ABSTRACT
Purpose
To describe the future steps and advances in the field of ocular imaging in uveitis
Methods
Narrative review
Results
There have been numerous advances in the field of imaging in uveitis in the past decade. Advanced techniques of imaging of the vitreous, vitreo-retinal interface, retinochoroid, and the sclera can provide significant information that helps in understanding the disease pathogenesis and manifestations. Imaging also helps in establishing a diagnosis in challenging cases, along with the laboratory and other assays. Notable developments in ocular imaging include wide-field and ultra-wide field imaging (including angiographies), automated quantification of the retinochoroidal vasculature using optical coherence tomography (OCT) and OCT angiography, quantification of vitreous cells, and intraoperative use of imaging in uveitis, among others.
Conclusions
We have summarized several technological achievements in ocular imaging in the field of uveitis and provided insights into the potential future developments.
Uveitis is a potentially sight-threatening condition responsible for about 10–15% of cases of legal blindness in the USA and up to 25% of cases of legal blindness in the developing world.Citation1 Considering its burden, efficient management of uveitis depends on proper classification and grading of the severity.
Several novel diagnostic and therapeutic resources for uveitis specialists have been introduced in the last decade, and others are in the pipeline in the upcoming years. On the one hand, state-of-the-art imaging devices have now been regularly employed in the clinical practice and have ushered a new era into the depiction of the retinal periphery and its vascular system.Citation2,Citation3 New sequencing and molecular tools have been developed, leading to a sensitive, unbiased, and comprehensive approach to the diagnosis of uveitis.Citation4
Despite continuous efforts in improving and standardizing the management of ocular inflammatory diseases, there are still unaddressed issues to be solved. Imaging of the retinal periphery has been limited so far by optical distortions, poor imaging definition, motion artifacts, narrow depth penetration, and increased scan time. Notwithstanding proposed standardized severity scales,Citation5 the grading of uveitis is currently assessed by slit-lamp examination and it is subjective and open to interobserver variation.Citation6,Citation7
The scope of this paper is to review the next steps in diagnosing, classifying, and treating patients with uveitis. Innovative approaches include the application of new imaging modalities to the clinical practice, as well as the development of novel applications of conventional ocular imaging and digital elaboration of conventional data (such as artificial intelligence). The final scope of these advancements is to design specific imaging-based diagnostic and treatment algorithms for the standardized, replicable management of uveitis among different practices and populations.
Applications of new imaging modalities
Posterior uveitis can be present with widespread involvement of the retina, including the far periphery. Although peripheral lesions might not affect central vision, their evaluation gives information for the differential diagnosis and contributes to detecting uveitis activity. Therefore, meticulous examination of the retinal mid- and far periphery may substantially impact the treatment and the prognosis of the underlying disease. Until recently, the field of view of conventional imaging devices have limited the study of peripheral lesions. The advent of ultra-widefield optical coherence tomography (UWF-OCT) devices and widefield OCT angiography (OCTA) have provided new information about the retinal and choroidal peripheral lesions and their associated vascular changes.
Ultrawide field optical coherence tomography (OCT)
Optos Silverstone UWF camera (Optos PLC; Dunfermline, UK) has embedded a swept-source OCT device with a 1,050-nm wavelength, a < 7-micron axial resolution, and a 100,000 A scans per second scanning speed. The scan is registered onto a 200° single-capture scanning laser ophthalmoscope red/green pseudo-colored UWF image and allows to scan the retina in a relatively fast, reliable, and straightforward manner.Citation8 In a series of 125 eyes, only 2% had pathologies that were not accessible by the UWF-OCT device.Citation8
The vitreous, the vitreoretinal interface, the retinal layers, and the choroid can be clearly visualized up to and beyond the anterior border of the vortex veins. shows a patient with fungal endophthalmitis; UWF-OCT shows vitritis and a white infiltrate on the surface of the retina, invading the vitreous chamber.Citation9 shows a patient with syphilitic .retinochoroiditis UWF-OCT contributed to establishing the activity of inflammation, revealing subretinal hyper-reflective material associated with active choroiditis. shows the UWF-OCT scan of a patient with birdshot retinochoroiditis and that of toxoplasma retinochoroiditis in an immunocompromised patient.
Figure 1. Candida endophthalmitis. Ultra-widefield pseudocolor shows vitritis and a white infiltrate on the surface of the retina, invading the vitreous chamber. The optical coherence tomography of infiltrate confirms its proximity to the retina.
