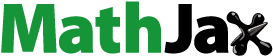
Abstract
The coherent organisation of thematic material into large-scale structures within a composition is an important concept in both traditional and cognitive theories of music. However, empirical evidence supporting their perception is scarce. Providing a more nuanced approach, this paper introduces a computational model of hypothesised cognitive mechanisms underlying perception of large-scale thematic structure. Repetition detection based on statistical learning forms the model's foundation, hypothesising that predictability arising from repetition creates perceived thematic coherence. Measures are produced that characterise structural properties of a corpus of 623 monophonic compositions. Exploratory analysis reveals the extent to which these measures vary systematically and independently.
1. Introduction
Repetition and structure – the two are intrinsically linked in music; the idea that, through repetition and variation of material, large-scale structures can be created has a long history in music theory (Epstein, Citation1980; Meyer, Citation1989). The basic building blocks of this repeated material are small salient landmarks – or motifs – the combination and variation of which create thematic development. The motivic structuring of music in this way is certainly no recent idea; the concept of the motif as the building block of a work can be found in many manuals of composition (even as early as Galeazzi, Citation2012). Through such inclusion of repeated motifs, sections of a composition are explicitly linked and a coherent sense of large-scale unity can be achieved across the work. It is also consistent with the implications of cognitively informed models of music, such as the hierarchical structures of CitationLerdahl & Jackendoff's (Citation1983) Generative Theory of Tonal Music.Footnote1
Large-scale structure – the global organisation of a work's material – encompasses several different concepts. First, a distinction can be made between thematic and tonal structures, the first concerning the structuring of repeated musical material, the second the hierarchical organisation of harmonies relating to key. The effects of large-scale structures over a composition can be summarised by the term coherence – the extent to which all elements of a piece can be considered to form a unified whole – the perception of which, on the part of a listener, requires that a work's material is sufficiently closely related to be experienced as belonging to the same entity.Footnote2 For both thematic and tonal structures, repetition of material plausibly leads to an increase in its perceived salience and a greater sense of coherence. It is large-scale thematic structural coherence with which we are concerned here.
Repetition seems very likely to play an important role in the perception of large-scale thematic structure but has received relatively little attention in empirical research on music perception, with effects that have been reported being rather weak (as reviewed below). In part, this may reflect the lack of a formalised model characterising the cognitive processes involved in perceiving large-scale thematic structure. The present paper presents an initial outline of such a formalised model. The model is based on statistical learning and probabilistic prediction, since repetition plays to the strengths of such accounts of music perception. Motivic salience and repetition can be understood in terms of the positive effect they have on predictions made by a listener as a piece progresses, their development can be viewed as variation according to learned regularities, based either on intra-opus thematic or extra-opus stylistic models. Repetition allows us to create a probabilistic model of the cognitive mechanisms that can be hypothesised to underlie the perception of large-scale thematic structure.
The goal of the present research is to understand the perception of thematic coherence by breaking-down the overall process into its component parts; to logically sequence how this process may function in cognition – and, equally importantly, where it fails, leading to the weak results of past behavioural studies (as reviewed below). For this to be fulfilled, a corpus of Western art music is curated and a model of large-scale thematic structure in music is developed based on the Information Dynamics of Music (IDyOM) framework (Pearce, Citation2005, Citation2018). This model uses repetition-based hypotheses about perception of large-scale thematic structure to develop a set of fundamental components along which thematic structure may be measured, as dictated by the theory, and seeks to demonstrate that these components vary systematically when applied to the corpus, reflecting the inherent variation in thematic structure within the corpus itself.
The paper is organised as follows. First, past empirical work investigating the perception of large-scale thematic structure, repetition and similarity is reviewed in Sections 1.1 and 1.2, and an outline of the IDyOM modelling framework is given in Section 1.3. Second, the corpus to which the model is applied is described in Section 2. Third, in Section 3, the structure of the present model is introduced in detail. Forth, in Section 4, an exploratory analysis of the model's output components, when applied to the corpus, is detailed and the model's effectiveness discussed. Finally, the limitations that exist within the current model, and areas of future work needed to empirically validate it, are discussed in Section 5.
1.1. Large-scale thematic structure
It is through the invocation of concepts of overall structural coherence or sense of unity, both by theorists and in behavioural studies, that a work's large-scale structure is often summarised and judged; such that a work's coherence arises from an affinity between all its material, creating a unified entity. Thus far, research attempting to assess listeners' perception of large-scale thematic structure has tended to approach the matter by disrupting a work's global thematic organisation, reordering sections or performing manipulative interventions. Whether it is through rearranging movements or variationsFootnote3 to disrupt cyclic forms in Konečni (Citation1984) and Gotlieb and Konečni (Citation1985), or by shuffling the order of a movement's internal divisions in Karno and Konečni (Citation1992) and Tillmann and Bigand (Citation1996), these paradigms have reported little or no preference for the original version. Although, in many cases, lack of significance may be attributed to a combination of small sample sizes testing for what may be quite a weak effect. Furthermore, we cannot know whether the original versions were actually more coherent than the manipulated versions. Similar criticisms of Cook (Citation1987) made by Gjerdingen (Citation1999) identify many such methodological shortcomings in the case of tonal coherence.
To introduce more comprehensive disruptions, Tan and Spackman (Citation2005) compared original works to pieces created from three sections of different works and pieces of just one section of a work repeated three times; some sensitivity to general repetition was found, as well as significant differences in perceived ‘unity’ between versions. Relatedly, Eitan and Granot (Citation2008) used stimuli exchanged between corresponding sonata form sections of the opening movements of two Mozart piano sonatas. The new and original versions were rated for perceived coherence and preference between versions – whether ‘the version is a masterpiece’ (Eitan & Granot, Citation2008, p. 405). The original versions were not significantly preferred over the new versions, nor considered more coherent.
The lack of empirical evidence for the ability of listeners to perceive structural coherence on this scale may indicate that the kind of experimental paradigm used up to this point is unable to uncover effects of large-scale coherence. In part, this may reflect the relatively small sample sizes that have plagued many studies. But there is also the additional problematic assumption in many of these studies that the original ordering of the piece is indeed the absolute best possible in terms of coherence; that the composer is infallible and lack of preference for the original equates to no preference for large-scale structure at all. These issues may account for the failure of listeners to distinguish modified and original musical structures; the resulting differences in coherence may be too subtle to give a large enough effect for the samples sizes used. Similar problems can also be found in the application of this paradigm to the perception of song form in popular music (Rolison & Edworthy, Citation2012).
Two linked studies by Granot and Jacoby (Citation2011, Citation2012) employ a new paradigm to try and answer these questions of structural coherence, also removing the focus on the composer's original compositional decisions. The task takes the form of a musical puzzle – disordered sections from a work (Mozart piano sonata first movement (Granot & Jacoby, Citation2011), and Haydn sonata first movement (Granot & Jacoby, Citation2012)) are presented to participants tasked with creating a coherent whole. The analysis of the participants' ordering is not focused on their relation to the original, instead seeking patterns between participants. The approach of Granot and Jacoby (Citation2011) yields encouraging implications for listeners' abilities to perceive large-scale structure, and the importance of thematic considerations; results indicated sensitivity to (form-like) structure, grouping and placement of developmental material, and placement of opening and closing gestures. Distance score measures applied between participants reveal some sensitivity to ‘directionality’; there was agreement as to the relative positioning of sections, even if not in the exact order.
The outcomes of this body of research – in many cases inconclusive – should, at the very least, advocate an approach that can account for a more detailed understanding of the psychological mechanisms involved in the perception of thematic structure. Existing work has operated, on the whole, either in response to music theoretic concepts of form, or without any prior theoretical assumptions as to the disruption of large-scale structure. There exists no comprehensive psychological model of thematic coherence that could provide specific hypotheses for a phenomenon that assumes great importance in music theory and yet has turned out to be quite evasive in empirical research. It is the purpose of the present research to embark on the specification of such a model.
1.2. Repetition and similarity
The hypotheses directing this model are reliant on the ability of listeners to perceive intra-opus repetition of material, and to judge the similarity between such repetitions. The past literature examining these topics, therefore, provides some important insight for the formation of this model.
A large amount of the research on repetition has been reviewed in Margulis (Citation2014). In particular, Margulis (Citation2012) asked participants to identify repetitions within short musical stimuli. The results indicated that not all types of repeat were valued equally, especially in regard to unit length; firstly, repetition within-phrase was more noticeable than that between-phrase (as might be expected), and, secondly, repetition was more noticeable for complete phrases, rather than fragments. For the excerpts used in CitationMargulis's study, repetition detection was found to be optimal for units of about six seconds. It was also found that extra exposures facilitated better repetition detection for longer units; conversely, however, detection for shorter units became impaired. These additional exposures have the effect of shifting attention towards larger time spans, possibly indicating how a motif is established by frequent repetition over short time periods. After the initial exposure, the motif no longer needs to be repeated on such a small scale.
Similarity and categorisation in the music perception literature encompass a vast field, and one that has indispensable elements when considering repetition and structure. Similarity can help to explain how thematic repetitions can be subjected to variation yet still retain their connection to an original thematic idea. Studies of similarity are wide-ranging and, in some cases, quite disparate. This may partly be due to the highly context-specific nature of similarity in music, particularly when judging similarity between material arising from the same work. However, several offerings to similarity research provide noteworthy perspectives on thematic repetition and structure.
