJ mixture at a reduced temperature of 2 and a reduced pressure of 3.4 as a function of the simulation box length (L).(a) Ð1,2, (b) Ð1,3, (c) Ð1,4, (d) Ð2,3, (e) Ð2,4, and (f) Ð3,4. The uncorrected MD results are shown with red circles. Grey diamonds show the corrected MS diffusion coefficients using Equation (Equation22(22) [D∞]=[DMD]+DYH[I](22) ). Blue dashed lines are the linear extrapolation of the MD results to the thermodynamic limit and black dashed lines are the extrapolated values. Simulations were performed for six system sizes consisting of 400, 800, 1200, 1600, 3200, and 4800 particles. The axes of subfigures scales differently.](/cms/asset/7502c4e8-a399-4b19-8b3c-0410f1104d6f/gmos_a_1810685_f0010_oc.jpg)
6. Conclusions
This review presents an in-depth discussion of the finite-size effects of diffusion coefficients computed with molecular dynamics simulations. The finite-size dependencies of self- and collective diffusivities in pure liquids, binary, ternary and quaternary mixtures are illustrated by considering various molecular and LJ systems. The effects of system size on the computation of diffusivities of confined liquids and rotational diffusivities are also briefly discussed. For all cases, we discuss the possible ways for obtaining the diffusivity values in the thermodynamics limit. It is shown that the self-diffusion coefficients of pure liquids and multicomponent mixtures scale linearly with the inverse of the simulation box length. A way to obtain self-diffusion coefficients in the thermodynamic limit is by linear extrapolation of the MD (finite-size) results to . The disadvantages of this approach are that it requires multiple long MD simulations of various system sizes, and that linear regression introduces uncertainties. Instead, an analytic correction term proposed by Yeh and Hummer (Equation (Equation1
(1)
(1) )) presents an alternative approach. The YH correction requires the shear viscosity of the fluid obtained from the conventional MD simulations (i.e. GK method, Einstein relations). Here, the YH correction for self-diffusion coefficient is illustrated for various molecular and LJ systems, i.e. SPC/E water, binary equimolar LJ, and toluene-acetone-water mixtures. The generalised form for finite-size corrections of MS and Fick diffusivities by Jamali et al. [Citation117] is also discussed. For collective diffusion, our main conclusions are as follows: For multicomponent mixtures (n > 2), the off-diagonal elements of the Fick diffusivity matrix do not depend on the system-size, while the diagonal elements show a system-size dependency which can be corrected using the YH term. In sharp contrast to Fick diffusivities, all MS diffusion coefficients are system-size dependent. The required correction for MS diffusivities can be considered as a modification of the YH expression, which includes the matrix of thermodynamic factors. The validity of the generalised correction model for collective diffusivities is illustrated for binary (i.e. a molecular methanol-methylamine and a LJ mixture), ternary (i.e. a molecular chloroform-acetone-methanol and a LJ mixture) and quaternary (i.e. a LJ colour mixture) systems.
Disclosure statement
No potential conflict of interest was reported by the author(s).
Additional information
Funding
References
- Cussler EL. Diffusion: mass transfer in fluid systems. 3rd ed. Cambridge: Cambridge University Press; 2009.
- Basu S, Kumar N. Modelling and simulation of diffusive processes. 1st ed. Cham: Springer; 2016.
- Tyrrell HJV, Harris K. Diffusion in liquids: a theoretical and experimental study. 1st ed. Oxford: Butterworth-Heinemann; 1984.
- Taylor R, Krishna R. Modelling reactive distillation. Chem Eng Sci. 2000;55:5183–5229.
- Wilson ID. Encyclopedia of separation science. 1st ed. San Diego (CA): Elsevier; 2000.
- Liu X, Martín-Calvo A, McGarrity E, et al. Fick diffusion coefficients in ternary liquid systems from equilibrium molecular dynamics simulations. Ind Eng Chem Res. 2012;51:10247–10258.
- Wesselingh J, Krishna R. Mass transfer in multicomponent mixtures. 1st ed. Delft: Delft University Press; 2000.
- Bird RB. Transport phenomena. Appl Mech Rev. 2002;55:R1–R4.
- Lyons WC, Plisga GJ. Standard handbook of petroleum and natural gas engineering. 3rd ed. Oxford: Elsevier; 2015.
- Liu X, Schnell SK, Simon J-M, et al. Fick diffusion coefficients of liquid mixtures directly obtained from equilibrium molecular dynamics. J Phys Chem B. 2011;115:12921–12929.
- Taylor R, Krishna R. Multicomponent mass transfer. 1st ed. Vol. 2. New York (NY): John Wiley & Sons; 1993.