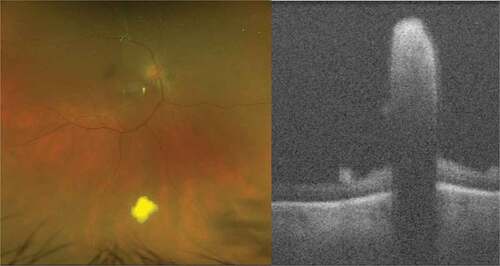
Figure 2. Syphilitic retinochoroiditis; Ultra-widefield pseudocolor shows multiple foci of choroiditis and optical coherence tomography reveals active inflammation associated with subretinal hyper-reflective material.
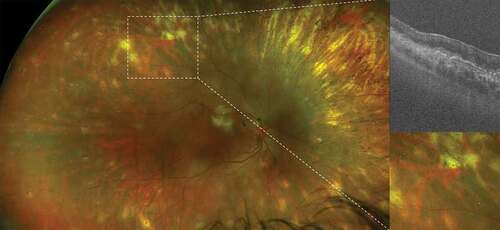
Figure 3. Birdshot retinochoroiditis. Ultra-widefield pseudocolor shows perivascular sheathing of retinal vessels; fluorescein angiography reveals diffuse retinal vasculitis and papillitis; indocyanine green angiography shows hypofluorescent foci of choroiditis, correspond to large hyporeflective areas within the choroid (asterisks) on optical coherence tomography (green line).
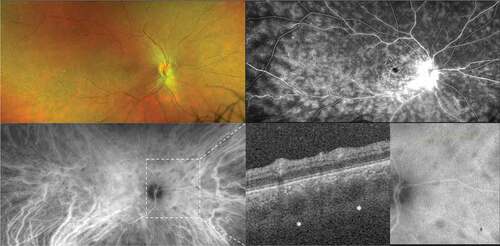
Figure 4. Toxoplasma retinochoroiditis in an immunocompromised patient. Ultra-widefield pseudocolor shows active patches of retinochoroiditis, which appears hyperreflective on optical coherence tomography. The presence of toxoplasma was confirmed by polymerase chain reaction (PCR) on an aqueous tap. The image in the bottom right shows the healed retinochoroiditis.
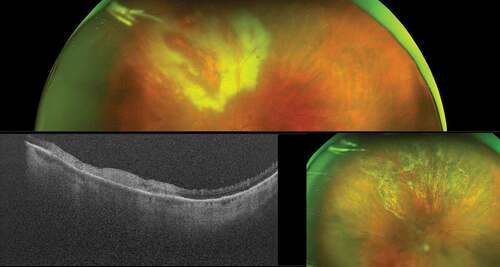
The use of this new tool has the potential to expand our understanding of various posterior-segment inflammatory diseases and to monitor the response to treatment.
Widefield optical coherence tomography angiography (OCTA)
Many reports have been published so far on the usefulness of OCTA in uveitis regarding patients with ischemic retinal vasculitis, choroiditis, and neovascular complications of uveitis.Citation10–13 OCTA devices have been improving in speed, field of view, and image quality, increasing their utility and application in clinical practice. OCTA segmentation software has also been becoming increasingly precise.
Alongside, widefield OCTA has been introduced to overcome the narrow field of view of traditional OCTA imaging devices. Widefield OCTA detects vascular perfusion abnormalities, peripheral vasculitis, and mid-peripheral choroidal granuloma.Citation11,Citation14 Given its potential in detecting retinal and choroidal pathology, widefield OCTA may potentially replace invasive dye imaging modalities such as fluorescein angiography and indocyanine green angiography in diagnosing and monitoring inflammatory retinal diseases. Improvements in speed, quality and field of view of OCTA devices are still required for a broader diffusion of this technique in uveitis.
