Abstract
Cassia obtusifolia, belonging to legume family, is important in many fields with high pharmaceutical, economic, and ecological values. These interests of C. obtusifolia triggered in-depth and fundamental genetic and molecular research. Therefore, the stable reference gene is necessary for normalization of the gene expression studies. In this study, 10 candidate reference genes were subjected to expression analysis in 12 different tissues and under different stresses by qRT-PCR. The expression stability was evaluated using geNorm, NormFinder, and BestKeeper software. In conclusion, different suitable reference genes were selected in different tissues and under different stress. CYP1, EF1α2, ACT2, UBQ1 were the most stable reference genes in all samples. The relative expression levels of WRKY gene were detected to confirm the reliability of the selected reference genes. These results provided suitable reference genes that could be used for normalization in C. obtusifolia tissues and under different stress.
Graphical abstract
The relative transcript level of WRKY gene in 12 tissues and under different stress normalized by the selected reference genes
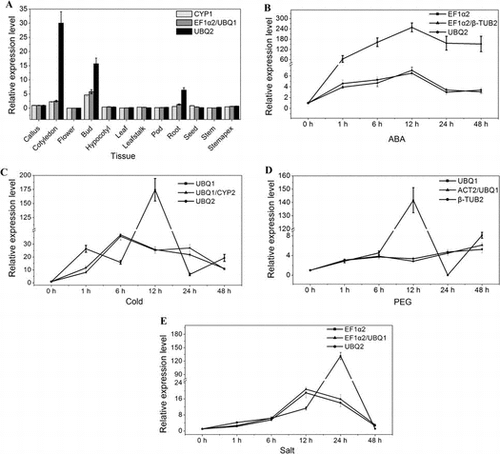
Gene expression analysis is fundamentally important in virtually all aspects of molecular biological research. In recent years, quantitative real-time PCR (qRT-PCR),Citation1) which has several advantages such as accuracy, sensitivity, and cost-effectiveness, has been the mostly preferred method for measurement and evaluation of gene expression levelCitation2) in animals, microbiology as well as plants. However, the results of qRT-PCR are inevitably impacted by different parameters such as amounts of plant material, quality and integrity of RNA, efficiency of reverse transcription, primer design, and PCR efficiency.Citation3) Additionally, random pipetting errors can add technical variability to the data.Citation4,5) Because of these reasons, it is critical to select stably expressed genes, called as reference genes, to normalize the expression levels of target genes.
The ideal reference genes should be stably expressed in all tissues under various conditions. They should not be influenced by the environmental change. Meanwhile, their expressing level should be relatively abundant and have minimal innate variability.Citation6) Commonly, reference genes have been chosen from housekeeping genes.Citation7,8) For example, Actin (ACT), Glyceraldehyde-3-phosphate dehydrogenase (GAPDH), Ubiquitin (UBQ), Tubulin (TUB), 18S rRNA (18S), translation elongation factor 1-α (EF1α), and cyclophilin (CYP1 and CYP2) are widely used in gene expression studies in Arabidopsis, Nicotiana tobacum, Oryza sativa, Cucumber Grown, Vitis amurensis, Triticum aestivum, and Glycine max,Citation9–15) etc. However, these genes have been shown to have variable expression levels across various plant species under different conditions.Citation16) Therefore, many studies have focused on reference gene selection among the several candidate reference genes in specific plant species under different conditions such as ArabidopsisCitation9), N. tobacumCitation10), O. sativaCitation11), C. GrownCitation12), etc. Because universal reference genes expressed at a constant level are not available, the selection of appropriate reference genes in specific plant species under different conditions is fundamental for the normalization of target gene expression levels.
