Abstract
In proteomics, more than 100,000 peptides are generated from the digestion of human cell lysates. Proteome samples have a broad dynamic range in protein abundance; therefore, it is critical to optimize various parameters of LC–ESI–MS/MS to comprehensively identify these peptides. However, there are many parameters for LC–ESI–MS/MS analysis. In this study, we applied definitive screening design to simultaneously optimize 14 parameters in the operation of monolithic capillary LC–ESI–MS/MS to increase the number of identified proteins and/or the average peak area of MS1. The simultaneous optimization enabled the determination of two-factor interactions between LC and MS. Finally, we found two parameter sets of monolithic capillary LC–ESI–MS/MS that increased the number of identified proteins by 8.1% or the average peak area of MS1 by 67%. The definitive screening design would be highly useful for high-throughput analysis of the best parameter set in LC–ESI–MS/MS systems.
Definitive screening design enables simultaneous optimization of LC-ESI-MS/MS parameters in proteomics.

Key words:
Proteome samples have a high complexity and broad dynamic range.Citation1–3 For example, in HeLa cell lysates, more than 8000 protein-encoding genes are expressed and some proteins have post-translational modifications.Citation4 Trypsin digestion generates more than 100,000 peptides and their abundance differs by 6 orders of magnitude.Citation5 Therefore, the separation and detection of such a complex peptide mixture are very difficult.
The optimization of each parameter of LC–ESI–MS/MS is essential for exerting the best performance to identify larger numbers of proteins.Citation6 Optimization has crucial effects; for example, in LC, appropriate selection of a column and gradient method is necessary to maximize the number of peptides eluted at a single time.Citation7 In ESI, an appropriate ESI spray voltage is required for a high ionization efficiency and low background. In MS, several parameters should be appropriately set for identifying eluting peptides.Citation7 Similar to these examples, the parameters of LC, ESI, and MS affect the number of identified proteins and peak area of MS1.Citation6,8 Hence, we attempted to optimize such parameters to identify larger numbers of proteins in non-targeted proteomics.
The Design of Experiments (DoE) could be a powerful tool to optimize combination of LC, ESI, and MS parameters. In several studies, the optimization of LC or MS has been performed by changing parameters one by one.Citation8,9 Actually, these studies optimized LC or MS parameters, but such an optimization procedure cannot detect two-factor interactions, which can be important to determine an optimal parameter set. To detect two-factor interactions, a recent study used DoE for optimizing MS parameters for non-targeted proteomics.Citation6 In that study, the authors adopted a fractional factorial design, which could detect two-factor interactions from approximately three parameters. Using the fractional factorial design, the authors systematically optimized MS parameters and increased the number of identified proteins, but they did not analyze LC parameters and two-factor interactions between LC and MS. Hence, in this study, we aimed to systematically optimize LC, ESI, and MS parameters using a novel DoE method.
For the systematic optimization of all LC, ESI, and MS parameters, we adopted a definitive screening design to optimize monolithic capillary LC–ESI–MS/MS parameters. The definitive screening design was recently developed by Jones et al.,Citation10 and it enables the identification of main factors and two-factor interactions from several parameters with less experiments. Here we report the optimization of monolithic capillary LC–ESI–MS/MS parameters using the definitive screening design.
Materials and methods
Sample preparation
Human whole K562 cell lysate was obtained from an MS compatible human protein extract, Intact (Promega, WI, USA). Reduction and alkylation were performed as described in the instruction manual, with a slight modification. Samples (400 μL of 2.5 μg/μL human protein extract) were reduced with 20 mM dithiothreitol (Nacalai Tesque, Kyoto, Japan) and incubated at 37 °C for 30 min. Further, the reduced proteins were alkylated with 60 mM iodoacetamide (Wako, Osaka, Japan) and incubated at room temperature for 30 min in the dark. Following the addition of 500 μL of 50 mM triethylammonium bicarbonate (TEAB) (Sigma-Aldrich, MO, USA), the proteins were digested to peptides by 20 μg sequencing grade trypsin (Promega) at 37 °C overnight. The protein concentration was adjusted to 1 μg/μL by the addition of 400 μL TEAB. The samples were desalted using MonoSpin C18 (GL Science, Tokyo, Japan) prior to analysis.
