ABSTRACT
Whether intended by education policy (e.g., via school entrance examinations) or unintended (e.g., via social stratification), selection effects in education (who goes where, gets what, and how much) shape educational opportunities – influencing the life chances of individuals and groups, and the structure of societies. However, within the quantitative approach to educational research, current statistical methods can struggle to simultaneously evaluate both the presence and impacts of these effects. In turn, this methodological limitation impedes efforts to facilitate equality of educational opportunity. This paper responds with a critical overview of types of selection effects in education, their consequences for educational opportunity, and the statistical methods used for their identification. Two empirical illustrations show how a new statistical method (“airbag moderation”) can enable better detection and evaluation of selection effects in education and help direct future research into selection effects in education with a focus on opportunities for equality of educational opportunity.
Selection effects in education: definition, differentiation, consequences for educational opportunity, and limitations to extent knowledge
Regardless of the ecological level at which one considers (or experiences) an issue in education – at the macro (societal) level, at the individual level, or at any level in between – there exist narratives that ask, “Who goes where, gets what, and how much of it?” Collectively, these questions therefore speak to “selection effects” in education – the processes that bring people and resources together in any given educational context. So ubiquitous are these questions that it is hard to imagine any issue in education where these effects are not a pertinent issue – even, say, for individual self-study where an individual wishes to engage with a text in a given study space. As a result, selection effects in education are a common narrative within discourses concerning education policymaking, educational practice, and within discourses of educational research that engage with both; for example, within narratives on educational effectiveness (e.g., Sarid, Citation2022), in narratives on educational inequality and inequity of opportunity (e.g., Belsky et al., Citation2019; Cara, Citation2022), and in narratives concerning educational ex/inclusion pertaining to both social inclusion (e.g., Alan et al., Citation2023; Juvonen et al., Citation2019) and inclusion in the context of special educational needs (e.g., Brock, Citation2018).
Selection effects in education may be grouped together and distinguished from one another in a variety of ways. shows two such differentiators in combination and mapped against (non-comprehensive) examples. Of the two differentiating dimensions shown in , the first concerns the location where a selection effect operates within the strata of a generalised national education system, and the second dimension differentiates whether a selection effect is intended or unintended by education policy.
Table 1. Examples of selection effects in education (who goes where, gets what, and how much): differentiation by educational system strata and the intent of education policies.
Looking at , the mechanisms by which the selection effects function can be understood to differ according to whether or not they are intended or unintended. For selection effects that are intended by education policies, the mechanisms of their effects are, by intent, often more readily observable than are the mechanisms of effect for unintended selection effects. Further, this is apparent across the strata of the educational system. At the macro societal level, for example, intended selection effects are self-evident in their educational policies (e.g., free school meals for children from disadvantaged backgrounds; see Micha et al., Citation2018). Likewise at the individual level, there will be clearly observable links between educational policies for a selection effect, that effect “in action”, and the consequences of that effect. Take, for example, educational policies that give students a choice of subject to study (e.g., Henriksen et al., Citation2015) and/or educational policies that aim to draw greater numbers of teachers into certain geographical areas (e.g., more socially disadvantaged urban areas; Aragon, Citation2016).
Considering next selection effects in education that are unintended by educational policy, their mechanisms of effect are, by definition, harder to directly link to educational policies, and are therefore, arguably, harder to directly observe. Of the variety of psychological, economic, and sociological processes alluded to by the examples presented in , these processes vary depending on whether they concern individuals (from policymakers to teachers, students, and parents), collective groups (e.g., school communities), or broader societal structures. It is, perhaps, also interesting to note that the socioeconomic heuristic device of “capital” has application across all of these layers (e.g., DiMaggio, Citation1991), and by defining matters of “Who has what?”, this concept is particularly relevant to the three questions at the heart of selection effects in education – “Who goes where, gets what, and how much of it?” Take, for example, the concept of “Matthew effects” in education (where the “rich” get richer and the “poor” get poorer over time; see Zuckerman, Citation2010). Another way to conceive this effect is that those with more capital (of one type or another) can use this to better control the what, where, and how much of any advantageous (in their opinion at least) education opportunity that they wish they and their dependents to receive with the belief that this will yield a competitive advantage in the capital that is subsequently generated.
From mechanisms to consequences and returning to the concept of selection effects in education (such as those shown in ), these are intrinsically linked to educational opportunity and the distribution of educational opportunities across people, places, and time – as per the example concerning capital presented above. Logically, narratives concerning the presence and impacts of individual selection effects (rather than the generalised concept) can therefore be observed wherever there are discussions concerning educational equality, equity, and inclusion. Take, for example, discourses regarding differential school effectiveness (e.g., Hübner et al., Citation2019), and differentiated instruction to support the inclusion of children with special educational needs in mainstream educational settings (e.g., Crockett et al., Citation2012).
Given the existence of a multitude of debates on the types of selection effect operating in education (intended or unintended and across education strata), one might be tempted to ask, “What is the point of a narrative that engages across these effects?” The answer is that there are common limitations to extent knowledge across these different effects. Primarily this is because of the common processes and mechanisms by which types of selection effects function (such as the role of capital in fostering Matthew effects), but this is also because common processes and mechanisms elicit common research methods and no research method exists without limitations in its capabilities.
Difficulties with existing statistical methods when used to evaluate selection effects in education
Rightly or wrongly, governmental funding of education research frequently results in greater financial expenditure on projects conducted within the quantitative tradition (e.g., Cheek, Citation2011; Edwards, Citation2022; Morse, Citation2002). In the context of selection effects in education, the frequent predominance of governmental spend on the quantitative tradition of research raises the importance of the methods that are used within this tradition to determine the presence, mechanisms, and impacts of selection effects.