- Bird RB, Klingenberg DJ. Multicomponent diffusion– A brief review. Adv Water Resour. 2013;62:238–242.
- Curtiss C, Bird RB. Multicomponent diffusion. Ind Eng Chem Res. 1999;38:2515–2522.
- Einstein A. On the motion required by the molecular kinetic theory of heat of small particles suspended in a stationary liquid. Ann Phys. 1905;17:549–560.
- Bian X, Kim C, Karniadakis GE. 111 years of Brownian motion. Soft Matter. 2016;12:6331–6346.
- Peters C, Wolff L, Vlugt TJH. Diffusion in liquids: experiments, molecular dynamics, and engineering models. In: Bedeaux D, Kjelstrup S, Sengers JV, editors. Experimental thermodynamics. Vol. X: Non-equilibrium thermodynamics with applications. 1st ed. Cambridge: Royal Society of Chemistry; 2015. p. 78–104.
- Cussler EL. Multicomponent diffusion. 1st ed. Amsterdam: Elsevier; 2013.
- Graham TI. The Bakerian lecture. On the diffusion of liquids. Phil Trans R Soc London. 1850;140:1–46.
- Fick AV. On liquid diffusion. Lond Edinb Dubl Phil Mag J Sci. 1855;10:30–39.
- Bardow A, Kriesten E, Voda MA, et al. Prediction of multicomponent mutual diffusion in liquids: model discrimination using NMR data. Fluid Phase Equilib. 2009;278:27–35.
- Peters C, Wolff L, Haase S, et al. Multicomponent diffusion coefficients from microfluidics using Raman microspectroscopy. Lab Chip. 2017;17:2768–2776.
- Piszko M, Batz K, Rausch MH. Diffusivities of binary mixtures consisting of carbon dioxide, methane, and propane by dynamic light scattering. J Chem Eng Data. 2019;65(3):1068–1082.
- Giraudet C, Knoll MS, Galvan Y, et al. Diffusion of gold nanoparticles in inverse opals probed by heterodyne dynamic light scattering. Transp Porous Media. 2020;131:723–737.
- Zangi P, Rausch MH, Fröba AP. Binary diffusion coefficients for gas mixtures of propane with methane and carbon dioxide measured in a loschmidt cell combined with holographic interferometry. Int J Thermophys. 2019;40:18.
- Wakeham WA, Nagashima A, Sengers JV. Measurement of the transport properties of fluids. Experimental thermodynamics. Vol. 3. International Union of Pure and Applied Chemistry. Commission on Thermodynamics. Oxford: Blackwell Scientific Publications; 1991.
- Wolff L, Koß H-J, Bardow A. The optimal diffusion experiment. Chem Eng Sci. 2016;152:392–402.
- Bardow A, Göke V, Koß H-J, et al. Concentration-dependent diffusion coefficients from a single experiment using model-based Raman spectroscopy. Fluid Phase Equilib. 2005;228:357–366.
- Rausch MH, Heller A, Fröba AP. Binary diffusion coefficients of glycerol–water mixtures for temperatures from 323 to 448 K by dynamic light scattering. J Chem Eng Data. 2017;62:4364–4370.
- Kriesten E, Voda M, Bardow A, et al. Direct determination of the concentration dependence of diffusivities using combined model-based Raman and NMR experiments. Fluid Phase Equilib. 2009;277:96–106.
- Celebi AT, Vlugt TJH, Moultos OA. Structural, thermodynamic and transport properties of aqueous reline and ethaline solutions from molecular dynamics simulations. J Phys Chem B. 2019;123(51):11014–11025.
- Edward JT. Molecular volumes and the Stokes-Einstein equation. J Chem Educ. 1970;47:261.
- Wilke C, Chang P. Correlation of diffusion coefficients in dilute solutions. AIChE J. 1955;1:264–270.
- Hopp M, Mele J, Gross J. Self-diffusion coefficients from entropy scaling using the PCP-SAFT equation of state. Ind Eng Chem Res. 2018;57:12942–12950.
- Mutoru JW, Leahy-Dios A, Firoozabadi A. Modeling infinite dilution and Fickian diffusion coefficients of carbon dioxide in water. AIChE J. 2011;57:1617–1627.
- Brokaw RS. Predicting transport properties of dilute gases. Ind Eng Chem Process Design Dev. 1969;8:240–253.
- Frenkel D, Smit B. Understanding molecular simulation: from algorithms to applications. 2nd ed. Vol. 1. San Diego (CA): Academic Press; 2002.
