Abstract
In natural resource management contexts, citizen science programs often involve a diversity of actors collaborating in broad social networks. However, the characteristics and functioning of these networks has received limited attention. In this article, we demonstrate the benefits of applying social network research to citizen science practice. Through a mixed methods approach, we compare data sharing and collaboration networks of two community-based water monitoring programs in Australia. The quantitative component revealed that despite similarities in actor diversity and activities, both programs have created divergent network structures (centralized vs decentralized) corresponding to their scale of influence in environmental decision-making (regional vs local impacts). The qualitative component showed how individuals navigated their relationships and managed key tensions inherent to network functioning. This study provides new insights on the role of social networks in citizen science and concludes by recommending program leaders adopt a “networking mindset” to maximize their sphere of influence.
Introduction
Citizen science—a growing practice involving members of the public in producing scientific knowledge—is increasingly embedded in government policy to improve the management of natural resources while strengthening the capacities of local communities (McKinley et al. Citation2017). Although academic literature highlights the importance of citizen science for meeting various governance objectives (Aceves-Bueno et al. Citation2015; Buytaert et al. Citation2014; Newman et al. Citation2017), the uptake and contribution of citizen science to environmental decision-making remain poorly understood (Hecker et al. Citation2018). Research suggests that effective citizen science in governance contexts depends on institutional support and cross-scale relationships (Irwin Citation2015; Stepenuck and Genskow Citation2018), locally adaptive community structures, functional coordination and pathways to facilitate the flow of information (Pollock and Whitelaw Citation2005). These insights are consistent with a growing body of literature demonstrating how social networks are crucial factors shaping knowledge uptake, learning, trust and collective action in collaborative environmental initiatives (Innes and Booher Citation2004; Leach and Sabatier Citation2005; Groce et al. Citation2019).
In citizen science, social networks are commonly cited as critical to the development of important social processes (i.e., knowledge sharing, communication) (Forrester et al. Citation2017; McGreavy et al. Citation2016), individual and program outcomes (i.e., behavior change, data uptake) (Chase and Levine Citation2018; Nerbonne and Nelson Citation2008) and social attributes (i.e., trust, social capital) (Fernandez-Gimenez, Ballard, and Sturtevant Citation2008; Overdevest, Orr, and Stepenuck Citation2004). Yet, few studies have sought to quantify or actively describe the relational patterns making up citizen science programs (Richter et al. Citation2018), opting, instead, to view social networks as a metaphor whose presence (or absence) have important consequences.
This article examines how social networks shape the contributions of two community-based water monitoring programs to water resource management and policy. Specifically, we ask: How is social network structure related to the capacity of citizen science networks to influence water resource management and policy, and how do the conceptual and methodological tools of social network analysis advance this understanding? We define citizen science networks as the overall patterns of relationships between individuals (e.g., volunteers, coordinators, scientists and decision-makers) who are regarded by a majority as members as influencing the planning, implementation and outcomes of a citizen science initiative. In what follows, we provide an overview of a social network perspective and its opportunities for citizen science theory and practice. This is empirically explored in a comparative study of two longstanding community-based water monitoring projects in Australia.
Citizen Science and Social Networks: Toward a “Structurally Explicit” Approach
Citizen science is a “profoundly social activity” (Irwin Citation2015, 2). A project’s capacity to recruit and coordinate volunteers, collaborate with others, collect and share data and influence people in positions of power largely depends on the characteristics and quality of human relationships. A wide range of actors are now partnering through citizen science, each possessing different values, motivations and capacities to exert influence (Göbel, Martin, and Ramírez-Andreotta Citation2016). However, the ways in which these actors are connected in broad social networks and particular sets of relationships is unknown. To gain such insights, it can be useful to adopt a social network perspective (a “structurally-explicit’ approach”), which centers investigations on the structure, meaning and consequences of social relationships (Bodin and Prell Citation2011).
In recent years, a social network perspective has proven useful in studying key issues in environmental governance, such as information diffusion, power relations, and individual behavior (Groce et al. Citation2019). Practically, the approach provides a mechanism to better understand the degree of connectivity among individuals or organizations, identify key stakeholders (Farr, Reed, and Pejchar Citation2018), or the implications of different network patterns and characteristics. Such a perspective may significantly benefit citizen science, since citizen science and environmental governance address related concepts, such as participation, collaboration, capacity-building, social-ecological resilience and learning for sustainability.
Analyzing Social Networks
Social network analysis refers to the set of tools used to systematically quantify and visualize social networks (Wasserman and Faust Citation1994). It makes use of social network theory and graph theory to characterize social structure in terms of nodes (e.g., individuals, organizations) connected by relations, or “edges.” Relations can be of many different kinds, such as communication, information sharing or trust, with each relation capable of giving rise to a single network with certain patterns and measurable features (Borgatti et al. Citation2009). For example, researchers may be interested in how citizen science data flows through a policy network and ask respondents to list the names of people with whom they share data. In this case, the resulting network can be described as a data sharing network. Other studied relations could be communication networks, collaboration networks or networks based on trust. The combination and characteristics of nodes and ties results in a network configuration composed of specific patterns and measurable features for analysis and visualization.
Only one study to our knowledge has investigated citizen science networks quantitatively using social network analysis. This study sought to understand drivers of long-term volunteer participation highlighting the role of central coordination and peer support in personal satisfaction and engagement (Richter et al. Citation2018). Other researchers have highlighted the importance of social networks but in a more descriptive manner. Overdevest, Orr, and Stepenuck (Citation2004) described how volunteer stream monitoring programs that create more “dense” networks of participants build social capital, enable individual political participation and promote program stability. Conrad (Citation2006) described how social structure can be a “social liability” that can create communication barriers and impact the performance of groups within a larger network. Jalbert, Kinchy, and Perry (Citation2014) described how community-based water monitoring programs in the United States operated in either centralized networks, where central hub organizations connected different groups together, or decentralized networks, where organizations shared resources and collaborated on projects without a central hub. Although these more descriptive studies highlight the importance role of structural and relational dimensions in citizen science, the study by Richter et al. (Citation2018) shows the possibilities of quantitative studies linking network structure to a particular outcome of interest.