Monolithic capillary LC–ESI–MS/MS analysis
The parameters that were not considered as variables are shown in this section. All analyses were performed using an LC (Ultimate 3000®; Thermo Fisher Scientific Waltham, USA) equipped with a long monolithic silica capillary column (4200 mm, ID 0.1 mm), and ESI (XYZ-stage; AMR, Tokyo, Japan)–MS/MS (LTQ Orbitrap Velos Mass Spectrometer®; Thermo Fisher Scientific) system. A meter-scale monolithic silica capillary column has a higher separation capacity than a particle-packed column.Citation11–13 A gradient was achieved by changing the ratio of the following two eluents: eluent A, 0.1% (v/v) formic acid (Wako); eluent B, 80% acetonitrile (Wako) containing 0.1% (v/v) formic acid. The separated peptides were directly electrosprayed into the mass spectrometer using a Fortis tip (AMR).
The LTQ Orbitrap Velos was operated in a data-dependent analysis mode with a full scan range of 350–1500 m/z in the orbitrap and subsequent CID MS/MS scans in the linear ion trap. CID was performed at a normalized collision energy of 35%. Blank analyses were included between different settings.
Data analysis
Data analysis was performed using Proteome Discoverer 2.1 (Thermo Fisher Scientific). Protein identification was performed using the Mascot algorithm against the Homo sapiens protein database (20,172 sequences, version 2015-11-11) from UniProt, with a precursor mass tolerance of 100 ppm and a fragment ion mass tolerance of 0.8 Da. Carbamidomethylation of cysteine was set as a fixed modification, and the oxidation of methionine and acetylation of protein N-terminus were set as dynamic modifications. The data were then filtered with a cut-off criteria of q-value ≤0.01, corresponding to a 1% false discovery rate (FDR) on a spectral level. Proteins that had more than one unique peptide were accepted as identified proteins.
In calculating the peak area, we used PSMs based on Proteome Discoverer 2.1.Citation14 We used the Top 3 method for calculating the peak area.Citation15 In this method, peptides with the three highest signal intensities for each protein were summed, and the summed value was used as a peak area of MS1. The expected mass deviation was set as 4 ppm when the same peaks were repeatedly measured in consecutive scans.
Selection of parameters to be optimized
In previous papers, the number of identified proteins has been used as a response variable to optimize non-targeted proteomic analysis.Citation6,8,9 In this study, the average peak area of MS1 was used as a response variable in addition to the number of identified proteins. Because the peak area of MS1 is used for label-free quantitative proteome analysis, we thought that maximization of this value is required for high sensitivity analysis in label-free quantitative proteome analysis. To screen the possible parameters affecting the number of identified proteins and the average peak area of MS1, we chose 14 parameters as explanatory variables. These parameters were selected by referring to previous papers,Citation8,9,16 which optimized LC or MS instruments. In LC parameters, the linear velocity, gradient, injection amount, and gradient time were chosen as explanatory variables. In ESI parameters, the ESI spray voltage was chosen. In MS parameters, the maximum ion injection time (IT) of MS1/MS2, target value of auto gain control (AGC) in MS1/MS2, dynamic exclusion, resolution, number of MS2 scans, minimum count threshold, and capillary temperature were chosen. AGC is the upper limit of ions introduced into MS. IT is the higher limit of time for accumulating ions in MS. Dynamic exclusion is the time interval between selecting the same m/z for isolation and fragmentation. Minimum count threshold is the lower limit of ion intensity applied to MS2. The reasons for selecting these 14 parameters are described in Supplemental Table 1. We selected standard parameter from previous proteome analysis using monolithic capillary LC–ESI–MS/MS.Citation11
Definitive screening design
The definitive screening design was adopted using JMP 11(SAS Institute Inc., Cary, NC.).Citation10 The number of identified proteins and average peak area of MS1 were used to generate the outcomes of the definitive screening design. The sequence of analysis was randomized to reduce the experimental error caused by the consecutive sequence of similar settings. After obtaining the analysis results, data analysis was performed. We initially explored the effects of each explanatory variable and two-factor interactions by minimizing Akaike’s information criterion with a correction for finite sample sizes (AICc)Citation17 in a stepwise manner. By applying AICc for model selection, we could balance the complexity of the model and model fitness. Further, effects of the selected parameters were calculated using multiple regression analysis, and explanatory variables with significantly affecting effects (p-value <0.05) were identified.