- Allen MP, Tildesley DJ. Computer simulation of liquids. 2nd ed. Oxford: Oxford University Press; 2017.
- Rapaport DC, Rapaport DCR. The art of molecular dynamics simulation. 2nd ed. Cambridge: Cambridge University Press; 2004.
- Tuckerman M. Statistical mechanics: theory and molecular simulation. 1st ed. Oxford: Oxford University Press; 2010.
- Evans DJ, Morriss GP. Statistical mechanics of nonequilibrium liquids. 2nd ed. Cambridge: Cambridge University Press; 2013.
- Maginn EJ. Molecular simulation of ionic liquids: current status and future opportunities. J Phys: Condens Matter. 2009;21:373101.
- Köddermann T, Paschek D, Ludwig R. Molecular dynamic simulations of ionic liquids: a reliable description of structure, thermodynamics and dynamics. Chem Phys Chem. 2007;8:2464–2470.
- Li Y, Xu J, Li D. Molecular dynamics simulation of nanoscale liquid flows. Microfluid Nanofluid. 2010;9:1011–1031.
- Todd B, Daivis PJ. Homogeneous non-equilibrium molecular dynamics simulations of viscous flow: techniques and applications. Mol Simul. 2007;33:189–229.
- Guevara-Carrion G, Janzen T, Muñoz-Muñoz YM, et al. Mutual diffusion of binary liquid mixtures containing methanol, ethanol, acetone, benzene, cyclohexane, toluene, and carbon tetrachloride. J Chem Phys. 2016;144:124501.
- Jiang H, Mester Z, Moultos OA, et al. Thermodynamic and transport properties of H2O+NaCl from polarizable force fields. J Chem Theory Comput. 2015;11:3802–3810.
- Orozco GA, Moultos OA, Jiang H, et al. Molecular simulation of thermodynamic and transport properties for the H2O+NaCl system. J Chem Phys. 2014;141:234507.
- Liu H, Maginn EJ. A molecular dynamics investigation of the structural and dynamic properties of the ionic liquid 1-n-butyl-3-methylimidazolium bis (trifluoromethanesulfonyl) imide. J Chem Phys. 2011;135:124507.
- Liu X, Schnell SK, Simon J-M, et al. Diffusion coefficients from molecular dynamics simulations in binary and ternary mixtures. Int J Thermophys. 2013;34:1169–1196.
- Liu X, Vlugt TJH, Bardow A. Maxwell–Stefan diffusivities in liquid mixtures: using molecular dynamics for testing model predictions. Fluid Phase Equilib. 2011;301:110–117.
- Guevara-Carrion G, Vrabec J, Hasse H. Prediction of self-diffusion coefficient and shear viscosity of water and its binary mixtures with methanol and ethanol by molecular simulation. J Chem Phys. 2011;134:074508.
- Fernández G, Vrabec J, Hasse H. Self diffusion and binary Maxwell–Stefan diffusion in simple fluids with the Green–Kubo method. Int J Thermophys. 2004;25:175–186.
- Fernández G, Vrabec J, Hasse H. Self-diffusion and binary Maxwell–Stefan diffusion coefficients of quadrupolar real fluids from molecular simulation. Int J Thermophys. 2005;26:1389–1407.
- Janzen T, Zhang S, Mialdun A, et al. Mutual diffusion governed by kinetics and thermodynamics in the partially miscible mixture methanol+ cyclohexane. Phys Chem Chem Phys. 2017;19:31856–31873.
- Janzen T, Vrabec J. Diffusion coefficients of a highly nonideal ternary liquid mixture: Cyclohexane–Toluene–Methanol. Ind Eng Chem Res. 2018;57:16508–16517.
- Jansen APJ. An introduction to kinetic Monte Carlo simulations of surface reactions. 1st ed. Vol. 856. Berlin: Springer; 2012.
- Wheeler DR, Newman J. Molecular dynamics simulations of multicomponent diffusion. 1. Equilibrium method. J Phys Chem B. 2004;108:18353–18361.
- Wheeler DR, Newman J. Molecular dynamics simulations of multicomponent diffusion. 2. Nonequilibrium method. J Phys Chem B. 2004;108:18362–18367.
- Erpenbeck JJ. Comparison of Green-Kubo and nonequilibrium calculations of the self-diffusion constant of a Lennard-Jones fluid. Phys Rev A. 1987;35:218.
- MacElroy J. Nonequilibrium molecular dynamics simulation of diffusion and flow in thin microporous membranes. J Chem Phys. 1994;101:5274–5280.
- Chempath S, Krishna R, Snurr RQ. Nonequilibrium molecular dynamics simulations of diffusion of binary mixtures containing short n-alkanes in faujasite. J Phys Chem B. 2004;108:13481–13491.
