ABSTRACT
Teachers make a difference for the outcome of their students in science classrooms. One focus in this context lies on teachers’ professional knowledge. We describe this knowledge according to three domains, namely (1) content knowledge (CK), (2) pedagogical content knowledge (PCK), and (3) curricular knowledge (CuK). We hypothesise a positive relationship between these three domains and students’ performance in science. Students’ science performance was conceptualised by system thinking performance in the context of biology teaching. In order to test our hypothesis, we examined the relationship between the knowledge triplet CK, PCK, and CuK and students’ performance. 48 biology teachers and their students (N = 1036) participated in this study. Teachers’ content-related professional knowledge and students’ performance were measured by paper-and-pencil tests. Moreover, we used concept maps to further assess students’ performance. By specifying doubly latent models, we found a significant positive relationship between biology teachers’ PCK and students’ performance. On the contrary, the results reveal no relationship between CK and CuK and students’ performance. These findings are discussed in respect to modelling the interrelationship of teachers’ content-related professional knowledge and students’ learning in science, as well as concerning their relevance for further research and teacher education programmes.
Introduction
The international large-scale study PISA (Programme for International Student Assessment) (e.g. OECD, Citation2013; Schiepe-Tiska, Schöps, Rönnebeck, Köller, & Prenzel, Citation2013) shows that students’ performance in science in many European countries (e.g. Spain, Norway, Hungary, Italy, Luxembourg, Portugal, and Sweden), in the U.S.A., and also in other countries outside Europe (e.g. Israel, Mexico, and Chile) still needs improvement. Thus, one of the overarching aims in current science education research is the identification of factors that could foster students’ performance in science.
It is well-established that teachers impact on the performance of their students (e.g. Cochran-Smith & Zeichner, Citation2005; Darling-Hammond, Citation2000b; Hattie, Citation2009; Rivkin, Hanushek, & Kain, Citation2005). Existing research indicates that teachers have a greater impact on students’ performance (d = .32) than class size (d = .21) or school finances (d = .23) (Hattie, Citation2009). Therefore, it is important to identify the characteristics of teachers (i.e. knowledge, attitudes, and beliefs) that are crucial for effective teaching and students’ performance. As teaching a particular subject matter is one of the core tasks of a science teacher, content-related professional knowledge should be of great importance when assessing teacher performance (e.g. Hashweh, Citation1987; Shulman, Citation1986; Tenorth, Citation2006). The importance of content-related professional knowledge is also reflected in standards for science teacher education (KMK, Citation2008 [Germany]; NSTA, Citation2012 [U.S.A.]). Nevertheless, there is no consensus on which domain of content-related professional knowledge most heavily impacts students’ science performance (e.g. Lange et al., Citation2015; Sadler, Sonnert, Coyle, Cook-Smith, & Miller, Citation2013). This study aims to contribute to the clarification of this problem. We consider three domains of content-related professional knowledge: (1) content knowledge (CK), pedagogical content knowledge (PCK), and curricular knowledge (CuK) (Shulman, Citation1986), and investigate how they are related to students’ science performance. To our knowledge, this is the first study to consider CuK as a unique domain of teachers’ content-related professional knowledge beyond CK and PCK. Moreover, it applies doubly latent multilevel analysis to elucidate the meaning of content-related professional knowledge for students’ performance, thus controlling for the hierarchical data structure, measurement error, and sampling error in the best possible way.
Background
Conceptualisation of content-related professional knowledge
Teachers’ professional knowledge is described as the core of professionalism (Baumert & Kunter, Citation2006). Shulman’s (Citation1986) initial description of teachers’ professional knowledge was a milestone for research on teacher effectiveness. He subsumed different knowledge domains that form the body of knowledge that is necessary for effective teaching. He originally considered four domains of professional knowledge: (a) pedagogical knowledge (PK), (b) CK, (c) PCK, and (d) CuK. PK is understood as the non-content-related domain of professional knowledge, whereas CK, PCK, and CuK represent the content-related domains of teachers’ professional knowledge. As teaching is a complex interplay between the teacher, the subject, and the student (Hashweh, Citation1987), this study focuses on content-related professional knowledge.
We engaged in the examination of the empirical structure of teachers’ content-related professional knwoledge in a previous study (Großschedl, Mahler, Kleickmann, & Harms, Citation2014) and found content-related professional knowledge to include CK, PCK, and CuK as unique domains. (See the Methods section for more information about this analysis.) Accordingly, the study at hand refers to (a) CK, (b) PCK, and (c) CuK as unique domains of teachers’ content-related professional knowledge.
CK. CK is a domain of knowledge which is relevant both for teachers and for content specialists. It not only covers factual knowledge (Ball & McDiarmid, Citation1989), but also offers a deep understanding of the structure of a specific domain (Shulman, Citation1986). This understanding includes knowledge about central concepts and principles as well as the knowledge of how validity and invalidity are established (Shulman, Citation1986). Moreover, CK concerns the awareness that a domain can be organised from different points of view. Biology is a good example to illustrate these different theoretical organisations: it can be understood not only as science of molecules, but also as science of ecosystems (Shulman, Citation1986). This deep conceptual understanding of the content is necessary as the provision of incoherent facts and information does not suffice to foster students in the acquisition of CK (Ball & McDiarmid, Citation1989).
PCK. PCK was initially described to comprise two facets. First, it includes the knowledge that allows the teacher to represent and formulate the subject matter in a manner that makes it comprehensible to students (Shulman, Citation1986). Grossman (Citation1990) further described this first facet as the knowledge of instructional strategies. The second facet of PCK includes knowledge of students’ (pre)conceptions (Shulman, Citation1986). Shulman’s initial model was extended by additional facets, but most of the recent models differentiate two common facets: (a) knowledge of instructional strategies for teaching and (b) knowledge of students’ understanding (cf. Großschedl, Harms, Kleickmann, & Glowinski, Citation2015; Lee & Luft, Citation2008; Park & Oliver, Citation2008; Schmelzing et al., Citation2013; Van Driel, Verloop, & de Vos, Citation1998). We follow this broad body of research and consider PCK to comprise these two facets.
The knowledge of instructional strategies for teaching includes the knowledge of subject-specific strategies like, for instance, the triggering of a cognitive conflict. Moreover, it covers the knowledge of topic-specific strategies. An example for such a topic-specific strategy is the support of students’ understanding of a specific science concept (Magnusson, Krajcik, & Borko, Citation1999). The use of representations (e.g. illustrations, models, examples, or analogies) is a very important aspect of this knowledge facet as they represent an effective tool to support students’ learning (Magnusson et al., Citation1999; Shulman, Citation1986). Beyond that, knowledge related to the implementation of subject-related activities (e.g. experiments) is further important for this facet of knowledge (Magnusson et al., Citation1999).
The knowledge of students’ understanding as the second facet of PCK covers the knowledge of the requirements of learning (e.g. required prior knowledge) as well as of learning difficulties (e.g. knowledge of abstract concepts that could cause misconceptions) (Magnusson et al., Citation1999).
What is important to keep in mind is that different studies consider different conceptualisations of PCK and thus have a different understanding of this knowledge domain. Although we understand PCK to be separable from CK and CuK, other scholars do not. Hill, Ball, Blunk, Goffney, and Rowan (Citation2007) consider mathematical knowledge for teaching, which comprises both CK and PCK within an integrated domain of content-related professional knowledge. Moreover, several studies assume CuK as an integrated facet of PCK (Grossman, Citation1990; Loughran, Milroy, Berry, Gunstone, & Mulhall, Citation2001; Magnusson et al., Citation1999; Park & Chen, Citation2012). These different conceptualisations of PCK have to be considered for the comparison of different studies.
CuK. Beyond CK and PCK, Shulman (Citation1986) defined CuK as a further category of content-related professional knowledge referring to the programmes and materials that serve as ‘tools of the trade’ for teachers (Shulman, Citation1987, p. 8). Grossman (Citation1990) expanded this initial definition of CuK by adding knowledge of the subject’s goals, the articulation of topics, and the knowledge of the vertical curriculum (i.e. the topics that surround the current topic). Furthermore, Magnusson and colleagues (Citation1999) include the knowledge of programmes and political regulations such as national educational standards. This short overview of theoretical conceptualisations demonstrates that CuK is understood in a very different manner depending on the different studies. Moreover, CuK is particularly considered as an integrated facet of PCK in the available research and there is accordingly a lack of research considering CuK as a unique domain. This vague understanding of CuK makes it difficult to identify the core facet of CuK. We follow the latter understanding of CuK (Magnusson et al., Citation1999) and focus on the knowledge of national educational standards for science education (KMK, Citation2010). This decision should not negotiate the relevance of the other described facets, but is grounded in the crucial relevance of science standards in the German educational system as well as in educational systems of several countries. The disappointing results in international studies (OECD, Citation2013; Schiepe-Tiska et al., Citation2013) and a lack of qualified trainees for scientific careers stress the need for the improvement of science education. In order to deal with this problem, several countries implemented science standards (e.g. ACARA, Citation2009 [Australia]; DfE, Citation2013 [Great Britain]; EDK, Citation2011 [Switzerland]; KMK, 2010 [Germany]; NGSS, Citation2013 [U.S.A.]). According to the findings of recent research in science education, science standards consider mandatory abilities that are necessary for a fundamental understanding of science (e.g. ACARA, Citation2009; DfE, Citation2013; EDK, Citation2011; KMK, Citation2010; NGSS, Citation2013). Moreover, science standards harmonise the assessment standards and educational requirements within one country (Cortina & Thames, Citation2013; EDK, Citation2011; KMK, Citation2010).