Linking Networks to Outcomes
Understanding the link between network structure and outcomes requires an additional data set alongside network connection data and beyond a single case (Groce et al. Citation2019). For citizen science projects monitoring natural resources, environmental improvements such as improved water quality are usually the ultimate goal. However, demonstrating a link between citizen science and environmental outcomes is a difficult task and few studies make this link (Conrad and Hilchey Citation2011). In acknowledging a similar challenge in catchment groups in the United States, Floress, Prokopy, and Allred (Citation2011) suggested a useful outcome measure is one that identifies outcomes beyond social processes but prior to environmental outcomes, or so-called “intermediate outcomes” (Carr, Blöschl, and Loucks Citation2012). For citizen science, an important intermediate outcome relates to a program’s contributions to natural resource management and policy decisions. This could be evidenced by instances of data uptake in reports, policies or management actions. Both Carr, Blöschl, and Loucks (Citation2012) and Groce et al. (Citation2019) suggest that intermediate outcomes can be considered a precursor to broader impacts on environmental improvement.
illustrates three typical network structures we may discover in citizen science projects: (a) a distributed network in which the number of “ties” between individuals or organizations are equally distributed; (b) a decentralized network in which there are multiple “hubs” of connectivity; and, (c) a centralized network in which ties are connected to a single or few actors (Baran Citation1964). Insights from environmental governance literature (e.g. Bodin and Crona Citation2009) show that centralized networks are more efficient at solving simple and easily identified tasks but can be vulnerable to collapse, due to a strong reliance on a few key individuals. Alternatively, decentralized network structures have higher resilience, promote deliberation and generate information at different parts and scales of the problem, but may require more intensive coordination efforts. Finally, distributed networks may be important to build trust and community capacity since all participants are equally committed to the goals of the network (Provan and Kenis Citation2007).
Understanding Social Networks through Qualitative Methods
Although quantitative approaches to social network analysis have many benefits, they have limitations when considering the content, quality, and meaning of relationships (Hollstein Citation2010; Fuhse and Mützel Citation2011). Qualitative methods are recommended when researchers are interested in how actors navigate and experience their relationships (Hollstein Citation2014; Bolíbar Citation2016). Additionally, applying a mixed methods social network analysis enables the triangulation of data and insights to enhance the validity and explanatory power of network studies (Bolíbar Citation2016).
A potential application of qualitative methods in social network analysis is to examine various network tensions or, what Provan and Kenis (Citation2007, 14) call, “contradictory logics.” The authors describe three such tensions which affect network function “that network members must deal with”:
efficiency versus inclusiveness, whereby efforts to promote inclusive and equitable participation in the network (i.e., through collaboration and more distributed network structures) might reduce a program’s capacity to act as a collective.
internal and external legitimacy, which aims to satisfy the expectations of participants within a network while also meeting expectations from external parties, such as funding agencies.
flexibility and stability, which refers to the need to be adaptive in response to changing individual and organizational needs or environmental conditions while maintaining consistency in actions over time.
To summarize, a social network perspective offers citizen science researchers a lens that centers investigations on social relationships, rather than individual attributes (Marin and Wellman Citation2011). Its application provides a way to understand the degree of connectivity between actors, identify relevant stakeholders and understand the implications of different network patterns and characteristics. Furthermore, qualitative methods provide an additional mechanism to validate and interpret the quantitative component of the social network analysis. They can also reveal how social networks and individual relations shape, and are shaped by, the specific contexts in which they occur. Here, we present a mixed-methods approach to examine how collaboration unfolds and how information is shared and applied in management and policy-oriented citizen science.
Methods
Comparative Case Study
In this study, the relationship between network structure, function and outcomes is explored through a comparative case study of two longstanding citizen science programs in Australia: Mill Stream Waterwatch and Regional Highlands Waterwatch.Footnote1 A comparative case study is a method of inquiry that investigates a social phenomenon across two or more cases in their situated contexts. Unlike single case studies, the core objective of a comparative case study is to investigate patterns, similarities and differences across selected cases (Mills, van de Bunt, and de Bruijn Citation2006). Through the comparative study, we were able formulate a specific understanding about how network structure impacts knowledge outcomes and collaboration in management and policy-oriented citizen science. It further enabled an understanding of how social context influenced tie formation and overall network effectiveness. While we acknowledge our capacity to generalize findings to a larger population of cases is limited (Yin, Citation2013), we consider the cases under study as typical examples of community-based water monitoring programs in other parts of the world (e.g. Buckland-Nicks, Castleden, and Conrad Citation2016; Latimore and Steen Citation2014; Weston and Conrad Citation2015), which can contribute to their generalizability.
Both Mill Stream Waterwatch and Regional Highlands Waterwatch form part of a national network of community-based water monitoring programs that conduct activities under the “Waterwatch” brand. Both programs share a similar philosophy of engaging the public in water resource management. However, they have each developed their own goals, activities and processes of coordination allowing them to adapt to their individual contexts ().
Table 1. Key attributes of the two case studies.
Case Selection Rationale
The study cases were chosen due to several key similarities, including (i) their long history involving volunteers in water resource management, (ii) established relationships with diverse organizations, (iii) a demonstrated capacity to contribute data to inform the decision-making and (iv) large numbers of volunteers and monitoring sites across broad geographic areas. Key differences between the cases relate to their primary objectives (as described above) and their focus in different geographical contexts. Mill Stream Waterwatch is largely focused on data collection activities in urban and peri-urban environments (urban-rural transition zones). Regional Highlands Waterwatch operates across more rural regions with some urban sites. Both programs deliver multiple subprograms, such as collecting data on frogs, macroinvertebrates, platypus and other water quality and environmental indicators. However, in this study we focus attention on the social networks associated with the long-term water quality monitoring component of each program.
Research Design
The comparative social network analysis employed a mixed methods approach through a convergent research design involving three independent phases (Fetters, Curry, and Creswell Citation2013): (1) a quantitative social network analysis of data collected through an online questionairre; (2) review of secondary data from a previous study; (3) collection and analysis of qualitative interview data. In a convergent design, the purpose of the quantitative strand is to analyze and visualize the structural characteristics of the social network whereas the qualitative strand provided a means to deepen our understanding of the social processes that contribute to network formation (Hollstein Citation2014). In this study, both data strands were collected across a similar timeframe, with analysis following the collection and analysis of each data strand (Fetters, Curry, and Creswell Citation2013). However, preliminary analysis of quantitative data inform the selection of some interviewees, which is known as an “interactive” approach to method integration (Fetters, Curry, and Creswell Citation2013).