The role of similarity in music has been covered in great detail by Deliège (Citation2007), who drew conclusions from research conducted during development of her Cue Abstraction model. CitationDeliège argued that implicit internal similarity contributes to a large range of musical properties, not least the unity or coherence of musical works. Lamont and Dibben (Citation2001) investigated ratings of similarity between pairs of extracts taken from two piano works by Beethoven and Schoenberg. Similarity ratings were found to be primarily based on surface-level features – such as dynamics, articulation, texture and contour – rather than on any deeper features indicating motivic relations. However, they acknowledged that this result may be due to lack of exposure to repetition; with more repetition, affording more thematic coherence and development, valuable contexts for judging similarity may be learned.
Counter to the results produced for Lamont andDibben (Citation2001), Ziv and Eitan (Citation2007) applied a new task to the same stimuli. Participants categorised extracts as belonging to one of two principal themes identified for each work, additionally taking independent ratings of the extent to which the extract belonged to either chosen theme. By comparing listeners' categorisations to published musicological thematic analyses, those for excerpts from the Beethoven work concurred significantly, whereas those for Schoenberg did not. The results of this study indicate an ability of listeners to perceive similarity between the elements of a single composition and, furthermore, highlight the role of musical themes in allowing listeners to perform categorisations of material with a high level of accuracy.
This research suggests, firstly, that listeners are able to perceive repetitions and similarity within compositions – properties that are vital to the model – and, furthermore, that this perception of similarity can contribute to the categorisation of material according to different thematic groups.
1.3. IDyOM
Cognitive modelling of thematic coherence in real music provides advantages over the stimulus manipulations used in existing experimental work. We can avoid making interventions in a work's structure – decisions that have to be motivated by some prior knowledge or expectation about the functioning of form – and pieces are used in their original entity, greatly aiding ecological validity. In modelling large-scale thematic structure, it is possible to propose explicitly and test multiple hypothesised cognitive mechanisms, rather than trying to interpret the implications of one or more experimental manipulations for underlying cognitive mechanisms.
A probabilistic interpretation of music, founded in statistical learning, lends itself particularly well to this task. Using such a conception, we can construct a model of large-scale thematic structure from small base-units of repetition. Repetition can function as it does in practice, allowing for the inclusion of variation and embellishment. Employing statistical learning in this way also provides a fruitful way of operationalising perceived coherence or unity – high intra-opus predictability would indicate greater internal coherence.
The basis of the present model's implementation is the Information Dynamics of Music, or IDyOM model (Pearce, Citation2005, Citation2018). IDyOM estimates how predictable each note-event in a musical sequence is, based on the number of times the event has followed the preceding context in the prior experience of the model. Depending on the configuration, IDyOM learns from a corpus of works, storing the statistical regularities of the corpus' style in a long-term model (LTM), or dynamically within an individual piece of music, storing intra-opus structure in a short-term model (STM), or both of these. The contexts used for prediction have no fixed maximum length, with the model smoothing together the predictions from contexts of different lengths. Given the conditional probability distribution returned for each note-event, information content (IC, the negative log probability) provides a measure of unpredictability – the unexpectedness of the note that actually occurs, given the context and the prior experience of the model. Low IC indicates a predictable event – one where much of the information provided is redundant – whereas high IC indicates an unexpected event.
IDyOM takes as its input musical sequences – here, monophonic melodies are used. Through its implementation of a multiple-viewpoint framework (Conklin & Witten, Citation1995), IDyOM has the ability to generate probabilities based on different representations of the musical surface. For example, viewpoints allow the representation and prediction of pitch structure in music, not only by absolute pitch, but also by interval, scale degree or contour, and the prediction of temporal structures.
IDyOM has been evaluated empirically and with success as a cognitive model of perceptual expectation and uncertainty (Egermann et al., Citation2013; Hansen & Pearce, Citation2014; Hansen et al., Citation2016; Omigie et al., Citation2012, Citation2013; Pearce, Citation2005; Pearce, Ruiz et al., Citation2010; Sauvé et al., Citation2018), boundary perception (Pearce, Müllensiefen et al., Citation2010), metre induction (van der Weij et al., Citation2017), similarity (Pearce & Müllensiefen, Citation2017), memory (Agres et al., Citation2018), emotional response (Egermann et al., Citation2013; Gingras et al., Citation2016) and aesthetic experience (Cheung et al., Citation2019; Gold et al., Citation2019).
While the hypotheses underlying the present approach are sufficiently generalisable that any statistical predictive framework for symbolic music could be applied, IDyOM provides certain features that make it particularly advantageous. Firstly, the ability to configure separately an LTM, trained on the entire corpus, and STM, constructed only for an individual composition, allow a distinction to be made between predictions deriving from inter-opus stylistic (including tonal) knowledge, and predictions based on thematic structure within a work. Secondly, the dynamic nature of IDyOM provides a real-time model of music listening – all predictions are made sequentially as a work progresses – that can be used to simulate online continuous perception of music; a feature that precludes many techniques from Music Information Retrieval. Finally, models generated using different viewpoint representations can be directly compared, providing insight in to the specific representations that are most relevant to the perception of thematic structure.
To outline how IDyOM can form the basis of a model of large-scale thematic structural coherence, a composition with a lot of repeated material will have a low mean IC for the STM, indicating that it has high thematic coherence. Thus IDyOM usefully embodies the hypothesised links between repetition, prediction and thematic coherence. Furthermore, inexact repetitions still have some degree of increased predictability by virtue of the variable-order smoothed Markov modelling of PPM* implemented in IDyOM (Cleary & Teahan, Citation1997), the multiple levels of abstraction provided by the use of different viewpoint representations, and smoothing IC within a moving window. Embellishment of repeated material in accordance with stylistic conventionsFootnote4 can be accounted for by searching for material possessing a low IC for the LTM, making it stylistically coherent (with respect to the corpus), but relatively high IC in the STM, making it thematically less coherent.
2. The corpus
A corpus was constructed to provide an application domain for the computational model of thematic structure.Footnote5 The spread of this corpus is intended to provide a broad representative sample of Western-classical tonal music. Corpus items were included or excluded subject to certain constraints: the works must be completely monophonic – starting with melodic structures avoids the numerous obstacles posed by tracking interrelating thematic material through multiple polyphonic layers; the works must be used in their entirety (or the entirety of a movement) – the model is intended to simulate perception of large-scale structure, so the full structures are required; and, pieces must be of sufficient length that such structures can be considered unambiguously present. Therefore, melodies were only included if they contained in excess of one hundred notes.
The corpus needed for this analysis falls in a somewhat limited area of focus for current digital symbolic music databases; datasets in music perception tend towards smaller, segmented stimuli, while collections of full works are largely polyphonic. The monophonic constraint, in particular, provides a significantly limiting factor in gathering the corpus; works must either be originally composed this way, or – for the majority – manipulated to produce a monophonic line. Therefore, in order to create a corpus of sufficient size and breadth, manipulations to the original sources have been made to extract melodic lines. These monophonic extractions, while certainly not the composers' original compositions, still provide melody lines that are comprehensible works of music on their own and, importantly, retain a large proportion of their thematic structure.
Original scores were gathered primarily from KernScoresFootnote6 MuseScore,Footnote7 and the Classical Archives MIDIFootnote8 collections. Work selection aimed at providing the broadest spread of composition date, balanced with the considerations of selecting types of piece for which the process of extracting a melodic line would be appropriate. The corpus consists of three categories of composition: those (a relatively small number) originally composed for a single-stave instrument and so already monophonic in nature; piano works to which a skyline algorithm (Uitdenbogerd & Zobel, Citation1998) was applied to extract the uppermost line; and works for solo instrument with accompaniment – from which the solo was used and any large gaps were filled by a skyline of the upper accompanying line. While the skyline algorithm cannot be guaranteed to always find the optimal melody line – the melody is not universally in the uppermost voice – it does provide a robust technique for extracting the vast majority of melodic material. The chance of these errors occurring is, in part, mitigated by the use of compositions for monophonic instruments, with or without accompaniment, so giving an indication of where the primary melodic material is to be found.
It should be noted that, due to the nature of the constraints on the curation of the corpus, the database contains some inherent biases. A bias exists towards particular instrumentations, and so influences towards certain genres. As a result of the selection process, all works included were originally composed for either one or two instruments, no pieces for a larger ensemble are present; the corpus, therefore, is confined to chamber music genres.
2.1. Corpus description
The corpus contains 623 works – or self-contained work movements – with composition dates spanning from 1703 to 1934, encompassing Western styles from Baroque to Early Twentieth-Century.Footnote9 The works are distributed with a mean of 124.6 pieces for each of the five half-century divisions. The distribution of corpus items by composition year, length and instrumentation is shown in Figure , alongside the types of instrumentation requiring different techniques for extracting a monophonic line (further descriptive statistics for the corpus are given in Table ).
3. The model
The purpose of the computational model is to implement an integrated collection of hypothesised cognitive processes that produce a set of quantitative measures of large-scale thematic coherence, such that the corpus can be described in a multidimensional space. Within this space, each item can be defined as a point, representing the extent to which it possesses various features of hypothesised importance to the perception of thematic structure. To achieve this, the model also needs to be able to extract potential themes and repeated thematic material from the works of the corpus. Each piece has multiple IDyOM models applied, based on different training material, viewpoints and LTM/STM configurations. These statistical models are combined and used to simulate listeners' perception of repetition and variation as it varies dynamically throughout listening to a composition.
An overview of the modelling process is as follows (and given schematically in Figure ). The symbolic music data of the corpus is first used to create an LTM for each piece, for which the entire corpus, with the exclusion of the target piece, is used in the training of the model; this corpus-trained model is used to calculate information content for each note-event in the composition. The process is repeated for each piece in the corpus. Training on the entirety of the corpus makes these LTMs models of stylistic structure, generating predictions based on learnt stylistic conventions.