While it is beyond the scope of this journal article to present a comprehensive mapping of the methods used in the quantitative tradition of research to determine the presence, mechanisms, and impacts of selection effects in education against their (seeming) ubiquity, it is important to highlight that there is at least one type of selection effect in education that prevailing methods in the quantitative tradition struggle to evaluate. These harder-to-evaluate selection effects concern group differences that trigger experiences that further magnify (or reduce) differences between these groups (e.g., processes that can broaden or restrict access to educational opportunities). For selection effects in education that are intended, these processes can be frequently observed in policies, practices, and interventions that have a targeting element coupled with the intent to reduce group differences. This includes macrolevel policies such as Head Start (e.g., Ludwig & Miller, Citation2007) and Sure Start Children’s Centres (see Hall et al., Citation2015) that target support at certain groups of families, plus interventions within schools that target support at certain groups of children such as those with special educational needs (e.g., the response to intervention model; Ridgeway et al., Citation2012).
For selection effects in education that are unintended by education policy, triggered processes that widen or narrow differences between groups are achieved either by an unintended targeting element (cf. “who gets what?”) or by the effect of “who gets what” on one or more differences between groups. Take, for example, gender differences in science, technology, engineering, and mathematics (STEM) careers, how these are influenced by gender differences in students’ choice of degree subject/major in university, and how these associations persist, at least in part, due to gender stereotypes of STEM careers that exist across multiple levels of society (e.g., Piatek-Jimenez et al., Citation2018; Wang & Degol, Citation2013). Gender stereotypes foster a gender difference in choice of subject/major (“who gets what”) at university that in turn acts as a barrier preventing access to many careers in STEM. Not only is the gender stereotyping fostering of an unintended selection effect in education that reduces educational opportunity, but this effect persists even in the face of intended educational policies and interventions with the purpose of countering selection effects such as these (e.g., Liben & Coyle, Citation2014).
describes (and illustrates) five different types of selection effect in education. These link the characteristics of individuals and groups (x) to one or more aspects of education (y; that can be selected) and to one or more educational outcomes (z). The terminology used in this table is purposefully broad to be inclusive of selection effects concerning both individuals and groups, selection effects that are intended and unintended by education policy, and selection effects that occur across educational strata (see ). It is the last two types of selection effect in education shown in (Types 4 and 5; with Type 5 being a simple extension of Type 4) that concern triggered processes that shrink or grow the differences between groups – the type of selection effect that current statistical methods struggle to evaluate. One of the reasons why current statistical methods struggle in application to these types of selection effect is because they blend together two distinct trivariate hypotheses (mediation and moderation) in a manner that is historically rare and, at times, historically counter to then contemporary statistical literature (for a more detailed historical explanation, see Hall, Malmberg, et al., Citation2020).
Table 2. Five types of selection effects in education: text description, conceptual illustration, and common statistical methods used in their empirical evaluation.
Towards a better statistical method for evaluating selection effects in education that shrink or grow differences between groups: airbag moderation
Progress continues to be made in the development of statistical methods that can be used in the analysis of the selection effects in education that shrink or grow differences between groups – the type of selection effect that current statistical approaches struggle to evaluate (Types 4 and 5 in ). One area of development concerns how two trivariate hypotheses that are used in the quantitative approach to research (mediation and moderation) can be blended together (e.g., B. Muthén et al., Citation2015; Preacher et al., Citation2007). Recently, Hall, Malmberg, et al. (Citation2020) documented a novel blend of these hypotheses termed “airbag moderation”, and this hypothesis, along with its accompanying statistical methods, now provides researchers with the methodological language, terminology, and accompanying statistical methods that are required to empirically test (and describe) the functioning of these types of selection effect (Types 4 and 5 in ); for online explanatory videos, see Hall (Citation2024a, Citation2024b).
provides an illustration of the trivariate hypothesis of airbag moderation plus illustrations of three statistical methods that can be used to test it empirically. For clarity, it is important to distinguish the conceptual illustration of airbag moderation that is shown in from the illustrations of the three statistical models. They look similar but are different. The conceptual illustration of airbag moderation refers to concepts and hypothesised relationships. The illustrated statistical models refer to statistical variables and to statistical relationships that are evaluated within a structural equation modelling (SEM) framework. For readers familiar with SEM, this distinction is akin to the difference between an illustration of the hypothesis of moderation and an illustration of a test of this hypothesis through specification of a multiplicative statistical interaction term (a distinction alluded to in Model 1 shown in with the “x.y” multiplicative statistical interaction variable).
Figure 1. A conceptual illustration of the hypothesis of airbag moderation and an illustration of three statistical models that can test this hypothesis (adapted from Hall, Malmberg, et al., Citation2020).
aNote the relationships between y and z and between x.y and z. These are required for an accurate evaluation of the statistical interaction term, but prevent this statistical model from representing selection effect Type 4 rather than selection effect Type 5 (as per ).
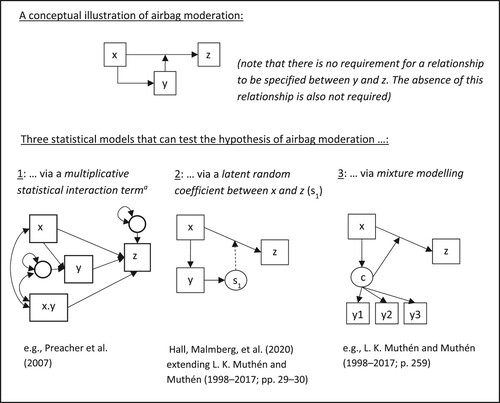
Of the three statistical models illustrated in , these vary from one another in how closely the statistical relationships that they model match the relationships contained within the trivariate hypothesis of airbag moderation – with the closest correspondence being with Model 2. Model 2 shows an SEM featuring a latent random coefficient (s1) between concepts/variables x and z (in the case of selection effects in education: a characteristic of an individual/group [x] and an educational outcome [z]).