Content-related professional knowledge and students’ performance
To improve classroom teaching and science teacher education, we necessarily need to know whether science teachers’ content-related professional knowledge affects the performance of students and, if so, which domains impact student performance most strongly.
CK is often identified as a construct related to different characteristics of effective teaching. CK enables the effective cognitive activation of (Cochran & Jones, Citation1998; Darling-Hammond, Citation2000b; Hashweh, Citation1987) and interaction with students (Leinhardt & Smith, Citation1985). Teachers with strong CK show flexible teaching strategies by avoiding textbook-oriented teaching and considering learning difficulties (Hashweh, Citation1987). Moreover, CK is assumed to be important for the design of well-structured and problem-oriented lessons, considering adequate content-related learning environments (Baumert & Kunter, Citation2006; Darling-Hammond, Citation2000b; Hashweh, Citation1987; Rowan, Chiang, & Miller, Citation1997; Shulman, Citation1986). Normative official documents (e.g. standards for science teacher education) strongly stress the relevance of CK for teaching (KMK, Citation2008; NSTA, Citation2012). Despite this broad theoretical base, empirical studies related to science and different other school subjects show no consensus concerning the relationship between teachers’ CK and students’ performance. Some scholars did find an effect of CK on students’ science performance (Ohle, Fischer, & Kauertz, Citation2011; Sadler et al., Citation2013) or mathematics performance (Baumert et al., Citation2010; Hill, Rowan, & Ball, Citation2005; Rowan et al., Citation1997). On the contrary, other studies did not find an effect of CK on students’ science performance (Lange et al., Citation2015) or students’ reading performance (Carlisle, Correnti, Phelps, & Zeng, Citation2009). A closer examination of the respective studies in part reveals methodological issues. The first issue concerns the role of CK as a unique construct. In the study of Baumert and colleagues (Citation2010), CK and PCK are highly correlated. Hill and colleagues (Citation2005, Citation2007) refer to a domain of professional knowledge which is called mathematical knowledge for teaching. Mathematical knowledge for teaching represents an integrated construct which includes CK and PCK. Accordingly, it is difficult to infer conclusions about the significance of CK as a unique construct from the results of these studies. Second, Rowan et al. (Citation1997) only refer to one item in their study. This very narrow operationalisation of CK in this study makes it difficult to infer general information about the role of CK for students’ performance. Further research is needed to understand the meaning of CK for students’ performance.
PCK is likewise theoretically described as important for student outcomes. Teachers with a strong PCK are more likely to individually support their students (Kunter et al., Citation2013) and to choose adequate tasks, representations, or demonstrations that are related to the students’ everyday lives (Ball, Lubienski, & Mewborn, Citation2001; Magnusson et al., Citation1999). Furthermore, PCK is positively related to students’ cognitive activation in the classroom (Ball et al., Citation2001; Kunter et al., Citation2013; Magnusson et al., Citation1999). In addition, PCK helps the teacher to decide which specific support is necessary to enable students to deal with a given science concept and to survey prior knowledge, learning gains, and learning difficulties (Ball et al., Citation2001; Magnusson et al., Citation1999). The impact of teachers’ PCK on students’ performance is supported by empirical studies related to mathematics (Baumert et al., Citation2010; Fennema et al., Citation1996) and physics (Lange et al., Citation2015; Sadler et al., Citation2013).
Due to the important role of science standards for lesson planning and teaching (e.g. ACARA, Citation2009; DfE, Citation2013; EDK, Citation2011; KMK, Citation2010; NGSS, Citation2013), we believe that CuK also is an important domain to be considered when characterising an effective teacher. As CuK is often not considered to be a unique domain of content-related professional knowledge, empirical evidence regarding its impact on students’ performance is missing from the literature. To our knowledge, this study is the first one to address this lacuna.
Students’ science performance
In our study, students’ science performance was conceptualised by the construct system thinking in biology. The ability to understand systems and to use this knowledge for solving scientific problems – summarised here as system thinking – is fundamental for a meaningful understanding of science (e.g. Brandstädter, Harms, & Großschedl, Citation2012; Hannon & Ruth, Citation2000).
Biology is the science of living and open systems, which exchange matter, energy, and information with their environment (Verhoeff, Citation2003). Dealing with biological systems is a challenge for each student (Assaraf & Orion, Citation2005). Accordingly, one central task of biology teachers is to support students in their system thinking abilities. The importance of system thinking is reflected in different educational standards of many countries by considering system as a superordinate concept (e.g. ACARA, Citation2009; DfE, Citation2013; EDK, Citation2011; KMK, Citation2010; NGSS, Citation2013). Several scholars, especially from geography and biology education, study which abilities are necessary to be able to deal with systems. Current definitions of system thinking (e.g. Assaraf & Orion, Citation2005, Citation2010; Evagorou, Korfiatis, Nicolaou, & Constantinou, Citation2009; Riess & Mischo, Citation2010; Sommer & Lücken, Citation2010; Verhoeff, Citation2003) share two fundamental aspects: (1) the ability to organise the system’s elements within a system’s framework and (2) the ability to understand the relationships between these elements. Brandstädter and colleagues (Citation2012) expanded this groundwork by describing two domains of system thinking. The first domain – structural system thinking – is defined as the ability to identify and connect the elements of a system, to organise these elements in a reference framework, and to identify and draw system borders (Brandstädter et al., Citation2012; Sommer & Lücken, Citation2010). The second domain – procedural system thinking – concerns the ability to differentiate between the properties of the system and the properties of its elements, as well as the ability to identify dynamic relationships and to understand and predict consequences of changes within a system. In addition, it covers the understanding and evaluation of effects and reactions within a system (Brandstädter et al., Citation2012; Sommer & Lücken, Citation2010).
Research question
Our aim is to further clarify the relationship between the three domains of biology teachers’ content-related professional knowledge and students’ science performance. Hence, we address the following research question: How are biology teachers’ (a) CK, (b) PCK, and (c) CuK related to students’ science performance?
Referring to the state of research (cf. Content-related professional knowledge and students’ performance), we assume a positive relationship between CK and students’ science performance. Likewise, we assume a positive relationship between PCK and students’ science performance. Moreover, we assume that the relationship between PCK and students’ science performance is stronger than the relationship between CK and students’ science performance (Baumert et al., Citation2010). As science standards intend the improvement of learning and teaching (e.g. ACARA, Citation2009; DfE, Citation2013; EDK, Citation2011; KMK, Citation2010; NGSS, Citation2013), we likewise hypothesise a positive relationship between CuK and students’ science performance.
Methods
Sample and procedure
Sample. A total of 48 biology teachers (75% female) participated in our study. The teachers were on average 40.91 years old (SD = 11.02, min = 24 years, max = 64 years) and had on average 11.58 years of teaching experience (SD = 10.46). The teachers were recruited from different secondary schools in Northern Germany (Hamburg, Schleswig-Holstein). In Germany, there is a distinction on the secondary level between schools qualifying their students for an academic career (German Gymnasium; academic track; 54.2% of the participating schools) or for a vocational career (German Hauptschule, Realschule, Gesamtschule, Gemeinschaftsschule; non-academic track; 45.8% of the participating schools). Accordingly, prospective secondary school teachers choose their teacher education programme qualifying them either for academic track schools or for non-academic track schools. In all, 58.3% of the participating biology teachers were certified for the academic track, 39.6% were certified for the non-academic track, and 2.1% held a degree which is not related to teacher education (e.g. a master’s degree in biology). The biology teachers participated together with their 7th-grade (20.1%) and 8th-grade (79.9%) students (N = 1036, 50.6% female). The students were on average 13.5 years old (SD = 0.73).
Procedure. The participating biology teachers were recruited by telephone or mail. To allow for testing, the relationship between teachers’ professional knowledge and students’ science performance of a science topic was selected that all participating teachers and students had to deal with in the course of the study: the ‘ecosystem Wadden Sea’ and the morphology and life of Mytilus edulis (the Blue Mussel; respiration, development, nutrition, predators, mussel farms, and fishing industry; for more details, see Fraune, Citation2014). This topic is related to the environment of students from Northern Germany and represents a common content in the local biology education programme.