- Tenney CM, Maginn EJ. Limitations and recommendations for the calculation of shear viscosity using reverse nonequilibrium molecular dynamics. J Chem Phys. 2010;132:014103.
- Kubo R. Statistical-mechanical theory of irreversible processes. I. general theory and simple applications to magnetic and conduction problems. J Phys Soc Japan. 1957;12:570–586.
- Green MS. Markoff random processes and the statistical mechanics of time-dependent phenomena. II. Irreversible processes in fluids. J Chem Phys. 1954;22:398–413.
- Zhou Y, Miller GH. Green–Kubo formulas for mutual diffusion coefficients in multicomponent systems. J Phys Chem. 1996;100:5516–5524.
- Zhang Y, Otani A, Maginn EJ. Reliable viscosity calculation from equilibrium molecular dynamics simulations: a time decomposition method. J Chem Theory Comput. 2015;11:3537–3546.
- Heyes DM. Self-diffusion and shear viscosity of simple fluids. A molecular-dynamics study. J Chem Soc Faraday Trans 2: Mol Chem Phys. 1983;79:1741–1758.
- Meier K, Laesecke A, Kabelac S. Transport coefficients of the Lennard-Jones model fluid. II self-diffusion. J Chem Phys. 2004;121:9526–9535.
- Pranami G, Lamm MH. Estimating error in diffusion coefficients derived from molecular dynamics simulations. J Chem Theory Comput. 2015;11:4586–4592.
- Alder B, Wainwright T. Velocity autocorrelations for hard spheres. Phys Rev Lett. 1967;18:988.
- Liu H, Silva CM, Macedo EA. Unified approach to the self-diffusion coefficients of dense fluids over wide ranges of temperature and pressure– hard-sphere, square-well, Lennard-Jones and real substances. Chem Eng Sci. 1998;53:2403–2422.
- Vaz RV, Magalhães AL, Fernandes DL, et al. Universal correlation of self-diffusion coefficients of model and real fluids based on residual entropy scaling law. Chem Eng Sci. 2012;79:153–162.
- Ohtori N, Ishii Y. Explicit expressions of self-diffusion coefficient, shear viscosity, and the Stokes–Einstein relation for binary mixtures of Lennard-Jones liquids. J Chem Phys. 2015;143:164514.
- Heyes DM, Cass M, Powles JG, et al. Self-diffusion coefficient of the hard-sphere fluid: system size dependence and empirical correlations. J Phys Chem B. 2007;111:1455–1464.
- Vaz RV, Magalhães AL, Silva CM. Improved Stokes–Einstein based models for diffusivities in supercritical CO 2. J Taiwan Inst Chem Eng. 2014;45:1280–1284.
- Raabe G, Sadus RJ. Molecular dynamics simulation of the effect of bond flexibility on the transport properties of water. J Chem Phys. 2012;137:104512.
- Aimoli CG, Maginn EJ, Abreu CR. Transport properties of carbon dioxide and methane from molecular dynamics simulations. J Chem Phys. 2014;141:134101.
- Moultos OA, Zhang Y, Tsimpanogiannis IN, et al. System-size corrections for self-diffusion coefficients calculated from molecular dynamics simulations: the case of CO 2, n-alkanes, and poly (ethylene glycol) dimethyl ethers. J Chem Phys. 2016;145:074109.
- Kowsari M, Alavi S, Ashrafizaadeh M, et al. Molecular dynamics simulation of imidazolium-based ionic liquids. I. dynamics and diffusion coefficient. J Chem Phys. 2008;129:224508.
- Perkins SL, Painter P, Colina CM. Experimental and computational studies of choline chloride-based deep eutectic solvents. J Chem Eng Data. 2014;59:3652–3662.
- Wang J, Hou T. Application of molecular dynamics simulations in molecular property prediction II: diffusion coefficient. J Comput Chem. 2011;32:3505–3519.
- Bizzarri AR, Cannistraro S. Molecular dynamics simulation evidence of anomalous diffusion of protein hydration water. Phys Rev E. 1996;53:R3040.
- Klein T, Lenahan FD, Kerscher M, et al. Characterization of long linear and branched alkanes and alcohols for temperatures up to 573.15 K by surface light scattering and molecular dynamics simulations. J Phys Chem B. 2020;124:4146–4163.
- Wolff L, Jamali SH, Becker TM, et al. Prediction of composition-dependent self-diffusion coefficients in binary liquid mixtures: the missing link for Darken-based models. Ind Eng Chem Res. 2018;57:14784–14794.
