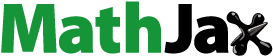
ABSTRACT
Variance components must be obtained to estimate genetic parameters and predict breeding values. In studies which take many traits into account, it is reasonable to use the Bayesian approach for the estimation of genetic parameters. The main goal of the present research was not only to consider the genetic correlations of the examined traits, but above all to estimate unknown genetic parameters and to gain profits from the selection. Bayesian inference was also useful for the selection of the best maize varieties. It was applied to predict genetic values in the multi-traits linear model. Thirteen maize cultivars representing the traits of our interest were studied by means of Bayesian inference. The traits are the number of plants before harvest, the grain yield, the length of the ears, the mass of leaves and the number of ears. The experiment involved a randomised block design with four replications and ten plants per plot. The highest correlation estimates were found between the number of plants before harvest and the number of ears, jointly with the grain yield and the number of ears. Lower correlation estimates were found between the length of the ears and the number of ears as well as the grain yield and the length of the ears. The research confirms that the best varieties to be grown are: Clarica, NK Cooler, Drim and PR 39K13. The Bayesian approach proved to be useful in selection studies, which can further be used to improve the studied genotypes.
Introduction
From 1 January 2014, pursuant to the 21 October 2009 Directive of the European Parliament and of the European Council establishing the framework for community action with regard to the use of pesticides (2009/128/CE), all professional users are obliged to observe the rules of integrated plant protection. The rules and guidelines for integrated plant protection specified in Annex III ‘General rules for integrated plant protection’ emphasise the application of all possible and available methods aimed at reducing the development of harmful organisms to a level of harmlessness. Integrated plant control emphasises the importance of research on plant diseases and breeding for resistance, as well as more extensive use of varieties resistant to diseases in production conditions (Piesik et al. Citation2007).
Maize (Zea mays L.), jointly with wheat and rice, is one of the main grain crops grown in the world. It is a widely used plant species and it forms the basis for agriculture in the European Union. As a valuable source of raw material for food, alcohol and chemical industries, it is also an excellent feed for animals (Aslam et al. Citation2011). Its future use may also include energy production. Furthermore, maize may be a remedy for climate change. It may prevent these changes thanks to its ability to intensively bind CO2 and to form yield using some amount of heat (Kim et al. Citation2007). Due to its origin, maize belongs to thermophilic plants (Szulc et al. Citation2017). Therefore, in the period of its growth and development, apart from appropriate agrotechnology, it requires more heat, compared to other cereal plants (Bos et al. Citation2000; Kim et al. Citation2007). The productivity of plants depends on the so-called photosynthetic production, that is, the amount of assimilates produced in the process of photosynthesis. It determines the biological yield of plants to a large extent, especially the potential to produce fresh or dry matter per unit area. In addition to the standard photosynthesis, characteristic of most species in our climatic zone, at higher air temperatures (above 25°C) and high light intensity, the maize launches the C4 assimilation track, doubling the photosynthetic efficiency. In one cycle, it connects 2 carbon dioxide molecules (Pengelly et al. Citation2011). Therefore, C4 plants, including maize, have an adaptive advantage over C3 plants, because the photodream loss in C3 plants increases with increasing temperature (Tausta et al. Citation2002). In other words, the maize uses light radiation and high air temperature very effectively, which translates into large increments of the leaf surface (the main photosynthetic apparatus). Varieties characterised by a high intensity of photosynthesis very often give lower yields than varieties showing a lower intensity of the process. It is due to the fact that two factors play an important role in the production of biomass: the intensity of photosynthesis and the size of the assimilation surface (leaves), in particular life expectancy and the period of capacity of assimilation bodies for effective photosynthesis (Szulc et al. Citation2012). Due to the unpredictability of weather conditions, one of the main challenges facing growers is to create maize hybrids capable of withstanding in environments featuring limited water resources. One solution is to breed plants characterised by greater health in a later period of vegetation or the ‘stay-green’ trait. This feature is an indicator of high plant health (Costa et al. Citation2002), reduced progressive aging and tolerance to drought after flowering. Greater durability (greenness of the leaf) or ‘stay-green’ trait are positively correlated with the yielding of plants (Osaki et al. Citation1993).