First, the teachers were asked to complete paper-and-pencil tests measuring their CK, PCK, and CuK. Subsequently, the teachers were requested to plan a short unit (four lessons) on the above described topic ‘ecosystem Wadden Sea’. Students’ performance was measured before and after being taught the unit ‘ecosystem Wadden Sea’ by their teachers.
The integration of the particular teaching unit in our study was a precondition for controlling for students’ prior knowledge in the following data analysis. We decided to measure teachers’ content-related professional knowledge previous to the unit as we were interested in the initial level of content-related professional knowledge when they start to teach their students concerning the ‘ecosystem Wadden Sea’.
Measures
Teachers’ content-related professional knowledge. Biology teachers’ content-related professional knowledge was measured using a paper-and-pencil test with the three subscales (a) CK, (b) PCK, and (c) CuK.
For the assessment of teachers’ professional knowledge (especially for PCK), there is no consensus in the literature concerning the adequateness of paper-and-pencil tests; both qualitative and quantitative methods were used to measure teachers’ professional knowledge. Qualitative approaches (e.g. interviews and lesson observations) have the advantage that they provide detailed information (Ball, Thames, & Phelps, Citation2008; Depaepe, Verschaffel, & Kelchtermans, Citation2013; Jüttner & Neuhaus, Citation2013; Park & Oliver, Citation2008; Peng, Citation2007; Van Driel, De Jong, & Verloop, Citation2002). A disadvantage is the amount of time for both the assessment and the analysis of the qualitative data. Thus, qualitative approaches are especially appropriate for studies with a small sample (e.g. Davis, Citation2009; Peng, Citation2007). Several studies with larger teacher samples successfully used paper-and-pencil tests to measure teachers’ content-related professional knowledge (e.g. Baumert et al., Citation2010; Großschedl & Harms, Citation2013; Hill, Schilling, & Ball, Citation2004; Jüttner, Boone, Park, & Neuhaus, Citation2013; Schmidt et al., Citation2007). The advantage of the application of paper-and-pencil tests is the adequate amount of time for the assessment as well as for the analysis of the data. In a paper-and-pencil test, both closed- and open-ended items can be included. Open-ended items give a more detailed insight into the mental processes of the teachers during answering the items (Brandstädter et al., Citation2012; Schoenfeld, Citation2007). Nevertheless, open-ended items have the disadvantage of a high amount of test time (i.e. applying open-ended items is a demanding procedure for the teachers) as well as a time-consuming rating of the items (e.g. Lipton & Huxham, Citation1970; Walstad & Becker, Citation1994). Moreover, the subjectivity of the raters could raise problems (Lipton & Huxham, Citation1970; Walstad & Becker, Citation1994). In contrast, closed-ended items, as for instance multiple-choice items, are applicable in an adequate amount of time also in large samples (Bacon, Citation2003; Lipton & Huxham, Citation1970) so that more items can be included (i.e. providing a broader overview of teachers’ content-related professional knowledge) (Lipton & Huxham, Citation1970; Walstad & Becker, Citation1994). Both item formats were found to be adequate (e.g. Bennett, Rock, & Wang, Citation1991; Wainer & Thissen, Citation1993; Walstad & Becker, Citation1994).
As we deal with a larger sample, we used a paper-and-pencil test to measure teachers’ content-related professional knowledge. We decided for a mixture of closed-ended and open-ended items depending on the domain of knowledge. Concerning PCK and CuK, we were interested in a more in-depth insight (e.g. into concrete lesson planning considerations) and applied open-ended items, whereas CK was measured with closed-ended items.
The subscales for CK and PCK both referred specifically to the topic ‘Ecosystem Wadden Sea’ focusing on the Blue Mussel. The subscale CuK addressed the entire subject biology. Here we refer to the German National Educational Standards for the Intermediate School Leaving Certificate in Biology (KMK, Citation2010). (See for further information about the measures and descriptive statistics. Appendix provides item examples.)
Ten per cent of the test booklets were randomly selected and the respective open-ended items were separately coded by two researchers to estimate intercoder reliability (Wirtz & Caspar, Citation2002). The intra-class correlation (ICC) coefficient was on average .97, which indicates good intercoder reliability. We used the ACER ConQuest software (version 1.0.0.1; Wu, Adams, Wilson, & Haldane, Citation2007) to analyse the data from the teachers’ paper-and-pencil test. In order to estimate the person ability, we used the weighted maximum likelihood (WLE) method, which is, compared to maximum likelihood estimation, less biased (Warm, Citation1989). We used the WLE scores for CK, PCK, and CuK for the following analysis.
Students’ science performance. In order to assess students’ system thinking as an example of science performance, we used a paper-and-pencil test as well as concept maps.
Students’ system thinking is assessed using different methods such as interviews, videotaping, paper-and-pencil tests (Assaraf & Orion, Citation2010; Evagorou, Korfiatis, Nicolaou, & Constantinou, Citation2009), and concept maps (Assaraf & Orion, Citation2005; Brandstädter et al., Citation2012; Sommer & Lücken, Citation2010). According to the large student sample, we decided to apply a paper-and-pencil test with a mixture of closed-ended and open-ended items to measure students’ system thinking. Moreover, as system thinking includes a conceptual understanding of a system’s structure, concept maps are a promising method to measure students’ system thinking (Brandstädter et al., Citation2012) and are considered as further instrument in the study at hand. Concept maps are used to make internal mental models visible (Yin, Vanides, Ruiz-Primo, Ayala, & Shavelson, Citation2005), and they allow one to assess these mental models with regard to a conceptual understanding of the given science content (Assaraf & Orion, Citation2005; Evagorou et al., Citation2009; Ruiz-Primo & Shavelson, Citation1996). Concept maps consist of terms connected by labelled lines, which represent the relations between the concepts. Different concept mapping techniques are applied in educational research; they are particularly determined by the medium used (paper–pencil vs. computer-based) and the level of directedness (high vs. low) (Brandstädter et al., Citation2012). In a highly directed setting, the students receive a set of concepts (e.g. Blue Mussel or star fish) and relations (e.g. protect or feed). Brandstädter and colleagues (Citation2012) identified the computer-based and highly directed method as most valid. Accordingly, we chose this setting for the study at hand.
The paper-and-pencil test consists of the subscales (a) structural system thinking and (b) procedural system thinking and was validated in a previous study (Brandstädter et al., Citation2012). The subscales refer to the topic ‘Ecosystem Wadden Sea’ focusing on the Blue Mussel as well. We analysed the data analogous to the paper-and-pencil test measuring biology teachers’ content-related professional knowledge (Großschedl et al., Citation2014). (See for further information related to the measures and descriptive statistics. Appendix provides item examples.)
The students developed their concept maps using the software MaNET (Eckert, Citation1998). Ten concepts (e.g. Blue Mussel; see ) and four linking words (e.g. protect; see ) were given to the students.
The user interface contained the different concepts and the different linking words shown in a pop-up window. The software MaNET (Eckert, Citation1998) was also used to analyse the students’ concept maps. The underlying principle of the analysis is the comparison between a student map and a reference map. The reference map (see ) was developed together with experts (science educators and biologists) as follows: we derived the set of concepts from expert maps related to the topic Mytilus edulis in the ecosystem Wadden Sea (e.g. development, enemies, and living). We used these concepts to develop a new concept map, which was finally revised by the experts. The correspondence coefficient (C) gives information about the similarity between concept maps (in this case, between the reference map and the student map). The underlying principle is provided in .
Table 1. Database for the correspondence coefficient C (translated; Eckert, Citation1998).
Different levels of strictness can be applied to the data. The least strict level merely considers the presence of a connection between two concepts (yes vs. no). Beyond that, the medium strict level considers the selection of a relation from the given set of relations (right vs. wrong). Finally, the strictest level additionally considers the direction of the relation (right vs. wrong) (Eckert, Citation1998). We refer to the strictest level in our study. The correspondence coefficient was weighted (Cw) to take account of the fact that a relation in a student map is more meaningful when the reference map has relatively few relations (Eckert, Citation1998). Eckert (Citation1998) recommends the weighted correspondence coefficient when concept maps are compared with a reference map. The weighted correspondence coefficient is estimated as follows (Eckert, Citation1998):
Note. ;
(see also ); HIT = correct connection; CR = correct rejection; MISS = missing connection despite connection in the reference map; FA = connection despite missing connection in the reference map (false alarm) (translated; Eckert, Citation1998).
We used the weighted correspondence coefficient for the following analysis.