Results and discussion
Experimental design
To prepare an experimental design for optimizing monolithic capillary LC, ESI and MS parameters, we adopted the definitive screening design based on JMP 11.Citation10 The settings used are shown in Table . As response variables, the number of identified proteins and average peak area of MS1 were chosen. The number of identified proteins has been generally used as a response variable to optimize non-targeted proteomic analysis in previous papers.Citation6,8,16 In contrast, the average peak area of MS1 has not been utilized as a response variable in non-targeted proteomics.Citation6,8,16 However, it is considered that optimization of the average peak area of MS1 is needed for current non-targeted proteomics. Recently, label-free quantification has been mainly employed for quantification in non-targeted proteomics.Citation15,18,19 In label-free quantification of protein abundance, MS peak intensities are summed, as described in the Materials and methods. Therefore, we tried to maximize the average peak area of MS1 to minimize ion suppression and reduce experimental error between analyses.
Table 1. Used parameters and values.
Fourteen parameters in Table were used to optimize combination of monolithic capillary LC, ESI, and MS to improve the number of identified proteins and average peak intensity. Among these parameters, resolution was considered as a categorical variable and other parameters were considered as continuous variables. In continuous variables, three levels (low, median, and high) were used, and in categorical variables, two levels (low and high) were used. Using these parameters, we adopted the definitive screening design for preparing the experimental design (Supplemental Table 2).
Parameters affecting response variables
We conducted all analyses, and the number of identified proteins and average peak area of MS1 of each setting are shown in Supplemental Table 3.
Along with the procedures mentioned in the Materials and methods, we explored explanatory variables and two-factor interactions, which affected response variables, by minimizing AICc in a stepwise manner. Following this, we calculated effects of the selected parameters using multiple regression analysis and acquired explanatory variables that significanlty affected the response variables (Fig. ). Furthermore, two-factor interactions between the explanatory variables selected in a stepwise manner are shown (Fig. ). In Figure , two-factor interaction plots with maximum response variables are shown.
Fig. 1. Effects of parameters on (a) the number of identified proteins and (b) average peak area of MS1.
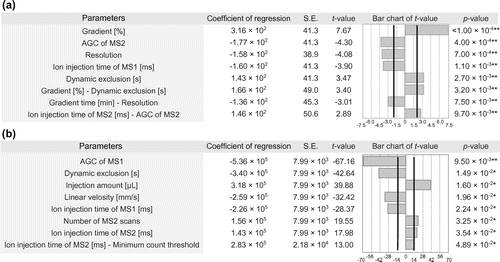
Fig. 2. Two-factor interaction plots of (a) the number of identified proteins and (b) average peak area of MS1.
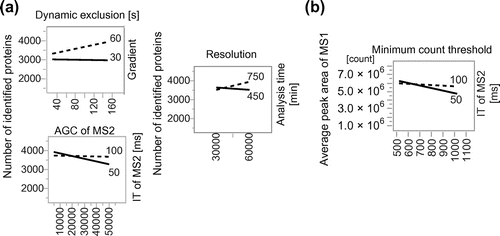
Fig. 3. (a) The number of proteins and (b) average peak area of MS1 obtained with optimized parameter sets.
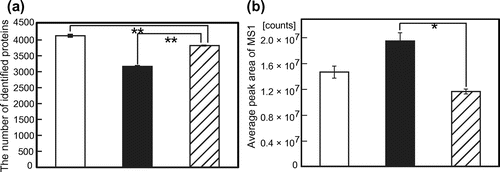
Contribution of possible effects of the parameters on increase in number of identified proteins
When the number of identified proteins was selected as a response variable, five main effects (gradient, AGC of MS2, resolution, ion injection time of MS1, and dynamic exclusion) and three two-factor interactions (gradient-dynamic exclusion, gradient time-resolution, and ion injection time of MS2-AGC of MS2) significantly affected the response variable.