Finding the estimators of the variance components, predicting the breeding values and calculating the genetic parameters are the necessary conditions to propose new breeding strategies for maize (Gonçalves-Vidigal et al. Citation2008; Fritsche-Neto et al. Citation2010; Peiffer et al. Citation2013; Oliveira et al. Citation2014). In standard tests in which one feature is considered, the first step is to estimate unknown components of the variance. To achieve it (in a standard case), the least squares method or the standard maximum likelihood method is usually used. If the experiment is carried out with additional restrictions, the restricted maximum likelihood (REML) method is appropriate. In a situation where many traits are examined and many factors are considered, it is recommended to use Bayesian methods. Such methods allow not only for the calculation of breeding values and genetic parameters, such as genotypic variability coefficients and residual variance, heritability but also for the determination of the reliability intervals of the variance components and the genotypic correlation. In general, two or more traits are considered in maize studies. In such analyses, in order to find relationships between the tested traits, multivariate methods based on multifactorial models are used. In such a situation, Bayesian methods are very useful. Bayesian multi-trait models have become useful statistics method for plant and animal genetic evaluations. Many authors have shown that this model is more flexible and effective than the least squares method since it is not only based on the likelihood function, but it also allows a priori knowledge assumption when defining prior distribution (Hayashi and Iwata Citation2013). The Bayesian methods are often used to estimation of genetic parameters in different plants: barley (Li and Sillanpää Citation2012), Eucalyptus bosistoana (Davies et al. Citation2017), Eucalyptus cladocalyx (Cané-Retamales et al. Citation2011), jatropha (Junqueira et al. Citation2016), maize (Cuevas et al. Citation2017; Torres et al. Citation2018), soybean (Arriagada et al. Citation2012), wheat (Gianola et al. Citation2011; Cuevas et al. Citation2017).
The aim of the research presented in this paper was to apply Bayesian approach, involving a multi-trait linear model, to obtain estimates of the genetic parameters, to assess the genetic correlation between traits, to predict breeding values, and to select the best maize cultivars.
Material and methods
The experiment was carried out at the Department of Agronomy, the Poznań University of Life Sciences, in 2016 in the fields of the Agricultural Experimental Station in Swadzim (52°26′20″ N; 16°44′58″ E). Thirteen cultivars (NK Cooler, Delitop, Gazele, NK Ravello, ES Palazzo, ES Paroli, Clarica, PR 39 G 12, SY Cooky, Drim, SY Mascotte, ES Fortran and PR 39K 13) of fodder maize, grown for grain and purchased from different seed production companies, were compared. The selection of cultivars for the tests was not accidental. The cultivars were chosen mainly on the basis of the popularity of a given farm on the domestic market and the market share of breeders depending on the direction of cultivation. In the case of maize grain cultivation, the largest share in the sowing structure in 2018 was characterised by Pioneer varieties (20%), followed by Syngenty (12%) and Euralis (10%). The trend has been maintained for a long time in Poland. Therefore, selecting 13 maize cultivars coming from three breeding and seeding companies, which are the leaders on the domestic market in grain cultivation, should be considered as one of the novel elements of the study. The experiment was established in a complete randomised block design with four field replications and ten plants per plot. It was carried out in a typical grey-brown podzolic soil, composed of coarse sandy soil, shallowly deposited on light loam. The same NPK fertilisation was applied throughout the experimental field: 100 kg N ha−1 in the form of urea, 80 kg P2O5·ha−1 in the form of NPK-fertiliser (6% N), and 120 kg K2O·ha−1 in the form of 60% potassium salt. All the agricultural and cultivation measures were performed, following the guidelines of good agricultural practices. In each plant, the number of plants before harvest, the grain yield, the length of the ears, the mass of leaves and the number of ears were evaluated.
Statistical analyses were carried out using the plot mean. In this paper, we consider the multi-trait mixed model offered by Henderson and Quaas (Citation1976). Let vector yt represent the nt observations for trait t. Then, the multi-trait mixed linear model for n traits can be written as follows:where: Xi denotes the incidence matrix of the block effects associated with trait i, βi denotes the vector of fixed blocks effects summed to the overall mean associated with trait i, Zi denotes the incidence matrix of the genetic effect of each genotype for trait i, ui denotes is the vector of random effects of genotypes associated with trait i, ei denotes the vector of random residual effects associated with trait i. The assumptions on the distribution of y, u, and e are described as:
here, R and G denote covariance matrices associated with the vector e of residuals and vector u of random effects. If R0 (of order 5×5) is the residual covariance for the five traits, then R can be calculated as R = R0⊗I (‘⊗’ – the Kronecker product of two matrices, I – the identity matrix). Similarly, the genetic covariance matrix G can be calculated as G = G0⊗I. In the mixed model used, β is considered the vector of solutions for the systemic effects; however, from the Bayesian point of view, it is a vector of random effects in which the initial distribution values have uninformative priors; thus, they do not provide much information about the parameter and, therefore, have a uniform probability distribution (Everling et al. Citation2014).