Control variables. We applied two subscales of a cognitive abilities test (verbal, non-verbal; KFT 4–12 R; Heller & Perleth, Citation2000) to control for cognitive abilities on the student level (see ). Cognitive abilities are an important predictor for students’ performance (Boulanger, Citation1981; Hattie & Hansford, Citation1982; Piburn, Citation1993). The verbal subscale refers to the relationships between words; the non-verbal subscale refers to figural relationships. We used two versions of the test (A and B) to keep the students from copying. Moreover, we considered students’ prior knowledge (performance in the pretest). (See for further information and descriptive statistics.) In addition, we considered the school type. We distinguish between academic track schools and non-academic track schools (see Sample and procedure).
Table 2. Measures – descriptive statistics.
Psychometric properties of the measures. This section provides the psychometric properties of the applied measures on the teacher and student levels. We applied a Rasch analysis to analyse the data. (See Großschedl et al., Citation2014 for a detailed consideration of the psychometric properties for the measures for CK, PCK, and CuK.)
The items forming the measures for CK, PCK, and CuK had an acceptable item fit (weighted mean square (WMNSQ)) ranging within 0.86–1.20. As displayed in , the items discriminate adequately (range: 0.20–0.87). Item difficulties vary between easy and difficult and range from 0.26 to 0.88. Expected a posteriori/plausible values reveal adequate reliability of the respective scales (range: 0.71–0.86). The items that were considered to measure students’ system thinking have an adequate item fit (WMNSQ) ranging within 0.90 and 1.09 for the pretest and 0.93 and 1.09 for the posttest. Both easy and difficult items are included in the measure. The item difficulty ranges from 0.13 to 0.85 for the pre-test and from 0.29 to 0.89 for the post-test. As expected, the pre-test is more difficult compared with the post-test. The items of the pre- and the post-test discriminated adequately; most of them are in a range between 0.20 and 0.80 (pre: n of items = 23; post: n of items = 23). (See for detailed information about the psychometric properties of the measures.)
Table 3. Psychometric properties of the measures.
Statistical analysis
Dimensionality of biology teachers’ content-related professional knowledge. As a first step, it is important to ensure that the three assumed domains of biology teachers’ content-related professional knowledge (1) CK, (2) PCK, and (3) CuK are also empirically separable. In order to do so, we conducted a Rasch analysis and compared different models. The first model assumes biology teachers’ content-related professional knowledge to be one dimensional. Accordingly, CK, PCK, and CuK are allocated to a single dimension. The second model assumes biology teachers’ CK to be allocated to one dimension and biology teachers’ PCK and CuK to be allocated to a second dimension. Finally, the third model assumes biology teachers’ content-related professional knowledge to be a three-dimensional construct, with CK, PCK, and CuK as unique dimensions. The Rasch analysis shows that the three-dimensional model (deviance: 6502.98, AIC (Akaike's [Citation1981] Information Criterion): 6620.98, BIC (Bayes' Information Criterion [Wilson, deBoeck, & Carstensen, Citation2008]): 6791.95) outperforms the one-dimensional model (deviance: 6713.86, AIC: 6821.86, BIC: 6978.34) and the two-dimensional model (deviance: 6560.09, AIC: 6672.09, BIC: 6834.37). A χ2-test was performed and reveals that the three-dimensional model significantly outmatches the one-dimensional model, χ2[2] = 210.88, p < .05, and the two-dimensional model, χ2[2] = 57.12, p < .05. Despite their separability, the three domains are correlated. We found a high correlation between PCK and CuK (r = .70, p < .001) and a medium correlation between CK and PCK (r = .48, p < .001) as well as between CK and CuK (r = .35, p < .001). The detailed results are reported in Großschedl et al. (Citation2014).
Doubly latent model. To investigate whether biology teachers’ CK, PCK, and CuK are predictive for students’ science performance, we specified a doubly latent model (Marsh et al., Citation2009), which integrates a structural equation model and a multilevel model. In this doubly latent model, the dependent variable (here: students’ performance measured in the post-test) is added as a latent trait on both the individual and the class level. This model controls for measurement error (sampling of items on both levels) and sampling error (sampling of individuals in the aggregation from the individual to the class level) (Marsh et al., Citation2009). We chose this method because of the hierarchical structure in our data set. A hierarchical structure occurs when data measured on one level are clustered in another level, for example, when individuals are clustered in groups (here: students in classes) (Nezlek, Schröder-Abé, & Schütz, Citation2006). As a result of the clustering, the observations are not independent. For a correct estimation of effects and standard errors, the consideration of the hierarchical structure is necessary (Nezlek et al., Citation2006). We used the software Mplus for this analysis (Muthén & Muthén, Citation2007).
In order to check whether specifying a doubly latent model is necessary for our data, we specified unconditional models to decompose the variance of the dependent variable (students’ performance in the post-test) into the proportion of variance lying within classes and the proportion of variance lying between classes. As students’ system performance is modelled as latent trait, separate unconditional models were computed for each indicator: (1) structural system thinking, (2) procedural system thinking, and (3) system thinking performance measured with concept maps. The estimation of the ICC shows that (1) 27.3%, (2) 22.7%, and (3) 23.9% of the variance are located between the classes. An ICC of .05 or even .01 (5% or 1% variance between the classes; Cohen, Raudenbush, & Ball, Citation2003) is required for the consideration of a multilevel structure.
As mentioned above, the dependent variable is considered as the latent trait on both levels. The indicators of the dependent variable appear as latent variables on the class level, because the variance between classes within these indicators (intercept modelled as random effect) is added as the latent variable on the class level (see ). To predict performance (post), (a) CK, (b) PCK, and (c) CuK are added as independent variables on the class level.
Figure 2. Multilevel model. Note: CK = content knowledge, PCK = pedagogical content knowledge, CuK = curricular knowledge, ST = system thinking, SST = structural system thinking, PST = procedural system thinking, CM = concept mapping performance, KFT n = cognitive abilities non-verbal, KFT v = cognitive abilities verbal, pre = pre-test, post = post-test, ● = intercept (DV on indicators) is modelled as random effect, varying between classes.
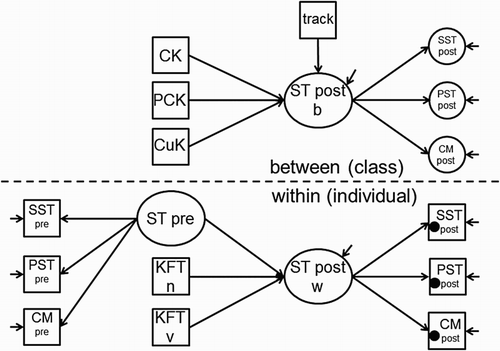
Control variables. We considered control variables on both the individual and the class level. On the individual level, we added students’ performance in the pre-test, which is modelled as a latent trait analogous to the performance in the post-test. Moreover, we added students’ cognitive abilities (verbal and non-verbal). On the class level, we added the track (academic track vs. non-academic track) as a control variable. gives an overview of the multilevel model.
Missing values. Our data set contains missing values (concept mapping performance pre: 11.7%, concept mapping performance post: 14.6%, cognitive abilities verbal: 0.19%, cognitive abilities non-verbal: 0.78%). Instead of deleting cases with missing values (list-wise deletion) which leads to a reduction of sample size and statistical power (Peugh & Enders, Citation2004), we used multiple imputation to estimate the missing values. Multiple imputation is applicable when values are missing completely at random or missing at random (Rubin, Citation1976). First, 10 imputed data sets are generated, each including plausible values instead of the missing values. Multiple imputation accounts for uncertainty in the imputation of plausible values. The following analysis is computed with each of these 10 data sets. Afterwards the estimates are combined to get one result. We used Mplus to generate and analyse the imputed data sets (Muthén & Muthén, Citation2007).
Results
To answer our research question, we investigated how the variance of students’ performance between classes, controlled for variables on the individual level and the class level, is predicted by biology teachers’ content-related professional knowledge. In order to do that, we specified different models.
Model 1 takes the control variables on the individual and the class level into account. The results show that students’ performance in the pre-test is significantly positively related to their performance in the post-test. Furthermore, there is a significant positive relationship between students’ verbal and non-verbal cognitive abilities and their performance in the post-test. Model 2 considers biology teachers’ CK as a predictor for students’ performance. The results show that there is no relationship between biology teachers’ CK and students’ performance. Consequently, adding CK to the model does not markedly contribute to the explanation of variance on the class level when compared with Model 1. Model 3 considers biology teachers’ PCK as a predictor. The results show a significant positive relationship between biology teachers’ PCK and students’ performance. A comparison of Model 1 and Model 3 shows that PCK contributes to the explanation of variance on the class level. Biology teachers’ CuK is considered as a predictor in Model 4. No significant relationship occurs and no noteworthy additional amount of variance is explained on the class level. The three domains of biology teachers’ content-related professional knowledge are considered together in Model 5. Analogous to the other models, the positive relationship between PCK and students’ performance remains significant, whereas no significant relationships occur between CK as well as CuK and students’ performance. As expected, this model is able to explain more variance than the models considering a single domain of biology teachers’ content-related knowledge. Detailed results are provided in .