- Maginn EJ, Messerly RA, Carlson DJ, et al. Best practices for computing transport properties 1. Self-diffusivity and viscosity from equilibrium molecular dynamics [article v1. 0]. Liv J Comp Mol Sci. 2018;1:6324.
- Moultos OA, Tsimpanogiannis IN, Panagiotopoulos AZ, et al. Atomistic molecular dynamics simulations of carbon dioxide diffusivity in n-hexane, n-decane, n-hexadecane, cyclohexane, and squalane. J Phys Chem B. 2016;120:12890–12900.
- Shah D, Mjalli FS. Effect of water on the thermo-physical properties of Reline: an experimental and molecular simulation based approach. Phys Chem Chem Phys. 2014;16:23900–23907.
- Baz J, Held C, Pleiss J, et al. Thermophysical properties of glyceline–water mixtures investigated by molecular modelling. Phys Chem Chem Phys. 2019;21:6467–6476.
- dos Santos TJ, Abreu CR, Horta BA, et al. Self-diffusion coefficients of methane/n-hexane mixtures at high pressures: an evaluation of the finite-size effect and a comparison of force fields. J Supercrit Fluids. 2020;155:104639.
- Krishna R, Van Baten JM. The darken relation for multicomponent diffusion in liquid mixtures of linear alkanes: an investigation using molecular dynamics (MD) simulations. Ind Eng Chem Res. 2005;44:6939–6947.
- Guevara-Carrion G, Fingerhut R, Vrabec J. Fick diffusion coefficient matrix of a quaternary liquid mixture by molecular dynamics. J Phys Chem B. 2020;124:4527–4535.
- Liu X, Bardow A, Vlugt TJH. Multicomponent Maxwell–Stefan diffusivities at infinite dilution. Ind Eng Chem Res. 2011;50:4776–4782.
- Van De Ven-Lucassen IM, Thijs AJVT, Vlugt JH, et al. Using molecular dynamics to obtain Maxwell–Stefan diffusion coefficients in liquid systems. Mol Phys. 1998;94:495–503.
- Krishna R, Wesselingh J. The Maxwell–Stefan approach to mass transfer. Chem Eng Sci. 1997;52:861–911.
- Par S, Guevara-Carrion G, Hasse H, et al. Mutual diffusion in the ternary mixture of water+ methanol+ ethanol and its binary subsystems. Phys Chem Chem Phys. 2013;15:3985–4001.
- Higgoda UA, Kankanamge CJ, Hellmann R, et al. Fick diffusion coefficients of binary fluid mixtures consisting of methane, carbon dioxide, and propane via molecular dynamics simulations based on simplified pair-specific ab initio-derived force fields. Fluid Phase Equilib. 2019;502:112257.
- Jamali SH, van Westen T, Moultos OA, et al. Optimizing nonbonded interactions of the OPLS force field for aqueous solutions of carbohydrates: how to capture both thermodynamics and dynamics. J Chem Theory Comput. 2018;14:6690–6700.
- Vella JR. Fick diffusion coefficients of the gaseous CH 4–CO 2 system from molecular dynamics simulations using traPPE force fields at 101.325, 506.625, 1013.25, 2533.12, and 5066.25 kPa. J Chem Eng Data. 2019;64:3672–3681.
- Onsager L. Theories and problems of liquid diffusion. Ann N Y Acad Sci. 1945;46:241–265.
- Krishna R, Van Baten JM. Onsager coefficients for binary mixture diffusion in nanopores. Chem Eng Sci. 2008;63:3120–3140.
- Kjelstrup S, Schnell SK, Vlugt TJH, et al. Bridging scales with thermodynamics: from nano to macro. Adv Nat Sci: Nanosci Nanotechnol. 2014;5:023002.
- Schnell SK, Liu X, Simon J-M, et al. Calculating thermodynamic properties from fluctuations at small scales. J Phys Chem B. 2011;115:10911–10918.
- Fingerhut R, Herres G, Vrabec J. Thermodynamic factor of quaternary mixtures from Kirkwood–Buff integration. Mol Phys. 2020;118:e1643046.
- Kirkwood JG, Buff FP. The statistical mechanical theory of solutions. I. J Chem Phys. 1951;19:774–777.
- Dawass N, Krüger P, Schnell SK, et al. Kirkwood–Buff integrals using molecular simulation: estimation of surface effects. Nanomaterials. 2020;10:771.
- Dawass N, Krüger P, Schnell SK, et al. Kirkwood–Buff integrals from molecular simulation. Fluid Phase Equilib. 2019;486:21–36.