Bayesian inference was used for estimating the variance components and predicting the breeding values of the accessions. The data analysis was carried out using Markov Chain Monte Carlo (MCMC) methods, through the Gibbs sampling algorithm. A Markov chain with 6,000,000 cycles was generated. It was considered a burn in of 300,000 cycles and a thinning interval of 3000 cycles, which ensured that the serial correlations were zero or very low. As a check criterion for convergence, the p-value of the Geweke test (Geweke Citation1992) was analysed. From the posterior distribution, the following parameters were calculated: the genetic variation coefficients: , the residual coefficients of variation:
, the variation index: Iv = CVg/CVe, the genotypic correlation coefficients:
, the heritability in the broad sense:
, and the gain with selection:
, where
denotes the genetic variance,
– the residual variance,
– the genetic covariance obtained by the multi-trait model,
– the trait overall mean, and
– the mean of the top 30% of individuals. For the variance components, e.g. genetic parameters and breeding values, we calculated the mean, median, mode and interval of higher density of posterior distribution (HPD). All the analyses were conducted using the GenStat v. 18 statistical software package.
Results
The p-value of the Geweke test was used as an indicator of convergence with the Markov Chains (). All the p-value estimates obtained in the Geweke test were lower than .05. Values closer to the mean, median and mode for the marginal posterior distribution of the parameters obtained were found. The highest heritability estimates were found for the mass of leaves and the number of ears (). The grain yield had the lowest heritability estimates. However, these estimates did not differ significantly from the credibility interval. The grain yield also had the lowest estimate for the genetic variation coefficient. This estimate did not significantly differ from that calculated for the number of plants before harvest. The number of ears and the mass of leaves presented the highest coefficient of genetic variation. The mode for the posterior distribution of the coefficient of environmental variation ranged from 8.79% for grain yield to 14.51% for the number of ears (). Only the grain yield and the number of ears showed significant differences in the estimates of the coefficient of environmental variation. Moreover, only in the case of the grain yield was the mode of the posterior distribution of the coefficient of relative variation lower than 1.00 (0.866). However, its estimates were not significantly lower than 1.00 according to the credibility interval (0.445, 1.416). The mass of leaves turned out the highest coefficient of relative variation, which differs significantly from the estimates found for the grain yield and the length of the ears ().
Table 1. Mean, median, mode, higher posterior density intervals (HPD), and p-value of the Geweke test for the posterior distribution of the estimates of heritability, coefficient of genotypic variation (%), coefficient of residual variation (%), relative variation index, and genotypic correlation in maize cultivars.
Table 2. Mean, median, mode, higher posterior density intervals (HPD), and p-value of the Geweke test for the posterior distribution of the estimates of heritability for individual genotypes.
Table 3. Mean, median, mode, higher posterior density intervals (HPD), and p-value of the Geweke test for the posterior distribution of the estimates of coefficient of genotypic variation (%) for individual genotypes.
Table 4. Mean, median, mode, higher posterior density intervals (HPD), and p-value of the Geweke test for the posterior distribution of the estimates of coefficient of residual variation (%) for individual genotypes.
Table 5. Mean, median, mode, higher posterior density intervals (HPD), and p-value of the Geweke test for the posterior distribution of the estimates of relative variation index for individual genotypes.
Table 6. Mean, median, mode, higher posterior density intervals (HPD), and p-value of the Geweke test for the posterior distribution of the estimates of genotypic correlation for individual genotypes.
The highest correlation estimates were found between (1) the number of plants before harvest and the number of ears (0.826), and (2) the grain yield and the number of ears (0.755) (). Lower correlation estimates were found between the length of the ears and the number of ears (−0.100) as well as the grain yield and the length of the ears (−0.170), which were not significantly different from zero.
The cultivars Clarica and Drim presented the highest stem diameters, which were significantly different only from the ES Palazzo and ES Paroli, according to the credibility interval. Cultivar ES Palazzo had the lowest grain yield, which was not significantly different from other cultivars. The cultivar with the lowest length of the ears was Gazele, and it did not significantly differ from the Clarica and PR 39K 13 cultivars. For the mass of leaves, the best results were found for the NK Cooler cultivars. The highest number of ears was observed for PR 39 G 12, and this value was significantly different from the number of ears for ES Palazzo, ES Paroli and Gazele cultivars. The genetic values for individual cultivars were presented in : estimates of heritability (), coefficient of genotypic variation (), coefficient of residual variation (), relative variation index (), and genotypic correlation ().