Network Data Collection
We followed a snowball sampling approach (Doreian and Woodard Citation1992) to survey actors participating in or interacting with each program, starting with program coordinators. The coordinators were asked to nominate potential participants who were then asked to nominate others. This approach continued as the central mechanism to achieve a sufficient sample size (detailed below). The questionnaire to map network structure followed a name generator and interpreter method (Robins Citation2015) and is presented in Supplementary Material A. The questionnaire asked respondents to recall up to ten individuals with whom they interact in citizen science networks. For the purposes of this study, an “interaction” was defined as (1) sharing citizen science monitoring data and (2) collaborating on monitoring activities and projects. These relations were chosen because data sharing and collaboration are fundamental social processes in management and policy-oriented citizen science. A second section of the questionnaire asked participants about group affiliation, role and their duration of employment or participation. To maximize the use of limited resources, we made a special effort to obtain responses from people frequently mentioned by others to ensure a more representative network. Moreover, we relied on coordinators to recruit volunteers on behalf of the research team due to ethical considerations limiting direct recruitment.
In total, 29 emails were sent to individuals in professional roles in the Mill Stream case while 27 were sent out in the Regional Highlands case. A total of 21 and 22 questionnaires were returned, respectively, for a response rate of 72% and 81%. The response rate for volunteers was difficult to measure due to our recruitment method. We received responses from 43 volunteers from Mill Stream Waterwatch and 38 volunteers in the Regional Highlands case. Given both programs report that they coordinate around 200 volunteers per program, the response rate for volunteers is considered relatively low at 19% and 22%, respectively.
Social Network Analysis
Our research design allowed the generation of two networks: data sharing and collaboration. We grouped respondents into six categories: volunteers, coordinators, scientists, waterway managers, policymakers and “other” actors, which included educators and staff from non-government organizations. Relationships were symmetrized to produce an “undirected” network where all ties are reciprocated (Borgatti, Everett, and Johnson Citation2013). By symmetrizing data, we have assumed that if one individual reported a tie, it was present in both directions, which can be a suitable approach in cases of high non-response rates (e.g. White et al. Citation2022).
To investigate the network structure, we quantified four network-level measures relevant to our research focus and considered important metrics to understand collaborative environmental governance (Bodin, Crona, and Ernstson Citation2006; Bodin and Crona Citation2009; Prell, Hubacek, and Reed Citation2009). The metrics included density, network centralization, core-periphery analysis and cross-boundary exchange. describes each measure assessed in this study and its social meaning for citizen science. Network measures were calculated using UCINET (Borgatti, Everett, and Freeman Citation2002). We illustrated the networks using the social network visualization software NetDraw (Borgatti Citation2002).
Table 2. Network measures analyzed in this study and their social meaning for citizen science.
Program Outcome Data
To understand the influence of different network structures on the outcomes of citizen science, we conducted an analysis on a secondary dataset collected as part of a previous study of freshwater citizen science in Australia. This dataset compiled evidence of citizen science data informing natural resource management decision-making in the form of organizational reports, government policies or management actions. From this dataset, we extracted and reviewed the evidence of data uptake for each case and categorized these instances along two axes: (1) the management scale (local, sub-catchment, catchment, regional) whereby each separate instance of data uptake corresponded to, and (2) their contribution across a typical adaptive management cycle (strategy and planning, monitoring and intervention, reporting and evaluation) (Jones Citation2005). This structuring acknowledges citizen science data can be useful at different management scales and at different stages of management and policy cycles (Aceves-Bueno et al. Citation2015).
Interview Data
To understand network functioning, a total of 40 semi-structured interviews were conducted (by phone and in-person) following a preliminary social network analysis. Participants included volunteers (n = 20), coordinators (n = 10), catchment managers, policymakers and scientists (n = 6) and other actors, including community engagement professionals (n = 4). Interview questions focused on: the nature of individual collaboration with other people and organizations; the contributions of citizen science to understanding and managing waterways; and the enablers and constraints of building new and strengthening existing relationships. The interview schedule is shown in Supplementary Material B. Interviews were audio recorded, transcribed and ranged in length between 30 and 90 min.
Analyzing the interview data was achieved through thematic analysis (Braun et al. Citation2019). Thematic analysis is a flexible method of analyzing qualitative data, which systematically identifies meaningful patterns of ideas of concepts across a data set. We first coded interview data through an inductive (i.e., data-driven) and deductive (i.e., theory-driven) approach (Fereday and Muir-Cochrane Citation2006). This approach acknowledges that observations were, to varying extents, informed by preexisting concepts and theories in social network and citizen science literature while, at the same time, being guided by the interview data to ensure an openness to emerging ideas. Coding was conducted using the software NVivo 12 (QSR International). Several cycles of coding were completed to identify consistency across the dataset within and across interviewees and study cases. A sample of coded transcripts were cross-checked by two coauthors to ensure coding consistency. However, inter-coder reliability scores were not taken.
The next stage involved constructing themes and subthemes that captured patterns of ideas or concepts that occurred within and across the study cases. Theme construction was a creative, active process (Braun et al. Citation2019), as the researcher makes choices about how best to structure and make sense of the coded data. Categories of codes were reviewed and clustered into themes when they appeared to share some underlying similarity or unifying feature about the relational processes and functions within and between study cases (see Supplementary Material C). We then applied Provan and Kenis (Citation2007) concept of network tensions to conceptually explain our findings.
Results
Our results are presented here in three parts. First, we present and compare the characteristics of the data sharing and collaboration networks for each study case based on the analysis of network data. Second, we describe the type and scale of data contributions each program has made to decision-making to enable the interpretation of correlations between network structure and outcomes. Finally, we discuss insights from the interview data detailing how actors navigated and experienced their relationships to examine how inherent network tensions affected the functioning of each program.