Novel applications of conventional ocular imaging
Quantification of vitritis
OCT imaging in uveitis has been focused so far on the retinal morphology, evaluating the presence of retinal and choroidal inflammatory lesions, macular edema, epiretinal membrane, and macular neovascularization and their response to treatment.Citation15–19 More recently, OCT scans have been employed to study the choroid and its quantitative metrics; the choroidal thickness and the choroidal vascularity index correlate with disease activity in posterior uveitis.Citation20–27
The OCT scans have also been applied to objective vitritis quantification. Since the Standardization of Uveitis Nomenclature (SUN) Workshop in 2005,Citation5 the gold standard for measuring vitreous haze has been the National Eye Institute vitreous haze (NEI VH) scale.Citation28 In this system, the fundus is compared with a set of six photographs and scored accordingly. Though recognized as a surrogate endpoint by the Food and Drug Administration (FDA) and the European Medical Agency (EMA), the NEI VH scale is subjective and based on a non-continuous grading system. Furthermore, it is flawed by moderate interobserver agreement and poor discriminatory capacity at lower levels of vitreous haze. To overcome these limitations, more objective instrument-based systems, such as retinal photography and OCT, have been proposed for measuring vitritis.Citation29 Retinal photography has been implemented into a 9-point scale (i.e., the Miami scale) using calibrated filters to blur fundus photographs,Citation30 allowing to detect more subtle differences within grades compared to the 6-point NEI VH scale in an operator-independent fashion.Citation30,Citation31
OCT scans have been used to derive continuous indices of vitritis by measuring the vitreous signal intensity, such as the Vitreous/RPE-relative intensity (VRI). The VRI is calculated as the absolute vitreous signal normalized to the RPE signal, which compensates for global reduction in the signal strength deriving in the case of media opacities.Citation32 The VRI is higher in eyes with active uveitis, positively correlates with vitreous opacity detected on dilated fundus exam,Citation32 and sensitively changes in response to steroid treatment.Citation33 This method is currently under validation; it is replicable under different imaging conditionsCitation34 and recent adjustments, based on a fully automated algorithm, have been proposed to improve its reliability.Citation35
A similar OCT-based approach has been applied to assess anterior chamber (AC) inflammation. In a study by Invernizzi et al., the aqueous-to-air relative intensity (ARI) index, i.e., the ratio between the intensity OCT values for the aqueous and air spaces, was shown to correlate with the clinical grading of the anterior chamber cells and flare.Citation36 The ARI index may replace conventional methods of flare quantification, such as flare laser photometry, whose use is limited by costs and low repeatability of results.Citation37
In summary, validating a widely available instrument, such as OCT, for the simultaneous measurement of AC cells, flare, and vitreous haze holds the potential for a rapid, reliable, and comprehensive assessment of intraocular inflammation.
Clinical applications of intraoperative OCT
Intraoperative OCT (iOCT) in vitreoretinal surgery is compelling in uveitic patients. Uveitis carries a relatively high risk of secondary vitreoretinal complications, including epiretinal membrane formation and retinal detachment (RD), which may result in irreversible visual loss.Citation38,Citation39 Moreover, surgical management of uveitis-related complications is more challenging and burdened by worse outcomes than non-uveitic patients.Citation38,Citation39
iOCT has been studied in various anteriorCitation40 and posteriorCitation41 segment surgical scenarios, but its use in uveitis patients has been limited.Citation42 Recently, a prospective study has supported the use of iOCT for surgical decision-making in 74 eyes with uveitis undergoing vitreoretinal surgery,Citation43 further increasing the interest in the development of high-quality live intraoperative imaging technologies for managing uveitis complications.
Novel applications of fundus autofluorescence
Conventional blue-light (488 nm) fundus autofluorescence (FAF) had shed light on the pathogenesis, diagnostic, and prognostic management of various uveitic entities.Citation44 Recent advances in this imaging modality are being developed and their application to the field of ocular immunopathology is currently underway.
Novel FAF technologies include color FAF or spectrally resolved FAF.Citation45 In color FAF, a confocal device excites the retinal fluorophores with a 450-nm-wavelength blue-light FAF and detects the full emission spectrum into long-wave and short-wave components (“red” and “green,” respectively). The application of color FAF to uveitis has yet to be tested.
Quantitative fundus autofluorescence (qAF) is a standardized approach that combines objective measurement of FAF intensity with spatial information.Citation46 qAF has been widely used in diagnosing and monitoring inherited retinal diseases, being employed as an outcome measure in clinical trials.Citation47 The only published study employing qAF in uveitis was conducted on acute zonal occult outer retinopathy (AZOOR),Citation48 with objective validation of the previously reported trizonal pattern.Citation49 Further studies are needed to determine whether qAF has a clinical utility in other uveitic diseases.
Fluorescence Lifetime Imaging Ophthalmoscopy (FLIO) measures the autofluorescence lifetime of the retinal fluorophores, that is, how long these molecules emit light after laser stimulation.Citation50 The emission time is then represented as a color-coded map. Normal retina displays a combination of warm and cold-colored areas; each eye condition creates a specific pattern that may help in the differential diagnosis and/or detect disease progression. Preliminary encouraging results of this technology have been reported in patients with uveitis.Citation51
Digital elaboration of conventional data
Recent advances in machine learning and deep learning have enabled artificial intelligence (AI)-based software to be trained on a large amount of imaging data and screen for retinal diseases with an accuracy equaling and even exceeding human performance. AI methods in the retina have been applied mainly on fundus photography, but recent studies have also supported the role of AI on OCT and OCTA imaging. AI-based technology has been developed for three main medical tasks: classification (automated diagnosis, disease stage, disease type, screening), segmentation (automatic delineation of fluid, fovea, retinal vessels, retinal layers, exudates, optic disc cup), and prediction (demographic data, clinical data, disease progression, treatment outcome).Citation52
AI methods have been successfully applied in diabetic retinopathy,Citation53 age-related macular degeneration,Citation54 and glaucoma,Citation55 since large population-based image datasets were available for these diseases. Uveitis has limitations that preclude creating large-scale data repositories, such as lack of standardization of nomenclature and coding, absence of error-checking and data quality in the available data sources, and poor generalizability of findings to different ethnicities and populations. For these reasons, the application of AI-methods to uveitis has been lagging behind other, more common, retinal conditions.