- Krüger P, Schnell SK, Bedeaux D, et al. Kirkwood–Buff integrals for finite volumes. J Phys Chem Lett. 2013;4:235–238.
- Hall D. Kirkwood–Buff theory of solutions. an alternative derivation of part of it and some applications. Trans Faraday Soc. 1971;67:2516–2524.
- Dawass N, Krüger P, Simon J-M, et al. Kirkwood–Buff integrals of finite systems: shape effects. Mol Phys. 2018;116:1573–1580.
- Schnell SK, Englebienne P, Simon J-M, et al. How to apply the Kirkwood–Buff theory to individual species in salt solutions. Chem Phys Lett. 2013;582:154–157.
- Ben-Naim A. Molecular theory of solutions. 1st ed. Oxford: Oxford University Press; 2006.
- Oseen CW. Neuere methoden und ergebnisse in der hydrodynamik. Leipzig: Akademische Verlagsgesellschaft mb H; 1927.
- Pratt LR, Hummer G. Simulation and theory of electrostatic interactions in solution: computational chemistry, biophysics and aqueous solutions, Santa Fe, New Mexico, USA, 23–25 June 1999. 1st ed. New York (NY): American Institute of Physics; 1999.
- Dünweg B, Kremer K. Molecular dynamics simulation of a polymer chain in solution. J Chem Phys. 1993;99:6983–6997.
- Yeh I-C, Hummer G. System-size dependence of diffusion coefficients and viscosities from molecular dynamics simulations with periodic boundary conditions. J Phys Chem B. 2004;108:15873–15879.
- Simonnin P, Noetinger B, Nieto-Draghi C, et al. Diffusion under confinement: hydrodynamic finite-size effects in simulation. J Chem Theory Comput. 2017;13:2881–2889.
- Jamali SH, Bardow A, Vlugt TJH. A generalized form for finite-size corrections in mutual diffusion coefficients of multicomponent mixtures Obtained from equilibrium molecular dynamics simulation. J Chem Theory Comput. 2020;16(6):3799–3806.
- Dünweg B, Kremer K. Microscopic verification of dynamic scaling in dilute polymer solutions: a molecular-dynamics simulation. Phys Rev Lett. 1991;66:2996.
- Young JM, Panagiotopoulos AZ. System-size dependence of electrolyte activity coefficients in molecular simulations. J Phys Chem B. 2018;122:3330–3338.
- Sellan DP, Landry ES, Turney J, et al. Size effects in molecular dynamics thermal conductivity predictions. Phys Rev B. 2010;81:214305.
- Klenk MJ, Lai W. Finite-size effects on the molecular dynamics simulation of fast-ion conductors: A case study of lithium garnet oxide Li 7La 3Zr 2O 1. Solid State Ionics. 2016;289:143–149.
- Krüger P, Vlugt TJH. Size and shape dependence of finite-volume Kirkwood–Buff integrals. Phys Rev E. 2018;97:051301.
- Dawass N, Krüger P, Schnell SK, et al. Finite-size effects of Kirkwood–Buff integrals from molecular simulations. Mol Simul. 2018;44:599–612.
- Mondello M, Grest GS. Viscosity calculations of n-alkanes by equilibrium molecular dynamics. J Chem Phys. 1997;106:9327–9336.
- Kim K-S, Kim C, Karniadakis GE, et al. Density-dependent finite system-size effects in equilibrium molecular dynamics estimation of shear viscosity: hydrodynamic and configurational study. J Chem Phys. 2019;151:104101.
- Jamali SH, Hartkamp R, Bardas C, et al. Shear viscosity computed from the finite-Size effects of self-diffusivity in equilibrium molecular dynamics. J Chem Theory Comput. 2018;14:5959–5968.
- Tsimpanogiannis IN, Moultos OA, Franco LF, et al. Self-diffusion coefficient of bulk and confined water: a critical review of classical molecular simulation studies. Mol Simul. 2019;45:425–453.
- Jamali SH, Wolff L, Becker TM, et al. Finite-size effects of binary mutual diffusion coefficients from molecular dynamics. J Chem Theory Comput. 2018;14:2667–2677.
- Cappelezzo M, Capellari C, Pezzin S, et al. Stokes–Einstein relation for pure simple fluids. J Chem Phys. 2007;126:224516.
- Moultos OA, Tsimpanogiannis IN, Panagiotopoulos AZ. Atomistic molecular dynamics simulations of CO2 diffusivity in H2O for a wide range of temperatures and pressures. J Phys Chem B. 2014;118:5532–5541.
- Plimpton S. Fast parallel algorithms for short-range molecular dynamics. J Comput Phys. 1995;117:1–19.