The grain yield presented the lowest gain with selection estimates. For this trait, the mode of the posterior distribution was −15%. The other traits turned out higher estimates for gain with the selection. The number of ears, the mass of leaves and the length of the ears had higher estimates of gain with the selection.
Discussion
The obtained asymmetric credible intervals for the variance components, breeding values and genetics parameters are a peculiarity of Bayesian inference. They make this approach notably informative (Mathew et al. Citation2012) and facilitative of hypothesis testing. Asymmetric credibility intervals obtained using posterior distribution, and shown in , make the conclusions more realistic than those based on symmetric confidence intervals of frequentist statistics (Apiolaza et al. Citation2011). Additionally, Bayesian inference allows the evaluation of unbalanced experiments and the study of more complex statistical models (Bink et al. Citation2008). Consequently, its use has become more common among breeders not only for the analysis of molecular data but also for phenotypic data (Kärkkäinen and Sillanpää Citation2012; Taylor and Friesen Citation2012). The results of the Geweke test presented in showed the reliability of the results for all analysed parameters. Therefore, it is reasonable to posit that the samples are truly representative in the underlying stationary distribution of the Markov chain (Cowles and Carlin Citation1996).
As has been shown in , the lowest heritability estimate found for the grain yield is the consequence of the lowest genotypic variation because the residual variation was low when compared with the other traits (). The grain yield also turned out the lowest estimate of genetic variation, which indicates a difficulty for the improvement of grain yield in this set of cultivars. However, there was an overlap of the credibility intervals between the traits with regard to the heritability and the coefficient of relative variation (). This outcome indicates that these estimates were not significantly different. The number of plants before harvest is not only influenced by genetic factors but also by environment effects, such as the weather conditions of the growing season (Synowiec et al. Citation2017). The reduction in the length of the ears is advantageous because it reduces the need for cultural practices. From a commercial point of view, another advantage of a lower length of the ears is the reduction of the potential for vegetative propagation of the plant, which can increase the continuous sale of seeds.
The correlation study turned out favourable associations for the breeding process between (1) the number of plants before harvest and the grain yield, (2) the number of plants before harvest and the mass of leaves as well as (3) the number of plants before harvest and the number of ears. This result indicates that the selection of genotypes characterised by a larger number of plants before harvest can provide a reduction in grain yield and increase the mass of leaves and the number of ears. The selection of genotypes with a lower grain yield may facilitate an increase in the mass of leaves and the number of ears, which is also desirable. However, the selection taking the lowest length of the ears into account can cause an increase in grain yield and reduce the mass of leaves and the number of ears, which is undesirable. According to Falconer and Mackay (Citation1996), the correlations between traits can be a result of pleiotropy or genetic linkage. Thus, if the undesirable correlations are caused by genetic linkage, these associations can be broken through recombination caused by crossing or self-fertilisation; consequently, these factors do not necessarily become major impediments to breeding programmes (Szulc and Bocianowski Citation2011b; Liersch et al. Citation2016; Radkowski et al. Citation2017). The increase in the maize yield is the result of a greater use of nitrogen fertilisers. On the other hand, lack of dose optimisation may have a huge negative impact on the natural environment. Therefore, it is necessary to have knowledge of the effectiveness of the fertiliser, with a simultaneous application of a crop control system (Erley et al. Citation2005). The improvement of agrotechnical applications of nitrogen fertilisers should always aim at increasing the use of the mineral fertiliser component (Szulc et al. Citation2016). On the other hand, breeding new varieties which more efficiently use nitrogen and, as a consequence, who feature a greater capability to absorb microelements from the soil and to manage the nitrogen collected, in synergy constitute a complementary strategy for managing the nutrient in the modern agriculture. Knowledge of genetics, physiology, as well as information about nitrogen collecting and utilisation, is indispensable in the process of breeding new varieties which are to be characterised by an effective nitrogen economy. The high population variability of maize, according to Paponov et al. (Citation2005), enhances chances of finding potent genotypes, thanks to which it is and will be possible to obtain varieties which produce efficiently, also under conditions of moderate stress of nitrogen deficiency. The observed large variation among maize genotypes in terms of nitrogen utilisation indicates that this characteristic is genetically determined and can be improved in the process of breeding. New crop varieties use the nitrogen better because their physiological features associated with the creation of the crop are comprehensively improved and corrected. The way in which plants use the accumulated nitrogen has a significant impact on the size of grain yield (Szulc and Bocianowski Citation2011a). It has been shown that a well-developed root system of maize is an important factor in the nitrogen uptake throughout the vegetation period of the plant. Therefore, the early creation of a large root system is crucial for increasing the efficiency of nitrogen uptake (Niu et al. Citation2007). Complementary action strategy comprises improving the efficiency of the use of nitrogen already collected by the plant, operationalised as using smaller amounts of nitrogen to produce as large a mass of assimilates as possible. When compared to hybrids which use nitrogen inefficiently, a hybrid well-managed with the component of nitrogen utilisation is characterised by a higher rate of photosynthesis during the pouring of kernels, even when the concentration of nitrogen in vegetative organs is comparable (Chen et al. Citation2006). The only possible explanation for this phenomenon is the increase in the efficiency of nitrogen transformation in the genotype. According to Hirel et al. (Citation2007), increasing the absorption of solar radiation by plants grown on less soils indirectly increases the use of nitrogen. Furthermore, stay-green hybrids have a larger assimilation area than traditional varieties do (Subedi and Ma Citation2005). Therefore, it is possible indirectly to explain the greater use of nitrogen by the type of hybrids.