As with all statistical modelling, the closer the correspondence between a statistical model and the hypothesised relationships that it is designed to empirically test, the greater the benefits for the validity of the findings (internal and external). This logic extends to the empirical testing of the two types of selection effect in education that hitherto current statistical models have struggled with (Types 4 and 5 shown in ). Model 2 in provides the closest correspondence of these effects (i.e., the estimated statistical relationships correspond one-to-one with the relationship in the hypothesis) and can therefore be regarded as an ideal “go to” option for researchers wishing to evaluate these kinds of selection effects using the airbag moderation hypothesis.
Note that despite the positive connotations of its name, airbag moderation effects may be both positive and negative – just as automobile airbags, though intended to be helpful, can also cause unintentional harm (e.g., if improperly installed, or used in improper circumstances such as with infants). Indeed, Hall, Malmberg, et al. (Citation2020) make a distinction between airbag moderation that is protective and increases the likelihood of a positive outcome versus airbag moderation that is harmful and decreases the likelihood of a positive outcome.
Empirical examples of selection effect in education that shrink or grow differences between groups – evaluated through use of airbag moderation
We now present two examples of selection effects in education from different countries (UK and USA), different phases of education (Early Childhood Education and Care [ECEC] and high school), and that may widen or shrink differences between groups of students in their educational attainment. Both examples are evaluated via use of the hypothesis of airbag moderation and structural equation models that specify a latent random coefficient (Model 2 in ). As with any worked example, the substantive findings presented below are to be interpreted with utmost caution; their purpose is to illustrate the topic of this paper.
The first example selection effect in education uses a relatively straightforward statistical model (to aide understanding). It extends beyond the statistical model presented in Hall, Malmberg, et al. (Citation2020) only through the inclusion of a “censored variable”. This was necessary to include so that accurate findings would be returned concerning ECEC usage where many families reported zero usage. It illustrates that selection effects in education can widen differences between groups of students in educational outcomes. The second example carries out more complex statistical modelling (closer to what might be found in a regular empirical paper) by testing an airbag moderation within multilevel data (students nested in high schools) and producing statistical associations that are net of statistical covariates/controls. It illustrates that selection effects can also reduce differences between groups of students in educational outcomes. Across both examples, judicious use of figures supplement the presentation of results.
Example 1. Evidence of an unintended selection effect in UK Early Childhood Education and Care: the socioeconomic status of families, the hours per week use of centre-based childcare, and the non-verbal cognition of 4-year-olds
Introduction
Studies of selection effects concerning young children’s placement into non-maternal ECEC often focus upon effects related to families’ socioeconomic status (SES; e.g., Sylva et al., Citation2007). Past research has shown that children from more socioeconomically advantaged backgrounds can be more likely to experience types of ECEC that are associated with an increased likelihood of positive developmental outcomes (e.g., Stein et al., Citation2013; Sylva et al., Citation2011) – Type 3 effects from . What we do not know though, partly due to the methodological difficulties discussed above, is whether there may also be Type 4 or Type 5 selection effects. More specifically, we can now ask: Might attending centre-based ECEC act as an airbag moderator increasing the strength of the relationship between children’s non-verbal cognition at 51 months and the SES of their families?
Materials and methods
Sample
Data come from the Families, Children and Child Care (FCCC) study (see Sylva et al., Citation2007), a prospective longitudinal study of the care of 1,201 UK children from birth to school age (601 living in Oxfordshire and 600 in North London). Data from this project are available for research and teaching purposes upon request (http://www.familieschildrenchildcare.org/data-disemination.html). Descriptive statistics and bivariate associations for all variables considered in this example are presented in .
Table 3. Variables, descriptive statistics, and bivariate associations for Example 1 data drawn from the Families, Children and Child Care (FCCC) study (see Sylva et al., Citation2007).
Measures
Data on family SES came from family questionnaires used when children were 3 months old. A family SES variable was subsequently created from the mean average of three z scored variables: father’s socioeconomic class, mother’s educational level, and family income (see Sylva et al., Citation2007).
Data on the hours each child spent in different forms of non-maternal ECEC (paternal, grandparent or relative, childminder, nanny, or centre-based) came from family questionnaires used when children were 3, 10, 18, 36, and 51 months of age and restructured into hours-by-type for each month. A variable was created that recorded the hours each child spent in centre-based ECEC (hereafter just “ECEC”) between 0 and 51 months. This variable was highly skewed due to being zero-rich with only 430 children having experienced any amount of ECEC. As a result, the variable was split into two parts: a censored variable indicating use of ECEC (1 = some; 0 = none) and a continuous variable capturing ECEC hours for the 430 ECEC-using children. These two variables were then analysed using a censored regression approach (see below).
Children’s cognition was assessed when they were 51 months of age, and data came from the judgements of trained and reliable fieldworkers who used the second edition of the British Ability Scales (BAS; Elliott et al., Citation1996). The BAS score for the “Picture Similarities” task provided a measure of non-verbal cognition.
Analytic approach
A Type 4 education selection effect was tested with the specification of a structural equation model that specified a latent random coefficient (Model 2 in ). Because of the zero-rich information available on ECEC hours within the FCCC data, this model also used a censored variable approach (https://www.statmodel.com/mplusbook/chapter7.shtml). Both the binary (i.e., 0 = no ECEC, 1 = some ECEC) and the continuous ECEC (i.e., hours in ECEC for 430 children) variables were considered as airbag moderators at the same time, and a statistical association was estimated between them following Clark (Citation2023) and the Heckman model (e.g., https://m-clark.github.io/models-by-example/heckman-selection.html). The resulting SEM model and statistical results are shown in full in the online supplementary material.