Cassia obtusifolia, belonging to Leguminosae, is commonly distributed in China, India, and Korea. C. obtusifolia is important in pharmacy, landscaping, and textile chemicals with multiple economic and ecological values. It has been widely used as medicinal herb for the treatment of headache, dizziness, dysentery, and eye disease for centuries.Citation17) Several classes of bioactive metabolites have been identified from C. obtusifolia, including anthraquinone,Citation18) terpenoid,Citation19) flavonoid,Citation20) and lipid,Citation21) etc. According to the Chinese Pharmacopoeia, aurantio-obtusin,Citation22) a kind of anthraquinone, was identified as the characteristic bioactive component in C. obtusifolia and Cassia tora. Therefore, C. obtusifolia was regarded as a plant model for research of the biosynthesis of anthraquinone in higher plants. The extract and trypsin inhibitor from C. obtusiflia showed resistance to fungus and pests, respectively, suggesting these components were involved in the resistance to biotic stress.Citation23,24) Furthermore, C. obtusifolia had high content of nutritional components and was suggested to be a supplementary vegetable and food resource for human and animals.Citation25) Rapidly growing interests in C. obtusifolia triggered the establishment of a series of genomic resources. Recently, we obtained the transcriptome of C. obtusifolia seeds.Citation26) Several genes, which are relevant to formation of seed, the biosynthesis of bioactive metabolites and stress response, were screened from the transcriptome data. In the study, 10 candidate reference genes, including 1 each encoding elongation factor 1α (EF1α2) and β-Tubulin (β-TUB2), 2 genes encoding the actin (ACT1 and ACT2), cyclophilin (CYP1 and CYP2), Ubiquitin (UBQ1 and UBQ2), and α-Tubulin (α-TUB1 and α-TUB2), were chosen from the transcriptome data of C. obtusifolia. The expression stability of 10 genes above in 12 different plant tissues and under different stress conditions was analyzed using geNorm,Citation27) NormFinderCitation28) and BestKeeperCitation29) programs. After comprehensive analysis, the relative expression levels of WRKY, coding a member of WRKY transcription factor family from C. obtusifolia, were detected using the most stable reference gene and least stable reference gene, respectively, to confirm the reliability of the selected reference genes.
Materials and methods
Plant various tissues preparation
Mature seeds of C. obtusifolia were from Henan province, China. The seeds were cultivated in pots containing a soil mixtureCitation30) and grown at 25 °C with 16 h light/8 h dark. The leaf, leafstalk, root, stem, stemapex, and flower were collected from five-month-old plants. The nonage seed and pod were collected on day 30 after flowering.
The callus and bud were induced using the following procedures. Mature seeds were sterilized by soaking in a solution of 70% alcohol for 30 s and 0.1% mercury dichloride for 10 min with occasional shaking. Then, the sterilized seeds were rinsed three times with sterile double-distilled water and placed on standard MS culture medium in triangle bottles. The cotyledons and hypocotyls of seedlings were collected on day 7 after germination (DAG). The cotyledons were then used to induce callus and bud on inducing culture medium (standard MS culture medium supplemented with 0.4 mg/L 6-BA and 2.0 mg/L 2, 4-D).
Twelve tissues, including leaf, leafstalk, root, stem, stemapex, flower, nonage seed, pod, cotyledon, hypocotyl, callus, and bud, were collected and immediately frozen in liquid nitrogen and stored at −80 °C for RNA isolation in the future.
Growth hormones and stresses
The sterile seedlings at 7 DAG were used for the stress experiments. For cold treatment, seedlings were placed on MS culture medium at 4 °C for 0, 1, 6, 12, 24, and 48 h. For osmosis, salt and growth hormone treatments, the seedlings were placed on the MS culture medium supplemented with PEG 6000 (100 mg/mL), NaCl (200 mM), or with ABA (100 μM) for 0, 1, 6, 12, 24, and 48 h, respectively. The cotyledons of all samples were collected and frozen immediately in liquid nitrogen and then stored at −80 °C for RNA isolation in the future.
Total RNA isolation and cDNA preparation
Total RNA was extracted using the RNA plant plus reagent (Omega, US). RNA samples were treated with Dnase I (Takara, Japan) at a concentration of 1 unit/μg of total RNA to remove the DNA contaminates. RNA quantity and quality were determined using Nanodrop based on absorbance ratios at 260 nm/280 nm and 260 nm/230 nm. An aliquot of the RNA sample was checked on 1% agarose gel electrophoresis for RNA integrity by the appearance of ribosomal RNA bands and lack of degradation products. The primary cDNA was synthesized with the M-MLV reverse transcriptase kit using oligo-dT primers (Takara, Japan).
Primer design and standard curve analysis
The sequences of 10 candidate reference genes were obtained from our previous study of the transcriptome of the C. obtusifolia seed. The primary specific primers for these candidate reference genes were designed using the prime 3 program. The major parameters for primer design were set as follows: PCR products ranging from 100 to 300 nt; optimal melting temperature in a rage of 60–65 °C, and GC content from 40 to 60% with an optimum of 50%. The specificity of the primary primers was further confirmed by BLAST search. Then, the nucleotide sequence of the RT-PCR products for the 10 candidate reference genes were obtained using standard molecular cloning and sequencing procedures. Finally, the specificity of the qRT-PCR reactions was determined by melt curve analysis of the amplified products.