Network Structure
illustrates the data sharing and collaboration networks for the two citizen science programs. Network size was measured by the number of nodes in each network. Regional Highlands Waterwatch was smaller in size than the Mill Stream case, but had a greater number of ties. While each program had similar role diversity, the proportions of actor groups varied across programs and network relations (). For example, Regional Highlands Waterwatch was composed of more scientists than Mill Stream Waterwatch for both networks (green nodes in ). Low policy-maker presence was evident in both programs: only one policymaker was found to be involved in the Regional Highlands case compared to none in the Mill Stream case.
Figure 2. Social networks based on data sharing and collaboration ties in (a) Regional Highlands Waterwatch and (b) Mill Stream Waterwatch. Size of nodes represents the number of ties of each node (degree) and the color of the node denotes actor roles: yellow (volunteers); red (coordinators); blue (catchment managers); purple (policymakers); and brown (other stakeholders).
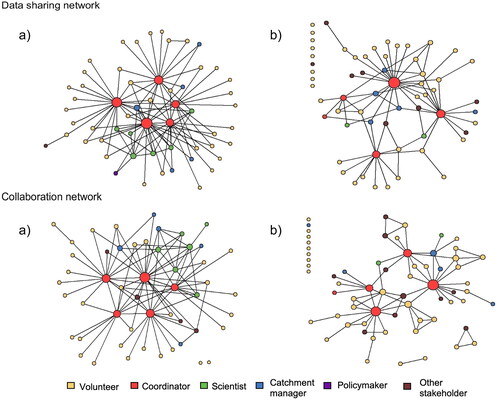
Table 3. Actor diversity in data sharing and collaboration networks.
The Mill Stream data sharing and collaboration networks were more loosely connected than Regional Highlands (). Mill Stream Waterwatch had lower densities and average number of relations (i.e., “edges”) per node (referred to as “degrees”), indicating fewer overall ties connecting actors. Further, Mill Stream Waterwatch was more fragmented, displaying a greater number of isolates and components. By contrast, the Regional Highlands Waterwatch program was fully formed in the data sharing network while only two isolates were present in the collaboration network.
Network centralization scores for Regional Highlands Waterwatch and Mill Stream Waterwatch were similar at 40% and 35%, respectively (). This means data are only moderately shared across a few central individuals, compared to a typical star network with a centralization index of 100%. However, for the collaboration network, Regional Highlands Waterwatch was much more centralized than Mill Stream Waterwatch at 42% and 20%, respectively, suggesting collaborative ties are more dispersed and evenly spread in the Mill Stream case.
Table 4. Whole-network properties of data sharing and collaboration network in Regional Highlands Waterwatch and Mill Stream Waterwatch.
A further measure of structural centralization was the assessment of the core-periphery profile of each program. The Regional Highlands case showed a strong core-periphery structure across both the data sharing and collaborative network, with correlations of 73% and 70%, respectively ().
For the collaboration network, the cross-boundary character of Regional Highlands Waterwatch was slightly higher than Mill Stream Waterwatch of 39% and 33% respectively (). For the data sharing network, Regional Highlands Waterwatch had a much lower cross-boundary exchange than Mill Stream where 28% and 40% of total ties, respectively, were between actors holding different roles (). Results suggest in Mill Stream Waterwatch, data are shared with a greater variety of stakeholders than in the Regional Highlands case, explicable by the more dispersed nature of the Mill Stream Network.
Table 5. Total cross-boundary exchange and volunteer within/cross-boundary ties across each network.
In both study cases, volunteers had different types of connections. Volunteers in Regional Highlands Waterwatch had few ties with one another, suggesting they usually conduct activities alone, while volunteers in Mill Stream had a higher number of within-boundary (volunteer-volunteer) ties. For example, 27% of all ties in the Mill Stream collaboration network were between volunteers compared to 1% in the Regional Highlands case. In the Mill Stream case, volunteers were also more likely to connect with actors in other roles, with 13% of total ties in the data sharing network and 12% in the collaboration network.
Network Outcomes
To understand the effects of different network structures in citizen science, we consolidated instances in which data from both programs have been used for management and policy decisions based on secondary analysis of a previous study. places each instance of data uptake along two categorical axes representing the level and stage of the adaptive management process. Results show both programs have contributed data to inform policy and management action across each point in the adaptive management cycle. For example, Regional Highlands Waterwatch displayed more instances of data uptake at a regional scale whereas Mill Stream Waterwatch was more likely to demonstrate successful instances of data use at a local level. A description of each instance of data uptake is shown in Supplementary Material D.
Navigating Network Tensions
The quantitative findings described above are complemented by in-depth interviews conducted with a diverse set of people in each study case’s network. We conceptualized the emergent themes from the thematic analysis according to the network tensions identified by Provan and Kenis (Citation2007) to show how each program has prioritized or balanced different sides of each tension.
Efficiency versus Inclusiveness
The first tension described is between efficiency and inclusiveness, which refers to the challenge of acting effectively as a whole while encouraging inclusive and equitable participation. A key theme to emerge from the data was related to how their program’s goals shaped the types of relationships that were built and maintained. In the Regional Highlands case the program facilitator explained how the program structure was key to its efficiency:
I sit in the [government] but the four coordinators who work directly with the volunteers sit in the community. I feel this is a key to success as I can connect with policy makers and promote the program and its data while the coordinators can get on with their job and be more accessible to the public. (program facilitator, RHW)
Prioritizing one aspect of the efficiency-inclusivity tension can simultaneously reduce the other. In the Regional Highlands case, we found the prioritization of efficiency has simultaneously reduced the program’s ability to be inclusive of diverse volunteer needs and interests, in particular, by limiting the capacity of volunteers to decide on how they participate in the program. For some volunteers, the prescribed nature of monitoring activities restricted their ability to determine the course of their participation:
A lot of the testing dates, they would prefer you to do them on a special day…I can see that their data sets would be a lot better if everybody did their testing on a certain day…I just can’t commit to that. (volunteer, RHW)
This tension between efficiency and inclusiveness was also visible in selection of monitoring sites. The program facilitator described how several sites were still being supported despite their low scientific utility, yet telling volunteers their sites were not meaningful to the broader monitoring program was “not exactly going to win hearts and minds” (program facilitator, RHW). The continued support for such sites, however, appears to highlight how the program facilitator has attempted to find a balance between community ownership of monitoring sites (i.e., inclusiveness) and scientific rigor (i.e., efficiency).