Listwise deletion from n = 1,201 to n = 1,019 was used to handle missing data (for pedagogical purposes). As discussed in Hall, Malmberg, et al. (Citation2020), traditional absolute SEM model fit indices (e.g., root-mean-square error of approximation, comparative fit index) are (not yet) available. Analyses were carried out in Mplus Version 8.8 (L. K. Muthén & Muthén, Citation1998–2017).
Results
Might attending centre-based ECEC act as an airbag moderator increasing the strength of the relationship between children’s non-verbal cognition at 51 months and the SES of their families?
Within the UK FCCC data, and looking at children born at the turn of the millennium, there is evidence of a (Type 4) selection effect in education relating to children’s non-verbal cognition at 51 months of age, family SES, and the hours per week that they attended centre-based ECEC from age 3 months – although not from simply attending. Socioeconomic advantage was associated with more hours per week of ECEC usage through to 51 months (β = 0.42, p < .001), and the greater the hours per week of ECEC used, the stronger the association between SES and children’s non–verbal cognition at 51 months (β = 0.14, p < .001; see ). In other words, SES differences in children’s non–verbal cognition at 51 months are magnified due to SES promoting more hours per week in used ECEC.
Figure 2. Statistically significant moderation of the relationship between family socioeconomic status (SES) and child non-verbal cognition at 51 months by increased hours per week in centre-based ECEC (β = 0.14, p < .001).
Note: Vertical lines from the dots are 95% confidence intervals; zero on the y-axis indicates zero relationship between family SES at 3 months (m) and child non-verbal cognition at 51 months.
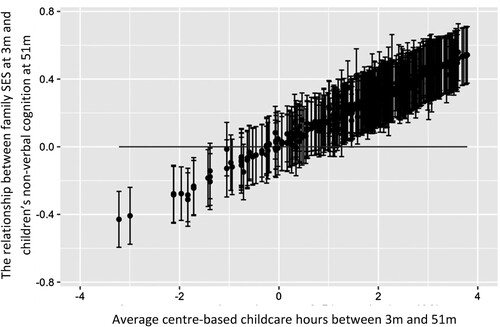
Discussion
There are multiple possible reasons that could explain the finding of an unintended selection effect in mid-2000s UK ECEC that widened SES gaps in children’s non-verbal cognition at age 51 months (or at least within the FCCC data). It is possible, for example, that the effects found here are the product of associations that were not included in these analyses – particularly effects linked to the quality of centre-based ECEC (e.g., Hall et al., Citation2009, Citation2013). Interested readers are encouraged to explore such possibilities further within the publicly available FCCC data, where there is a wealth of additional data on children, families, and ECEC.
What we do know however is that in the past 20 years, there has been a substantial increase in spend by UK governments on families’ access to nominally free ECEC (e.g., Farquharson, Citation2019). While this may have lessened the selection effect shown here, the consequences of this government policy for children and families are complex. Take, for example, the concerns that the UK government funding provided to ECEC providers can be inadequate to cover these “free” places (e.g., Pascal et al., Citation2021). This risks the financial viability of ECEC providers and therefore families’ opportunities to access the ECEC that this government policy is designed to support. In other words, the implementation of this policy risks introducing a new unintended selection effect in education.
Example 2. Evidence of an unintended selection effect in US high school: student SES, student academic track, and 4-year US college enrolment
Introduction
Academic tracking shapes educational opportunities and, in the USA, typically begins in late middle school or at the start of high school (Oakes, Citation2005). Students are typically placed in academic tracks based on previous academic performance, such as grades and achievement test scores. Low-SES and underserved minority (UM) students are disproportionately placed in the lower/less academic track. In turn, track placement is predictive of college readiness and college enrolment because the track-based curricular and peer influences tend to increase the gaps in academic skills and educational aspirations between students in the low track and high tracks (Domina et al., Citation2017). This conceptual framework suggests that tracking may serve as an airbag moderator of the college enrolment gap between low- and high-SES students.
This example considers a Type 4 education selection effect. More specifically, we ask: Might academic track placement act as an airbag moderator reducing the strength of the relationship between American high school students’ SES and 4-year college enrolment? Both the curriculum and peer influences present on a high/academic track are expected to serve as protective agents for students from lower SES backgrounds, increasing students’ academic performance and postsecondary educational aspirations; this, in turn, increasing the likelihood of college enrolment (Palardy, Citation2013).
Materials and methods
Sample
Data are publicly available and come from the Education Longitudinal Study (ELS), a survey of American high school sophomores conducted by the National Center for Education Statistics (NCES; https://nces.ed.gov/surveys/els2002/). The ELS started in 2002 and provides accurate data on student progressions through high school and subsequent college enrolment. The sample used here features 10,151 students who attended 580 public high schools.
Measures
describes the variables used in this example, including descriptive statistics broken down by total, low-, medium-, and high-SES groupings, and the variable names used in the ELS: 2002 database (enabling replication). The low-SES group is the bottom quintile, the medium group is the middle three quintiles, and the high-SES group is the upper quintile. Whether the student enrolled in a 4-year college programme after high school is the (categorical) outcome variable (0 = did not enrol; 1 = did enrol). The airbag moderator is track placement, which is also a categorical measure (0 = non-academic; 1 = academic). SES is a continuous measure based on five equally weighted components including mother’s and father’s educational attainment and occupational status, plus family income. The values in the table show a systematic pattern of educational disadvantage for students from low-SES families. They are less likely to be placed in an academic track, tend to have lower grade point averages (GPA), achievement test scores, and educational aspirations, and are less likely to enrol in college.