The amplification efficiency (E = 10slope) and correlation coefficients (R2 values) of the 10 candidate reference genes were calculated based on the slopes of the standard curves obtained from the serial dilution. This melt curve and efficiency experiments were repeated three times.
qRT-PCR amplification
The quantitative real-time PCR was performed using SYBR Premix Ex TaqTM II (Takara, Japan) with LightCycler 96 system (Roche Diagnostics, Mannheim, Germany). In each run, 1 μL cDNA template was added to 10 μL lreaction buffer using gene-specific primer pairs under the following conditions: pre-denaturation at 95 °C for 30 s, followed by 40 cycles of 10 s at 95 °C, 10 s at 60 °C, and 20 s at 72 °C. All qRT-PCR reactions were carried out in triplicate. The final cycle threshold (Ct) values were the mean of three values and calculated automatically by the LightCycler 96 software.
Expression stability of reference genes
The expression stability of each candidate reference gene was analyzed using geNorm, NormFinder, and BestKeeper programs, which have been widely used to screen reference gene for qRT-PCR. The Ct values, which were calculated by the LightCycler 96 software, were imported into the above software. The geNorm software provided expression stability (M values) of each candidate reference gene. The NormFinder software provided a rank of the candidate reference genes according to the expression stability. The BestKeeper software provided the average, standard deviation, and coefficient of variation of each candidate reference gene. Collectively, the analytic results from geNorm, NormFinder, and BestKeeper determined the most stable reference genes for qRT-PCR.
Expression level analysis of WRKY gene in various tissues and under different stress
In order to confirm the reliability of the validated candidate reference genes in qRT-PCR, the expression level of WRKY gene in 12 tissues and under cold, PEG, salt, and ABA treatment was determined using the most stable and least stable reference genes. The primers of WRKY gene were listed in Table . The comparative ΔΔCt-method was used to evaluate the relative quantities of each amplified products.
Table 1. Details of primers and parameters derived from qRT-PCR in this study.
Results
Selection of candidate reference genes
Extensive studies have revealed that housekeeping genes are widely applied as the reference genes for researches of plant gene expression in qRT-PCR. Based on the reference genes in other plantsCitation9–15) and the result of pre-screening (the pre-screening was introduced in Discussion), we chose 10 candidate reference genes including ACT1, ACT2, CYP1, CYP2, EF1α2, UBQ1, UBQ2, α-TUB1, α-TUB2, and β-TUB2. Details of these genes were shown in Table .
Amplification specificity and efficiency of the candidate reference genes
Prior to carrying out qRT-PCR reactions, the variability of RNA samples was evaluated by RT-PCR for 18S rRNA gene. All the RT-PCR reactions produced a single 18S rRNA specific band with a predicted molecular weight (approximately 260 bp) on agarose gel, confirming the extracted RNA samples were suitable for transcript level analysis (the primers used to amplify 18S rRNA were listed in the supplemental Supplemental Table S1). The specificity of amplification using the candidate primers was firstly examined by RT-PCR. All the RT-PCR reactions produced a single band in agrose gel electrophoresis (Supplemental Fig. S1A). The amplicons were subsequently sequenced and the sequences have been deposited in the GenBank (the sequences were also shown in Supplemental materials). Furthermore, the specificity of amplification was confirmed by the appearance of a single, dominant peak in the qRT-PCR melting curve analyses (Supplemental Fig. S1B).
Considering the ideal efficiency of qRT-PCR was required from 1.8 to 2.0, the efficiency of the qRT-PCR for the 10 candidate reference genes varied from 1.872 to 1.969. Based on the amplification specificity and efficiency, the reaction system in this study was quantified for the following studies.
Expression profile of the reference genes
The cycle threshold (Ct) value represented the cycle at which a significant increase of the qRT-PCR product and was widely used to identify stable expressed genes. Gene expression analyses of the 10 candidate reference genes exhibited a narrow mean Ct value in 12 different experimental tissues and under different stress (Fig. ). The Ct values ranged from 17 to 30, while most of them ranged from 20 to 23. Among all the candidate reference genes, EF1α2 showed the most abundant expression level with an average Ct value of 17.9, whereas ACT1 showed the least abundant expression level with an average Ct value of 29.5.