In addition to the commercial cultivars, the following genotypes are recommended to integrate into breeding programmes: Clarica because it is one of the genotypes with the largest grain yield; NK Cooler because it is one of the genotypes with the largest mass of leaves; Drim because it is one of the cultivars with the highest production of plants before harvest; and PR 39K 13 because it is one of the cultivars with the highest production of ears. The estimates of gain with selection found in this study indicate the potential of selection for the genetic improvement of the genotypes evaluated. The rules for integrated plant protection emphasise the application of all possible and available methods reducing the development of a population of harmful organisms to a level of harmlessness. Guidelines for integrated protection indicate the priority of using non-chemical methods in pests and diseases control before applying chemical treatments. One of the easiest methods of protecting plants from pests and diseases may be using resistant plant varieties, therefore understanding resistance and adaptation in crop varieties is of great importance. All disturbances in the system of environmental factors cause an imbalance in the plant life activities, becoming the cause of stress. Maize yields are limited by a number of abiotic and biotic factors. Hence, crop production may fail to meet the objectives without introducing technological solutions required by the new varieties. The main reasons for the low level of maize yield are due to too acidic soils, insufficient supply of nutrients, as well as maladjustment of the fertilisation system to quantitative needs, and especially the dynamics of nutrient uptake by plants throughout the growing season. Periodic shortages of water in the soil (drought) during the growing season, limit the yield potential of new maize varieties. Due to unpredictable weather conditions, one of the main challenges for breeders is to create hybrids with features that give an advantage in an environment with limited water resources. Breeding works should be directed towards greater plant health in the later growing season or/and work on increasing the greenness of the leaf, known as ‘stay-green’. This feature is an indicator of high plant health, slower aging rate and tolerance to drought after flowering. The ‘stay-green’ feature shows a positive correlation with the yielding of plants. The conducted studies clearly showed that stay-green hybrids are definitely better compared to traditional varieties.
Disclosure statement
No potential conflict of interest was reported by the authors.
Notes on contributors
Jan Bocianowski has an M.Sc. in mathematics awarded in 1998; he achieved a Ph.D. in agriculture six years later in 2004. He is an interdisciplinary researcher, specialising in biometry and statistics for agriculture, plant breeding, biology and genetics. Jan has authored or co-authored over 300 scientific publications, of which around 150 were published in journals listed in Journal Citation Reports by Thompson Reuters.
Kamila Nowosad achieved a Ph.D. in agriculture in 2009. Her main research area is plant genetics, agrobiotechnology and plant breeding.
Piotr Szulc obtained his master's degree in 1998. He obtained the Ph.D. degree in 2003. Since 2017 he has held the position of associate professor at the Department of Agronomy of the Poznan, University of Life Sciences. His research interests mainly cover agrotechnics of agricultural plants with particular emphasis on cereal plants. He is the author of many scientific publications on national and international coverage.
Anna Tratwal achieved a Ph.D. in agriculture in 2006, since 2015 she has held the position of associate professor of the Institute of Plant Protection – National Research Institute. She is the author of about 170 scientific publications on national and international coverage. Within the framework of her professional activity she participate in seminars and post-graduate studies organised by IPP – NRI for the employees of National Health and Seed Inspection Service, Agricultural Advisory Center, agricultural producers and other parties interested in issues of signalling, forecasting and methods of monitoring of pests of cultivated plants.