AI-methods have been mainly applied to uveitis for classification purposes; while diagnostic criteria emphasize sensitivity, classification criteria emphasize specificity and seek to minimize misclassification. In April 2021, the SUN Working Group announced a collection of papers containing the AI-based classification criteria for 25 different uveitis entities.Citation56 The objective was proposing validated standardized criteria to define specific uveitic conditions in upcoming clinical studies. The project proceeded in four stages: 1) informatics (development of a standardized vocabulary and a standardized, hierarchical classification instrument),Citation2 case collection (collection of a preliminary pool of 5,766 cases),Citation3 case selection (selection of final 4,046 cases), andCitation4 machine learning. Machine learning was accomplished by randomly separating the database into a learning set (∼85% of the cases) and a validation set (∼15% of the cases) for each disease. The learning set was used to scrutinize and select the diagnostic criteria. The validation set was used to test the proposed criteria; for both the learning set and the validation set, the misclassification rate was calculated as the proportion of cases classified incorrectly by the machine learning algorithm when compared to the consensus human-based diagnosis. Overall accuracies by uveitic class in the validation set were as follows: anterior uveitides 96.7%; intermediate uveitides 99.3%; posterior uveitides 98.0%; panuveitides 94.0%; and infectious posterior uveitides/panuveitides 93.3%. The misclassification rate in the validation set was 0% or very low for anterior viral uveitides,Citation57–59 intermediate uvetitis,Citation60 retinal vasculitis,Citation61 infectious retinitis,Citation62–64 white dot syndromes,Citation65–68 and stromal choroiditis.Citation69,Citation70
The next steps for AI in uveitis include disease grading and prediction. Sun et al. recently described an AI model capable of objective evaluation and three-level severity grading of experimental autoimmune uveitis (EAU) in murine models.Citation71 Earlier this year, a deep learning-based OCT method for automated scoring of ocular inflammation in experimental mycobacterial uveitis (PMU) animal models has been proposed, but the automated classification did not perform as well as human graders.Citation72
Deep learning models have undeniable advantages in at least three aspects:Citation1 eliminating inter- and intra-observer variability;Citation2 accuracy in successfully learning complex features and patterns to achieve a high rate of accuracy; andCitation3 cost-effectiveness in reducing the tedious and costly process of annotations by human operators. Nevertheless, additional studies are needed to address the need for widely accepted and validated AI-based diagnostic criteria in uveitis.
Next steps: imaging-based diagnostic and treatment algorithms
With the introduction of such a broad armamentarium of different imaging techniques and elaboration systems, the next challenge for uveitis specialists is how to make their best use in clinical practice. The hope is to create automatized, digital systems capable of putting together all the available information and produce imaging-based diagnostic and treatment algorithms. Standardized algorithms can assist clinicians in decision-making and therapeutic monitoring. represents an example of diagnostic and treating algorithm applied to epiretinal and optic disc neovascularization in uveitisCitation73; color fundus picture, fluorescein angiography findings (e.g., peripheral ischemia or leakage), patients’ demographics and clinical characteristics, OCT, and OCTA findings are all potential information that may implement this flowchart, optimizing the treatment outcomes.
Conclusions
The field of diagnostic imaging in uveitis is rapidly expanding with continuous technological innovations and the introduction of AI and machine learning. With these tools now available in research laboratories around the world, it is possible to feed the patient images into automated or semi-automated algorithms to generate quantitative and qualitative data. The data from these techniques helps immensely in monitoring the disease activity, deciding therapeutic interventions and in establishing the diagnosis in challenging cases. The SUN working group has already proved the utility of AI and machine learning in updating the classification systems for various uveitic entities. Faster acquisition times and wide field of view is now possible in our clinics providing the uveitis specialist with a wide range of diagnostic devices to choose from. The patients can undergo a comprehensive evaluation that leads to a complete understanding of the patho-anatomical alterations due to the ocular inflammatory disease.
In the future, imaging biomarkers are likely to be as relevant as diagnostic laboratory tests and polymerase chain reactions in the diagnosis and management of subjects non-invasively. While certain tools such as OCT are now considered essential in the management of uveitis, there is no doubt that OCTA and wide field imaging including angiography are desirable in the clinical setting. These technologies bring with them the hope for improved outcomes for our patients with uveitis.