Figure 2. Outline of the statistical model of large-scale thematic structure using the IDyOM framework.
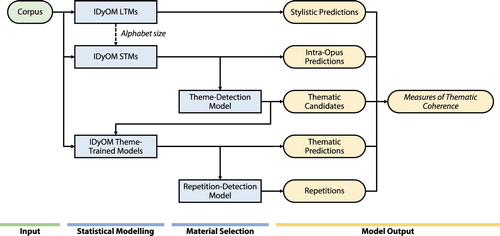
The training of the LTMs over the whole corpus also provides the full alphabet covered in that viewpoint representation – for example, the complete list of absolute pitch values or the entire collection of note durations used in the corpus. Since PPM* produces non-zero probabilities over the entire alphabet defined for the model, IC is sensitive to alphabet size; by maintaining the use of these full alphabets in the subsequent creation of models that are trained on subsets of the corpus, these ICs are directly comparable between all works for a given viewpoint.
Short-term models for each piece can then be implemented. Once again, an IC value is generated for each event, based on the online accumulation of context data within that composition. These ICs provide a measure of the unexpectedness of each note, given the preceding context based on a model that learns incrementally within each composition.
In addition to the extra-opus LTM and intra-opus STM, a third IDyOM model type is used in this analysis. Using a theme-detection model based on the STM (see Figure ); described fully in Section 3.3, thematic candidates are identified in the compositions. For each composition, these thematic candidates are then used as the training material for new models, one for each candidate (‘IDyOM theme-trained models’, Figure ; described in Section 3.4). These models produce ICs for each note-event giving the predictability of that event relative to the chosen thematic parent.
3.1. Viewpoints
As previously mentioned, one of IDyOM's advantages is the ability to represent music using different viewpoint representations. As the present model seeks to tease apart the musical features that have the most pronounced effect on perception of thematic structure, six initial viewpoint combinations are selectedFootnote10 that employ different representations of musical pitch for the compositions in the monophonic corpus.
This selection covers different levels of abstraction, ranging from the exact MIDI chromatic pitch number (pitch) – unable to account for transpositional invariance – the interval between these pitches – that can – and the rather more abstract representation of contour – whether the pitch ascends, descends, or remains the same. To these are added the chromatic scale-degree of the note, providing a representation of pitch relative to a tonal centre. The final two viewpoints used here are the linked representations of pitch⊗interval and interval⊗scale-degree that assume alphabets corresponding to the Cartesian product of the alphabets of their two respective components.
Models generated for this set of viewpoints can be used in competition with each other, comparing the ability of each to predict thematic repetition using a series of metrics generated within the model.
Of course, representations of rhythm undoubtedly play a part in our perception of thematic structure in music. However, rhythmic representations of music behave substantially differently to those of pitch. Specifically, it is perfectly viable in rhythm to have only a single note duration (or perhaps a single rhythmic pattern) for the vast majority of a composition, with the useful structure lying wholly in the pitch domain.Footnote11 The effects of these isochronous or isorhythmic pieces have the ability to mask other effects of repetition and structure when using these rhythmic viewpoints. More generally, for the styles represented in the corpus, it seems likely that pitch structure will tend to be a stronger influence on thematic structure than rhythmic structure. As a result of these issues, rhythmic viewpoints are omitted in the main analysis of this model and instead provided in the Appendix (see supplementary material).
3.2. Large-scale structure in the STM
Based on the principles of statistical learning, repetitions of material become more predicable as they occur successively. In the IDyOM STM, improved prediction of these later repetitions will be measurable by a reduced IC. The online processing of the STM means that all repeated material is subject to this effect. If material is repeated a greater number of times, its IC will continue to drop. Figure displays the IC for each note using the interval viewpoint in the first movement of Mozart's Piano Sonata No. 12, K. 332, serving as an example. Visually, the prediction set provided by the STM for this composition contains several prominent areas of densely populated low-IC (very predictable) events with a complete absence of more unpredictable material. These correspond to the exact repetitions of sections in the movement – the first, between 280 and 558 quarter-notes from the start of the piece, being the repeat of the sonata form exposition section – compressing the same patterns as the first exposition into a lower range of IC. Across the piece, low-IC repeated material is interspersed with higher, more unexpected, pitches; however, repeated thematic material can still be distinguished. The visibly different section at 559 quarter-notes corresponds to the start of the development section. Here patterns of thematic statements – not necessarily in their exact form – intersperse regions of more distantly-related material.
Figure 3. Information content generated by an interval short-term model predicting each note in Mozart's Piano Sonata No. 12, K. 332, first movement. The histogram on the right-hand side shows the overall distribution of IC.
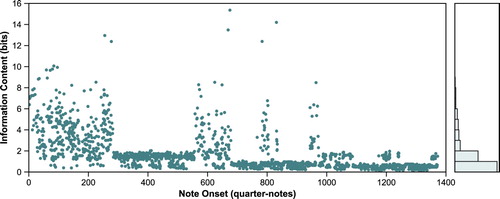
Repetition in the STM is undoubtedly obscured by a large amount of noise; even over the duration of a single composition, the model is beginning to learn other statistical regularities present – forming a basis for tonality and style that are independent of thematic repetition. Were our perception of thematically salient material to function solely in this manner, it would take many repetitions of a theme for reliable identification – while possible, this is not a wholly convincing representation of our cognitive processes. More likely, this statistical salience is reinforced by other mechanisms and prior knowledge of stylistic convention – phrasing, positioning, local melodic structure, and others. We assume, as a first approximation, that these conventions manifest themselves together under the concept of theme, in a way more imminent and meaningful than mere thematic repetition; our perceived salience through repetition may be bolstered through the identification of one or more themes, patterns that play a particularly important role in perception of thematic structure. In the rather noisy STM, the identification of a thematic candidate allows repetitions of derived material to be prominently predictable.
3.3. Theme-detection model
Computational identification of themes in music is a task of some difficulty. Methods for repetition detection in general for symbolic music have been produced for an array of purposes within the field of Music Information Retrieval, and with varying degrees of success (a summary of this body of research is given in Janssen et al., Citation2014, as well as the more recent work of Ren et al. (Citation2017); Melkonian et al., Citation2019; Laaksonen & Lemström, Citation2019). These methods seek to identify repeated material by searching for matching subsequences – with either exact or inexact matches – within the string of a given musical representation, or using ‘geometric’ approaches where melodies are considered as shapes in a multi-dimensional feature space (classification and summary given in Janssen et al., Citation2014). Existing theme detection methods are themselves derived as specific cases of string-matching repetition detection; different selection criteria are used to identify themes from repetitions, often using the longest matching substring or the most frequent substantial repetition. Explicit methods for theme detection using the exact matching of substrings are given by Hsu et al. (Citation2001), Meek and Birmingham (Citation2001) (with some deviations in rhythm allowed), Wang et al. (Citation2006), and Karydis et al. (Citation2007). Approximate matching methods also exist that find themes based on repeated similar material, such as Uitdenbogerd and Zobel (Citation1999) that uses edit distance based similarity measures.
All of these methods, however, operate in a manner contrary to that needed for our model of large-scale thematic structure – they first identify repetitions throughout a composition, then select the most likely theme. For a cognitive model simulating perception of thematic material on first listening, detection of themes must precede detection of thematic repetitions and both must be achieved dynamically on a single passing of the composition. As it is beyond their original scope, MIR theme detection methods have additional limitations for use in a cognitive model – many only consider finding a single primary theme for each composition in which many may exist, and there is also a significant difficulty in determining a theme's length.
The IDyOM STM can be employed to find potential themes – or thematic candidates – in this incremental online manner, avoiding the need for exact repetition matching. While the level of definition in the STM is not, in practice, great enough to allow for direct identification of thematically-derived material (as exemplified above), it can clearly identify the locations of multiple new thematic candidates by the presence of sudden increases in information content. If a composition were to contain a single thematic candidate – presented at the beginning – from which all subsequent material derives in some way, the STM would show a decrease in overall IC as the piece progressed. The introduction of a subsequent new thematic candidate in the composition would present unrelated, and so unpredictable, material – causing an increase in IC (see Figure below).
Figure 4. Theme detection for Mozart K. 332, movt. 1, exposition. Four onsets are identified at 0, 60, 121 and 253 quarter-notes, based on the interval IDyOM STM-ICs. Thematic candidates are identified by phrase mean ICs being greater than a threshold of a half standard deviation above the cumulative mean (sub-plot 2), and having a difference in phrase minimums greater than one standard deviation (sub-plot 3).
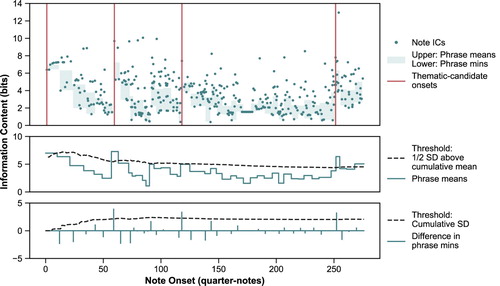
In order to give some estimation as to the length of a thematic candidate, it is useful to take into account the sequential grouping of material in music perception. Here, grouping boundaries between phrases are identified using CitationTemperley's (Citation2001) Grouper.Footnote12 Grouper segments a melody according to three Phrase Structure Preference Rules (PSPRs) (Temperley, Citation2001, pp. 68–71): the gap rule, PSPR 1, tries to locate boundaries at large IOIs or large offset–onset intervals; the phrase length rule, PSPR 2, favours phrases that are close to a predetermined length – here, ten notes;Footnote13 and the metrical parallelism rule, PSPR 3, favours boundaries occurring on the same position within a bar. Although it has been demonstrated that IDyOM can be extended to provide a probabilistic method of boundary segmentation (Pearce, Müllensiefen et al., Citation2010), Grouper continues to be one of the best performing and most robust methods available (Cenkerová et al., Citation2018). Its relative simplicity allows for it to be adapted and implemented incrementally note-by-note – rather than offline, as originally intended – with little adverse effect.