In the Mill Stream case, the promotion of community-directed monitoring and local projects came at the expense of program efficiency. Program coordinators suggested they generally work on their own projects in their regions, constraining efficiency in delivering actions across a wider geographic scale. This finding is in line with the social network analysis described above which revealed no reported ties among coordinators, and few ties between coordinators and scientists, waterway managers or policymakers. While their approach was found to encourage the investigation of locally-defined issues, additional challenges reported by coordinators related to providing balanced resourcing of volunteer groups across the region.
Internal versus External Legitimacy
The second network tension is between internal and external legitimacy, or the responsiveness of the program to the potentially conflicting needs and expectations of internal participants, such as volunteers, and external actors, such as funding bodies and decision-makers. A key theme to emerge from our interview data was that the programs have taken different approaches in their efforts to contribute to natural resource management and policymaking, which revealed whose needs and expectations were being prioritized.
In the Regional Highlands case, a necessary task for the program has been to demonstrate scientific utility for decision-making. As described by one scientist, Regional Highlands Waterwatch “generally has struggled from the point of view that they’re not considered to be accurate, useful data, and just side-lined” (scientist, RHW). The program facilitator explained that delivering a robust monitoring program accepted externally by decision-makers, that could then inform policy, was critical to ensure the program remained functional:
Definitely that’s been my biggest driver…I could tell there was this real ambivalence to Waterwatch, that it was this thing that just kept going on and on and on and they couldn’t really see any clear tangible evidence of what they did and the effect they were having. (program facilitator, RHW)
I want [volunteers] to feel like they are still very much an independent person who is dealing with a coordinator in the field, that they’re not beholden to the [the government] and can’t speak their opinions. (program facilitator, RHW)
In Mill Stream Waterwatch, one coordinator commented that finding uses for citizen science data at a regional policy-making level within the funding agency was a “harder sell”, which the coordinator attributed due resistance from scientists and decision-makers: The program is written off by some [who say], “it’s citizen science; that’s less valuable than the other data being collected across the business” (coordinator, MSW).
Unlike Regional Highlands Waterwatch, demonstrating the external legitimacy of Mill Stream was difficult for the coordinators, in part, because they lacked a contact able to assist in connecting their programs with decision-makers within the funding agency:
I don’t have someone who has a lot of time internally to make that link between the data and the research that’s done by consultants. And then the potential with this to be a way to make data more meaningfully used. (coordinator, MSW)
Flexibility versus Stability
The final tension inherent to network governance is between flexibility and stability, which refers to being adaptable to changing conditions while maintaining consistent actions over time. Our interviews with participants revealed a key theme about divergence in the flexibility of each program, which impacted on their capacity to address environmental at different scales.
In Regional Highlands Waterwatch efforts to embed the program within government decision-making has enabled the program to maintain consistency in data collection and reporting activities. Despite this, the program facilitator acknowledged the centralized position they occupied was a risk to the program’s stability if they were no longer employed and this, therefore, required careful management:
I try to have processes in place that over-ride personalities so that if I left or if that other person left, that it isn’t just dependent on a good personality relationship. (program facilitator, RHW)
It can be frustrating constantly getting that data and seeing something that’s a problem and not being able to do anything about it; that’s not our job to take that data and turn that monitoring into action kind of thing, because we don’t really have the capacity to do that. (program facilitator, RHW)
A local resident started complaining about a bad smell in the lake and then we took samples and that’s when we realised that there was E. coli in the water. We sent that sample to [the funding agency] and after couple of days we understood that was there a sewage pipe had burst and then [the funding agency] sent some tankers of clean water to flush out that sewage water. And ultimately the problem was solved, but it was only because of the Waterwatch [Mill Stream] program that we were able to identify the issue and get rid of the pollutants and sewage water. (volunteer, MSW)
The information that is collected by Waterwatch [Mill Stream] wasn’t the key value [as perceived by the funding agency], but the volunteers saw that the information that they were collecting was the most important thing they were doing…I think that’s the risk with the program. (catchment manager, MSW)
Discussion
This study employed a comparative social network analysis to examine how differences between data sharing and collaborative relations in two freshwater citizen science projects shape program function and outcomes. The social network analysis showed that although programs were characterized by a similar diversity of actors, including government agencies, universities, community-based organizations, local councils and private businesses, they were found to have divergent social network structures across both studied relations. By connecting the social network analysis with secondary data of program impacts on decision-making and interviews with key actors, we find that social network structure played a significant role in both enabling and constraining possibilities for collaboration and knowledge outcomes in each program. Below, we discuss our findings in more detail, emphasizing how the “dual nature” of social networks can enable certain outcomes while limiting others.
In the Mill Stream case, the social network analysis revealed a decentralized network structure. Decentralized networks have been identified as being a preferred approach for complex and uncertain policy contexts since actors can understand and share information across different parts and scales of an environmental problem (Newig, Günther, and Pahl-Wostl Citation2010). However, a decentralized approach may also pose difficulties in coordinating the entire network to achieve collective aims (Bodin and Crona Citation2009). Our interviews with program coordinators generally supported these theoretical insights, which was further corroborated by evidence of the program’s contributions to decision-making. This analysis showed that Mill Stream Waterwatch actively encouraged the development of local network structures to enable the resolution of local waterway problems. At the same time, the program lacked the ability to influence decision-making at broader levels, as was evidenced by the lack of observed connections with scientists and decision-makers and limited reported instances of data uptake in regional management and policy.
In connecting our analysis to the three tensions of network governance (Provan and Kenis Citation2007), we argue that Mill Stream Waterwatch has placed greater importance on inclusiveness over efficiency, internal over external legitimacy, and flexibility over stability. Prioritizing these aspects to network governance appears to be crucial to the main functions of the program: to build local community leaders who take ownership of local waterways, to enable quick responses to heterogeneous environmental problems and to engage volunteers and catchment managers in short-term, locally relevant monitoring projects. Furthermore, the urban setting in which Mill Stream Waterwatch operates may necessitate these priorities as volunteers were often associated with local environmental groups focused on local environmental issues pertaining to urban areas, such as pollution events and restoration projects.