Table 4. Variables and descriptive statistics for Example 2 data drawn from the Education Longitudinal Study (ELS; see https://nces.ed.gov/surveys/els2002/).
In addition to the three variables essential for fitting an airbag moderation model, three student control variables were used in this example. These variables controlled for the effects of academic background (GPA and achievement test scores) and educational aspirations on college enrolment, as they are student characteristics typically used to assess college admissions and college readiness. These controls helped “home in” on the SES effects apart from these other interrelated factors. GPA is grade point average for academic courses during 10th grade. The achievement test score is an equally weighted composite of reading and mathematics achievement, measured at the end of 10th grade. Attainment expectations is an ordinal measure of how far in schooling a student plans to go, also collected at the end of 10th grade (0 = high school diploma or less, 7 = doctorate).
Analytic approach
A Type 4 education selection effect was tested with two structural equation models that each included a latent random coefficient (Model 2 in ). The models estimated the effect of SES on the likelihood of enrolling in college after high school, which was specified to randomly vary across the sample based on student SES. The model also estimated the degree to which academic track placement moderated this association.
Two models were fitted to the data: a base model excluding student controls/statistical covariates, and a second model including controls for academic background (GPA, achievement test scores) and attainment expectations. Showing the results of both provides a sense of the degree to which academic background and educational aspirations inform the education selection effect tested via the airbag moderation model. The Mplus outputs for these models are provided in the online supplementary material. Missing data were replaced using maximum likelihood methods (extending upon the methods used in Example 1). The multilevel structure of the data (students in schools) was accounted for with modification of standard errors (e.g., Wu & Kwok, Citation2012). As discussed in Hall, Malmberg, et al. (Citation2020), traditional absolute SEM model fit indices are (not yet) mathematically available for the model considered in this example. However, comparative fit indices are available (e.g., chi square, Akaike information criterion, and Bayesian information criterion). Analyses were carried out in Mplus Version 8.8 (L. K. Muthén & Muthén, Citation1998–2017).
Results
Might academic track placement act as an airbag moderator reducing the strength of the relationship between American high school students’ SES and 4-year college enrolment? shows the results for the base model. Student SES has a strong positive association with the likelihood of enrolling in college after high school, β1 = 1.316 (logit), 3.73 (odds ratio [OR]), p < .001, indicating students from higher SES families are more likely to enrol. This effect varied significantly across the sample, Var(β1) = 0.243, p < .001, suggesting that effect of SES on college enrolment tends to differ for low- versus high-SES students. Student SES was also positively associated with academic track placement, β2 = 0.415 (logit), 1.51 (OR), p < .001, indicating higher SES students were more likely to be placed in an academic track in high school. Track placement, the airbag moderator in this model, had a negative and statistically significant statistical effect on the association between SES and college enrolment (β3 = −0.418, p < .001), indicating placement in the academic track served as a protective factor for lower SES students – it reduced college enrolment gaps between high- and low-SES students.
One limitation of the base model is that it does not account for differences in students’ academic background and attainment expectations. This is problematic because these factors are generally the basis for college admissions and tend to be correlated with SES (Domina et al., Citation2017; Oakes, Citation2005; Palardy, Citation2013). Therefore, this limitation may result in effects that link differences in academic background and attainment expectations to college enrolment being subsumed by the SES effect, inflating its magnitude. To address that concern, a second model was fitted to the data that included measures of academic background and attainment expectations (see ) to disentangle their effects from the effects of student SES on college enrolment.
The results for the second model are summarised in (with statistical associations concerning the statistical control variables being shown in the online supplementary material). The addition of the student control variables substantially reduced the magnitude of the effects from the base model, but did not change the direction of those effects or their statistical significances. Even after controlling for GPA, achievement test scores, and educational aspirations, SES remains positively associated with the likelihood of college enrolment, β1 = 0.703 (logit), 2.02 (OR), p < .001. That effect continues to vary across the sample, Var(β1) = 0.111, p < .001, but that variation was reduced by 54% compared with the base model. SES remained positively associated with being on an academic track in high school, β2 = 0.415 (logit), 1.51 (OR), p < .001. Despite this SES association, track placement also continued to act as a protective force for low-SES students – increasing the likelihood that they would enrol at a 4-year college (SES -> Enrolment: β3 = −0.237, p < .001). However, that boost is substantially reduced after controlling for academic background and aspirations.
Discussion
This example focused on examining whether track placement can serve as an airbag moderator to reduce SES-based gaps in 4-year college enrolment. The results show it does, even after controlling for academic achievement and educational aspirations. Looking forward, these results can be extended by a more detailed consideration of the multilevel (students nested in schools) data. The airbag moderation effect found here may vary significantly across schools, and certain aspects or features of the schools (e.g., structures, resources, practices, or characteristics of the student body) may account for that between-school variation in the airbag moderation effect.
Future directions
With respect to future substantive developments regarding selection effects in education: First, we suggest that more opportunities to increase equality of educational opportunity would come from a more systematic consideration of all types of selection effects in education. These effects seem almost ubiquitous, and yet we seem to lack coherent narratives on their types, presence, effects, and malleability, and therefore also cumulative empirical investigations.