Ewa Bakinowska has an M.Sc. in mathematics awarded in 1995; she achieved a Ph.D. in agriculture nine years later in 2004. She is an interdisciplinary researcher, specialising in statistics and biometry for agriculture, plant breeding and soil science. In the area of her scientific activities are, in particular, statistical analyses of categorical data using the logistic models. She is the author of many scientific publications on national and international coverage.
Dariusz Piesik graduated master's degree in 1995. He obtained the Ph.D. degree in 2000 and D.Sc. in 2009. Since 2018 he received the title of full professor. His research interests mainly cover pests of agricultural plants, volatile organic compounds released as consequence of abiotic and biotic stress, communication between plants. He is an author of many scientific publications on a global scale.
ORCID
Jan Bocianowski http://orcid.org/0000-0002-0102-0084
Kamila Nowosad http://orcid.org/0000-0001-6837-7806
Piotr Szulc http://orcid.org/0000-0002-9670-3231
Anna Tratwal http://orcid.org/0000-0001-9611-8799
Ewa Bakinowska http://orcid.org/0000-0002-1211-5046
Dariusz Piesik http://orcid.org/0000-0003-4192-9783
References
- Apiolaza LA, Chauhan SS, Walker JCF. 2011. Genetic control of very early compression and opposite wood in Pinus radiata and its implications for selection. Tree Genet Genomes. 7(3):563–571. doi: 10.1007/s11295-010-0356-0
- Arriagada O, Mora F, Dellarosa JC, Ferreira MFS, Cervigni GDL, Schuster I. 2012. Bayesian mapping of quantitative trait loci (QTL) controlling soybean cyst nematode resistant. Euphytica. 186:907–917. doi: 10.1007/s10681-012-0696-y
- Aslam M, Iqbal A, Ibni Zamir MS, Mubeen M, Amin M. 2011. Effect of different nitrogen levels and seed rates on yield and quality of maize fodder. Crop Environ. 2(2):47–51.
- Bink MCAM, Boer MP, ter Braak CJF, Jansen J, Voorrips RE, van de Weg WE. 2008. Bayesian analysis of complex traits in pedigreed plant populations. Euphytica. 161(1-2):85–96. doi: 10.1007/s10681-007-9516-1
- Bos HJ, Tijani-Eniola H, Struik PC. 2000. Morphological analysis of leaf growth of maize: responses to temperature and light intensity. Neth J Agr Sci. 48:181–198.
- Cané-Retamales C, Mora F, Vargas-Reeve F, Perret S, Contreras-Soto R. 2011. Bayesian threshold analysis of breeding values, genetic correlation and heritability of flowering intensity in Eucalyptus cladocalyx under arid conditions. Euphytica. 178:177–183. doi: 10.1007/s10681-010-0292-y
- Chen F, Chun L, Bao J, Zhang F, Mi GH. 2006. Vegetative growth and photosynthetic characteristics of maize hybrids differing in nitrogen use efficiency. J Maize Sci. 14:127–130.
- Costa CL, Dwyer LM, Stewart DW, Smith DL. 2002. Nitrogen effect on kernel yield and yield components of leafy and non-leafy maize genotypes. Crop Sci. 42:1556–1563. doi: 10.2135/cropsci2002.1556
- Cowles MK, Carlin BP. 1996. Markov chain Monte Carlo convergence diagnostics: a comparative review. J Am Stat Assoc. 91(434):883–904. doi: 10.1080/01621459.1996.10476956
- Cuevas J, Crossa J, Montesinos-López OA, Burgueño J, Pérez-Rodríguez P, de los Campos G. 2017. Bayesian genomic prediction with genotype ( environment interaction kernel models. G3-Genes Genom Genet. 7:41.
- Davies NT, Apiolaza LA, Sharma M. 2017. Heritability of growth strain in Eucalyptus bosistoana: a Bayesian approach with leftcensored data. New Zeal J For Sci. 47:5. doi: 10.1186/s40490-017-0086-2
- Erley GS, Kaul H, Krause M, Aufhammer W. 2005. Yield and nitrogen utilization efficiency of the pseudocereals amaranth, quinna, and buckwheat under differing nitrogen fertilization. Eur J Agron. 22:95–100. doi: 10.1016/j.eja.2003.11.002
- Everling DM, Bresolin T, Rorato PRN, Araujo RO, Boligon AA, Weber T, Dornelles MA, Campos LT. 2014. Finishing precocity visual score and genetic associations with growth traits in Angus beef cattle. Genet Mol Res. 13(3):7757–7765. doi: 10.4238/2014.September.26.13
- Falconer DS, Mackay TFC. 1996. Introduction to quantitative genetics. Edinburgh, SC: Addison Wesley Longman.