Table 4. Results of the multilevel analysis (standard errors in parentheses).
Discussion
The aim of our study was to elucidate the meaning of biology teachers’ content-related professional knowledge for students’ science performance.
Contrary to our assumption, the results show no direct relationship between biology teachers’ CK and students’ performance. This finding is in line with other studies focusing on language teachers’ (Carlisle et al., Citation2009) and primary science teachers’ CK, respectively (Lange et al., Citation2015). However, other scholars did find such relationship (Baumert et al., Citation2010; Hill et al., Citation2005, Citation2007; Ohle et al., Citation2011; Rowan et al., Citation1997; Sadler et al., Citation2013). The studies which were able to find an effect are related to mathematics and physics. A reason for the incoherent results could be the underlying biological topic of our measure. It focuses on open living biological systems. To deal with open living systems, students have to go beyond the acquisition of factual knowledge. This means that they have to reconstruct knowledge in complex networks as well as understand interrelationships and effects within a system. Obviously, mere CK does not suffice to support students in the acquisition of system thinking abilities in biology. Open living systems are not in the focus of mathematics and physics education. The review of the underlying physical topics that were considered in the study of Sadler et al. (Citation2013) as well as the mathematical items that were applied in the Coactiv study (Baumert et al., Citation2010) or in the study of Rowan et al. (Citation1997) supports this assumption.
However, we do not assume that CK is completely irrelevant for students’ performance. As CK is an important prerequisite for the development of PCK (e.g. Ball et al., Citation2001; Großschedl et al., Citation2015; Krauss et al., Citation2008; Ma, Citation1999; Magnusson et al., Citation1999; Riese & Reinhold, Citation2012), we suppose an indirect effect of CK on system thinking being mediated by PCK. To clarify this, further studies are needed.
The positive relationship we found between PCK and students’ performance is in line with the results of different studies related to mathematics teachers’ PCK (Baumert et al., Citation2010; Fennema et al., Citation1996; Kunter et al., Citation2013), physics teachers’ PCK (Sadler et al., Citation2013), or primary science teachers’ PCK (Lange et al., Citation2015). As the conceptualisation of PCK in these different studies is relatively similar to our study (e.g. due to the consideration of students’ misconceptions or instructional strategies), a more consistent picture compared with the results related to CK seems plausible.
In addition, PCK is theoretically described as important for students’ performance (Ball et al., Citation2001; Kunter et al., Citation2013; Magnusson et al., Citation1999). Unlike CK, PCK seems to be effective in supporting students’ ability to face the complex demands described above, when acquiring system thinking abilities.
As science standards intend the improvement of learning and teaching (e.g. ACARA, Citation2009; DfE, Citation2013; EDK, Citation2011; KMK, Citation2010; NGSS, Citation2013), we considered a positive relationship between teachers’ CuK and students’ system thinking performance. Contrary to our expectation, we found no relationship between biology teachers’ CuK and students’ performance, indicating that CuK does not help students to acquire system thinking abilities. System is a superordinate issue in these standards. Thus, we do not assume that biology teachers’ CuK is completely irrelevant for students’ system thinking performance.
Although we consider CuK as a unique domain in the study at hand, we do not assume that PCK and CuK are completely independent of each other. In other words, teachers must be knowledgeable concerning the standards they should meet in their lessons. It could be assumed that the knowledge of the German National Educational Standards for the Intermediate School Leaving Certificate in Biology (KMK, Citation2010) helps the teacher to identify difficult and abstract concepts as well as the relevant knowledge base and necessary skills for the understanding of these concepts. Accordingly, an indirect effect between CuK and students’ performance with PCK as the mediating variable can be assumed. This interplay between CuK and PCK is supported by the result that CuK and PCK are, despite their separability, correlated.
We hypothesise that an instrument which is more strongly related to the activity of lesson planning, teaching, and assessment (i.e. the implementation of the educational standards in the daily teaching routine) would have given more information about the meaning of CuK for students’ performance.
To summarise, our results indicate that PCK seems to be the driving force for the improvement of students’ science performance. Moreover, they allow us to carefully assume a relevance of PCK also for other teacher populations. System thinking abilities are described to be likewise relevant for other science subjects beyond biology (Australia: ACARA, Citation2009; England: DfE, Citation2013; Switzerland: EDK, Citation2011; U.S.A.: NGSS, Citation2013) and geography (Rempfler & Uphues, Citation2012).
Limitations
Concerns are related to the instrument measuring CuK. This instrument basically refers to factual knowledge about the German National Educational Standards for the Intermediate School Leaving Certificate in Biology (KMK, Citation2010). These standards aimed a massive change in educational requirements and assessment standards (KMK, Citation2005). To reflect these changes, it is obviously not sufficient to measure only factual knowledge. A measure which focuses more strongly on the implementation of the standards would have been more applicable.
Our study was conducted in a cross-sectional setting. Due to this, it is not possible to draw causal conclusions concerning the relationship between biology teachers’ content-related professional knowledge and students’ system thinking performance. A longitudinal design would help to solve this methodological issue.
A further remark is related to the application of the measure for teachers’ content-related professional knowledge. As mentioned in the ‘Methods’ section, we measured teachers’ CK, PCK, and CuK previous to the four lessons the teachers conducted. We did this in order to measure the initial level of content-related knowledge the teachers referred to. Nevertheless, it would have been fruitful to measure teachers’ content-related professional knowledge also after the teaching unit to consider the change in teachers’ content-related professional knowledge.
Implications
Implications for further research
The findings concerning CK and its meaning for students’ performance are inconsistent in recent research. Although we did not find a relationship in our study as well, we do not assume that CK is irrelevant for students’ performance. Further exploration is needed. A topic for further research is to explore whether a certain level of CK is necessary to foster students’ performance. This question leads to two assumptions. On the one hand, it could be assumed that CK is only effective until a certain level is reached (Darling-Hammond, Citation2000b). Apart from that, CK has no additional effect on students’ performance. Thinking the other way round, only teachers with a well-developed CK could be effective in influencing their students’ performance. Comparing teacher groups with different levels of CK would contribute to the clarification of this issue. A larger teacher sample would have been necessary in order to compare groups in a multilevel design.
CuK as a unique category of content-related professional knowledge has been neglected in research so far. Given that many countries have moved to develop and implement educational standards (e.g. ACARA, Citation2009; DfE, Citation2013; EDK, Citation2011; KMK, Citation2010; NGSS, Citation2013), further research into the influence of CuK on student performance is warranted. To get an in-depth understanding about the meaning of CuK for students’ performance, the development of a more applicable instrument is necessary. This instrument should combine factual knowledge about the official requirements formulated in the standards and the implementation of these standards in teaching and assessment. It should be easily adaptable to the different requirements in the different countries.
Our analysis focused only on direct effects, but it would be fruitful to consider possible indirect effects in the model. As CK is identified as important for the development of PCK (e.g. Ball et al., Citation2001; Großschedl et al., Citation2015; Krauss et al., Citation2008; Ma, Citation1999; Magnusson et al., Citation1999; Riese & Reinhold, Citation2012), we assume an indirect effect of CK on students’ performance mediated by PCK. A further possible indirect relationship concerns the relationship between CuK and students’ performance. As CuK could be assumed to be a precursor for PCK, it would be interesting to consider an indirect relationship between CuK and students’ performance including PCK as a mediator. This would further clarify the role of CuK for effective teaching. As we have a relatively small teacher sample and as PCK and CuK had been simultaneously measured, we were not able to test this assumption in the framework of our study. To further understand the meaning of teachers’ content-related professional knowledge and its significance for students’ performance, it would be interesting to add the quality of lesson planning as a mediator. A large proportion of students’ learning process is situated in the respective lessons and the preparation of these lessons is thus an important determining factor for the quality of the lessons. Several studies give hints that at least CK (Baumert & Kunter, Citation2006; Rowan et al., Citation1997; Shulman, Citation1986) and PCK (Ball et al., Citation2001; Magnusson et al., Citation1999) are important for effective lesson planning. We assume also CuK to be relevant in this regard. In order to investigate this indirect relationship, participating teachers should provide detailed information about their lesson planning (e.g. grids for an overview of the structure of a lesson, information about the aims of the lesson, and allocation of these aims in the educational standards).
The activity of teaching was not observed in our study. More qualitative approaches such as lesson observations (e.g. videotaping) could have given a deeper insight on how CK, PCK, and CuK are reflected in the activity of teaching. Doing this could contribute to the clarification of the role of CK and CuK for students’ achievement.