- Berendsen HJ, van der Spoel D, van Drunen R. GROMACS: a message-passing parallel molecular dynamics implementation. Comput Phys Commun. 1995;91:43–56.
- Ramírez J, Sukumaran SK, Vorselaars B, et al. Efficient on the fly calculation of time correlation functions in computer simulations. J Chem Phys. 2010;133:154103.
- Jamali SH, Wolff L, Becker TM, et al. A tool for on-the-fly calculation of transport properties of fluids with the order-n algorithm in LAMMPS. J Chem Inf Model. 2019;59:1290–1294.
- https://github.com/omoultosEthTuDelft/OCTP.
- Dubbeldam D, Ford DC, Ellis DE, et al. A new perspective on the order-n algorithm for computing correlation functions. Mol Simul. 2009;35:1084–1097.
- Humbert MT, Zhang Y, Maginn EJ. PyLAT: python LAMMPS analysis tools. J Chem Inf Model. 2019;59:1301–1305.
- Deublein S, Eckl B, Stoll J, et al. ms2: A molecular simulation tool for thermodynamic properties. Comput Phys Commun. 2011;182:2350–2367.
- Jorgensen WL, Chandrasekhar J, Madura JD, et al. Comparison of simple potential functions for simulating liquid water. J Chem Phys. 1983;79:926–935.
- Kikugawa G, Ando S, Suzuki J, et al. Effect of the computational domain size and shape on the self-diffusion coefficient in a Lennard-Jones liquid. J Chem Phys. 2015;142:024503.
- Hasimoto H. On the periodic fundamental solutions of the Stokes equations and their application to viscous flow past a cubic array of spheres. J Fluid Mech. 1959;5:317–328.
- Tazi S, Boţan A, Salanne M, et al. Diffusion coefficient and shear viscosity of rigid water models. J Phys: Condens Matter. 2012;24:284117.
- Erdõs M, Michalis F, Vlugt TJH, et al. Diffusivity of [ α]-, [ β]-, [ γ]-cyclodextrin and the inclusion complex of [ β]-cyclodextrin:Ibuprofen in aqueous solutions, a molecular dynamics simulation study. Submitted in Phase Equilibria; 2020.
- Tsimpanogiannis IN, Jamali SH, Economou IG. On the validity of the Stokes–Einstein relation for various water force fields. Mol Phys. 2019;118:e1702729.
- Mark P, Nilsson L. Structure and dynamics of the TIP3P, SPC, and SPC/E water models at 298 K. J Phys Chem A. 2001;105:9954–9960.
- Enders S, Kahl H, Winkelmann J. Surface tension of the ternary system water+ acetone+ toluene. J Chem Eng Data. 2007;52:1072–1079.
- Jorgensen WL, Maxwell DS, Tirado-Rives J. Development and testing of the OPLS all-atom force field on conformational energetics and properties of organic liquids. J Am Chem Soc. 1996;118:11225–11236.
- Zhao L, Cheng T, Sun H. On the accuracy of predicting shear viscosity of molecular liquids using the periodic perturbation method. J Chem Phys. 2008;129:144501.
- Berendsen H, Grigera J, Straatsma T. The missing term in effective pair potentials. J Phys Chem. 1987;91:6269–6271.
- Bocquet L, Barrat J-L. Diffusive motion in confined fluids: mode-coupling results and molecular-dynamics calculations. Europhys Lett. 1995;31:455.
- Lyklema J. Fundamentals of interface and colloid science: soft colloids. 1st ed. San Diego (CA): Elsevier; 1995.
- Karniadakis G, Beskok A, Aluru N. Microflows and nanoflows: fundamentals and simulation. 2nd ed. Vol. 29. New York (NY): Springer Science & Business Media; 2005.
- Erdõs M, Galteland O, Bedeaux D, et al. Gibbs ensemble Monte Carlo simulation of fluids in confinement: relation between the differential and integral pressures. Nanomaterials. 2020;10:293.
- Rauter MT, Galteland O, Erdõs M, et al. Two-phase equilibrium conditions in nanopores. Nanomaterials. 2020;10:608.
- Hannaoui R, Galliero G, Hoang H, et al. Influence of confinement on thermodiffusion. J Chem Phys. 2013;139:114704.
- Collell J, Galliero G. Determination of the thermodynamic correction factor of fluids confined in nano-metric slit pores from molecular simulation. J Chem Phys. 2014;140:194702.
- Suk M, Aluru NR. Molecular and continuum hydrodynamics in graphene nanopores. RSC Adv. 2013;3:9365–9372.