The theme-detection model defines thematic candidates based on sequential comparisons of Grouper-segmented phrases to the cumulative body of all preceding material. The first event in the piece is considered an implicit beginning of a thematic candidate. Otherwise, a candidate is declared at the start of a phrase if: (1) the mean IC of the phrase is greater than the cumulative mean of the preceding material by at least one-half the standard deviation; and (2) there is a complete absence of low-IC material in the STM, indicated by the phrase minimum IC (i.e. the note with the lowest IC in the phrase) rising by more than one cumulative standard deviation over the minimum of the preceding phrase. The values of these thresholds are chosen with the intention of providing a robust identification of significantly novel material within all compositions. Figure illustrates this process in the detection of thematic candidates in the Mozart K. 332 movement's exposition section, where, in this instance, all the detected candidates lie. Four thematic candidates are identified with onsets at 0, 60, 121 and 253 quarter-notes.
The precise length of thematic candidates, once a start point is detected, is still unknown. Using the phrase boundary segmentation, this length can be considered something of a free parameter, defined in terms of a given number of phrases. A length of two phrases, for example, functions well to account for the antecedent/consequent phrase pattern in much music of the Classical period and is used in the present analysis. To limit the number of models, theme detection is run using the interval (transposition insensitive) viewpoint. Thematic candidates are returned as symbolically notated fragments, used in the training of a new set of statistical models for the composition.
It should be stressed that the thematic candidates extracted by the model do not necessarily possess all the properties traditionally associated with the concept of theme in music analysis. True themes possess additional perceptual salience. In many cases this salience may occur through the repetition of the theme's material, but also through other influences – such as form, where there is often a strong tonal element not covered at all here. This model is not, therefore, intended as a tool by which a new analysis can be performed. Thematic candidates here should be considered simply as regions within the piece at which novel material is introduced. The extent to which thematic candidates actually contribute to the model's output – and are likely to be considered as actual themes – is determined through the identification of repetition of their material.
The four, two-phrase thematic candidates extracted from the example K. 332 movement are shown in Figure . As with many of the opening movements of Mozart piano sonatas, copious analyses for this work exist belonging to numerous different schools (Allanbrook, Citation1992; Beach, Citation1994; Beghin, Citation2014; Caplin, Citation2001; Galand, Citation2014; Hatten, Citation2014; Hepokoski & Darcy, Citation2006; Irving, Citation2010; Kinderman, Citation2006; Rumph, Citation2014; Schenker, Citation1994).Footnote14 It is perhaps unusual in that it contains more unique thematic material than may otherwise be expected in a sonata form exposition, and, although a direct alignment with any of these is not intended, the comparison below of the extracted thematic candidates to those identified by music theorists provides a concrete illustration of how the theme-detection model performs.
A brief summary of a generic traditional analysis of this sonata form exposition section could be as follows: there is an opening theme that (slightly unusually) is three phrases long (bars 1–12); this is followed by a new thematic idea (bars 13–22) in the second half of this first thematic group (Beach, Citation1994); a dramatically different transition passage in bars 23–40 occurs in the relative minor (Kinderman, Citation2006, p. 52); then the second thematic group is presented in the dominant (bars 41–48) – variations of this material alternate with darker syncopated passages (bars 56–66) until the closing codetta of the exposition in bars 86–93 (Kinderman, Citation2006, p. 52). In the model's detection of thematic candidates, we can see, in Figure , that many of these inner thematic ideas within the wider groups are not found to be distinct enough for separate classification (at least in part due to many of their distinguishing features being lost in the removal of texture, harmony and rhythm). Instead, what we have (in Figure ) is (1) the opening theme; (2) the start of the transition section, prepended by a small amount of material leading into it;Footnote15 (3) the second subject; and (4) material leading into, and the beginning of, the codetta. After the initial theme, the thematic candidate of the transition section is found to be highly prominent, even more so than the second subject which only just qualifies above the threshold for mean IC. The codetta material is perhaps a slightly spurious classification – the material is novel for the STM but it might be considered to contain purely stylistic content that is not directly relevant to thematic organisation.
When compared to the traditional analyses, these thematic candidates do not completely cover all the themes of the original. In particular, the second theme in the first thematic group (bars 13–22) is not identified; the pitch content of these phrases is not sufficiently novel for a candidate to be detected. However, using the counterpart rhythmic theme detection in the Appendix (see supplementary material), a candidate is located at this position in the music.
3.4. Repetition-detection model
By pre-training an IDyOM model on the data for a single thematic candidate (the ‘IDyOM theme-trained models’ in Figure ), a model can be created to provide a predictive probability for each note, based on the thematic candidate (‘thematic predictions’, Figure ); the noise in the STM is effectively removed as the models are not incrementally updated with every new note in the piece. Where multiple thematic candidates are identified, an IDyOM theme-trained model is constructed for each one, beginning at its onset, creating separate models for each candidate. Figure shows the note information contents generated for the first movement of Mozart K. 332 when a model is trained on each of the four thematic candidates extracted (shown in Figure ). The resulting note ICs need to be classified as to whether or not they constitute thematically-derived repetitions (or ‘motivic’ material). Once again, this process needs to function incrementally within the work's progression.
Figure 6. Prediction sets and their distributions for the four theme-trained models of Mozart K. 332 movt. 1. A thematic threshold is generated by an incrementally updated GMM at the phrase level – material below this boundary is classified as thematic.
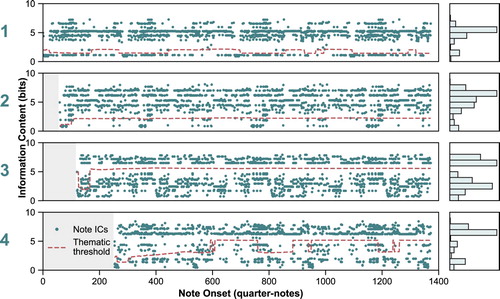
Models generated in this way have the property of producing clearly stratified ICs, reflected in a degree of bi-modality in their distribution (as can be seen in the distribution histograms of Figure ). Each note's IC values can be considered as either belonging to a lower-IC thematic distribution, or to the high-IC distribution of the remainder. This bi-modality holds for compositions where the two distributions are far less distinct.
To perform this classification, clustering with Gaussian Mixture Models (GMM) is applied to the distributions (Reynolds, Citation2009). Two Gaussians are fitted to the IC data using expectation–maximisation (Dempster et al., Citation1977). The starting parameters of the GMM are calculated so that the intended distributions are identified – for example, so that the two Gaussians avoid favouring some other multimodal components in the remainder distribution, or at other local minima – and to ensure consistent results. To provide the best chance of finding the correct thematic distribution and the remainder, starting means of the lowest value and the median (respectively) are used. Gaussians are initially equally weighted and the standard deviation of the remainder is specified as double that of the thematic. This clustering for the model trained on the first thematic candidate of K. 332 is illustrated in Figure . After the initial occurrence of a thematic candidate, for which the question of thematic association is not needed, this GMM can categorise each note as thematic or remainder (i.e. non-thematic) based on the cumulative previous distribution, updated at the phrase level – shown in Figure for all four thematic candidates identified in the first movement of Mozart K. 332.
Figure 7. Note IC distribution and Gaussian Mixture Model Clustering for a model trained on thematic-candidate 1 and applied to Mozart K. 332 movt. 1. A lower cluster is identified as the thematic material and the upper the remainder. The vertical dashed line indicates the threshold identified.
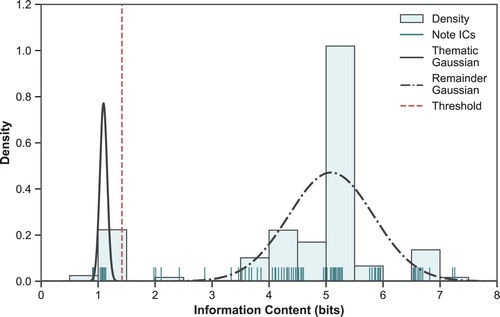
The categorisation of thematic material on an individual note basis, as described above, provides rather a harsh and exacting process; the local context of each note is not taken into account and the resulting material extracted is highly fragmented. Performing the same operation again with smoothing on a phrase-based level allows larger – still low-IC and salient – sections to be extracted, following perceived sequential groupings of material, providing a larger-scale output more akin to motifs. For this purpose, Temperley's Grouper is once more applied. The threshold IC is still computed on the note-event level; the mean IC for each phrase is then categorised based on this threshold.