By contrast, the Regional Highlands Waterwatch program was characterized by a core-periphery network structure. Core-periphery networks with strong central leadership have been discussed as important in developing consensus on goals and values and can ease knowledge sharing (Newig, Günther, and Pahl-Wostl Citation2010). The qualitative analysis suggested that this network structure served an important role in coordinating activities and facilitating knowledge uptake for regional policy. This evidence of consistent data uptake at a regional level can be partially explained by the presence of a tightly connected group of scientists who actively collaborate with the programs and apply its data in regional policymaking settings. Yet, the qualitative insights also suggested that the program was less efficient at addressing and solving local environmental concerns when they arise, which may be due to the lack of local network structures observed in the social network analysis. Despite this, most volunteers we interviewed were more inclined to “contribute to the whole” by assisting scientists and decision-makers attend to the region’s waterway problems, potentially reinforcing the program’s centralized nature.
Regional Highlands Waterwatch was found to promote a form of network governance favoring efficiency over inclusiveness, external over internal legitimacy and stability over flexibility. These dimensions of network governance reflected the largely rural focus of activities and often diffuse nature of environmental problems in this part of the country, such as drought and the impacts of fire, which were well-suited to a broadscale monitoring approach. The continued functioning (or stability) of the program was dependent on the program demonstrating its scientific value (or external legitimacy) to scientists and decision-makers who are increasingly using citizen science data. As a result, the program operates from a strong foundation of central and regional coordination that promotes high efficiency of activities. However, the overly centralized nature of the program may be vulnerable to collapse due to the strong reliance on one or few individuals. We suggest this is a particular risk Regional Highlands Waterwatch, where data sharing and collaborative ties were strongly centered around the main program facilitator.
This study has provided valuable insights relating to the role of social network analysis in enhancing and strengthening network ties, and through this, program potential. Our findings extend previous research on the factors that drive successful citizen science-policy linkages. However, the design of the study had some limitations. While responses were captured from most actors in professional roles across the case studies, the response rate of volunteers was more limited due to the ethical considerations relating to direct contact. While this could have resulted in a narrow picture, sufficient interviews were undertaken to indicate a saturation point was reached where little new data was being offered by the final interviewees. A valuable lesson was learned relating to the importance of building relationships with research participants prior to implementing a social network study, which we found increased the willingness of individuals to participate (Borgatti, Everett, and Johnson Citation2013). A second limitation was the study’s cross-sectional nature capturing only a snapshot of network connections at a single point in time. Future research could adopt longitudinal studies to address this shortfall, which would provide valuable insight into how the patterns of relationships within a given citizen science program change over time. However, we note that such studies are difficult to achieve in real world settings. Nevertheless, the comparative nature in this study, and the use of mixed methods, provided a way to, at least partly, offset the negative effects of these two limitations (Hollstein Citation2010).
Conclusion
For researchers interested in the relationship between citizen science and environmental management, the complex reality of the practice and its possible benefits means there are many points of conceptual and theoretical departure. Previous research has tended to focus on specific scientific, technical, and programmatic explanations to understand and manage this relationship. While this literature has been invaluable in advancing the field, both theoretically and practically, it lacks a coherent account of how members of citizen science programs interact with one another to promote productive engagement and outcomes. For citizen science to be effectively integrated within natural resource management contexts, an understanding of the nature and complexity of social networks is crucial. Our study shows how actors in two freshwater citizen science programs are embedded in a social network structure, and how these structures shape program function and outcomes. It reveals that similar citizen science networks may create different network structures, which both enable and constrain collaboration and knowledge outcomes. This study has highlighted that the use of social network analysis provides an alternative perspective on barriers and enablers that shape the impact of citizen science in natural resource management contexts. Practically, this study highlights the importance for citizen science practitioners to adopt a networking mindset that take into consideration the functional relationships both within and external to their program.
Supplemental Material
Download MS Word (28.2 KB)Supplemental Material
Download MS Word (32.6 KB)Supplemental Material
Download MS Word (30.3 KB)Supplemental Material
Download MS Word (28.2 KB)Acknowledgements
The authors gratefully acknowledge the contributions of participants to this study. The manuscript has benefited from thoughtful comments by J. Reeves and A. Sexton, and from four anonymous reviewers.
Additional information
Funding
Notes
1 Pseudonyms have been used to protect the identity and confidentiality of programs and participants.
References
- Aceves-Bueno, E., A. S. Adeleye, D. Bradley, W. T. Brandt, P. Callery, M. Feraud, K. L. Garner, R. Gentry, Y. Huang, I. McCullough, et al. 2015. Citizen science as an approach for overcoming insufficient monitoring and inadequate stakeholder buy-in in adaptive management: Criteria and evidence. Ecosystems 18 (3):493–506. doi: 10.1007/s10021-015-9842-4.
- Baran, P. 1964. On distributed communications networks. IEEE Transactions on Communications 12 (1):1–9. doi: 10.1109/TCOM.1964.1088883.
- Bodin, O., and B. I. Crona. 2009. The role of social networks in natural resource governance: What relational patterns make a difference? Global Environmental Change 19 (3):366–74. doi: 10.1016/j.gloenvcha.2009.05.002.
- Bodin, Ö., and C. Prell. 2011. Social networks and natural resource management: Uncovering the social fabric of environmental governance. Cambridge, UK: Cambridge University Press.
- Bodin, Ö., B. Crona, and H. Ernstson. 2006. Social networks in natural resource management: What is there to learn from a structural perspective? Ecology and Society 11 (2):r2. doi: 10.5751/ES-01808-1102r02.
- Bolíbar, M. 2016. Macro, meso, micro: Broadening the ‘social’ of social network analysis with a mixed methods approach. Quality & Quantity 50 (5):2217–36. doi: 10.1007/s11135-015-0259-0.
- Borgatti, S. P. 2002. NetDraw. Harvard, MA: Analytic Technologies.
- Borgatti, S. P., A. Mehra, D. J. Brass, and G. Labianca. 2009. Network analysis in the social sciences. Science 323 (5916):892–5. doi: 10.1126/science.1165821.