It is clearly stated in the literature and supported by our findings that the teacher plays an important role for the performance of students. It would be fruitful to expand the model to further investigate which aspects besides content-related professional knowledge are important for students’ science performance. Non-content-related professional knowledge (e.g. PK) and motivational orientations (e.g. teacher enthusiasm) are promising predictors to further clarify the teachers’ role for students’ system thinking performance in biology.
Implications for teacher education
The professionalisation of teachers is important to ensure quality within educational systems (e.g. Ashton & Crocker, Citation1987; Baumert & Kunter, Citation2006; Darling-Hammond, Citation2000a; Evertson, Hawley, & Zlotnik, Citation1985; Grossman, Citation1990; Lipowsky, Citation2006). As our study confirms that PCK has an impact on students’ science performance, the acquisition of PCK in pre-service and in-service science teacher education should be strengthened.
Pre-service teacher education at a university particularly aims at the acquisition of teachers’ content-related professional knowledge (KMK, Citation2008) and represents a very important phase for its development (Kleickmann & Anders, Citation2013; Schmidt et al., Citation2007; Tatto & Senk, Citation2011). Hence, arguing for the support of PCK-related courses during teacher education at a university is mandatory. The consideration of the acquisition of CK is of further importance, as CK is described as an important prerequisite for the development of PCK (e.g. Ball et al., Citation2001; Großschedl et al., Citation2015; Krauss et al., Citation2008; Ma, Citation1999; Magnusson et al., Citation1999; Riese & Reinhold, Citation2012). This assumption is supported by the finding that academic track teachers, who received – compared to non-academic track teachers – a higher amount of CK training but a comparable amount of PCK training, score higher concerning both CK and PCK (Baumert et al., Citation2010; Krauss et al., Citation2008). This interrelationship between CK and PCK during the development of PCK points towards possible benefits of integrated courses. These courses should provide the opportunity to acquire CK related to a certain science topic and to simultaneously acquire knowledge about the integration of this science topic in lessons as well about possible learning difficulties (i.e. PCK related to a certain science topic). As teaching experience acquired during practical phases in teacher education at a university is found to be beneficial for the development of PCK (Großschedl et al., Citation2015), the implementation of more compulsory practical phases and more possibilities for voluntary commitment in school could be further beneficial for the improvement of teachers’ PCK.
Also in-service teacher education provides fruitful opportunities for the development of PCK (e.g. Brunner et al., Citation2006; Clermont, Krajcik, & Borko, Citation1993). Several scholars examined the effectiveness of different formats of professional development courses. Due to their findings, different implications for the organisation or possible content of such courses can be inferred. Clermont and colleagues (Citation1993) found that chemistry teachers’ PCK benefits from intensive professional development (here: a two-week summer workshop) with a combination of both theoretical and practical phases. Concerning possible content of professional development courses, the reflection of own teaching practice and the teaching practice of colleagues (e.g. using videos of the respective lessons) have been identified as meaningful for the development of science and biology teachers’ PCK (Magnusson et al., Citation1999; Rozenszajn & Yarden, Citation2014). Furthermore, workshops that aim at supporting teachers in integrating new teaching methods and materials in their lessons are found to support PCK of biology teachers (Rozenszajn & Yarden, Citation2013). Knowledge of students’ understanding represents an important facet of PCK (e.g. Shulman, Citation1986). To foster teachers’ PCK, Magnusson and colleagues (Citation1999) recommend the consideration of teachers’ own understanding of specific science concepts and learning difficulties in professional development courses.
Conclusions
The aim of this study was to investigate the meaning of the different domains of teachers’ content-related professional knowledge for students’ performance in science. Both the consideration of CuK as a unique domain and the conduction of a doubly latent multilevel analysis are new in this field of research. An additional strength of our study is that we were able to gather a deep insight into students’ performance by using concept maps in addition to the paper-and-pencil test. Our results support the relevance of teachers’ PCK and indicate that merely CK is not enough to foster students’ performance in science. This supports the special role of the science teacher, in contrast to a scientist, for students’ performance. Moreover, our study illustrates the relevance to further consider CuK in research.
Students’ science performance is a function of multiple factors, both individual and contextual ones. Contrary to relatively stable factors such as socio-economic status or cognitive abilities, the ‘science teacher factor’ is malleable and can be positively influenced particularly by teacher education (e.g. content-related professional knowledge [Großschedl et al., Citation2014] and motivational orientations [Mahler, Großschedl, & Harms, Citationsubmitted for publication]). This implies that students’ science performance could be actively improved by considering science teachers and their education as important parameters.
Acknowledgements
We would like to thank Kerstin Münchhoff for her fundamental contribution to the realisation of the project. Furthermore, we are very grateful to Jeffrey Nordine for commenting and proofreading this article.
Disclosure statement
No potential conflict of interest was reported by the authors.
Additional information
Funding
References
- Akaike, H. (1981). Likelihood of a model and information criteria. Journal of Econometrics, 16, 3–14. doi: 10.1016/0304-4076(81)90071-3
- Ashton, P., & Crocker, L. (1987). Systematic study of planned variations: The essential focus of teacher education reform. Journal of Teacher Education, 38(3), 2–8. doi: 10.1177/002248718703800302
- Assaraf, O. B. Z., & Orion, N. (2005). Development of system thinking skills in the context of earth system education. Journal of Research in Science Teaching, 42(5), 518–560. doi: 10.1002/tea.20061
- Assaraf, O. B. Z., & Orion, N. (2010). System thinking skills at the elementary school level. Journal of Research in Science Teaching, 47(5), 540–563.
- Australian Curriculum Assessment and Reporting Authority [ACARA]. (2009). Shape of the Australian curriculum: Science. Retrieved June 9, 2016, from http://www.acara.edu.au/verve/_resources/Australian_Curriculum_-_Science.pdf
- Bacon, D. R. (2003). Assessing learning outcomes: A comparison of multiple-choice and short-answer questions in a marketing context. Journal of Marketing Education, 25, 31–36. doi: 10.1177/0273475302250570
- Ball, D. L., Lubienski, S. T., & Mewborn, D. S. (2001). Research on teaching mathematics: The unsolved problem of teachers’ mathematical knowledge. Handbook of Research on Teaching, 4, 433–456.
- Ball, D. L., & McDiarmid, G. W. (1989). The subject matter preparation of teachers. Issue Paper 89–4. National Center for Research on Teacher Education, Michigan State University.
- Ball, D. L., Thames, M. H., & Phelps, G. (2008). Content knowledge for teaching: What makes it special? Journal of Teacher Education, 59, 389–407. doi: 10.1177/0022487108324554
- Baumert, J., & Kunter, M. (2006). Stichwort: Professionelle Kompetenz von Lehrkräften [Teachers’ professional competence]. Zeitschrift für Erziehungswissenschaft, 9(4), 469–520. doi: 10.1007/s11618-006-0165-2
- Baumert, J., Kunter, M., Blum, W., Brunner, M., Voss, T., Jordan, A., … Tsai, Y. M. (2010). Teachers’ mathematical knowledge, cognitive activation in the classroom, and student progress. American Educational Research Journal, 47(1), 133–180. doi: 10.3102/0002831209345157
- Bennett, R. E., Rock, D. A., & Wang, M. (1991). Equivalence of free-response and multiple-choice items. Journal of Educational Measurement, 28, 77–92. doi: 10.1111/j.1745-3984.1991.tb00345.x
- Boulanger, F. D. (1981). Ability and science learning: A quantitative synthesis. Journal of Research in Science Teaching, 18(2), 113–121. doi: 10.1002/tea.3660180203
- Brandstädter, K., Harms, U., & Großschedl, J. (2012). Assessing system thinking through different concept-mapping practices. International Journal of Science Education, 34(14), 2147–2170. doi: 10.1080/09500693.2012.716549
- Brunner, M., Kunter, M., Krauss, S., Baumert, J., Blum, W., Dubberke, T., … Neubrand, M. (2006). Welche Zusammenhänge bestehen zwischen dem fachspezifischen Professionswissen von Mathematiklehrkräften und ihrer Ausbildung sowie beruflichen Fortbildung? [How is content specific professional knowledge of mathematics teachers related to their teacher education and in-service training?]. Zeitschrift für Erziehungswissenschaft, 9, 521–544. doi: 10.1007/s11618-006-0166-1
- Carlisle, J. F., Correnti, R., Phelps, G., & Zeng, J. (2009). Exploration of the contribution of teachers’ knowledge about reading to their students’ improvement in reading. Reading and Writing, 22(4), 457–486. doi: 10.1007/s11145-009-9165-y
- Clermont, C. P., Krajcik, J. S., & Borko, H. (1993). The influence of an intensive in-service workshop on pedagogical content knowledge growth among novice chemical demonstrators. Journal of Research in Science Teaching, 30, 21–43. doi: 10.1002/tea.3660300104
- Cochran, K. F., & Jones, L. L. (1998). The subject matter knowledge of preservice science teachers. In B. J. Fraser, & K. G. Tobin (Eds.), International handbook of science education. Part two (pp. 707–718). London: Kluwer Academic.