‘Gradient’ had the most significant effect on the number of identified proteins. Larger numbers of proteins were identified in a 5–60% gradient than in other gradients. As shown in the previous report, the peak capacity became higher in the 5–60% gradientCitation20,21 and more peptides eluted per unit time. Furthermore, high concentrations of acetonitrile increased the ionization efficiency.Citation22 These previous observations explained the reason why a high level of gradient increased the number of identified proteins.
‘Smaller AGC of MS2’ significantly increased the number of identified proteins. No difference in the quality of the spectrum should be observed between AGCs at 5000 and 50000. Therefore, we could detect more peptides with faster scan speeds of AGC of MS2 at 5000, as described previously.Citation16
Further, a ‘higher resolution’ significantly increased the number of identified proteins. A high separation capacity of monolithic columns may not be sufficient for separating peptides with minor differences in the sequence and composition of amino acids. Then, a ‘high resolution’ may be required to detect more peptides with a high mass accuracy. In a previous report using Escherichia coli lysate as the analyte,Citation23 larger numbers of proteins were identified at a resolution of 30,000 than at a resolution of 60,000. In our analysis using human cell lysates, we identified larger number of proteins at a resolution of 60,000. Human produces more proteins than E. coli and therefore sample complexity become higher. Thus, we could identify more proteins in resolution of 60,000.
‘Shorter IT of MS1’ increased the number of identified proteins, probably because of a shorter scan time. Prior to the analysis, we had expected that a longer ion injection time made it possible to detect peptides around the limit of detection; however, a longer ion injection time did not increase the number of identified proteins because peptides with small ion intensities may not produce detectable MS spectra, as described previously.Citation8
A ‘longer dynamic exclusion’ increased the number of identified proteins. In a shorter dynamic exclusion time, peptides with the same m/z were detected multiple times in one analysis and the number of identified proteins became lesser, as described in previous reportsCitation16
‘Dynamic exclusion’ and ‘gradient’ had a two-factor interaction. In the larger gradient, we detected more proteins with a longer dynamic exclusion. In the smaller gradient, the number of identified proteins did not change between longer and shorter dynamic exclusion. Previous analysis suggests relationship between LC and MS, but in this analysis we confirmed a two-factor interaction between both monolithic capillary LC and MS parameters.Citation24 As shown in the above gradient section, we obtained a high peak capacity in the larger gradient. A larger gradient increased peptide elution per unit time; therefore, a longer dynamic exclusion time had a larger effect than low peak capacity with a smaller gradient.
Further, ‘resolution’ and ‘gradient time’ had a two-factor interaction. A longer gradient time was necessary for a higher resolution. However, in a shorter gradient time, the effect of resolution became lesser. In a shorter gradient time, many peptides may co-elute; therefore, the number of identified proteins did not increase because of the limited scan speed in higher resolution. On the other hand, a longer gradient time would reduce co-eluting peptides and increase the margin for scan speed with high resolution. Hence, a high resolution could separate the proximal peaks and the number of identified proteins greatly increased. When multiple peptides co-elute in one scan, a high resolution may not contribute to an increase in the number of identified proteins because of a limited scan speed.
‘IT of MS2’ and ‘AGC of MS2’ had a two-factor interaction. In 100 ms of IT of MS2, ions were maximally introduced into MS in our AGC setting.Citation16 The scan number and number of identified proteins did not change significantly between AGC of MS2 at 5000 and 50000. In 50 ms of IT of MS2, the amount of introduced ions did not reach the upper limit with AGC at 5000, as shown in the previous report.Citation16 Therefore, the scan number and number of identified proteins increased.
Contribution of possible effects of parameters on increase in average peak area of MS1
When the average peak area of MS1 was considered as a response variable, it was significantly affected by seven main effects (AGC of MS1, dynamic exclusion, injection amount, linear velocity, ion injection time of MS1, number of MS2 scans, and ion injection time of MS2) and one two-factor interaction (ion injection time of MS2-minimum count threshold) (Fig. ).
‘Smaller AGC of MS1’ and ‘shorter IT of MS1’ significantly increased the average peak area of MS1. As the amount of ions increases, mass deviation increases.Citation8 Therefore, many ions may have a negative effect on the average peak area of MS1.