- Ghorbanian J, Celebi AT, Beskok A. A phenomenological continuum model for force-driven nano-channel liquid flows. J Chem Phys. 2016;145:184109.
- Thomas JA, McGaughey AJ. Reassessing fast water transport through carbon nanotubes. Nano Lett. 2008;8:2788–2793.
- Hoang H, Galliero G. Grand canonical-like molecular dynamics simulations: application to anisotropic mass diffusion in a nanoporous medium. J Chem Phys. 2012;136:184702.
- Celebi AT, Barisik M, Beskok A. Electric field controlled transport of water in graphene nano-channels. J Chem Phys. 2017;147:164311.
- Travis KP, Todd B, Evans DJ. Departure from Navier–Stokes hydrodynamics in confined liquids. Phys Rev E. 1997;55:4288.
- Qiao R, Aluru NR. Ion concentrations and velocity profiles in nanochannel electroosmotic flows. J Chem Phys. 2003;118:4692–4701.
- Celebi AT, Nguyen CT, Hartkamp R, et al. The role of water models on the prediction of slip length of water in graphene nanochannels. J Chem Phys. 2019;151:174705.
- Bocquet L, Charlaix E. Nanofluidics, from bulk to interfaces. Chem Soc Rev. 2010;39:1073–1095.
- Hoang H, Galliero G. Local viscosity of a fluid confined in a narrow pore. Phys Rev E. 2012;86:021202.
- Celebi AT, Barisik M, Beskok A. Surface charge-dependent transport of water in graphene nano-channels. Microfluid Nanofluid. 2018;22:7.
- Giannakopoulos A, Sofos F, Karakasidis T, et al. Unified description of size effects of transport properties of liquids flowing in nanochannels. Int J Heat Mass Transf. 2012;55:5087–5092.
- Sofos F, Karakasidis TE, Liakopoulos A. How wall properties control diffusion in grooved nanochannels: a molecular dynamics study. Heat and Mass Transf. 2013;49:1081–1088.
- Marry V, Rotenberg B, Turq P. Structure and dynamics of water at a clay surface from molecular dynamics simulation. Phys Chem Chem Phys. 2008;10:4802–4813.
- Wei M-J, Zhang L, Lu L, et al. Molecular behavior of water in TiO 2 nano-slits with varying coverages of carbon: a molecular dynamics simulation study. Phys Chem Chem Phys. 2012;14:16536–16543.
- Joseph S, Aluru NR. Why are carbon nanotubes fast transporters of water? Nano Lett. 2008;8:452–458.
- Guigas G, Weiss M. Size-dependent diffusion of membrane inclusions. Biophys J. 2006;91:2393–2398.
- Bashardanesh Z, Elf J, Zhang H, et al. Rotational and translational diffusion of proteins as a function of concentration. ACS Omega. 2019;4:20654–20664.
- Haridasan N, Kannam SK, Mogurampelly S, et al. Rotational diffusion of proteins in nanochannels. J Phys Chem B. 2019;123:4825–4832.
- Linke M, Köfinger J, Hummer G. Fully anisotropic rotational diffusion tensor from molecular dynamics simulations. J Phys Chem B. 2018;122:5630–5639.
- Linke M, Köfinger J, Hummer G. Rotational diffusion depends on box size in molecular dynamics simulations. J Phys Chem Lett. 2018;9:2874–2878.
- Vögele M, Köfinger J, Hummer G. Finite-Size-Corrected rotational diffusion coefficients of membrane proteins and carbon nanotubes from molecular dynamics simulations. J Phys Chem B. 2019;123:5099–5106.
- Taylor R, Kooijman HA. Composition derivatives of activity coefficient models (for the estimation of thermodynamic factors in diffusion). Chem Eng Commun. 1991;102:87–106.
- Chen B, Potoff JJ, Siepmann JI. Monte Carlo calculations for alcohols and their mixtures with alkanes. Transferable potentials for phase equilibria. 5. United-atom description of primary, secondary, and tertiary alcohols. J Phys Chem B. 2001;105:3093–3104.
- Wick CD, Stubbs JM, Rai N, et al. Transferable potentials for phase equilibria. 7. Primary, secondary, and tertiary amines, nitroalkanes and nitrobenzene, nitriles, amides, pyridine, and pyrimidine. J Phys Chem B. 2005;109:18974–18982.
- Martin MG, Siepmann JI. Transferable potentials for phase equilibria. 1. United-atom description of n-alkanes. J Phys Chem B. 1998;102:2569–2577.
- Jorgensen WL, Madura JD, Swenson CJ. Optimized intermolecular potential functions for liquid hydrocarbons. J Am Chem Soc. 1984;106:6638–6646.