Disclosure statement
No potential conflict of interest was reported by the authors.
Additional information
Funding
References
- Tsirouki T, Dastiridou A, Symeonidis C, et al. A focus on the epidemiology of uveitis. Ocul Immunol Inflamm. 2018;26(1):2–16. doi:10.1080/09273948.2016.1196713.
- Kalra G, Pichi F, Kumar Menia N, et al. Recent advances in wide field and ultrawide field optical coherence tomography angiography in retinochoroidal pathologies. Expert Rev Med Devices. 2021;18(4):375–386. doi:10.1080/17434440.2021.1902301.
- Deak GG, Zhou M, Sporysheva A, Goldstein DA. Novel imaging modalities in patients with uveitis. Can J Ophthalmol. 2020;55(1):20–29. doi:10.1016/j.jcjo.2019.06.005.
- Valdes L, Bispo P, Sobrin L. Application of metagenomic sequencing in the diagnosis of infectious uveitis. Semin Ophthalmol. 2020;35(5–6):276–279. doi:10.1080/08820538.2020.1818795.
- Jabs DA, Nussenblatt RB, Rosenbaum JT. Standardization of uveitis nomenclature working g. standardization of uveitis nomenclature for reporting clinical data. Results of the first international workshop. Am J Ophthalmol. 2005;140:509–516.
- Kempen JH, Ganesh SK, Sangwan VS, Rathinam SR. Interobserver agreement in grading activity and site of inflammation in eyes of patients with uveitis. Am J Ophthalmol. 2008;146(6):813–8 e1. doi:10.1016/j.ajo.2008.06.004.
- Hornbeak DM, Payal A, Pistilli M, et al. Interobserver agreement in clinical grading of vitreous haze using alternative grading scales. Ophthalmology. 2014;121(8):1643–1648. doi:10.1016/j.ophtha.2014.02.018.
- Sodhi SK, Golding J, Trimboli C, Choudhry N. Feasibility of peripheral OCT imaging using a novel integrated SLO ultra-widefield imaging swept-source OCT device. Int Ophthalmol. 2021;41(8):2805–2815. doi:10.1007/s10792-021-01837-7.
- Invernizzi A, Symes R, Miserocchi E, et al. Spectral domain optical coherence tomography findings in endogenous candida endophthalmitis and their clinical relevance. Retina. 2018;38(5):1011–1018. doi:10.1097/IAE.0000000000001630.
- Marchese A, Miserocchi E, Modorati G, et al. Widefield OCT angiography of idiopathic retinal vasculitis, aneurysms, and neuroretinitis. Ophthalmol Retina. 2017;1(6):567–569. doi:10.1016/j.oret.2017.03.010.
- Pichi F, Smith SD, Neri P, et al. Choroidal granulomas visualized by swept-source optical coherence tomography angiography. Retina. 2021;41(3):602–609. doi:10.1097/IAE.0000000000002864.
- Pichi F, Sarraf D, Arepalli S, et al. The application of optical coherence tomography angiography in uveitis and inflammatory eye diseases. Prog Retin Eye Res. 2017;59:178–201. doi:10.1016/j.preteyeres.2017.04.005.
- Agarwal A, Aggarwal K, Mandadi SKR, et al. Longitudinal follow-up of tubercular serpiginous-like choroiditis using optical coherence tomography angiography. Retina. 2021;41(4):793–803. doi:10.1097/IAE.0000000000002915.
- Noori J, Shi Y, Yang J, et al. A novel method to detect and monitor retinal vasculitis using swept-source OCT angiography. Ophthalmol Retina. 2021;5(12):1226–1234. doi:10.1016/j.oret.2021.02.007.
- Markomichelakis NN, Halkiadakis I, Pantelia E, et al. Patterns of macular edema in patients with uveitis: qualitative and quantitative assessment using optical coherence tomography. Ophthalmology. 2004;111(5):946–953. doi:10.1016/j.ophtha.2003.08.037.
- Gupta V, Gupta P, Singh R, Dogra MR, Gupta A. Spectral-domain Cirrus high-definition optical coherence tomography is better than time-domain Stratus optical coherence tomography for evaluation of macular pathologic features in uveitis. Am J Ophthalmol. 2008;145(6):1018–1022. doi:10.1016/j.ajo.2008.01.021.
- Nazari H, Dustin L, Heussen FM, Sadda S, Rao NA. Morphometric spectral-domain optical coherence tomography features of epiretinal membrane correlate with visual acuity in patients with uveitis. Am J Ophthalmol. 2012;154(1):78–86 e1. doi:10.1016/j.ajo.2012.01.032.