- Borgatti, S. P., M. G. Everett, and J. C. Johnson. 2013. Analyzing social networks. Thousand Oaks, CA: Sage.
- Borgatti, S. P., M. G. Everett, and L. C. Freeman. 2002. UCINET for Windows: Software for social network analysis. Harvard, MA: Analytic Technologies. doi: 10.1111/j.1439-0310.2009.01613.x.
- Braun, V., V. Clarke, N. Hayfield, and G. Terry. 2019. Thematic analysis. In Handbook of research methods in health social sciences, ed. P. Liamputtong, 843–60. Singapore: Springer Singapore. doi: 10.1007/978-981-10-5251-4_103.
- Buckland-Nicks, A., H. Castleden, and C. Conrad. 2016. Aligning community-based water monitoring program designs with goals for enhanced environmental management. Journal of Science Communication 15 (03):A01–23. doi: 10.22323/2.15030201.
- Buytaert, W., Z. Zulkafli, S. Grainger, L. Acosta, T. C. Alemie, J. Bastiaensen, B. De Bièvre, J. Bhusal, J. Clark, A. Dewulf, et al. 2014. Citizen science in hydrology and water resources: Opportunities for knowledge generation, ecosystem service management, and sustainable development. Frontiers in Earth Science 2:1–21. doi: 10.3389/feart.2014.00026.
- Carr, G., G. Blöschl, and D. P. Loucks. 2012. Evaluating participation in water resource management: A review. Water Resources Research 48 (11):1–17. doi: 10.1029/2011WR011662.
- Chase, S. K., and A. Levine. 2018. Citizen science: Exploring the potential of natural resource monitoring programs to influence environmental attitudes and behaviors. Conservation Letters 11 (2):e12382–10. doi: 10.1111/conl.12382.
- Conrad, C. 2006. Towards meaningful community-based ecological monitoring in Nova Scotia: Where are we versus where we would like to be. Environments 34 (1):25–36.
- Conrad, C. C., and K. G. Hilchey. 2011. A review of citizen science and community-based environmental monitoring: Issues and opportunities. Environmental Monitoring and Assessment 176 (1–4):273–91. doi: 10.1007/s10661-010-1582-5.
- Diani, M., and D. McAdam. 2003. Social movements and networks: Relational approaches to collective action. New York: Oxford University Press.
- Doreian, P., and K. L. Woodard. 1992. Fixed list versus snowball selection of social networks. Social Science Research 21 (2):216–33. doi: 10.1016/0049-089X(92)90016-A.
- Farr, C. M., S. E. Reed, and L. Pejchar. 2018. Social network analysis identifies key participants in conservation development. Environmental Management 61 (5):732–40. doi: 10.1007/s00267-018-1017-6.
- Fereday, J., and E. Muir-Cochrane. 2006. Demonstrating rigor using thematic analysis: A hybrid approach of inductive and deductive coding and theme development. International Journal of Qualitative Methods 5 (1):80–92. [Database] doi: 10.1177/160940690600500107.
- Fernandez-Gimenez, M. E., H. L. Ballard, and V. E. Sturtevant. 2008. Adaptive management and social learning in collaborative and community-based monitoring: A study of five community-based forestry organizations in the western USA. Ecology and Society 13 (2):1–22. doi: 10.5751/ES-02400-130204.
- Fetters, M. D., L. A. Curry, and J. W. Creswell. 2013. Achieving integration in mixed methods designs-principles and practices. Health Services Research 48 (6pt2):2134–56. doi: 10.1111/1475-6773.12117.
- Floress, K., L. S. Prokopy, and S. B. Allred. 2011. It’s who you know: Social capital, social networks, and watershed groups. Society & Natural Resources 24 (9):871–86. doi: 10.1080/08941920903493926.
- Forrester, T. D., M. Baker, R. Costello, R. Kays, A. W. Parsons, and W. J. McShea. 2017. Creating advocates for mammal conservation through citizen science. Biological Conservation 208:98–105. doi: 10.1016/j.biocon.2016.06.025.
- Fuhse, J., and S. Mützel. 2011. Tackling connections, structure, and meaning in networks: Quantitative and qualitative methods in sociological network research. Quality & Quantity 45 (5):1067–89. doi: 10.1007/s11135-011-9492-3.
- Göbel, C., V. Y. Martin, and M. Ramírez-Andreotta. 2016. Stakeholder analysis: International citizen science stakeholder analysis on data interoperability final report. Woodrow Wilson International Center for Scholars, Washington, DC.
- Groce, J. E., M. A. Farrelly, B. S. Jorgensen, and C. N. Cook. 2019. Using social-network research to improve outcomes in natural resource management. Conservation Biology 33 (1):53–65. doi: 10.1111/cobi.13127.
- Hecker, S., R. Bonney, M. Haklay, F. Hölker, H. Hofer, C. Goebel, M. Gold, Z. Makuch, M. Ponti, A. Richter, et al. 2018. Innovation in citizen science – Perspectives on science-policy advances. Citizen Science: Theory and Practice 3 (1):1–14. doi: 10.5334/cstp.114.
- Hollstein, B. 2010. Qualitative approaches. In Sage handbook of social network analysis, ed. J. Scott and P. Carrington, 404–16. London: Sage Publications Ltd., .
- Hollstein, B. 2014. Mixed methods social networks research: An introduction. In Mixed methods social networks research: Design and applications, ed. B. Hollstein and S. Dominguez, 3–34. Cambridge, UK: Cambridge University Press.
- Innes, J. E., and D. E. Booher. 2004. Reframing public participation: Strategies for the 21st century. Planning Theory & Practice 5 (4):419–36. doi: 10.1080/1464935042000293170.
- Irwin, A. 2015. Citizen science and scientific citizenship: same words, different meanings? In Science communication today – 2015, ed. B. Schiele, J. L. Marec, and P. Baranger, 29–38. Nancy: Presses Universitaires de Nancy.
- Jalbert, K., A. J. Kinchy, and S. L. Perry. 2014. Civil society research and Marcellus Shale natural gas development: Results of a survey of volunteer water monitoring organizations. Journal of Environmental Studies and Sciences 4 (1):78–86. doi: 10.1007/s13412-013-0155-7.