Second, the potential for education to improve its evaluation of selection effects in education that shrink or grow differences between groups requires that the hypothesis of airbag moderation be taken up by more researchers. How likely is this though? In context, 20 years ago the hypotheses of moderation and mediation were just emerging into mainstream parlance within the quantitative tradition of (educational) research. For example, an informal search of papers published in School Effectiveness and School Improvement between 1990 and 1999 revealed that just three used both the terms “mediation” and “moderation” – a figure that rose to 10 between 2000 and 2009 and to 33 between 2010 and 2019. However, the history of these terms goes back much further – at least to the 1950s (i.e., Saunders, Citation1955, Citation1956). However, given the current “age of information” (e.g., Acquisti et al., Citation2015), one would hope that the uptake of airbag moderation might be swifter than the 50+ years that it took for the widespread uptake of the concepts of mediation and moderation. The recent uptake of the Johnson–Neyman technique for plotting statistical interaction effects (Johnson & Neyman, Citation1936) suggests that rapid uptake of ideas is possible when it has clear utility. At the time of writing, Google Scholar reports this paper to have been cited 714 times between 1936 and 2017, then 944 times between 2018 and 2023. With the (seeming) ubiquity of selection effects in education, there is a practical utility in the uptake of airbag moderation across multiple areas of educational research – but whether this happens and at what pace is hard to predict.
Regarding future methodological developments, the examples presented in this paper evaluated hypotheses of airbag moderation through generation of multiple statistical estimates. This is not in keeping with the statistical methods used with two other long-established trivariate hypotheses: mediation and moderation. For these, there are statistical methods available that generate a single statistical estimate for use in rejecting or accepting these hypotheses. While this may yet prove possible for airbag moderation – this possibility has yet to be explored. A separate issue is the accessibility of statistical methods for airbag moderation and thus the statistical evaluation of selection effects in education that shrink or grow differences between groups (labelled Type 4 and Type 5 in ). At the time of writing, the simplest statistical approach (from James & Brett, Citation1984; Statistical Model 1 in ) that was once available within the SPSS PROCESS macro (see Hayes, Citation2022) as “Model 74” is no longer available. Therefore, there is now a need for an alternative means of implementing this statistical model (ideally one that is both free to access and accessible as regards statistics and software knowledge) – such as might be achieved via developments within existing SEM procedures in freeware software packages.
There is also much potential for an enhanced multilevel perspective on airbag moderations within a hierarchical ecological understanding of education. The examples in this paper considered individual-level concepts and how these had statistical effects that were, in part, linked to institutional effects that functioned as airbag moderators. There are likely to be other effects that function as airbag moderators as well. Group-level concepts may be airbag moderated by individual-level effects, and institutional concepts and outcomes may be altered by airbag moderators at the level of central government (e.g., policy differences for private and public schools). The underlying point is two-fold: (a) Airbag moderation is wholly compatible with the multilevel theoretical and analytic frameworks that many educational researchers are familiar with; (b) an enhanced multilevel perspective on airbag moderation has the potential to advance our existing theorisations regarding the “who goes where, gets what, and how much?” of issues concerning educational equality, equity, and inclusion.
To bring together the above methodological suggestions, our recommendation for a meaningful methodological advancement in this area (one that would hold potential for advancing our understanding of education selection effects) would be for a study that (a) develops statistical single estimates of airbag moderation (cf. indirect effects), (b) develops statistical approaches for modelling the three concepts in a hypothesis of airbag moderation across any combination of levels in a multilevel structural equation model (where there is particular potential to advance the “random slope” approach to testing hypotheses of airbag moderation), and (c) presents the results of these developments as methods for researchers that they can implement for free – for example, as packages within the R freeware programming language.
Conclusions
This paper is a response to a limitation in our current understanding of a range of real-world processes in education. There are types of education selection effects that exist but for which there has been no coherent investigation. This limitation is a result of, in part, a limitation to the conceptual terms and concepts that educational researchers have had at their disposal. Selection effects in education thoroughly permeate education systems – from central government policymaking through to the decisions made by individual students. These effects can be both intended and unintended by education policy and have the potential to both widen and narrow differences between groups to inform discussions of policy and practice concerning educational equity and inclusion. Within the quantitative tradition to educational research, the recent emergence of airbag moderation enables both the identification of new types of selection effect in education (Types 4 and 5; see ) and provides the means to statistically evaluate the presence and impacts of these types of selection effect. The examples in the paper show the potential presence of these effects in UK and US education systems, in ECEC and high school systems, and that these effects can both widen and narrow differences between groups of students in their educational attainment and progress. What is needed next is further explorations of gap widening/narrowing selection effects in education within the quantitative tradition coupled with further development of the underlying research terminology and methods that facilitate this new narrative.
Online Supplementary Material.docx
Download MS Word (17.8 KB)Disclosure statement
The corresponding author is a voluntary and unpaid “Core Member” of the Early Years Pedagogy and Practice Forum maintained by the UK Government’s Office for Standards in Education, Children’s Services and Skills (Ofsted).
Additional information
Notes on contributors
James Hall
James Hall is Associate Professor of Psychology of Education at the University of Southampton, UK. There he leads the Quantitative Methods in Education group in the Southampton Education School, and serves as Deputy Director of the UK’s National Centre for Research Methods (NCRM). His research aims to improve our understanding of the complex interactions between parents, Early Childhood Education, and early interventions as they shape young children’s development and educational progress.
Gregory Palardy
Gregory Palardy is on the faculty at the University of California, Riverside. His research focuses on school effectiveness, educational equity, and quantitative methods. His latest project addresses factors that influence college readiness and equitable access to post-secondary education.
Lars-Erik Malmberg
Lars-Erik Malmberg is Professor of Quantitative Methods in Education, at the Department of Education, University of Oxford, UK. He has published on effects of education, child care and parenting on developmental and educational outcomes, and teacher development. He applies advanced quantitative models, including multilevel and dynamic structural equation models to the investigation of substantive research questions in education. His current research interests are on intraindividual approaches to learning processes, and the modelling of intensive longitudinal data.