Expression stability analysis of the reference genes
Firstly, geNorm software was employed to evaluate the stability of candidate reference genes. This software automatically calculated the average expression stability value (M) of a particular gene compared with all other candidate reference genes. The gene with higher M value was less stably expressed, conversely, the gene with lower M value was more stably expressed and would make it as a good reference gene. The M values of 10 candidate reference genes in different tissues and under different stress were presented in Fig. . Most of the candidate reference genes, except UBQ2, in different tissues had high expression stability with relatively low M value of less than 1.0, which were below the default limit of M ≤ 1.5. The EF1α2/UBQ1 was identified as the most stable genes combination with the lowest M value of 0.336. These were followed by CYP1 (0.449), ACT2 (0.581), α-TUB1 (0.665), CYP2 (0.747), β-TUB2 (0.824), ACT1 (0.881), and α-TUB2 (0.932). In contrast, UBQ2 was the least stable gene with the highest M value of 1.06. Under cold stress, UBQ1/CYP2 was the most stable genes combination with the least M value of 0.449, while UBQ2 was the least stable gene with the high M value of 1.37. Under PEG treatment, genes combination ACT2/UBQ1 showed the most stable expression with the M value of 0.243, β-TUB2 showed the least stable expression with the M value of 2.42. Under salt treatment, the most stable gene was EF1α2/UBQ1 combination with the M value of 0.267, and the lowest stable gene was UBQ2 with an M value of 1.41. EF1α2 and β-TUB2 genes ranked highest with the M value of 0.285 under ABA treatment. When all samples in different tissues and under different stress were combined together, CYP1 and EF1α2 were the most stable genes with the M value of 0.461, while the UBQ2 was the least stable gene with the M value of 1.819.
Fig. 2. Average expression stability (M) values of candidate reference genes by geNorm.
Notes: (A) The M values calculated for all plant samples. (B) The M values calculated for 12 various tissues. (C) The M values calculated under ABA treatment. (D) The M values calculated under cold stress. (E) The M values calculated under PEG treatment. (F) The M values calculated under salt stress.
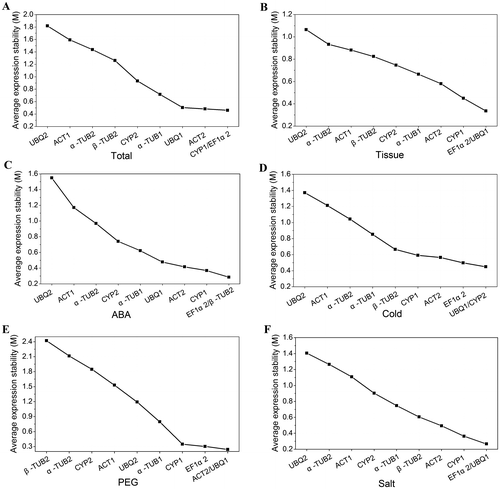
Meanwhile, geNorm software was used to calculate the pair-wise variation (Vn/Vn+1) between the sequential normalization factors NFn and NFn+1. According to the original description of geNorm, if the pair-wise variation was below the threshold of 0.15, the additional reference genes would be unnecessary. The result is showed in Fig. . The pair-wise variation V2/3 of 0.148, which was lower than 0.15, indicated that the combination of the two most stable reference genes was sufficient for gene normalization in different tissues. According to geNorm, the best combinations among the 10 candidate reference genes were EF1α2 and UBQ1 in different tissues (V2/3 = 0.148). All of the V2/3 value under different stress was less than 0.15 (Fig. ). The best combinations were UBQ1 and CYP2 under cold treatment (V2/3 = 0.139), ACT2 and UBQ1 under PEG treatment (V2/3 = 0.129), EF1α2 and UBQ1 under salt treatment (V2/3 = 0.116), EF1α2 and β-TUB2 under ABA treatment (V2/3 = 0.113).
Fig. 3. Pairwise variation analysis of the candidate reference genes.
Notes: Pairwise variation (Vn/Vn+1) was analyzed between the normalization factors NFn and NFn+1. Arrows indicated the optimal number of reference genes required for normalization.
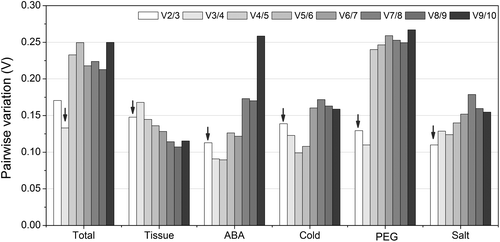
Then, the expression stability of 10 candidate reference genes was analyzed by NormFinder and the results were summarized in Table . Analysis of the NormFinder data showed that the ranking of the candidate reference genes was similar to that determined by geNorm program. We observed CYP1 (0.014), UBQ1 (0.024), and ACT2 (0.024) were the most stable genes in different tissues with the lowest stability value; EF1α2 (0.008), CYP2 (0.010), and UBQ1 (0.011) under cold stress; UBQ1 (0.007), CYP1 (0.010), and ACT2 (0.021) under PEG treatment; EF1α2 (0.007), CYP1 (0.024), and UBQ1 (0.025) under salt stress; CYP1 (0.011), β-TUB2 (0.011), and EF1α2 (0.012) under ABA treatment; and ACT2 (0.006), UBQ1 (0.017), and CYP1 (0.020) in all samples (Table ). Compared with the results from NormFinder with those from geForm, although there were some differences, the four most stable genes such as ACT2, UBQ1, CYP1, and EF1α2 in all samples were identical.