3.4.1. Compression distance
The repetitions of thematic candidates identified by the repetition-detection model can be used to simulate the degree of intra-opus variation of thematic material. A computational measure of similarity between each phrase categorised as being thematic and its parent thematic candidate can describe how much variation thematic material undergoes. As with all the steps of this model so far described, the similarity metric should be well-motivated in terms of representing actual cognitive processes. We use an information-theoretic measure of compression distance which has shown promise in simulating perceived melodic similarity (Pearce & Müllensiefen, Citation2017). A similarity metric based on normalised compression distance was introduced by Li et al. (Citation2003). The dissimilarity between two sequences, x and y is a function of the predictability (or compressed length) of one sequence given a model trained on the other. Pearce and Müllensiefen (Citation2017) employ IDyOM to estimate dissimilarity between two musical sequences as the average IC of the notes making up one sequence, given a model trained on the other sequence. In the same way, the dissimilarity between an identified thematic fragment and its parent thematic candidate can be modelled as the sum total of the ICs for each note in the thematic fragment given the IDyOM model trained on the parent thematic candidate. The sum of the ICs is then normalised with respect to the longest sequence – the candidate. This asymmetric measure of compression distance is appropriate given that we are only concerned with the amount of variation moving forward through the composition.
3.5. Measures of thematic coherence
The model of large-scale thematic structure described above extracts thematic candidates and thematic repetitions for the compositions making up the corpus. Here we are particularly interested in using this model to produce quantitative measures of large-scale thematic coherence. By applying the model to the corpus, we can describe quantitatively how real musical compositions differ in a number of key dimensions relating to large-scale thematic structure. These dimensions – or measures – belong to three categories: (1) two concerning general features of the compositions – year of composition and length; (2) four describing features of a composition's thematic candidates (as detected by the model); and (3) four describing the properties of repeated thematic material (again, as detected by the model). The measures are further described in Table . Of these measures, we are primarily interested in those directly arising from repetition – particularly amount of repetition, degree of variation and stylistic predictability – as the main parameters influencing structural coherence.
Table 1. Model-derived measures of large-scale thematic structure, subdivided into general features, measures relating to theme, and measures relating to subsequent repeated thematic material.
Table 2. Results for measures of large-scale thematic structure and their correlations. Pearson's r, n = 623.
4. The analysis
Our model is founded in the idea that repetition is the principal enabler for large-scale structural coherence in music. More specifically, according to the probabilistic conception of music espoused here, through learning, this repetition increases the intra-opus predictability of a composition, giving rise to perceived coherence. Repetition of thematic material strengthens its salience – the more material is repeated, the greater its perceived prominence. By following stylistic or work-specific structural regularities, such repetitions can undergo embellishment and variation and still reinforce future predictions. The purpose of the analysis below is to examine the ways in which the measures defined contribute to systematic variation of thematic structure within the corpus.
4.1. Viewpoint performance
For the majority of the measures, there is also the added dimension of viewpoint representation. The IDyOM long-term, short-term and theme-trained models were all produced for each of the six chosen representations of musical pitch (see Section 3.1). While it is acknowledged that – as listeners – we likely use a combination of multiple different representations of music at any one time, the precise way in which these representations are weighted and combined is unknown. Given this, and the fact that the different pitch representations show a degree of overlap in their representation of musical structure, and for conciseness in exploring the multidimensional set of measures, a single representation is selected for further analysis.
There is no inherent method by which to select the best-performing viewpoints for the model. However, a comparison with respect to features produced by the model sheds some light on the representations of music that might facilitate perception of thematic coherence. Therefore, in the absence of more explicit criteria, a comparison of the amount of repetition detected with each pitch viewpoint reveals that the interval viewpoint captures the highest proportion of thematic material, averaged across the corpus (see Figure ).
4.2. Results
For the selected pitch viewpoint of interval, summary statistics of the measures of thematic coherence and their respective pairwise correlations are given in Table . Measures derived directly from information content values – namely stylistic predictability of theme and stylistic predictability of thematic material – have a scale that is lower for greater predictability/expectedness. For strength of theme prominence, higher values indicate more prominent thematic candidates in the short-term model. The compression distance measure of degree of variation gives a lower score when material is more closely related.
Due to the nature of how they are calculated, not all measures are completely independent of each other. For those correlations with a coefficient greater than 0.5 (shown in Table in bold): length and number of themes are highly correlated – if a composition is longer, thematic-candidate extraction is more likely to identify a greater number of candidates; stylistic predictability of theme and stylistic predictability of thematic material are both derived from the same LTM with the former limited just to the parent thematic candidate(s) and the latter calculated for thematic material identified by the repetition model as being related to the parent candidates; and amount of thematic repetition and degree of variation – in works where there is a greater amount of repetition, there is an increased opportunity for repetitions to be embellished further from their original.Footnote16
The choice and definition of these measures largely arise from the probabilistic model constructed above. The relative importance of these measures in actually explaining the variance of structure observed in the corpus needs to be established. This variation can be explored in greater depth by performing Principal Component Analysis and Independent Component Analysis, as dimensionality reduction and importance exploration techniques, on the set of measures applied to the corpus.
4.2.1. Principal component analysis
Principal Component Analysis (PCA) allows us to geometrically reduce the original measures into a smaller set of orthogonal components (Abdi & Williams, Citation2010). These new components consist of linear combinations of the original measures, attempting to account for the maximum amount of variance in the structure of the data by the smallest number of components. While the output components do not correspond directly to the input measures, performing a PCA with equal numbers of each will produce components that account for all variance, and – importantly for its use here – the proportion of explained variance for which each component is responsible. The ordering of the principal components is such that the first accounts for the largest proportion of variation in the set, the next attempts to explain additional variance while remaining orthogonal to the first.
The purpose of using PCA in the present analysis is to assess the importance of each of the ten measures of thematic structure in terms of accounting for variance in the corpus. Figure shows the output of the PCA for the ten input measures using a model configured with the interval viewpoint. The first six components alone account for 88% of the total variance. The inner weightings of measures for each component are also given. Direction of weighting is determined by the nature of the model measure; for those based on information contents from the long-term, short-term and theme-trained model, lower values correspond with greater predictability, and for those based on compression distance, lower values imply less variation of material.
Figure 9. Principal Component Analysis – overall explained variances for each measure and total explained variance for each output component.
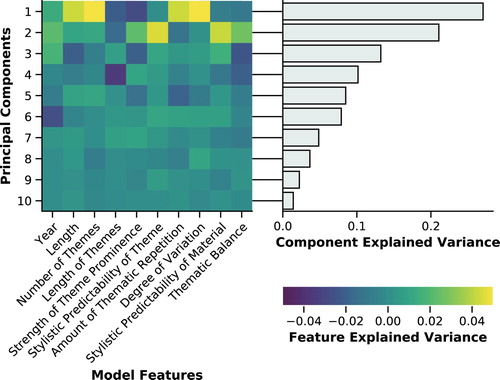
The first component is dominated by four measures with positive weights – length, number of themes, amount of thematic repetition and degree of variation. Based on this first component, and the significant correlations discussed for Table , the biggest differences in thematic structure between pieces in the corpus can be attributed to variation in the number of thematic candidates (which is constrained by the length of the piece), amount of thematically repeated material (normalised against the effects of length) and how much that material is varied within the piece.
The second principal component is dominated by measures in which stylistic congruence has the greatest influence – stylistic predictability of theme, stylistic predictability of thematic material and thematic balance (lower, favouring the LTM). The third principal component is more weakly associated with measures of the intra-opus models. Component four is dominated by length of themes, five contains less prominent combinations of how stylistically novel a thematic candidate is and how much its material is repeated, and six is accounted for by variation in year.
The results of this PCA indicate a strong influence of three of the measures that we are particularly interested in – amount of thematic repetition, degree of variation and stylistic predictability of thematic material – with the first two appearing in the first component and the latter in the second. Overall, the PCA provides insight into the relative importance of the model-generated measures in explaining inherent variation in thematic structure within the corpus. However, we still know little about the relations between them. An Independent Component Analysis is employed to examine their independence by isolating ‘noise’ within each measure from the others.
4.2.2. Independent component analysis
Independent Component Analysis (ICA) can be considered an extension of the previous Principal Component Analysis – instead of optimising components according to first- and second-order statistics in the covariance matrix, for an ICA higher-order statistics (such as kurtosis) are optimised (Tharwat, Citation2018). PCA finds orthogonal uncorrelated components that account for the most variance in the data, ICA finds statistically independent components that are not necessarily orthogonal. When the number of components matches the number of input features, ICA will effectively try to extract ‘original’ sources from the multivariate features – attempting to minimise the mutual information between components. ICA first performs ‘whitening’ of the data, so that it is centred and uncorrelated. Whitened data is then rotated so as to minimise Gaussianity in all dimensions, resulting in statistically independent components.Footnote17
When applied to the corpus-generated measures, an ICA has the effect of isolating a component for each measure, in which any of the ‘noise’ related to other measures is effectively removed. In this analysis, the ordering of components is without meaning and there is no ordering the relative weight of each component in accounting for the data. The ICA mixings for each component are used to indicate how much each input measure influences each independent component, as shown in Figure . Mixing direction shows the direction in which the measure influences each component.
Five of the statistically independent components produced have only one main contributor, indicating that they are ‘noise-free’ with limited interaction with any of the other measures. These are: (1) length, (2) length of themes, (3) stylistic predictability of theme, (5) strength of theme prominence, and (6) year (although this latter is not quite as independent as the others). Number of themes maintains its connection with composition length but with some degree of independence in component four. Component seven strongly links together amount of thematic repetition and degree of variation. Components eight and nine are both influenced by stylistic predictability of thematic material, with thematic balance only having a relatively small influence in any component.
Aside from knowing that several of our measures are independent of all others, the case of the connection between amount of thematic repetition and degree of variation suggests that if there is more repetition within a work, it tends to undergo embellishment and variation (at least in the case of pitch and for the works in this corpus).