- Cochran-Smith, M., & Zeichner, K. M. (2005). Studying teacher education: The report of the AERA panel on research and teacher education. Copenhagen: Lawrence Erlbaum Associates.
- Cohen, D. K., Raudenbush, S. W., & Ball, D. L. (2003). Resources, instruction, and research. Educational Evaluation and Policy Analysis, 25(2), 119–142. doi: 10.3102/01623737025002119
- Cortina, K. S., & Thames, M. H. (2013). Teacher education in Germany. In M. Kunter, J. Baumert, W. Blum, U. Klusmann, S. Krauss, & M. Neubrand (Eds.), Cognitive activation in the mathematics classroom and professional competence of teachers (pp. 49–62). New York, NY: Springer.
- Darling-Hammond, L. (2000a). How teacher education matters. Journal of Teacher Education, 51(3), 166–173. doi: 10.1177/0022487100051003002
- Darling-Hammond, L. (2000b). Teacher quality and student achievement. Education Policy Analysis Archives, 8(1), 1–44. doi: 10.14507/epaa.v8n1.2000
- Davis, J. D. (2009). Understanding the influence of two mathematics textbooks on prospective secondary teachers’ knowledge. Journal of Mathematics Teacher Education, 12, 365–389. doi: 10.1007/s10857-009-9115-2
- Depaepe, F., Verschaffel, L., & Kelchtermans, G. (2013). Pedagogical content knowledge: A systematic review of the way in which the concept has pervaded mathematics educational research. Teaching and Teacher Education, 34, 12–25. doi: 10.1016/j.tate.2013.03.001
- Department for Education [DfE]. (2013). Science programmes of study: key stage 3. Retrieved June 7, 2016, from https://www.gov.uk/government/uploads/system/uploads/attachment_data/file/335174/SECONDARY_national_curriculum_-_Science_220714.pdf
- Eckert, A. (1998). Die ‘Netzwerk Elaborierungs Technik (NET)’ – Ein computergestütztes wissensdiagnostisches Instrumentarium [‘The network elaboration technique (NET)’ – A computer-based diagnostic instrument]. Diagnostica, 44(4), 220–224.
- Evagorou, M., Korfiatis, K., Nicolaou, C., & Constantinou, C. (2009). An investigation of the potential of interactive simulations for developing system thinking skills in elementary school: A case study with fifth-graders and sixth-graders. International Journal of Science Education, 31(5), 655–674. doi: 10.1080/09500690701749313
- Evertson, C. M., Hawley, W. D., & Zlotnik, M. (1985). Making a difference in educational quality through teacher education. Journal of Teacher Education, 36(3), 2–12. doi: 10.1177/002248718503600302
- Fennema, E., Carpenter, T. P., Franke, M. L., Levi, L., Jacobs, V. R., & Empson, S. B. (1996). A longitudinal study of learning to use children’s thinking in mathematics instruction. Journal for Research in Mathematics Education, 27(4), 403–434. doi: 10.2307/749875
- Fraune, K. (2014). Modeling system thinking: Assessment, structure, validation, and development (Doctoral dissertation). Christian-Albrechts-University of Kiel, Kiel.
- Großschedl, J., & Harms, U. (2013). Assessing conceptual knowledge using similarity judgments. Studies in Educational Evaluation, 39, 71–81. doi: 10.1016/j.stueduc.2012.10.005
- Großschedl, J., Harms, U., Kleickmann, T., & Glowinski, I. (2015). Preservice biology teachers’ professional knowledge: Structure and learning opportunities. Journal of Science Teacher Education, 26(3), 291–318. doi: 10.1007/s10972-015-9423-6
- Großschedl, J., Mahler, D., Kleickmann, T., & Harms, U. (2014). Content-related knowledge of biology teachers from secondary schools: Structure and learning opportunities. International Journal of Science Education, 36(14), 2335–2366. doi: 10.1080/09500693.2014.923949
- Grossman, P. (1990). The making of a teacher: Teacher knowledge and teacher education. New York, NY: Teachers College Press.
- Hannon, B., & Ruth, M. (2000). Dynamic modeling (2nd ed.). New York, NY: Springer.
- Hashweh, M. Z. (1987). Effects of subject-matter knowledge in the teaching of biology and physics. Teaching and Teacher Education, 3(2), 109–120. doi: 10.1016/0742-051X(87)90012-6
- Hattie, J. A. C. (2009). Visible learning: A synthesis of 800 meta-analyses on achievement. Abingdon: Routledge.
- Hattie, J. A., & Hansford, B. C. (1982). The relationship between self and achievement/performance measures. Review of Educational Research, 52(1), 123–142. doi: 10.3102/00346543052001123
- Heller, K. A., & Perleth, C. (2000). Kognitiver Fähigkeitstest für 4. bis 12. Klassen, Revision: KFT 4–12+ R [ Cognitive abilities test for 4th to 12th grade students]. Weinheim: Beltz-Test.
- Hill, H. C., Ball, D. L., Blunk, M., Goffney, I. M., & Rowan, B. (2007). Validating the ecological assumption: The relationship of measure scores to classroom teaching and student learning. Measurement: Interdisciplinary Research and Perspectives, 5(2–3), 107–118.
- Hill, H. C., Rowan, B., & Ball, D. L. (2005). Effects of teachers’ mathematical knowledge for teaching on student achievement. American Educational Research Journal, 42(2), 371–406. doi: 10.3102/00028312042002371
- Hill, H. C., Schilling, S. G., & Ball, D. L. (2004). Developing measures of teachers’ mathematics knowledge for teaching. The Elementary School Journal, 105, 11–30. doi: 10.1086/428763
- Jüttner, M., Boone, W., Park, S., & Neuhaus, B. J. (2013). Development and use of a test instrument to measure biology teachers’ content knowledge (CK) and pedagogical content knowledge (PCK). Educational Assessment, Evaluation and Accountability, 25(1), 45–67. doi: 10.1007/s11092-013-9157-y
- Jüttner, M., & Neuhaus, B. J. (2013). Validation of a paper-and-pencil test instrument measuring biology teachers’ pedagogical content knowledge by using think-aloud interviews. Journal of Education and Training Studies, 1(2), 113–125. doi: 10.11114/jets.v1i2.126
- Kleickmann, T., & Anders, Y. (2013). Learning at university. In M. Kunter, J. Baumert, W. Blum, U. Klusmann, S. Krauss, & M. Neubrand (Eds.), Cognitive activation in the mathematics classroom and professional competence of teachers: Results from the COACTIV project (pp. 321–332). New York, NY: Springer.
- Krauss, S., Brunner, M., Kunter, M., Baumert, J., Blum, W., Neubrand, M., & Jordan, A. (2008). Pedagogical content knowledge and content knowledge of secondary mathematics teachers. Journal of Educational Psychology, 100(3), 716-725. doi: 10.1037/0022-0663.100.3.716
- Kunter, M., Klusmann, U., Baumert, J., Richter, D., Voss, T., & Hachfeld, A. (2013). Professional competence of teachers: Effects on instructional quality and student development. Journal of Educational Psychology, 105(3), 805–820. doi: 10.1037/a0032583
- Lange, K., Ohle, A., Kleickmann, T., Kauertz, A., Möller, K., & Fischer, H. (2015). Zur Bedeutung von Fachwissen und fachdidaktischem Wissen für Lernfortschritte von Grundschülerinnen und Grundschülern im naturwissenschaftlichen Sachunterricht [The meaning of content knowledge and pedagogical content knowledge for primary students’ science performance]. Zeitschrift für Grundschulforschung, 8(1), 23–38.
- Lee, E., & Luft, J. A. (2008). Experienced secondary science teachers’ representation of pedagogical content knowledge. International Journal of Science Education, 30(10), 1343–1363. doi: 10.1080/09500690802187058
- Leinhardt, G., & Smith, D. A. (1985). Expertise in mathematics instruction: Subject matter knowledge. Journal of Educational Psychology, 77(3), 247–271. doi: 10.1037/0022-0663.77.3.247
- Lipowsky, F. (2006). Auf den Lehrer kommt es an. Empirische Evidenzen für Zusammenhänge zwischen Lehrerkompetenzen, Lehrerhandeln und dem Lernen der Schüler [The teacher matters. Empirical evidence for the relationship between teachers’ competences, teaching, and learning]. Zeitschrift für Pädagogik, 51, 47–70.