In a ‘shorter dynamic exclusion time,’ the average peak area of MS1 increased. We could identify stronger ions in the MS2 spectrum by shorter dynamic exclusion. Then, the average peak area of MS1 significantly increased. In this analysis, the average peak area of MS1 was calculated by integrating PSMs of Top3 peptides. Therefore, the average peak area of MS1 became higher when peptides with higher ion intensities were identified in the MS2 spectrum.
A ‘higher injection amount of the sample’ increased the average peak area of MS1. When a higher amount of peptides was introduced into MS, a higher amount of ions was introduced to MS. Therefore, the amount of ions, which reached the detector, increased and the average peak area of MS1 significantly increased, as described in the previous report.Citation9
A ‘slow linear velocity’ significantly increased the average peak area. A slow linear velocity leads to a high ionization efficiency and reduction in ion suppression.Citation25 Furthermore, a small flow rate enriches the analyte on the column and leads to a high ion intensity.Citation26 These effects were critical for increasing the ion intensity because of a high S/N ratio.
A ‘higher number of MS2 scans’ increased the average peak area of MS1. We could detect peptides with high ion intensities within each scan by a higher number of MS2 scans. Therefore, the average peak area of MS1 increased, as described in the section of dynamic exclusion.
A ‘longer IT of MS2’ increased the average peak area of MS1. A longer IT of MS2 leads to a slightly increased number of identified proteins. A larger number of identified proteins implied that a longer IT of MS2 enables to obtain a better spectrum. In addition, PSMs assigned to peak areas may increase, as described in the above section on dynamic exclusion.
The ‘minimum count threshold’ and ‘IT of MS2’ had a two-factor interaction on the average peak area of MS1. In IT of MS2 at 100 ms, the average peak area of MS1 did not change depending on the minimum count threshold. In IT of MS2 at 50 ms, the average peak area of MS1 increased at a smaller minimum count threshold. In a longer IT of MS2 at a small minimum count threshold, IT of MS2 became longer to introduce ions maximally to MS. Therefore, the scan speed may become slower in IT of MS2 at 100 ms than in IT of MS2 at 50 ms. Hence, the average peak area of MS1 may increase, as described in the above section on dynamic exclusion.
Compared with the two-factor interaction between the number of identified proteins and average peak area of MS1, the average peak area of MS1 had a two-factor interaction only between MS parameters. To maximize the peak area of monolithic capillary LC–ESI–MS/MS, we may not need to optimize the parameters of LC and MS simultaneously.
Optimization of LC, ESI, and MS parameters
The optimized parameter sets for maximizing the number of identified proteins or average peak area of MS1 are shown in Table . We performed proteome analysis with human cell lysate in triplicate to verify that an increase of the number of identified proteins and the average peak area of MS1 with these parameter sets is not due to run-to-run variance. As a result, the number of identified proteins was significantly increased by 8.1% and the average peak area of MS1 was increased by 67% (Fig. ). In these analyses, we could optimize LC, ESI, and MS parameters depending on the target response variables with a high reproducibility. We should mention that these parameters could be local maximum. In further research, we will improve our method to find global maximum by using wide range of values for each parameter. These parameters cannot be readily adopted to other MS instruments and LC column, but this DoE methodology can be adopted to other MS instruments and LC column.
Table 2. Optimized parameters for increasing number of identified proteins or average peak area of MS1.
With respect to the parameter set for maximizing the number of identified proteins, the number of identified proteins increased by 8.1% and gradient time increased by 25%, i.e., the increasing rate of gradient time was larger than that of the number of identified proteins. Long gradient times would not be ideal for increasing the number of identified proteins per minute. To identify a higher number of proteins in one-shot analysis with high time efficiency, a high scan speed with high resolution is required, particularly in human proteome analysis.Citation27 In our settings, the scan speed of MS is approximately 2–3 s per MS1 scan and subsequent ten MS2 scans.Citation23 Shishkova et al.Citation27 recently described that a high peak capacity with a longer separation time or highly efficient chromatography did not lead to an increase in the number of identified proteins without a high scan speed. In addition, even if the scan speed increases, the number of identified proteins does not readily increase unless the peak capacity increases. The scan speed of the most recent MS, e.g., Orbitrap Fusion, is approximately five times faster than that of another recent MS, e.g., Orbitrap Velos. To utilize such cutting-edge MS, we should simultaneously optimize LC, ESI, and MS parameters such as scan speed, resolution, and peak capacity.