4.2.3. Example pieces
The performance of the model in capturing appropriate structural variation can be illustrated by example compositions taken from the extremes of the measures amount of thematic repetition, degree of variation and stylistic predictability of thematic material. Based on the underlying hypotheses of the model, these three measures are of particular importance in capturing the effects of large-scale thematic structure; they are also found to be the repetition-based measures that account for most variance in the PCA. The five works at the extremes of each of these measures are listed in Table .
Table 3. Example works from the extremes (top and bottom five) of amount of thematic repetition, degree of variation and stylistic predictability of thematic material using the interval representation.
The greater extreme end of the amount of thematic repetition measure can be characterised well by the bourrée of Bach Cello Suite No. 4 (one of the shortest works in the dataset), that contains two detected thematic candidates and several exact repetitions of them, and the far more substantial Schubert Impromptu, in which seven thematic candidates are detected, each of which is repeated frequently and exactly. Such pieces have clear statements of theme – produced by fairly rigid structural blocks, between which there is little sharing of material – and highly frequent repetition of material with little variation. Works at the lower end of the scale have little repeated material detected. For some compositions, this lack of repeated thematic material may be a feature of their style; for example, the ‘impressionistic’ compositional style used by Debussy in the Arabesque frequently introduces thematic material, not necessarily closely related to that preceding it, and revisits little (Potter, Citation2003, p. 144; Grout et al., Citation2010, p. 792). In other cases, this may be due to the loss of information that occurs from the necessary monophonic manipulation of compositions – for example, affecting the Beethoven Piano Sonata.
Degree of variation captures how far repeated material within a piece strays from the original thematic candidates. A continuation of the effects of simple theme-statement and exact repetition pieces is also seen here – little actual variation of material occurs. At the end of greater variation, these are, for the most part, pieces that contain substantial development sections, giving greater opportunity to embellish upon previously stated themes, and those of a more fantasy-like composition style.
The works that have the lowest values for stylistic predictability of thematic material, and so are the most stylistically predictable or congruent, are those whose repeated material mostly follows the conventions of style, as taken from the corpus.Footnote18 This is illustrated very clearly in the Chopin Etude – a composition that almost entirely consists of chromatic scale passages. At the opposite end of this measure, works are all still tonal (as is all the corpus) – possibly the biggest factor of style in the model – and it does not necessarily follow that the entire compositions themselves are stylistically unpredictable, it is simply that the thematic material and its derived repetitions are stylistically novel in the context of the corpus.
5. Limitations and future directions
As a computational account of the real-world perception of large-scale thematic musical structure, this model does posses some limitations. For the most part, these limitations are brought about through the necessity to reduce the processes involved to a form that is tractable with the methods in existence, and to avoid unjustified assumptions about cognitive processes for which empirical evidence is lacking. The model is, of course, a simplification of human perception and cognition, and of its parallels in music theory and analysis. The limitations faced by this model can be broadly attributed to three areas: (1) constraints on the types of musical information the model deals with – particularly the monophonic constraint discussed in Section 2; (2) limitations on the ways in which music can be represented, and how multiple such representations can be validly combined; and (3) limitations resulting from the selection of parameters within the model and its constituent components. While some of these limitations contribute to the occasional spurious identification of – for example – thematic candidates, these appear to be exceptions rather than the rule. Furthermore, these limitations all have potential remedies that are possible with future research; with empirical testing, the validity of additional computational methods can be ascertained, addressing the gaps presented here. This section discusses the limitations of the model, their impact on the model in its current form, and the ways in which they can be addressed in the future.
The monophonic constraint imposed on the corpus in this paper (see Section 2 reduces all the model's input to melodic content only. This constraint leads to a loss of information – particularly when compared with the original works from which the melodies were extracted. For example, in the earlier example of the Mozart Piano Sonata in Sections 3.3 and 3.4, some occurrences of themes in traditional analyses rely on harmonic or cadential cues that are not present in the melody. However, we caution that, while illustrative, the melody should not be considered as being directly the same work as the original composition from which it was extracted. Instead, we maintain that these melodies can function as compositions in their own right, with different auditory information available. This constraint is currently needed to avoid the complications of processing thematic material in many polyphonic layers. For example, cognitive models of phrase boundary detection (such as Grouper) are currently limited to melody. To tackle this constraint, firstly, accurate modelling of auditory streams is needed to extract all perceptually salient voices from the polyphonic textureFootnote19 and, secondly, cognitively valid methods need to be developed to apply probabilistic modelling, theme detection and repetition detection to multiple voices. These are currently significant unresolved research challenges in their own right, and since there are multiple possibilities for such approaches, it is imperative that candidate methods are psychologically evaluated.
For this model, as with many symbolic models of music, limitations also stem from how the music can be meaningfully represented; in particular, how to represent and combine the separate domains of pitch and rhythm. For the main analysis, in which only pitch (interval) is used, it may be assumed that there is some loss of information from the rhythmic domain. In the example of Section 3.3, certain themes identified in traditional analyses that do not feature in the list of thematic candidates may not have been identified due to their novelty only substantially existing in the rhythmic domain. The Appendix (see supplementary material) provides a companion analysis using rhythmic representations, in which additional thematic candidates are identified in the exposition of this example piece. However, as musical compositions can be, and often are, rhythmically isochronous – which limits the utility of the rhythmic modelling – the combination of the two domains with equal weighting would still propagate this issue. The route for combination likely exists in some selection by listeners of the appropriate representations for each piece of music, including rhythmic representations and excluding (or down-weighting) them for others. The exact nature of this mechanism requires further development and experimental exploration.
Finally, in order for the model to make classifications on phrase boundaries, thematic candidates and thematic repetitions, the model requires certain conditions to be met. These conditions exist as free parameters within the model – for example, the number of phrases that are extracted to make up a theme. While values for these parameters are informed by theory or statistics, the true optimum will vary between compositions, styles and listeners. As a result, these mechanisms can miss or misclassify their elements on certain occasions. For example, the phrase boundaries identified by the Grouper algorithm (Temperley, Citation2001) may differ from those perceived by a listener, or a theme may not be identified due to it narrowly missing the novelty criteria (as can be seen in Figures A1 and A2 of the Appendix). The only remedy for this is through repeated evaluation of both the model as a whole, and its components, against the behaviour of listeners. In particular, research is needed into listeners' perceptions of theme – an area where there is little experimental research.
6. Conclusion
We have presented a statistical model of large-scale thematic structure in music, based on the underlying theory that the perception of such structures is facilitated by the repetition, and variation, of material within a composition. While structure is considered important in music theory and in theoretical models of music cognition, past psychological work in search of the effects of large-scale coherence has often proved inconclusive. The statistical model is presented as the beginnings of a concrete specification of the cognitive processes involved in the perception of large-scale thematic coherence. The model expresses explicitly a plausible computational account of how large-scale thematic structure might be perceived and allows us to derive a set of formal, quantitative measures of thematic structure.
The model uses the IDyOM framework to create extra-opus models of style – in which the whole corpus is used to calculate the unexpectedness of the notes in each piece, given the context – intra-opus models of piece interrelatedness – where the training context is provided online as a piece progresses – and theme models trained on the extracted thematic candidates of a composition. Not only does this model provide novel techniques for extracting thematic candidates and thematic repetitions (or motifs) from a composition, it also produces a multidimensional set of measures, with which the variation of structurally important elements present in music can be captured.
To investigate the behaviour of the model, a corpus of 623 monophonic Western-Classical works was gathered, within which there is variation of large-scale structure. The analysis of the model was intended to explore the extent to which the quantitative measures of large-scale coherence can account for variation within the corpus. To do so, principal component and independent component analyses were used to understand the nature of the multiple dimensions produced. This resulted in the identification of three measures that show both independence from the others and importance in terms of accounting for large proportions of variance in the corpus: amount of thematic repetition, degree of variation and stylistic predictability of thematic material. Example compositions from the extremes of these three measures were assessed to further illustrate the properties of this variation.
The results of this model analysis are in many ways, at present, rather open-ended, given the lack of an appropriate ‘ground-truth’ against which the model's accuracy can be judged. Therefore, full testing of the model as an accurate simulation of cognitive processes underlying the perception of structural coherence requires further empirical research with human listeners. This empirical work first needs to find variation in the corresponding behavioural equivalents of the quantitative measures generated by the model. As noted above, we view the current model very much as a first step and envisage a process in which its various components are iteratively tested against empirical data and revised or extended accordingly (Desain et al., Citation1998). Ultimately, the utility of the model (and the underlying theory of thematic coherence) will be tested by its ability to advance knowledge where past studies have not – both to account for listeners' overall perception of coherence of a piece of music, and to provide detailed understanding about the large-scale structures that may, or may not, facilitate this perceived coherence.
The model is based on several underlying cognitive mechanisms that are hypothesised to allow the perception of large-scale thematic coherence, each corresponding to an individual modelling process (outline given in Figure ). With this model, we can avoid some of the difficulties faced by past researchers and instead form a set of specific psychological hypotheses: (1) that the material most repeated is the most salient; (2) that salience arising from repetitions affirms structural coherence – through the re-enforcement of statistical models of intra-opus structure; and (3) that repeated material does not have to be exact to achieve coherence, since variations according to extra-opus style and intra-opus development may be present. Testing these hypotheses may shed light on the psychological mechanisms involved when listeners perceive large-scale thematic structures in music.
Acknowledgments
This research utilised Queen Mary's Apocrita HPC facility, supported by QMUL Research-IT (doi.org/10.5281/zenodo.438045). We would like to thank Emmanouil Benetos and two anonymous reviewers for their helpful comments on earlier drafts of the manuscript.
Disclosure statement
No potential conflict of interest was reported by the author(s).