- Invernizzi A, Agarwal AK, Ravera V, et al. Comparing optical coherence tomography findings in different aetiologies of infectious necrotising retinitis. Br J Ophthalmol. 2018;102(4):433–437. doi:10.1136/bjophthalmol-2017-310210.
- Zicarelli F, Azzolini C, Cornish E, et al. Optical coherence tomography features of choroidal neovascularization and their correlation with age, gender, and underlying disease. Retina. 2021;41(5):1076–1083. doi:10.1097/IAE.0000000000002984.
- Baltmr A, Lightman S, Tomkins-Netzer O. Examining the choroid in ocular inflammation: a focus on enhanced depth imaging. J Ophthalmol. 2014;2014:459136. doi:10.1155/2014/459136.
- Sonoda S, Sakamoto T, Shirasawa M, Yamashita T, Otsuka H, Terasaki H. Correlation between reflectivity of subretinal fluid in OCT images and concentration of intravitreal VEGF in eyes with diabetic macular edema. Invest Ophthalmol Vis Sci. 2013;54(8):5367–5374. doi:10.1167/iovs.13-12382.
- Neudorfer M, Weinberg A, Loewenstein A, Barak A. Differential optical density of subretinal spaces. Invest Ophthalmol Vis Sci. 2012;53(6):3104–3110. doi:10.1167/iovs.11-8700.
- Murakami T, Nishijima K, Akagi T, et al. Optical coherence tomographic reflectivity of photoreceptors beneath cystoid spaces in diabetic macular edema. Invest Ophthalmol Vis Sci. 2012;53(3):1506–1511. doi:10.1167/iovs.11-9231.
- Horii T, Murakami T, Nishijima K, et al. Relationship between fluorescein pooling and optical coherence tomographic reflectivity of cystoid spaces in diabetic macular edema. Ophthalmology. 2012;119(5):1047–1055. doi:10.1016/j.ophtha.2011.10.030.
- Barthelmes D, Sutter FK, Gillies MC. Differential optical densities of intraretinal spaces. Invest Ophthalmol Vis Sci. 2008;49(8):3529–3534. doi:10.1167/iovs.07-1320.
- Barthelmes D, Gillies MC, Sutter FK. Quantitative OCT analysis of idiopathic perifoveal telangiectasia. Invest Ophthalmol Vis Sci. 2008;49(5):2156–2162. doi:10.1167/iovs.07-0478.
- Ahlers C, Golbaz I, Einwallner E, et al. Identification of optical density ratios in subretinal fluid as a clinically relevant biomarker in exudative macular disease. Invest Ophthalmol Vis Sci. 2009;50(7):3417–3424. doi:10.1167/iovs.08-2759.
- Nussenblatt RB, Palestine AG, Chan CC, Roberge F. Standardization of vitreal inflammatory activity in intermediate and posterior uveitis. Ophthalmology. 1985;92(4):467–471. doi:10.1016/S0161-6420(85)34001-0.
- Liu X, Hui BT, Way C, et al. Noninvasive instrument-based tests for detecting and measuring vitreous inflammation in uveitis: a systematic review. Ocul Immunol Inflamm. 2020:1–12. doi:10.1080/09273948.2020.1808228.
- Davis JL, Madow B, Cornett J, et al. Scale for photographic grading of vitreous haze in uveitis. Am J Ophthalmol. 2010;150(5):637–41 e1. doi:10.1016/j.ajo.2010.05.036.
- Madow B, Galor A, Feuer WJ, Altaweel MM, Davis JL. Validation of a photographic vitreous haze grading technique for clinical trials in uveitis. Am J Ophthalmol. 2011;152(2):170–6 e1. doi:10.1016/j.ajo.2011.01.058.
- Keane PA, Karampelas M, Sim DA, et al. Objective measurement of vitreous inflammation using optical coherence tomography. Ophthalmology. 2014;121(9):1706–1714. doi:10.1016/j.ophtha.2014.03.006.
- Sreekantam S, Macdonald T, Keane PA, Sim DA, Murray PI, Denniston AK. Quantitative analysis of vitreous inflammation using optical coherence tomography in patients receiving sub-Tenon’s triamcinolone acetonide for uveitic cystoid macular oedema. Br J Ophthalmol. 2017;101(2):175–179. doi:10.1136/bjophthalmol-2015-308008.
- Montesano G, Way CM, Ometto G, et al. Optimizing OCT acquisition parameters for assessments of vitreous haze for application in uveitis. Sci Rep. 2018;8(1):1648. doi:10.1038/s41598-018-20092-y.