Conclusion
With a definitive screening design, we could optimize LC, ESI, and MS parameters for maximizing the number of identified proteins and average peak area of MS1 in non-targeted proteomics. It is required to optimize instruments with a definitive screening design depending on the samples, column length, inner diameter, and MS instruments. By referring to this study, such parameters can be optimized with fewer experiments.
Previous papers have only focused on LC or MS parameters individually for optimizing LC–ESI–MS/MS.Citation6,8,9,16 In our analysis, we could detect two-factor interactions between LC and MS for the first time, to the best of our knowledge. We should now take into consideration two-factor interactions between both LC and MS for optimizing LC, ESI, and MS. Optimizing parameters using a definitive screening design will be a versatile method for any LC–ESI–MS/MS.
Author contribution
SA designed and performed the experiments, analyzed the data, and completed the manuscript. HM prepared monolithic columns. SA, WA, HM, and MU discussed the results, commented on the manuscript, and approved the manuscript submission.
Disclosure statement
No potential conflict of interest was reported by the authors.
Funding
This research was supported by JST, CREST [grant number JPMJCR16G2], and a Grant-in-Aid for JSPS Fellows [grant number 16J08791] from Japan Society for the Promotion of Science.
Supplemental materials
The supplemental material for this paper is available at https://doi.org/10.1080/09168451.2017.1391685.
Supplemental_Table1__Correct_file_.pdf
Download PDF (14.1 KB)Supplemental_Table_3_Final_.pdf
Download PDF (29.4 KB)Supplemental_Table_2_Final_.pdf
Download PDF (108 KB)References
- Beck M, Schmidt A, Malmstroem J, et al. The quantitative proteome of a human cell line. Mol Sys Biol. 2011;7(1):549.
- Nagaraj N, Wisniewski JR, Geiger T, et al. Deep proteome and transcriptome mapping of a human cancer cell line. Mol Sys Biol. 2010;7:548–548.
- Taniguchi Y, Choi PJ, Li G-W, et al. Quantifying E. coli proteome and transcriptome with single-molecule sensitivity in single cells. Science. 2010;329(5991):533–538.10.1126/science.1188308
- Geiger T, Wehner A, Schaab C, et al. Comparative proteomic analysis of eleven common cell lines reveals ubiquitous but varying expression of most proteins. Mol Cell Proteomics. 2012;11(3): M111-014050.
- Michalski A, Cox J, Mann M. More than 100,000 detectable peptide species elute in single shotgun proteomics runs but the majority is inaccessible to data-dependent LC−MS/MS. J Proteome Res. 2011;10(4):1785–1793.10.1021/pr101060v
- Andrews GL, Dean RA, Hawkridge AM, et al. Improving proteome coverage on a LTQ-orbitrap using design of experiments. J Am Soc Mass Spectrom. 2011;22(4):773–783.10.1007/s13361-011-0075-2
- Vollmer M, Hörth P, Nägele E. Optimization of two-dimensional off-line LC/MS separations to improve resolution of complex proteomic samples. Anal Chem. 2004;76(17):5180–5185.10.1021/ac040022u
- Kalli A, Hess S. Effect of mass spectrometric parameters on peptide and protein identification rates for shotgun proteomic experiments on an LTQ-orbitrap mass analyzer. Proteomics. 2012;12(1):21–31.10.1002/pmic.v12.1
- Xu P, Duong DM, Peng J. Systematical optimization of reverse-phase chromatography for shotgun proteomics. J Proteome Res. 2009;8(8):3944–3950.10.1021/pr900251d
- Jones B, Nachtsheim CJ. A class of three-level designs for definitive screening in the presence of second-order effects. J Qual Technol. 2011;43(1):1.