Additional information
Funding
Notes
1 The Generative Theory of Tonal Music's Time-Span Reduction Preference Rule of parallelism (TSRPR 4) – to assign parallel heads in the hierarchy to time spans if their material is similar – when applied on the scale of a complete composition, gives some account of large-scale structure created through repetition.
2 Form, from music theory, is a specific manifestation of large structure, often implying a relationship between the construction of a work and certain stylistic thematic and tonal conventions – such as in sonata form (Whittall, Citation2001).
3 Whether or not these movements can be expected to contain thematically linking material is debatable. It should be noted that Bach's Goldberg Variations – used here – differ significantly to the later ideas of classical or romantic ‘theme and variations’ form. The concept of a theme reoccurring and being constantly developed in each subsequent variation does not really exist here in the same way, allowing individual variations to be self-contained in themselves – as argued by Batt (Citation1987) in his criticism of the studies.
4 For example, material becoming intensely scalic when approaching a cadence in a work from the Classical era. Variation not due to this stylistic embellishment could be considered thematic development, taking place over the course of the piece.
5 The corpus, along with information on the individual works, can be found at osf.io/dg7ms/.
9 All piece description labels and genre/style classifications are taken from the International Music Score Library Project.
10 See github.com/mtpearce/idyom/wiki/List-of-viewpoints for a complete list.
11 For example, such as is common in many of the Bach Cello Suites.
12 Implementation, as used here, available through The Melisma Music Analyzer (Sleator & Temperley, Citation2003) accessible at www.link.cs.cmu.edu/music-analysis/.
13 This value was arrived at by Temperley through an optimisation of the algorithm to an annotated subset of the Essen Folk Song Collection (Temperley, Citation2001, p. 74).
14 It has become a particular favourite for analyses of topic theory.
15 This premature thematic-candidate identification can likely be attributed to a small difference in phrase boundary placement by Grouper, when compared those made in the analyses described above. Inclusion of the C♯ (bar 22, beat 3) in the preceding phrase, rather than the following, alters the phrase in which novel pitch content occurs.
16 There is no corresponding correlation between length and amount of thematic repetition, as there is with thematic candidates, because the latter is computed as a proportion of composition length.
17 Due to the Central Limit Theorem – the sum of independent random variables will fit the normal distribution more closely than the parent distributions.
18 The Bartók Bagatelle may seem to be something of an anomaly in this high stylistic predictability category as it is one of the compositions in the corpus that strays furthest from tonality. However, for the purpose of completeness it is included in this list. Its appearance can be accounted for by three reasons: (1) due to the skylining process, a fair proportion of stylistically unconventional material is lost – opposing pitch classes are often used simultaneously in the two hands; (2) the resulting main thematic candidate detected is relatively stylistically predictable; and (3) it has a low level of repeated material detected which undergoes little variation, meaning all thematic material detected is still predictable.
19 Multiple successful models of voice separation have been designed, however, further research is needed to investigate the extent to which such voices are perceptible to listeners. (Cambouropoulos, Citation2008; Sauvé, Citation2018)
References
- Abdi, H., & Williams, L. J. (2010). Principal component analysis. WIREs Computational Statistics, 2(4), 433–459. https://doi.org/10.1002/wics.101
- Agres, K., Abdallah, S., & Pearce, M. (2018). Information-theoretic properties of auditory sequences dynamically influence expectation and memory. Cognitive Science, 42(1), 43–76. https://doi.org/10.1111/cogs.12477
- Allanbrook, W. J. (1992). Two threads through the labyrinth: Topic and process in the first movement of K. 332 and K. 333. In W. J. Allanbrook, J. M. Levy, & W. P. Mahrt (Eds.), Convention in Eighteenth- and Nineteenth-Century Music Essays in Honor of Leonard G. Ratner (pp. 125–171). Pendragon Press.
- Batt, R. (1987). Comments on “The effects of instrumentation, playing style, and structure in the ‘Goldberg Variation’ by Johann Sebastian Bach”. Music Perception: An Interdisciplinary Journal, 5(2), 207–213. https://doi.org/10.2307/40285393
- Beach, D. (1994). The initial movements of Mozart's Piano Sonatas K. 280 and K. 332: Some striking similarities. Intégral, 8, 125–146.
- Beghin, T. (2014). Recognizing musical topics versus executing rhetorical figures. In D. Mirka (Ed.), The Oxford Handbook of Topic Theory (pp. 551–576). Oxford University Press.
- Cambouropoulos, E. (2008). Voice and stream: Perceptual and computational modeling of voice separation. Music Perception: An Interdisciplinary Journal, 26(1), 75–94. https://doi.org/10.1525/mp.2008.26.1.75
- Caplin, W. E. (2001). Classical form: A theory of formal functions for the instrumental music of Haydn, Mozart, and Beethoven. Oxford University Press.
- Cenkerová, Z., Hartmann, M., & Toiviainen, P. (2018). Crossing phrase boundaries in music. In A. Georgaki, & A. Andreopoulou (Eds.), SMC 2018: Proceedings of the 15th Sound and Music Computing Conference 2018 (pp. 66–71).
- Cheung, V. K. M., Harrison, P. M. C., Meyer, L., Pearce, M. T., Haynes, J. -D., & Koelsch, S. (2019). Uncertainty and surprise jointly predict musical pleasure and Amygdala, Hippocampus, and Auditory Cortex activity. Current Biology, 29(23), 4084–4092. https://doi.org/10.1016/j.cub.2019.09.067
- Cleary, J. G., & Teahan, W. J. (1997). Unbounded length contexts for PPM. The Computer Journal, 40(2/3), 67–75. https://doi.org/10.1093/comjnl/40.2_and_3.67
- Conklin, D., & Witten, I. H. (1995). Multiple viewpoint systems for music prediction. Journal of New Music Research, 24(1), 51–73. https://doi.org/10.1080/09298219508570672
- Cook, N. (1987). The perception of large-scale tonal closure. Music Perception: An Interdisciplinary Journal, 5(2), 197–205. https://doi.org/10.2307/40285392
- Deliège, I. (2007). Similarity relations in listening to music: How do they come into play? Musicae Scientiae, 11(1 suppl), 9–37. https://doi.org/10.1177/1029864907011001021
- Dempster, A. P., Laird, N. M., & Rubin, D. B. (1977). Maximum likelihood from incomplete data via the EM algorithm. Journal of the Royal Statistical Society. Series B (Methodological), 39(1), 1–38. https://doi.org/10.1111/rssb.1977.39.issue-1
- Desain, P., Honing, H., Vanthienen, H., & Windsor, L. (1998). Computational modeling of music cognition: Problem or solution? Music Perception, 16(1), 151–166. https://doi.org/10.2307/40285783
- Egermann, H., Pearce, M. T., Wiggins, G. A., & McAdams, S. (2013). Probabilistic models of expectation violation predict psychophysiological emotional responses to live concert music. Cognitive, Affective, & Behavioral Neuroscience, 13(3), 533–553. https://doi.org/10.3758/s13415-013-0161-y
- Eitan, Z., & Granot, R. Y. (2008). Growing oranges on Mozart's apple tree: “Inner form” and aesthetic judgment. Music Perception: An Interdisciplinary Journal, 25(5), 397–418. https://doi.org/10.1525/mp.2008.25.5.397
- Epstein, D. (1980). Beyond orpheus: Studies in musical structure. Journal of Aesthetics and Art Criticism, 38(4), 480–482. https://doi.org/10.2307/430342
- Galand, J. (2014). Topics and tonal processes. In D. Mirka (Ed.), The Oxford Handbook of Topic Theory (pp. 453–473). Oxford University Press.
- Galeazzi, F. (2012). The theoretical-practical elements of music, parts III and IV (D. & G. W. Harwood, Trans.). University of Illinois Press.(Original work published 1796).
- Gingras, B., Pearce, M. T., Goodchild, M., Dean, R. T., Wiggins, G., & McAdams, S. (2016). Linking melodic expectation to expressive performance timing and perceived musical tension. Journal of Experimental Psychology: Human Perception and Performance, 42(4), 594–609. https://doi.org/10.1037/xhp0000141
- Gjerdingen, R. (1999). An experimental music theory? In N. Cook, & M. Everist, (Eds.) Rethinking Music (pp. 161–170). Oxford University Press.
- Gold, B. P., Pearce, M. T., Mas-Herrero, E., Dagher, A., & Zatorre, R. J. (2019). Predictability and uncertainty in the pleasure of music: A reward for learning? Journal of Neuroscience, 39(47), 9397–9409. https://doi.org/10.1523/JNEUROSCI.0428-19.2019
- Gotlieb, H., & Konečni, V. J. (1985). The effects of instrumentation, playing style, and structure in the Goldberg Variations by Johann Sebastian Bach. Music Perception: An Interdisciplinary Journal, 3(1), 87–101. https://doi.org/10.2307/40285323
- Granot, R. Y., & Jacoby, N. (2011). Musically puzzling I: Sensitivity to overall structure in the sonata form? Musicae Scientiae, 15(3), 365–386. https://doi.org/10.1177/1029864911409508
- Granot, R. Y., & Jacoby, N. (2012). Musically puzzling II: Sensitivity to overall structure in a Haydn E-minor sonata. Musicae Scientiae, 16(1), 67–80. https://doi.org/10.1177/1029864911423146
- Grout, D. J., Burkholder, J. P., & Palisca, C. V. (2010). A history of Western music (8th ed.). W. W. Norton.
- Hansen, N. C., & Pearce, M. T. (2014). Predictive uncertainty in auditory sequence processing. Frontiers in Psychology, 5, https://doi.org/10.3389/fpsyg.2014.01052Article 1052.