- Jones, G. 2005. Is the management plan achieving its objectives? Case study. In Protected Area Management. Principles and Practice, ed. G. Worboys, T. De Lacy, and M. Lockwood. Oxford: Oxford University Press.
- Latimore, J. A., and P. J. Steen. 2014. Integrating freshwater science and local management through volunteer monitoring partnerships: the Michigan Clean Water Corps. Freshwater Science 33 (2):686–92. doi: 10.1086/676118.
- Leach, W. D., and P. A. Sabatier. 2005. Are trust and social capital the keys to success? Watershed partnerships in California and Washington. In Swimming upstream: Collaborative approaches to watershed management, ed. P. A. Sabatier, W. Focht, M. Lubell, Z. Trachtenberg, A. Vedlitz, and M. Matlock, 233–58. Cambridge MA: MIT Press.
- Marin, A., and B. Wellman. 2011. Social network analysis: An introduction. In The SAGE handbook of social network analysis, ed. J. Scott and P. Carrington, 11–25. London: SAGE Publications. doi: 10.4135/9781446294413.
- McGreavy, B., A. J. K. Calhoun, J. Jansujwicz, and V. Levesque. 2016. Citizen science and natural resource governance: Program design for vernal pool policy innovation. Ecology and Society 21 (2):48. doi: 10.5751/ES-08437-210248.
- McKinley, D. C., H. Brown, K. F. Stepenuck, J. F. Weltzin, A. Wiggins, O. D. Boyle, R. D. Briggs, S. F. Chapin, D. A. Hewitt, P. W. Preuss, et al. 2017. Citizen science can improve conservation science, natural resource management, and environmental protection. Biological Conservation 208:15–28. doi: 10.1016/j.biocon.2016.05.015.
- Mills, M., G. G. van de Bunt, and J. de Bruijn. 2006. Comparative research. International Sociology 21 (5):619–31. doi: 10.1177/0268580906067833.
- Mills, M., J. G. Álvarez-Romero, K. Vance-Borland, P. Cohen, R. L. Pressey, A. M. Guerrero, and H. Ernstson. 2014. Linking regional planning and local action: Towards using social network analysis in systematic conservation planning. Biological Conservation 169:6–13. doi: 10.1016/j.biocon.2013.10.015.
- Nerbonne, J. F., and K. C. Nelson. 2008. Volunteer macroinvertebrate monitoring: Tensions among group goals, data quality, and outcomes. Environmental Management 42 (3):470–9. doi: 10.1007/s00267-008-9103-9.
- Newig, J., D. Günther, and C. Pahl-Wostl. 2010. Synapses in the network: Learning in governance networks in the context of environmental management. Ecology and Society 15 (4):24. doi: 10.1197/jamia.M2385.
- Newman, G., M. Chandler, M. Clyde, B. McGreavy, M. Haklay, H. Ballard, S. Gray, R. Scarpino, R. Hauptfeld, D. Mellor, et al. 2017. Leveraging the power of place in citizen science for effective conservation decision making. Biological Conservation 208:55–64. doi: 10.1016/j.biocon.2016.07.019.
- Newman, L., and A. Dale. 2005. Network structure, diversity, and proactive resilience building: A response to Tompkins and Adger. Ecology and Society 10 (1):r2. doi: 10.5751/ES-01396-1001r02.
- Overdevest, C., C. H. Orr, and K. Stepenuck. 2004. Volunteer stream monitoring and local participation in natural resource issues. Human Ecology Review 11 (2):177–85.
- Pollock, R. M., and G. S. Whitelaw. 2005. Community-based monitoring in support of local sustainability. Local Environment 10 (3):211–28. doi: 10.1080/13549830500075438.
- Prell, C., K. Hubacek, and M. Reed. 2009. Stakeholder analysis and social network analysis in natural resource management. Society & Natural Resources 22 (6):501–18. doi: 10.1080/08941920802199202.
- Provan, K. G., and P. Kenis. 2007. Modes of network governance: Structure, management, and effectiveness. Journal of Public Administration Research and Theory 18 (2):229–52. doi: 10.1093/jopart/mum015.
- Richter, A., J. Hauck, R. Feldmann, E. Kühn, A. Harpke, N. Hirneisen, A. Mahla, J. Settele, and A. Bonn. 2018. The social fabric of citizen science—Drivers for long-term engagement in the German butterfly monitoring scheme. Journal of Insect Conservation 22 (5):731–43. doi: 10.1007/s10841-018-0097-1.
- Robins, G. 2015. Doing social network research: Network-based research design for social scientists. London: SAGE Publications Ltd.
- Sandström, A., and C. Rova. 2010. Adaptive co-management networks: A comparative analysis of two fishery conservation areas in Sweden. Ecology and Society 15 (3):art14. doi: 10.5751/ES-03531-150314.
- Shirk, J. L., H. L. Ballard, C. C. Wilderman, T. Phillips, A. Wiggins, R. Jordan, E. McCallie, M. Minarchek, B. V. Lewenstein, M. E. Krasny, et al. 2012. Public participation in scientific research: A framework for deliberate design. Ecology and Society 17 (2):art29. doi: 10.5751/ES-04705-170229.
- Stepenuck, K. F., and K. D. Genskow. 2018. Traits of volunteer water monitoring programs that influence natural resource management and policy impacts. Society & Natural Resources 32:275–91. doi: 10.1080/08941920.2018.1511022.
- Wasserman, S., and K. Faust. 1994. Social network analysis: Methods and applications. Cambridge: Cambridge University Press. doi: 10.1525/ae.1997.24.1.219.
- Weston, S., and C. Conrad. 2015. Community-based water monitoring in nova scotia: Solutions for sustainable watershed management. Environment and Natural Resources Research 5 (2):1–13. doi: 10.5539/enrr.v5n2p1.
- White, K. B., Z. N. Resmondo, J. A. C. Jennings, L. M. Creel, and B. N. Kelly Pryor. 2022. A social network analysis of interorganisational collaboration: Efforts to improve social connectedness. Health & Social Care in the Community 30:e6067-e6079. doi: 10.1111/hsc.14044.
- Yin, R. K. 2013. Validity and generalization in future case study evaluations. Evaluation 19 (3):321–32. doi: 10.1177/1356389013497081.