Table 2. Ranking of the candidate reference genes according to their stability estimated by NormFinder.
Furthermore, the BestKeeper analysis, which could determine the coefficient of variation (CV) and standard deviation (SD) using crossing point (CP) value of each candidate reference gene, was performed. According to the BestKeeper result, UBQ1, CYP1, and ACT2 were selected as most stably expressed genes in 12 different tissues; UBQ1, ACT2, and EF1α2 under cold stress; CYP1, EF1α2, and UBQ1 under PEG treatment; EF1α2, CYP1, and UBQ1 under salt stress; ACT2, β-TUB2, and EF1α2 under ABA treatment; and UBQ1, EF1α2, and CYP1 in all samples (Table ).
Table 3. Ranking of the candidate reference genes according to their stability calculated by BestKeeper.
Finally, the collective results from geNorm, NormFinder, and BestKeeper were compared to select the most stable genes and the least stable genes. In this procedure, we considered the top-ranked reference genes from each program firstly. For example, the three best genes in the geNorm program were EF1α2, UBQ1, and CYP1 in 12 tissues. CYP1, UBQ1, and ACT2 ranked at the top in the NormFinder and BestKeeper programs. Therefore, EF1α2, UBQ1, CYP1, and ACT2 were selected as the most stable reference genes for normalization of expression level in 12 tissues. Based on this criterion, the most stable genes and least stable gene(s) were presented in Table .
Table 4. Ranking of candidate reference gene stability according to geNorm, NormFinder, and BestKeeper.
Expression level analysis of WRKY gene in various tissues and under different stress
To demonstrate the reliability of the stable reference gene selected by the three programs in above study, the relative expression level of WRKY gene in 12 different tissues and under different stress was investigated. The most stable reference gene and gene combination (CYP1 and EF1α2/UBQ1 in 12 tissues, UBQ1 and UBQ1/CYP2 under cold stress, UBQ1 and ACT2/UBQ1 under PEG treatment, EF1α2 and EF1α2/UBQ1 under salt stress, EF1α2 and EF1α2/β-TUB2 under ABA treatment), and the least stable gene (UBQ2 in 12 tissues, under cold, salt and ABA treatment, β-TUB2 under PEG treatment) were used in this study. As shown in Fig. , if the CYP1 and the combination of EF1α2/UBQ1 were used as the reference gene(s), WRKY gene showed similar fluctuation pattern with the highest expression level in bud. However, if the least stable reference gene UBQ2 was used, WRKY had the highest expression level in cotyledon. The expression level of WRKY gene increased in the early stage and decreased after the top expression level appeared under cold, salt, and ABA treatment. When using UBQ1 and combination of UBQ1/CYP2 as the reference gene(s), the similar fluctuation pattern of WRKY gene was observed under cold stress. On the contrary, the expression pattern changed greatly if the least stable UBQ2 was used as reference gene (Fig. ). When using EF1α2 and EF1α2/UBQ1 as the reference gene(s) under salt stress, the highest expression level of WRKY gene appeared at 12 h, whereas the highest level appeared at 24 h with UBQ2 as the reference gene. Under ABA treatment, the highest expression level appeared simultaneously at 12 h when EF1α2, combination of EF1α2/β-TUB2, or UBQ2 were used. However, the expression level with UBQ2 as reference gene was far more than those with EF1α2 and EF1α2/β-TUB2 as reference gene(s). The transcript level of WRKY increased after PEG treatment with UBQ1 and ACT2/UBQ1 as reference gene(s), inversely, the transcript level of WRKY varied irregularly during the 48 h treatment. According to these results, the stable reference gene and gene combination selected in this study were reliable for qRT-PCR for target gene.
Fig. 4. The relative transcript level of WRKY gene in 12 tissues and under different stress normalized by the selected reference genes.