- Aburaya S, Esaka K, Morisaka H, et al. Elucidation of the recognition mechanisms for hemicellulose and pectin in Clostridium cellulovorans using intracellular quantitative proteome analysis. AMB Express. 2015;5(1):67.10.1186/s13568-015-0115-6
- Morisaka H, Matsui K, Tatsukami Y, et al. Profile of native cellulosomal proteins of Clostridium cellulovorans adapted to various carbon sources. AMB Express. 2012;2(1):37.10.1186/2191-0855-2-37
- Yamana R, Iwasaki M, Wakabayashi M, et al. Rapid and deep profiling of human induced pluripotent stem cell proteome by one-shot nano LC–MS/MS analysis with meter-scale monolithic silica columns. J Proteome Res. 2013;12(1):214–221.10.1021/pr300837u
- Lemeer S, Hahne H, Pachl F, et al. Software tools for MS-based quantitative proteomics: a brief overview. In: Marcus K, editor. Quantitative methods in proteomics. Totowa, NJ: Humana Press; 2012. p. 489–499.10.1007/978-1-61779-885-6
- Silva JC, Gorenstein MV, Li GZ, et al. Absolute quantification of proteins by LCMS(E) a virtue of parallel MS acquisition. Mol Cell Proteomics. 2006;5(1):144–156.10.1074/mcp.M500230-MCP200
- Kalli A, Smith GT, Sweredoski MJ, et al. Evaluation and optimization of mass spectrometric settings during data-dependent acquisition mode: focus on LTQ-orbitrap mass analyzers. J Proteome Res. 2013;12(7):3071–3086.10.1021/pr3011588
- Hurvich CM, Tsai CL. Regression and time series model selection in small samples. Biometrika. 1989;76:297–307.
- Cox J, Hein MY, Luber CA, et al. Accurate proteome-wide label-free quantification by delayed normalization and maximal peptide ratio extraction, termed MaxLFQ. Mol Cell Proteomics. 2014;13(9):2513–2526.10.1074/mcp.M113.031591
- Feltcher ME, Gunawardena HP, Zulauf KE, et al. Label-free quantitative proteomics reveals a role for the Mycobacterium tuberculosis SecA2 pathway in exporting solute binding proteins and Mce transporters to the cell wall. Mol Cell Proteomics. 2015;14(6):1501–1516.10.1074/mcp.M114.044685
- Horie K, Sato Y, Kimura T, et al. Estimation and optimization of the peak capacity of one-dimensional gradient high performance liquid chromatography using a long monolithic silica capillary column. J Chromatogr A. 2012;1228:283–291.10.1016/j.chroma.2011.12.088
- Köcher T, Swart R, Mechtler K. Ultra-high-pressure RPLC hyphenated to an LTQ-orbitrap velos reveals a linear relation between peak capacity and number of identified peptides. Anal Chem. 2011;83(7):2699–2704.10.1021/ac103243t
- Horie K, Kamakura T, Ikegami T, et al. Hydrophilic interaction chromatography using a meter-scale monolithic silica capillary column for proteomics LC–MS. Anal Chem. 2014;86(8):3817–3824.10.1021/ac4038625
- Kim MS, Kandasamy K, Chaerkady R. Assessment of resolution parameters for CID-based shotgun proteomic experiments on the LTQ-orbitrap mass spectrometer. J Am Soc Mass Spectrom. 2010;21(9):1606–1611.10.1016/j.jasms.2010.04.011
- Pirmoradian M, Budamgunta H, Chingin K, et al. Rapid and deep human proteome analysis by single-dimension shotgun proteomics. Mol Cell Proteomics. 2013;12(11):3330–3338.10.1074/mcp.O113.028787
- Schmidt A, Karas M, Dülcks T. Effect of different solution flow rates on analyte ion signals in nano-ESI MS, or: when does ESI turn into nano-ESI? J Am Soc Mass Spectrom. 2003;14(5):492–500.10.1016/S1044-0305(03)00128-4
- Meiring HD, der Heeft VE. Nanoscale LC–MS (n): technical design and applications to peptide and protein analysis. J Sep Sci. 2002;25(9):557–568.10.1002/(ISSN)1615-9314
- Shishkova E, Hebert AS, Coon JJ. Now, more than ever, proteomics needs better chromatography. Cell Syst. 2016;3(4):321–324.10.1016/j.cels.2016.10.007