- Fritsche-Neto R, Gonçalves MC, Vencovsky R, de Souza Junior CL. 2010. Prediction of genotypic values of maize hybrids in unbalanced experiments. Crop Breed Appl Biot. 10:32–39. doi: 10.12702/1984-7033.v10n01a05
- Geweke J. 1992. Evaluating the accuracy of sampling-based approaches to the calculation of posterior moments. In: Bernardo JM, Berger JO, Dawid AP, Smith AFM, editors. Bayesian statistics 4. Oxford: Oxford University Press; p. 169–193.
- Gianola D, Okut H, Weigel KA, Rosa GJM. 2011. Predicting complex quantitative traits with Bayesian neural networks: a case study with Jersey cows and wheat. BMC Genet. 12:87–100. doi: 10.1186/1471-2156-12-87
- Gonçalves-Vidigal MC, Mora F, Bignotto TS, Munhoz REF, Souza LD. 2008. Heritability of quantitative traits in segregating common bean families using a Bayesian approach. Euphytica. 164(2):551–560. doi: 10.1007/s10681-008-9758-6
- Hayashi T, Iwata H. 2013. A Bayesian method and its variational approximation for prediction of genomic breeding values in multiple traits. BMC Bioinform. 14(1):34. doi: 10.1186/1471-2105-14-34
- Henderson CR, Quaas RL. 1976. Multiple trait evaluation using relatives records. J Anim Sci. 43(434):1188–1197. doi: 10.2527/jas1976.4361188x
- Hirel B, Le Gouis J, Gallais A. 2007. The challenge of improving nitrogen use efficiency in crop plants: towards a more central role for genetic variability and quantitative genetics within integrated approaches. J. Exp. Bot. 58(9):2369–2387. doi: 10.1093/jxb/erm097
- Junqueira VS, de Azevedo Peixoto L, Laviola BG, Bhering LL, Mendonça S, da Silveira Agostini Costa T, Antoniassi R. 2016. Bayesian multi-trait analysis reveals a useful tool to increase oil concentration and to decrease toxicity in jatropha curcas L. PLoS One. 11(6):e0157038. doi: 10.1371/journal.pone.0157038
- Kärkkäinen HP, Sillanpää MJ. 2012. Back to basics for Bayesian model building in genomic selection. Genetics. 191(3):969–987. doi: 10.1534/genetics.112.139014
- Kim SH, Gitz DC, Sicher RC, Baker JT, Timlin DJ, Reddy VR. 2007. Temperature dependence of growth, development, and photosynthesis in maize under elevated CO2. Environ Exp Bot. 61:24–236.
- Li Z, Sillanpää MJ. 2012. Estimation of quantitative trait locus effects with epistasis by variational Bayes algorithms. Genetics. 190(1):231–249. doi: 10.1534/genetics.111.134866
- Liersch A, Bocianowski J, Woś H, Szała L, Sosnowska K, Cegielska-Taras T, Nowosad K, Bartkowiak-Broda I. 2016. Assessment of genetic relationships in breeding lines and cultivars of Brassica napus and their implications for breeding winter oilseed rape. Crop Sci. 56(4):1540–1549. doi: 10.2135/cropsci2015.08.0530
- Mathew B, Bauer AM, Koistinen P, Reetz TC, Léon J, Sillanpaa MJ. 2012. Bayesian adaptive Markov chain Monte Carlo estimation of genetic parameters. Heredity. 109(4):235–245. doi: 10.1038/hdy.2012.35
- Niu J, Chen FJ, Mi GH, Li CJ, Zhang FS. 2007. Transpiration and nitrogen uptake and flow in two maize (Zea mays L.) inbred lines as affected by nitrogen supply. Ann Bot – London. 99:153–160. doi: 10.1093/aob/mcl237
- Oliveira EJ, Santana FA, Oliveira LA, Santos VS. 2014. Genetic parameters and prediction of genotypic values for root quality traits in cassava using REML/BLUP. Genet Mol Res. 13(3):6683–6700. doi: 10.4238/2014.August.28.13
- Osaki M, Fujisaki Y, Morikawa K, Matsumoto M, Shinano T, Tadano T. 1993. Productivity of high-yielding crops. IV. parameters determining differences of productivity among field crops. Soil Sci Plant Nutr. 39:605–615. doi: 10.1080/00380768.1993.10419177
- Paponov IA, Sambo P, Erley GS, Presterl T, Geiger HH, Engels C. 2005. Grain yield and kernel weight of two maize genotypes differing in nitrogen use efficiency at various levels on nitrogen and carbohydrate availability during flowering and grain filling. Plant Soil. 272:111–123. doi: 10.1007/s11104-004-4211-7
- Peiffer JA, Spor A, Koren O, Jin Z, Tringe SG, Dangl JL, Buckler ES, Ley RE. 2013. Diversity and heritability of the maize rhizosphere microbiome under field conditions. Proc Natl Acad Sci USA. 110(16):6548–6553. doi: 10.1073/pnas.1302837110
- Pengelly JL, Kwasny S, Bala S, Evans JR, Voznesenskaya EV, Koteyeva NK, Edwards GE, Furbanak RT, Caemmerer S. 2011. Functional analysis of corn husk photosynthesis. Am Soc Pl Biol. 156(2):503–513.