- Keane PA, Balaskas K, Sim DA, et al. Automated analysis of vitreous inflammation using spectral-domain optical coherence tomography. Transl Vis Sci Technol. 2015;4(5):4. doi:10.1167/tvst.4.5.4.
- Invernizzi A, Marchi S, Aldigeri R, et al. Objective quantification of anterior chamber inflammation: measuring cells and flare by anterior segment optical coherence tomography. Ophthalmology. 2017;124(11):1670–1677. doi:10.1016/j.ophtha.2017.05.013.
- Wakefield D, Herbort CP, Tugal-Tutkun I, Zierhut M. Controversies in ocular inflammation and immunology laser flare photometry. Ocul Immunol Inflamm. 2010;18(5):334–340. doi:10.3109/09273948.2010.512994.
- Branson SV, McClafferty BR, Kurup SK. Vitrectomy for epiretinal membranes and macular holes in uveitis patients. J Ocul Pharmacol Ther. 2017;33(4):298–303. doi:10.1089/jop.2016.0142.
- Kerkhoff FT, Lamberts QJ, van den Biesen PR, Rothova A. Rhegmatogenous retinal detachment and uveitis. Ophthalmology. 2003;110(2):427–431. doi:10.1016/S0161-6420(02)01744-X.
- Juthani VV, Goshe JM, Srivastava SK, Ehlers JP. Association between transient interface fluid on intraoperative OCT and textural interface opacity after DSAEK surgery in the PIONEER study. Cornea. 2014;33(9):887–892. doi:10.1097/ICO.0000000000000209.
- Ehlers JP, Modi YS, Pecen PE, et al. The DISCOVER study 3-year results: feasibility and usefulness of microscope-integrated intraoperative OCT during ophthalmic surgery. Ophthalmology. 2018;125(7):1014–1027. doi:10.1016/j.ophtha.2017.12.037.
- Browne AW, Ehlers JP, Sharma S, Srivastava SK. Intraoperative optical coherence tomography-assisted chorioretinal biopsy in the DISCOVER study. Retina. 2017;37(11):2183–2187. doi:10.1097/IAE.0000000000001522.
- Kumar JB, Ehlers JP, Sharma S, Srivastava SK. Intraoperative OCT for uveitis-related vitreoretinal surgery in the DISCOVER study. Ophthalmol Retina. 2018;2(10):1041–1049. doi:10.1016/j.oret.2018.02.013.
- Marchese A, Agarwal A, Moretti AG, et al. Advances in imaging of uveitis. Ther Adv Ophthalmol. 2020;12:2515841420917781. doi:10.1177/2515841420917781.
- Borrelli E, Battista M, Zuccaro B, et al. Spectrally resolved fundus autofluorescence in healthy eyes: repeatability and topographical analysis of the green-emitting fluorophores. J Clin Med. 2020;9(8):2388. doi:10.3390/jcm9082388.
- Delori F, Greenberg JP, Woods RL, et al. Quantitative measurements of autofluorescence with the scanning laser ophthalmoscope. Invest Ophthalmol Vis Sci. 2011;52(13):9379–9390. doi:10.1167/iovs.11-8319.
- Sparrow JR, Duncker T, Schuerch K, Paavo M, de Carvalho JRL Jr. Lessons learned from quantitative fundus autofluorescence. Prog Retin Eye Res. 2020;74:100774. doi:10.1016/j.preteyeres.2019.100774.
- Boudreault KA, Schuerch K, Zhao J, et al. Quantitative autofluorescence intensities in acute zonal occult outer retinopathy vs healthy eyes. JAMA Ophthalmol. 2017;135(12):1330–1338. doi:10.1001/jamaophthalmol.2017.4499.
- Mrejen S, Khan S, Gallego-Pinazo R, Jampol LM, Yannuzzi LA. Acute zonal occult outer retinopathy: a classification based on multimodal imaging. JAMA Ophthalmol. 2014;132(9):1089–1098. doi:10.1001/jamaophthalmol.2014.1683.
- Sauer L, Vitale AS, Modersitzki NK, Bernstein PS. Fluorescence lifetime imaging ophthalmoscopy: autofluorescence imaging and beyond. Eye (Lond). 2021;35(1):93–109. doi:10.1038/s41433-020-01287-y.
- Vitale A, Sauer L, Modersitzki NK, et al. A phenotypic analysis of uveitic diseases using fluorescence lifetime imaging ophthalmoscopy (FLIO). Invest Ophthalmol Vis Sci. 2021;62:2315.
- Schmidt-Erfurth U, Sadeghipour A, Gerendas BS, Waldstein SM, Bogunovic H. Artificial intelligence in retina. Prog Retin Eye Res. 2018;67:1–29. doi:10.1016/j.preteyeres.2018.07.004.