- Hansen, N. C., Vuust, P., & Pearce, M. (2016). “If you have to ask, you'll never know”: Effects of specialised stylistic expertise on predictive processing of music. PloS One, 11(10), https://doi.org/10.1371/journal.pone.0163584Article e0163584.
- Hatten, R. (2014). The troping of topics in Mozart's instrumental works. In D. Mirka (Ed.), The Oxford Handbook of Topic Theory (pp. 514–536). Oxford University Press.
- Hepokoski, J., & Darcy, W. (2006). Elements of sonata theory: Norms, types, and deformations in the late-eighteenth-century sonata. Oxford University Press.
- Hsu, J.-L., Liu, C.-C., & Chen, A. (2001). Discovering nontrivial repeating patterns in music data. IEEE Transactions on Multimedia, 3(3), 311–325. https://doi.org/10.1109/6046.944475
- Irving, J. (2010). Understanding Mozart's piano sonatas. Routledge.
- Janssen, B., de Haas, W. B., Volk, A., & van Kranenburg, P. (2014). Finding repeated patterns in music: State of knowledge, challenges, perspectives. In M. Aramaki, O. Derrien, R. Kronland-Martinet, & S. L. Ystad (Eds.), Sound, Music, and Motion, Lecture Notes in Computer Science (pp. 277–297). Springer International Publishing.
- Karno, M., & Konečni, V. J. (1992). The effects of structural interventions in the first movement of Mozart's Symphony in G Minor K. 550 on aesthetic preference. Music Perception: An Interdisciplinary Journal, 10(1), 63–72. https://doi.org/10.2307/40285538
- Karydis, I., Nanopoulos, A., & Manolopoulos, Y. (2007). Finding maximum-length repeating patterns in music databases. Multimedia Tools and Applications, 32(1), 49–71. https://doi.org/10.1007/s11042-006-0068-5
- Kinderman, W. (2006). Mozart's piano music. Oxford University Press.
- Konečni, V. J. (1984). Elusive effects of artists' ‘messages’. In W. R. Crozier, & A. J. Chapman (Eds.), Cognitive Processes in the Perception of Art (pp. 71–93). Elsevier.
- Laaksonen, A., & Lemström, K. (2019). Transposition and time-warp invariant algorithm for detecting repeated patterns in polyphonic music. In 6th International Conference on Digital Libraries for Musicology (pp. 38–42), Association for Computing Machinery.
- Lamont, A., & Dibben, N. (2001). Motivic structure and the perception of similarity. Music Perception: An Interdisciplinary Journal, 18(3), 245–274. https://doi.org/10.1525/mp.2001.18.3.245
- Lerdahl, F., & Jackendoff, R. (1983). A generative theory of tonal music. MIT Press.
- Li, M., Chen, X., Li, X., Ma, B., & Vitányi, P. (2003). The similarity metric. In Proceedings of the Fourteenth Annual ACM-SIAM Symposium on Discrete Algorithms (pp. 863–872), Society for Industrial and Applied Mathematics.
- Margulis, E. H. (2012). Musical repetition detection across multiple exposures. Music Perception: An Interdisciplinary Journal, 29(4), 377–385. https://doi.org/10.1525/mp.2012.29.4.377
- Margulis, E. H. (2014). On repeat: How music plays the mind. Oxford University Press.
- Meek, C., & Birmingham, W. P. (2001). Thematic extractor. In J. S. Downie & D. Bainbridge (Eds.), Proceedings of the 2nd Annual International Symposium on Music Information Retrieval (pp. 119–128).
- Melkonian, O., Ren, I. Y., Swierstra, W., & Volk, A. (2019). What constitutes a musical pattern? In Proceedings of the 7th ACM SIGPLAN International Workshop on Functional Art, Music, Modeling, and Design (pp. 95–105), Association for Computing Machinery.
- Meyer, L. B. (1989). Style and music: Theory, history, and ideology. University of Chicago Press.
- Omigie, D., Pearce, M. T., & Stewart, L. (2012). Tracking of pitch probabilities in congenital amusia. Neuropsychologia, 50(7), 1483–1493. https://doi.org/10.1016/j.neuropsychologia.2012.02.034
- Omigie, D., Pearce, M. T., Williamson, V. J., & Stewart, L. (2013). Electrophysiological correlates of melodic processing in congenital amusia. Neuropsychologia, 51(9), 1749–1762. https://doi.org/10.1016/j.neuropsychologia.2013.05.010
- Pearce, M. T. (2005). The construction and evaluation of statistical models of melodic structure in music perception and composition [Doctoral dissertation, City University London]. City Research Online. https://openaccess.city.ac.uk/id/eprint/8459/.
- Pearce, M. T. (2018). Statistical learning and probabilistic prediction in music cognition: Mechanisms of stylistic enculturation. Annals of the New York Academy of Sciences, 1423(1), 378–395. https://doi.org/10.1111/nyas.2018.1423.issue-1
- Pearce, M. T., & Müllensiefen, D. (2017). Compression-based modelling of musical similarity perception. Journal of New Music Research, 46(2), 135–155. https://doi.org/10.1080/09298215.2017.1305419
- Pearce, M. T., Müllensiefen, D., & Wiggins, G. A. (2010). The role of expectation and probabilistic learning in auditory boundary perception: A model comparison. Perception, 39(10), 1367–1391. https://doi.org/10.1068/p6507
- Pearce, M. T., Ruiz, M. H., Kapasi, S., Wiggins, G. A., & Bhattacharya, J. (2010). Unsupervised statistical learning underpins computational, behavioural, and neural manifestations of musical expectation. NeuroImage, 50(1), 302–313. https://doi.org/10.1016/j.neuroimage.2009.12.019
- Potter, C. (2003). Debussy and nature. In S. Trezise (Ed.), The Cambridge Companion to Debussy (pp. 137–152). Cambridge University Press.
- Ren, I. Y., Koops, H. V., Volk, A., & Swierstra, W. (2017). In search of the consensus among musical pattern discovery algorithms. In Proceedings of the 18th International Society for Music Information Retrieval Conference (pp. 671–678). ISMIR Press.
- Reynolds, D. (2009). Gaussian mixture models. In S. Z. Li, & A. Jain (Eds.), Encyclopedia of Biometrics, (pp. 659–663). Springer.
- Rolison, J. J., & Edworthy, J. (2012). The role of formal structure in liking for popular music. Music Perception: An Interdisciplinary Journal, 29(3), 269–284. https://doi.org/10.1525/mp.2012.29.3.269
- Rumph, S. (2014). Topical figurae: The double articulation of topics. In D. Mirka (Ed.), The Oxford Handbook of Topic Theory (pp. 493–513). Oxford University Press.
- Sauvé, S. A. (2018). Prediction in polyphony: modelling musical auditory scene analysis [Doctoral dissertation, Queen Mary University of London]. Queen Mary Research Online. https://qmro.qmul.ac.uk/xmlui/handle/123456789/46805.
- Sauvé, S. A., Sayed, A., Dean, R. T., & Pearce, M. T. (2018). Effects of pitch and timing expectancy on musical emotion. Psychomusicology: Music, Mind, and Brain, 28(1), 17–39. https://doi.org/10.1037/pmu0000203
- Schenker, H. (1994). The masterwork in music: Volume 1, 1925 (W. Drabkin, Ed.; I. Bent, W. Drabkin, R. Kramer, J. Rothgeb, H. Siegal, Trans.). Cambridge University Press. (Original work published 1925).
- Sleator, D., & Temperley, D. (2003). The Melisma Music Analyzer [Computer software]. http://www.link.cs.cmu.edu/music-analysis/.
- Tan, S.-L., & Spackman, M. P. (2005). Listeners' judgments of the musical unity of structurally altered and intact musical compositions. Psychology of Music, 33(2), 133–153. https://doi.org/10.1177/0305735605050648
- Temperley, D. (2001). The cognition of basic musical structures. MIT Press.
- Tharwat, A (2018). Independent component analysis: An introduction. Applied Computing and Informatics, 17(2), 222–249. https://doi.org/10.1016/j.aci.2018.08.006
- Tillmann, B., & Bigand, E. (1996). Does formal musical structure affect perception of musical expressiveness? Psychology of Music, 24(1), 3–17. https://doi.org/10.1177/0305735696241002
- Uitdenbogerd, A., & Zobel, J. (1999). Melodic matching techniques for large music databases. In Proceedings of the 7th ACM International Conference on Multimedia (Part 1) (pp. 57–66). Association for Computing Machinery.
- Uitdenbogerd, A. L., & Zobel, J. (1998). Manipulation of music for melody matching. In Proceedings of the 6th ACM International Conference on Multimedia (pp. 235–240), Association for Computing Machinery.
- van der Weij, B., Pearce, M. T., & Honing, H. (2017). A probabilistic model of meter perception: Simulating enculturation. Frontiers in Psychology, 8, https://doi.org/10.3389/fpsyg.2017.00824Article 824.
- Wang, C., Li, J., & Shi, S. (2006). N-gram inverted index structures on music data for theme mining and content-based information retrieval. Pattern Recognition Letters, 27(5), 492–503. https://doi.org/10.1016/j.patrec.2005.09.012
- Whittall, A. (2001). Form. In Grove Music Online (pp. 493–513). Oxford University Press. https://doi.org/10.1093/gmo/9781561592630.article.09981
- Ziv, N., & Eitan, Z. (2007). Themes as prototypes: Similarity judgments and categorization tasks in musical contexts. Musicae Scientiae, 11(1 suppl), 99–133. https://doi.org/10.1177/1029864907011001051