References
- Acquisti, A., Brandimarte, L., & Loewenstein, G. (2015, January 30). Privacy and human behavior in the age of information. Science, 347(6221), 509–514. https://doi.org/10.1126/science.aaa1465
- Alan, S., Duysak, E., Kubilay, E., & Mumcu, I. (2023). Social exclusion and ethnic segregation in schools: The role of teacher’s ethnic prejudice. The Review of Economics and Statistics, 105(5), 1039–1054. https://doi.org/10.1162/rest_a_01111
- Aragon, S. (2016). Teacher shortages: What we know. Education Commission of the States. https://www.ecs.org/wp-content/uploads/Teacher-Shortages-What-We-Know.pdf
- Belsky, D. W., Caspi, A., Arseneault, L., Corcoran, D. L., Domingue, B. W., Harris, K. M., Houts, R. M., Mill, J. S., Moffitt, T. E., Prinz, J., Sugden, K., Wertz, J., Williams, B., & Odgers, C. L. (2019, April 8). Genetics and the geography of health, behaviour and attainment. Nature Human Behaviour, 3(6), 576–586. https://doi.org/10.1038/s41562-019-0562-1
- Brock, M. E. (2018). Trends in the educational placement of students with intellectual disability in the United States over the past 40 years. American Journal on Intellectual and Developmental Disabilities, 123(4), 305–314. https://doi.org/10.1352/1944-7558-123.4.305
- Cara, O. (2022). Geography matters: Explaining education inequalities of Latvian children in England. Social Inclusion, 10(4), 79–92. https://doi.org/10.17645/si.v10i4.5809
- Cheek, J. (2011). The politics and practices of funding qualitative inquiry. In N. K. Denzin & Y. S. Lincoln (Eds.), The SAGE handbook of qualitative research (4th ed., pp. 251–268). SAGE Publications.
- Clark, M., (2023). Model estimation by example. Demonstrations with R. https://m-clark.github.io/models-by-example/
- Crockett, J. B., Filippi, E. A., & Morgan, C. L. (2012). Included, but underserved? Rediscovering special education for students with learning disabilities. In B. Wong (Ed.), Learning about learning disabilities (3rd ed., pp. 405–436). Academic Press.
- DiMaggio, P. (1991). Social structure, institutions, and cultural goods: The case of the United States. In P. Bourdieu & J. S. Coleman (Eds.), Social theory for a changing society (pp. 133–155). Westview Press.
- Domina, T., Penner, A., & Penner, E. (2017). Categorical inequality: Schools as sorting machines. Annual Review of Sociology, 43, 311–330. https://doi.org/10.1146/annurev-soc-060116-053354
- Edwards, R. (2022). Why do academics do unfunded research? Resistance, compliance and identity in the UK neo-liberal university. Studies in Higher Education, 47(4), 904–914. https://doi.org/10.1080/03075079.2020.1817891
- Elliott, C. D., Smith, P., & McCullock, K. (1996). British Ability Scales, BAS II technical manual. National Foundation for Educational Research–Nelson.
- Farquharson, C. (2019). Early education and childcare spending (IFS Briefing Note BN258). Institute for Fiscal Studies. https://ifs.org.uk/sites/default/files/output_url_files/BN258-Early-education-and-childcare-spending.pdf
- Hall, J. (2024a). An introduction to Airbag Moderation: Definition, explanation and real-world examples [Video]. Sage Research Methods. https://doi.org/10.4135/9781529697391
- Hall, J. (2024b). An introduction to Airbag Moderation: Statistically testing hypotheses [Video]. Sage Research Methods. https://doi.org/10.4135/9781529697407
- Hall, J., Eisenstadt, N., Sylva, K., Smith, T., Sammons, P., Smith, G., Evangelou, M., Goff, J., Tanner, E., Agur, M., & Hussey, D. (2015). A review of the services offered by English Sure Start Children’s Centres in 2011 and 2012. Oxford Review of Education, 41(1), 89–104. https://doi.org/10.1080/03054985.2014.1001731
- Hall, J., Malmberg, L.-E., Lindorff, A., Baumann, N., & Sammons, P. (2020). Airbag moderation: The definition and statistical implementation of a new methodological model. International Journal of Research & Method in Education, 43(4), 379–394. https://doi.org/10.1080/1743727X.2020.1735334
- Hall, J., Sammons, P., & Lindorff, A. (2020). Continuing towards international perspectives in Educational Effectiveness Research. In J. Hall, A. Lindorff, & P. Sammons. (Eds.), International perspectives in Educational Effectiveness Research (pp. 383–406). Springer. https://doi.org/10.1007/978-3-030-44810-3_14
- Hall, J., Sylva, K., Melhuish, E., Sammons, P., Siraj-Blatchford, I., & Taggart, B. (2009). The role of pre-school quality in promoting resilience in the cognitive development of young children. Oxford Review of Education, 35(3), 331–352. https://doi.org/10.1080/03054980902934613
- Hall, J., Sylva, K., Sammons, P., Melhuish, E., Siraj-Blatchford, I., & Taggart, B. (2013). Can preschool protect young children’s cognitive and social development? Variation by center quality and duration of attendance. School Effectiveness and School Improvement, 24(2), 155–176. https://doi.org/10.1080/09243453.2012.749793
- Hayes, A. F. (2022). Introduction to mediation, moderation, and conditional process analysis: A regression-based approach (3rd ed.). The Guilford Press.
- Henriksen, E. K., Dillon, J., & Ryder, J. (Eds.). (2015). Understanding student participation and choice in science and technology education. Springer. https://doi.org/10.1007/978-94-007-7793-4
- Hübner, N., Wagner, W., Nagengast, B., & Trautwein, U. (2019). Putting all students in one basket does not produce equality: Gender-specific effects of curricular intensification in upper secondary school. School Effectiveness and School Improvement, 30(3), 261–285. https://doi.org/10.1080/09243453.2018.1504801
- James, L. R., & Brett, J. M. (1984). Mediators, moderators, and tests for mediation. Journal of Applied Psychology, 69(2), 307–321. https://doi.org/10.1037/0021-9010.69.2.307
- Johnson, P. O., & Neyman, J. (1936). Tests of certain linear hypotheses and their application to some educational problems. Statistical Research Memoirs, 1, 57–93.