- Gulshan V, Peng L, Coram M, et al. Development and validation of a deep learning algorithm for detection of diabetic retinopathy in retinal fundus photographs. JAMA. 2016;316(22):2402–2410. doi:10.1001/jama.2016.17216.
- Burlina PM, Joshi N, Pekala M, Pacheco KD, Freund DE, Bressler NM. Automated grading of age-related macular degeneration from color fundus images using deep convolutional neural networks. JAMA Ophthalmol. 2017;135(11):1170–1176. doi:10.1001/jamaophthalmol.2017.3782.
- Kim KE, Kim JM, Song JE, Kee C, Han JC, Hyun SH. Development and validation of a deep learning system for diagnosing glaucoma using optical coherence tomography. J Clin Med. 2020;9(7):2167.
- Standardization of Uveitis Nomenclature Working G. Development of classification criteria for the uveitides. Am J Ophthalmol. 2021;228:96–105. doi:10.1016/j.ajo.2021.03.061.
- Standardization of Uveitis Nomenclature Working G. Classification criteria for cytomegalovirus anterior uveitis. Am J Ophthalmol. 2021;228:89–95. doi:10.1016/j.ajo.2021.03.060.
- Standardization of Uveitis Nomenclature Working G. Classification criteria for varicella zoster virus anterior uveitis. Am J Ophthalmol. 2021;228:165–173. doi:10.1016/j.ajo.2021.03.037.
- Standardization of Uveitis Nomenclature Working G. Classification criteria for herpes simplex virus anterior uveitis. Am J Ophthalmol. 2021;228:231–236. doi:10.1016/j.ajo.2021.03.053.
- Standardization of Uveitis Nomenclature Working G. Classification criteria for multiple sclerosis-associated intermediate uveitis. Am J Ophthalmol. 2021;228:72–79. doi:10.1016/j.ajo.2021.03.044.
- Standardization of Uveitis Nomenclature Working G. Classification criteria for behcet disease uveitis. Am J Ophthalmol. 2021;228:80–88. doi:10.1016/j.ajo.2021.03.058.
- Jabs DA, Belfort R Jr., Bodaghi B, et al. Classification criteria for syphilitic uveitis. Am J Ophthalmol. 2021;228:182–191.
- Standardization of Uveitis Nomenclature Working G. Classification criteria for tubercular uveitis. Am J Ophthalmol. 2021;228:142–151. doi:10.1016/j.ajo.2021.03.040.
- Standardization of Uveitis Nomenclature Working G. Classification criteria for toxoplasmic retinitis. Am J Ophthalmol. 2021;228:134–141. doi:10.1016/j.ajo.2021.03.042.
- Standardization of Uveitis Nomenclature Working G. Classification criteria for birdshot chorioretinitis. Am J Ophthalmol. 2021;228:65–71. doi:10.1016/j.ajo.2021.03.059.
- Standardization of Uveitis Nomenclature Working G. Classification criteria for serpiginous choroiditis. Am J Ophthalmol. 2021;228:126–133. doi:10.1016/j.ajo.2021.03.038.
- Standardization of Uveitis Nomenclature Working G. Classification criteria for multifocal choroiditis with panuveitis. Am J Ophthalmol. 2021;228:152–158. doi:10.1016/j.ajo.2021.03.043.
- Standardization of Uveitis Nomenclature Working G. Classification criteria for punctate inner choroiditis. Am J Ophthalmol . 2021;228:275–280.
- Standardization of Uveitis Nomenclature Working G. Classification criteria for vogt-koyanagi-harada disease. Am J Ophthalmol. 2021;228:205–211. doi:10.1016/j.ajo.2021.03.036.
- Standardization of Uveitis Nomenclature Working G. ClassificationCriteriaforSympatheticOphthalmia. Am J Ophthalmol. 2021;228:212–219. doi:10.1016/j.ajo.2021.03.048.
- Sun J, Huang X, Egwuagu C, et al. Identifying mouse autoimmune uveitis from fundus photographs using deep learning. Transl Vis Sci Technol. 2020;9(2):59. doi:10.1167/tvst.9.2.59.
- John S, Na JJ, Swaminathan S, Wilson L, Wu Y, Pepple K. Developing a deep learning classifier for uveitis scoring on mouse OCT images. ARVO Annual Meeting 2021; San Francisco, California.
- Zaguia F, Marchese A, Cicinelli MV, Miller VJ, Miserocchi E, Goldstein DA. Long-term success treating inflammatory epiretinal neovascularization with immunomodulatory therapy. Graefes Arch Clin Exp Ophthalmol. 2021. doi:10.1007/s00417-021-05396-6.