- Piesik D, Wenda-Piesik A, Weaver DK, Morrill WL. 2007. Influence of Fusarium crown rot disease on semiochemical production by wheat plants. J Phytopathol. 155:488–496. doi: 10.1111/j.1439-0434.2007.01266.x
- Radkowski A, Sosin-Bzducha E, Radkowska I. 2017. Effects of silicon foliar fertilization of meadow plants on the nutritional value of silage fed to dairy cows. J Elem. 22(4):1311–1322.
- Subedi KD, Ma BL. 2005. Nitrogen uptake and partitioning in stay-green and leafy maize hybrids. Crop Sci. 45:740–747. doi: 10.2135/cropsci2005.0740
- Synowiec A, Kalemba D, Drozdek E, Bocianowski J. 2017. Phytotoxic potential of essential oils from temperate climate plants against the germination of selected weeds and crops. J Pest Sci. 90:407–419. doi: 10.1007/s10340-016-0759-2
- Szulc P, Bocianowski J. 2011a. Hierarchy of mineral uptake in the creation of generative yield. Fresen Environ Bull. 20(8a):2135–2140.
- Szulc P, Bocianowski J. 2011b. Variability and correlation of grain yield structure components of two different types of maize cultivars (Zea mays L.). Fresen Environ Bull. 20(10):2684–2692.
- Szulc P, Bocianowski J, Rybus-Zając M. 2012. Accumulation of N, P, K and Mg nutrient elements and nutrient remobilization indices in the biomass of two contrasting maize (Zea mays L.) hybrids. Fresen Environ Bull. 21(8):2062–2071.
- Szulc P, Michalski T, Waligóra H, Wilczewska W. 2017. The dynamics of accumulation of N, P, K and Mg in the initial period of growth as a reaction of maize cultivars (Zea mays L.) on the method of the application of a nitrogen fertilizer. Fresen Environ Bull. 26(12):7189–7198.
- Szulc P, Waligóra H, Michalski T, Rybus-Zając M, Olejarski P. 2016. Efficiency of nitrogen fertilization based on the fertilizer application method and type of maize cultivar (Zea mays L.). Plant Soil Environ. 62(3):135–142. doi: 10.17221/654/2015-PSE
- Tausta SL, Coyle HM, Rothermel B, Stiefel V, Nelson T. 2002. Maize C4 and non-C4 NADP-dependence malic enzymes are encoded by distinct genes derived from a plastid-localized ancestor. Plant Mol Biol. 50(4–5):635–652. doi: 10.1023/A:1019998905615
- Taylor SA, Friesen VL. 2012. Use of molecular genetics for understanding seabird evolution, ecology and conservation. Mar Ecol Prog Ser. 451:285–304. doi: 10.3354/meps09694
- Torres LG, Rodrigues MC, Lima NL, Trindade TFH, Silva FFE, Azevedo CF, DeLima RO. 2018. Multi-trait multi-environment Bayesian model reveals G x E interaction for nitrogen use efficiency components in tropical maize. PLoS One. 13(6):e0199492. doi: 10.1371/journal.pone.0199492
- Viana JMS, Sobreira FM, De Resende MDV, Faria VR. 2010. Multi-trait BLUP in half-sib selection of annual crops. Plant Breed. 129(6):599–604. doi: 10.1111/j.1439-0523.2009.01745.x
- Waldmann P, Ericsson T. 2006. Comparison of REML and Gibbs sampling estimates of multi-trait genetic parameters in Scots pine. Theor Appl Genet. 112(8):1441–1451. doi: 10.1007/s00122-006-0246-x