- Juvonen, J., Lessard, L. M., Rastogi, R., Schacter, H. L., & Smith, D. S. (2019). Promoting social inclusion in educational settings: Challenges and opportunities. Educational Psychologist, 54(4), 250–270. https://doi.org/10.1080/00461520.2019.1655645
- Liben, L. S., & Coyle, E. F. (2014). Chapter three – Developmental interventions to address the STEM gender gap: Exploring intended and unintended consequences. Advances in Child Development and Behavior, 47, 77–115. https://doi.org/10.1016/bs.acdb.2014.06.001
- Ludwig, J., & Miller, D. L. (2007). Does Head Start improve children’s life chances? Evidence from a regression discontinuity design. The Quarterly Journal of Economics, 122(1), 159–208. https://doi.org/10.1162/qjec.122.1.159
- Micha, R., Karageorgou, D., Bakogianni, I., Trichia, E., Whitsel, L. P., Story, M., Peñalvo, J. L., & Mozaffarian, D. (2018). Effectiveness of school food environment policies on children’s dietary behaviors: A systematic review and meta-analysis. PloS ONE, 13(3), Article e0194555. https://doi.org/10.1371/journal.pone.0194555
- Morse, J. M. (2002). Myth #53: Qualitative research is cheap. Qualitative Health Research, 12(10), 1307–1308. https://doi.org/10.1177/1049732302238744
- Muthén, B., Muthén, L., & Asparouhov, T. (2015). Random coefficient regression. https://www.statmodel.com/download/Random_coefficient_regression.pdf
- Muthén, L. K., & Muthén, B. O. (1998–2017). Mplus user’s guide (8th ed.).
- Oakes, J. (2005). Keeping track: How schools structure inequality (2nd ed.). Yale University Press.
- Palardy, G. J. (2013). High school socioeconomic segregation and student attainment. American Educational Research Journal, 50(4), 714–754. https://doi.org/10.3102/0002831213481240
- Pascal, C., Bertram, T., & Cole-Albäck, A. (2021). What do we know about the 30 hour entitlement? Literature review and qualitative stakeholder work. The Sutton Trust. https://www.suttontrust.com/wp-content/uploads/2021/08/What-do-we-know-about-the-30-hour-entitlement-literature-review.pdf
- Piatek-Jimenez, K., Cribbs, J., & Gill, N. (2018). College students’ perceptions of gender stereotypes: Making connections to the underrepresentation of women in STEM fields. International Journal of Science Education, 40(12), 1432–1454. https://doi.org/10.1080/09500693.2018.1482027
- Preacher, K. J., Rucker, D. D., & Hayes, A. F. (2007). Addressing moderated mediation hypotheses: Theory, methods, and prescriptions. Multivariate Behavioral Research, 42(1), 185–227. https://doi.org/10.1080/00273170701341316
- Ridgeway, T. R., Price, D. P., Simpson, C. G., & Rose, C. A. (2012). Reviewing the roots of response to intervention: Is there enough research to support the promise? Administrative Issues Journal, 2(1), Article 9. https://dc.swosu.edu/aij/vol2/iss1/9
- Sarid, A. (2022). Theoretical contributions to the investigation of educational effectiveness: Towards a dilemmatic approach. Cambridge Journal of Education, 52(1), 117–136. https://doi.org/10.1080/0305764X.2021.1948971
- Saunders, D. R. (1955). The “moderator variable” as a useful tool in prediction. In Proceedings of the Invitational Conference on Testing Problems (pp. 54–58). Educational Testing Service.
- Saunders, D. R. (1956). Moderator variables in prediction. Educational and Psychological Measurement, 16(2), 209–222. https://doi.org/10.1177/001316445601600205
- Stein, A., Malmberg, L.-E., Leach, P., Barnes, J., Sylva, K., & the FCCC Team. (2013). The influence of different forms of early childcare on children’s emotional and behavioural development at school entry. Child: Care, Health and Development, 39(5), 676–687. https://doi.org/10.1111/j.1365-2214.2012.01421.x
- Sylva, K., Stein, A., Leach, P., Barnes, J., Malmberg, L.-E., & the FCCC-team. (2007). Family and child factors related to the use of non-maternal infant care: An English study. Early Childhood Research Quarterly, 22(1), 118–136. https://doi.org/10.1016/j.ecresq.2006.11.003
- Sylva, K., Stein, A., Leach, P., Barnes, J., Malmberg, L.-E., & the FCCC-team. (2011). Effects of early child-care on cognition, language, and task-related behaviours at 18 months: An English study. British Journal of Developmental Psychology, 29(1), 18–45. https://doi.org/10.1348/026151010X533229
- Wang, M.-T., & Degol, J. (2013). Motivational pathways to STEM career choices: Using expectancy–value perspective to understand individual and gender differences in STEM fields. Developmental Review, 33(4), 304–340. https://doi.org/10.1016/j.dr.2013.08.001
- Wu, J.-Y., & Kwok, O. (2012). Using SEM to analyze complex survey data: A comparison between design-based single-level and model-based multilevel approaches. Structural Equation Modeling: A Multidisciplinary Journal, 19(1), 16–35. https://doi.org/10.1080/10705511.2012.634703
- Zuckerman, H. (2010). Dynamik und Verbreitung des Matthäus-Effekts: Eine kleine soziologische Bedeutungslehre [The Matthew effect writ large and larger: A study in sociological semantics]. Berliner Journal für Soziologie, 20(3), 309–340. https://doi.org/10.1007/s11609-010-0133-9