Notes: (A) The relative transcript level of WRKY gene in 12 tissues. (B) The relative transcript level of WRKY gene under ABA treatment. (C) The relative transcript level of WRKY gene under cold stress. (D) The relative transcript level of WRKY gene under PEG treatment. (E) The relative transcript level of WRKY gene under salt stress.
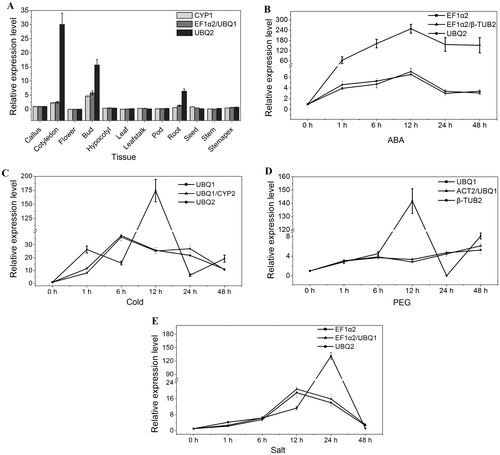
Discussion
Normalization is essential for obtaining accurate gene expression results from qRT-PCR experiments. Most of the gene expression studies generally used reference gene for normalization; therefore, reference gene choosing is the key for normalization. However, there was no ideal reference gene that could express stably in all plant species and under different conditions. The stability of reference gene was relative in various plant species and under different conditions. Therefore, it was necessary to validate the expression stability of the candidate reference gene for various plant species and under different conditions prior to its use for normalization.
In the beginning of the present study, we carried out a pre-screening. In the pre-screening, we chose 15 candidate reference genes including 14 genes from the transcriptome data and another 18S rRNA gene cloned by our lab previously. The 14 candidate reference genes, included the 10 genes used in the present study and other 5 genes such as glyceraldehyde-3-phosphate dehydrogenase (GAP1 and GAP2), EF1α1, UBQ1, and β-TUB1. We detected and analyzed the expression level of the 15 candidate genes in 12 tissues using geNorm, NormFinder, and BestKeeper. The results indicated that five genes (EF1α2, CYP1, UBQ1, ACT1, and ACT2) were most stable and five genes (UBQ2, α-TUB1, α-TUB2, β-TUB2, and CYP2) were comparatively stable. Therefore, the 10 genes were subsequently used in the present study. The primers information, Ct value, geForm, NormFinder, and BestKeeper results were presented in the supplemental materials (Supplemental Tables S1 and S2).
After the pre-screening, the expression of 10 potential candidate reference genes was compared in 12 different tissues and under different stress conditions. Although there were some differences in the results calculated by geNorm, NormFinder, and BestKeeper programs, the general trend was consistent (Fig. , Tables ). After the comprehensive analysis, the most and least stable reference genes were summarized. CYP1, EF1α2, ACT2, UBQ1 in all samples, EF1α2, UBQ1, CYP1, ACT2 in various tissues, UBQ1, CYP2, EF1α2 under cold stress, ACT2, UBQ1, CYP1, EF1α2 under PEG treatment, EF1α2, UBQ1, CYP1 under salt stress, EF1α2, β-TUB2, CYP1 under ABA treatment were the most stable genes, respectively. Interestingly, there was no single gene that can be ranked in the top three stable genes by three methods. EF1α2/UBQ1 in different tissues, UBQ1/CYP2 under cold stress, ACT2/UBQ1 under PEG treatment, EF1α2/UBQ1 under salt stress, and EF1α2/β-TUB2 under ABA treatment were the most stable dual combinations.
Finally, the relative expression levels of WRKY in different tissues and under different stress was detected and normalized by the selected most stable and least stable genes, respectively. The most stable gene and the gene combination showed consistent fluctuation patterns, whereas the least stable gene led to the inaccurate analysis results (Fig. ). The results indicated that the most stable gene and gene combination selected in this study would enable more accurate normalization and quantification of target gene expression in different C. obtusifolia tissues and under different stress. WRKYs were an important family of transcription factors in plants and played essential role in plant development and resistance to biotic and abiotic stress.Citation31,32) In our study, the sensitive response of WRKY to a variety of stresses suggested that it might be involved in the defense mechanism of C. obtusifolia.
Based on our knowledge, this is the first time to select and evaluate appropriate reference genes in C. obtusifolia. These results could be applied in molecular and genetic study in C. obtusifolia in future.
Author contribution
Zubi Liu and Qiankun Zhu took equal contribution to the work and were the performers of experiments and finishers of manuscript. Juanjuan Li, Jihua Yu, and Yangyang Li were assistors of the experiments. Xinhe Huang, Wanjun Wang, and Rui Tan provided some available comments for the manuscript. Jiayu Zhou and Hai Liao provided the instructions for performing experiments and writing manuscript, were responsible for the article.
Supplemental material
The supplemental material for this paper is available at http://dx.doi.org/10.1080/09168451.2015.1052771
Disclosure statement
No potential conflict of interest was reported by the authors.
Funding
This work was supported by the National Natural Science Foundation of China [grant number 31371232], the National Science and Technology Major Project of China [grant number 2014ZX09304307001-019], and the Fundamental Research Funds for the Central Universities of China [grant number 2682014RC14].
Supplemental Materials
Download MS Word (600.5 KB)References
- Heid CA, Stevens J, Livak KJ, Williams PM. Real time quantitative PCR. Genome Res. 1996;6:986–994.10.1101/gr.6.10.986
- Huggett J, Dheda K, Bustin S, Zumla A. Real-time RT-PCR normalisation strategies and considerations. Genes Immun. 2005;6:279–284.10.1038/sj.gene.6364190
- Andersen CL, Jensen JL, Ørntoft TF. Normalization of real-time quantitative reverse transcription-PCR data: a model-based variance estimation approach to identify genes suited for normalization, applied to bladder and colon cancer data sets. Cancer Res. 2004;64:5245–5250.10.1158/0008-5472.CAN-04-0496
- Bustin SA, Nolan T. Pitfalls of quantitative real-time reverse-transcription polymerase chain reaction. J. Biomol. Tech. 2004;15:155–166.
- Fleige S, Pfaffl MW. RNA integrity and the effect on the real-time qRT-PCR performance. Mol. Aspects Med. 2006;27:126–139.10.1016/j.mam.2005.12.003
- Radonić A, Thulke S, Mackay IM, Landt O, Siegert W, Nitsche A. Guideline to reference gene selection for quantitative real-time PCR. Biochem. Biophys. Res. Commun. 2004;313:856–862.10.1016/j.bbrc.2003.11.177
- Li Q-F, Sun SS, Yuan D-Y, Yu H-X, Gu M-H, Liu Q-Q. Validation of candidate reference genes for the accurate normalization of real-time quantitative RT-PCR data in rice during seed development. Plant Mol. Biol. Rep. 2010;28:49–57.10.1007/s11105-009-0124-1
- Chen L, Zhong H-y, Kuang J-f, Li J-g, Lu W-j, Chen J-y. Validation of reference genes for RT-qPCR studies of gene expression in banana fruit under different experimental conditions. Planta. 2011;234:377–390.10.1007/s00425-011-1410-3
- Czechowski T, Stitt M, Altmann T, Udvardi MK, Scheible W-R. Genome-wide identification and testing of superior reference genes for transcript normalization in Arabidopsis. Plant Physiol. 2005;139:5–17.10.1104/pp.105.063743
- Schmidt GW, Delaney SK. Stable internal reference genes for normalization of real-time RT-PCR in tobacco (Nicotiana tabacum) during development and abiotic stress. Mol. Genet. Genomics. 2010;283:233–241.10.1007/s00438-010-0511-1
- Narsai R, Ivanova A, NgS, Whelan J. Defining reference genes in Oryza sativa using organ, development, biotic and abiotic transcriptome datasets. BMC Plant Biol. 2010;10:56–65.10.1186/1471-2229-10-56
- Warzybok A, Migocka M. Reliable reference genes for normalization of gene expression in Cucumber Grown under different nitrogen nutrition. PLoS One. 2013;8:e72887.10.1371/journal.pone.0072887
- Reid KE, Olsson N, Schlosser J, Peng F, Lund ST. An optimized grapevine RNA isolation procedure and statistical determination of reference genes for real-time RT-PCR during berry development. BMC Plant Biol. 2006;6:27–36.10.1186/1471-2229-6-27
- Long X-Y, Wang J-R, Ouellet T, Rocheleau H, Wei Y-M, Pu Z-E, Jiang Q-T, Lan X-J, Zheng Y-L. Genome-wide identification and evaluation of novel internal control genes for Q-PCR based transcript normalization in wheat. Plant Mol. Biol. 2010;74:307–311.10.1007/s11103-010-9666-8
- Hu R, Fan C, Li H, Zhang Q, Fu Y-F. Evaluation of putative reference genes for gene expression normalization in soybean by quantitative real-time RT-PCR. BMC Mol. Biol. 2009;10:93–99.10.1186/1471-2199-10-93
- Barsalobres-Cavallari CF, Severino FE, Maluf MP, Maia IG. Identification of suitable internal control genes for expression studies in Coffea arabica under different experimental conditions. BMC Mol. Biol. 2009;10:1–10.10.1186/1471-2199-10-1
- Kim S-J, Kim K-W, Kim D-S, Kim M-C, Jeon Y-D, Kim S-G, Jung H-J, Jang H-J, Lee B-C, Chung W-S. The protective effect of Cassia obtusifolia on DSS-induced colitis. Am. J. Chin. Med. 2011;39:565–577.10.1142/S0192415X11009032
- Dave H, Ledwani L. A review on anthraquinones isolated from Cassia species and their applications. Indian J. Nat. Prod. Res. 2012;3:291–319.
- Sadiq I, Shuaibu M, Bello A, Isah STA, Izuagie T, Nasiru S, Kamaru M. Phytochemistry and antimicrobial activities of Cassia occidentalis used for herbal remedies. Methods. 2012;18:19–28.
- Singh D, Sharma S, Rani R, Mishra S, Sharma R. A new flavonoid and other two flavonoids isolated from different plant parts of selected Cassia species. Asian J. Res. Chem. 2011;4:818–821.
- Tzeng T-F, Lu H-J, Liou S-S, Chang CJ, Liu I-M. Reduction of lipid accumulation in white adipose tissues by Cassia tora (Leguminosae) seed extract is associated with AMPK activation. Food Chem. 2013;136:1086–1094.10.1016/j.foodchem.2012.09.017
- Dai, Y-c, Deng, N, Liu, W, Tan, B-y. Extraction, isolation and purification of aurantio-obtusin in Cassia seeds. Cent. South Pharm. 2011;3:1–7.
- Liao H, Ren W, Kang Z, Jiang J-H, Zhao X-J, Du L-F. A trypsin inhibitor from Cassia obtusifolia seeds: isolation, characterization and activity against Pieris rapae. Biotechnol. Lett. 2007;29:653–658.10.1007/s10529-006-9281-6
- Yang Y-C, Lim M-Y, Lee H-S. Emodin isolated from Cassia obtusifolia (Leguminosae) seed shows larvicidal activity against three mosquito species. J. Agric. Food Chem. 2003;51:7629–7631.10.1021/jf034727t
- Vandesompele J, De Preter K, Pattyn F, Poppe B, Van Roy N, De Paepe A, Speleman F. Accurate normalization of real-time quantitative RT-PCR data by geometric averaging of multiple internal control genes. Genome Biol. 2002;3:1–34.
- Liu Z, Song T, Zhu Q, Wang W, Zhou J, Liao H. De novo assembly and analysis of Cassia obtusifolia seed transcriptome to identify genes involved in the biosynthesis of active metabolites. Biosci. Biotechnol. Biochem. 2014;78:791–799.
- Schlotter YM, Veenhof EZ, Brinkhof B, Rutten VP, Spee B, Willemse T, Penning LC. A GeNorm algorithm-based selection of reference genes for quantitative real-time PCR in skin biopsies of healthy dogs and dogs with atopic dermatitis. Vet. Immunol. Immunopathol. 2009;129:115–118.10.1016/j.vetimm.2008.12.004
- Wang Q, Ishikawa T, Michiue T, Zhu B-L, Guan D-W, Maeda H. Stability of endogenous reference genes in postmortem human brains for normalization of quantitative real-time PCR data: comprehensive evaluation using geNorm, NormFinder, and BestKeeper. Int. J. Legal Med. 2012;126:943–952.10.1007/s00414-012-0774-7
- Pfaffl MW, Tichopad A, Prgomet C, Neuvians TP. Determination of stable housekeeping genes, differentially regulated target genes and sample integrity: BestKeeper—Excel-based tool using pair-wise correlations. Biotech. Lett. 2004;26:509–515.10.1023/B:BILE.0000019559.84305.47
- Al-Menaie H, Al-Ragam O, Al-Shatti A, Mathew M, Suresh N. Interactive effect of soil mixture and fertilizer concentration on the growth of Cassia seedlings. Eur. J. Sci. Res. 2013;94:61–66.
- Rushton PJ, Somssich IE, Ringler P, Shen QJ. WRKY transcription factors. Trends Plant Sci. 2010;15:247–258.10.1016/j.tplants.2010.02.006
- Chen L, Song Y, Li S, Zhang L, Zou C, Yu D. The role of WRKY transcription factors in plant abiotic stresses. Biochim. Biophys. Acta (BBA)—Gene Regul. Mech. 2012;1819:120–128.10.1016/j.bbagrm.2011.09.002