- Lipton, A., & Huxham, G. J. (1970). Comparison of multiple-choice and essay testing in preclinical physiology. British Journal of Medical Education, 4, 228–238. doi: 10.1111/j.1365-2923.1970.tb01629.x
- Loughran, J., Milroy, P., Berry, A., Gunstone, R., & Mulhall, P. (2001). Documenting science teachers’ pedagogical content knowledge through PaP-eRs. Research in Science Education, 31, 289–307. doi: 10.1023/A:1013124409567
- Ma, L. (1999). Knowing and teaching elementary mathematics: Teachers’ understanding of fundamental mathematics in China and the United States. Hillsdale, NJ: Erlbaum.
- Magnusson, S., Krajcik, J. S., & Borko, H. (1999). Nature, sources and development of pedagogical content knowledge for science teaching. In J. Gess-Newsome, & N. G. Lederman (Eds.), Examining pedagogical content knowledge (pp. 95–132). Dordrecht: Kluwer Academic.
- Mahler, D., Großschedl, J., & Harms, U. (submitted for publication). Teachers’ self-efficacy and enthusiasm: Opportunities for teachers to develop motivational orientations.
- Marsh, H. W., Lüdtke, O., Robitzsch, A., Trautwein, U., Asparouhov, T., Muthén, B., & Nagengast, B. (2009). Doubly-latent models of school contextual effects: Integrating multilevel and structural equation approaches to control measurement and sampling error. Multivariate Behavioral Research, 44(6), 764–802. doi: 10.1080/00273170903333665
- Muthén, L. K., & Muthén, B. O. (2007). MPlus (Version 5.21) [ComputerSoftware]. Los Angeles, CA: Author.
- National Science Teachers Association [NSTA]. (2012). Preservice science standards. Retrieved June 7, 2016, from http://www.nsta.org/preservice/docs/2012NSTAPreserviceScienceStandards.pdf
- Next Generation Science Standards [NGSS]. (2013). DCI arrangements of the next generation science standards. Retrieved June 7, 2016, from www.nextgenscience.org/sites/ngss/files/NGSS20DCI20Combined%2011.6.13.pdf
- Nezlek, J. B., Schröder-Abé, M., & Schütz, A. (2006). Mehrebenenanalysen in der psychologischen Forschung: Vorteile und Möglichkeiten der Mehrebenenmodellierung mit Zufallskoeffizienten [Multilevel analysis in psychological research: Advantages and possibilities of multilevel modelling with random coefficients]. Psychologische Rundschau, 57(4), 213–223. doi: 10.1026/0033-3042.57.4.213
- Ohle, A., Fischer, H. E., & Kauertz, A. (2011). Der Einfluss des physikalischen Fachwissens von Primarstufenlehrkräften auf Unterrichtsgestaltung und Schülerleistung [The meaning of physics-related content knowledge of primary science teachers for teaching and student performance ]. Zeitschrift für Didaktik der Naturwissenschaften, 17, 357–389.
- Organisation for Economic Cooperation and Development [OECD]. (2013). PISA 2012 results in focus: What 15-year-olds know and what they can do with what they know. Paris: OECD.
- Park, S., & Chen, Y.-C. (2012). Mapping out the integration of the components of pedagogical content knowledge (PCK): Examples from high school biology classrooms. Journal of Research in Science Teaching, 49(7), 922–941. doi: 10.1002/tea.21022
- Park, S., & Oliver, J. S. (2008). Revisiting the conceptualization of pedagogical content knowledge (PCK): PCK as a conceptual tool to understand teachers as professionals. Research in Science Education, 38(3), 261–284. doi: 10.1007/s11165-007-9049-6
- Peng, A. (2007). Knowledge growth of mathematics teachers during professional activity based on the task of lesson explaining. Journal of Mathematics Teacher Education, 10, 289–299. doi: 10.1007/s10857-007-9041-0
- Peugh, J. L., & Enders, C. K. (2004). Missing data in educational research: A review of reporting practices and suggestions for improvement. Review of Educational Research, 74(4), 525–556. doi: 10.3102/00346543074004525
- Piburn, M. D. (1993). Evidence from meta-analysis for an expertise model of achievement in science. Paper presented at the annual meeting of the National Association for Research in Science Teaching, Atlanta, GA.
- Rempfler, A., & Uphues, R. (2012). System competence in geography education development of competence models, diagnosing pupils’ achievement. European Journal of Geography, 3(1), 6–22.
- Riese, J., & Reinhold, P. (2012). Die professionelle Kompetenz angehender Physiklehrkräfte in verschiedenen Ausbildungsformen. Zeitschrift für Erziehungswissenschaft, 15(1), 111–143. doi: 10.1007/s11618-012-0259-y
- Riess, W., & Mischo, C. (2010). Promoting systems thinking through biology lessons. International Journal of Science Education, 32(6), 705–725. doi: 10.1080/09500690902769946
- Rivkin, S. G., Hanushek, E. A., & Kain, J. F. (2005). Teachers, schools, and academic achievement. Econometrica, 73(2), 417–458. doi: 10.1111/j.1468-0262.2005.00584.x
- Rowan, B., Chiang, F. S., & Miller, R. J. (1997). Using research on employees’ performance to study the effects of teachers on students’ achievement. Sociology of Education, 70, 256–284. doi: 10.2307/2673267
- Rozenszajn, R., & Yarden, A. (2014). Expansion of biology teachers’ pedagogical content knowledge (PCK) during a long-term professional development program. Research in Science Education, 44(1), 189–213. doi: 10.1007/s11165-013-9378-6
- Rubin, D. B. (1976). Multivariate matching methods that are equal percent bias reducing, II: Maximums on bias reduction for fixed sample sizes. Biometrics, 32(1), 121–132. doi: 10.2307/2529343
- Ruiz-Primo, M. A., & Shavelson, R. J. (1996). Problems and issues in the use of concept maps in science assessment. Journal of Research in Science Teaching, 33(6), 569–600. doi: 10.1002/(SICI)1098-2736(199608)33:6<569::AID-TEA1>3.0.CO;2-M
- Sadler, P. M., Sonnert, G., Coyle, H. P., Cook-Smith, N., & Miller, J. L. (2013). The influence of teachers’ knowledge on student learning in middle school physical science classrooms. American Educational Research Journal, 50(5), 1020–1049. doi: 10.3102/0002831213477680
- Schiepe-Tiska, A., Schöps, K., Rönnebeck, S., Köller, O., & Prenzel, M. (2013). Naturwissenschaftliche Kompetenz in PISA 2012: Ergebnisse und Herausforderungen. In M. Prenzel, C. Sälzer, E. Klieme, & O. Köller (Eds.), PISA 2012. Fortschritte und Herausforderungen (pp. 189–216). New York, NY: Waxmann.
- Schmelzing, S., van Driel, J. H., Jüttner, M., Brandenbusch, S., Sandmann, A., & Neuhaus, B. J. (2013). Development, evalutation, and validation of a paper-and-pencil test for measuring two components of biology teachers’ pedgagogical content knowledge concerning the cardiovascular system. International Journal of Science and Mathematics Education, 11(6), 1369–1390. doi: 10.1007/s10763-012-9384-6
- Schmidt, W. H., Tatto, M. T., Bankov, K., Blömeke, S., Cedillo, T., Cogan, L., et al. (2007). The preparation gap: Teacher education for middle school mathematics in six countries. MT21 report. East Lansing, MI: MSU Center for Research in Mathematics and Science Education.
- Schoenfeld, A. H. (2007). The complexities of assessing teacher knowledge. Measurement: Interdisciplinary Research and Perspectives, 5, 198–204.
- Secretariat of the Standing Conference of the Ministers of Education and Cultural Affairs in the Federal Republic of Germany [KMK]. (2010). Bildungsstandards im Fach Biologie für den Mittleren Schulabschluss [National educational standards for the intermediate school leaving certificate in biology]. München: Luchterhand.
- Secretariat of the Standing Conference of the Ministers of Education and Cultural Affairs of the Länder in the Federal Republic of Germany [KMK]. (2005). Bildungsstandards der Kultusministerkonferenz. Erläuterungen zur Konzeption und Entwicklung [Educational standards of the KMK – Explanation of conception and development ]. Retrieved June 7, 2016, from http://www.kmk.org/fileadmin/Dateien/veroeffentlichungen_beschluesse/2004/2004_12_16-Bildungsstandards-Konzeption-Entwicklung.pdf
- Secretariat of the Standing Conference of the Ministers of Education and Cultural Affairs of the Länder in the Federal Republic of Germany [KMK]. (2008). Ländergemeinsame inhaltliche Anforderungen für die Fachwissenschaften und Fachdidaktiken in der Lehrerbildung [Content requirements for subject-related studies and subject-related didactics in teacher training which apply to all Länder]. Retrieved June 7, 2016, from http://www.kmk.org/fileadmin/veroeffentlichungen_beschluesse/2004/2004_12_16-Standards-Lehrerbildung.pdf
- Shulman, L. (1987). Knowledge and teaching: Foundations of the new reform. Harvard Educational Review, 57(1), 1–23. doi: 10.17763/haer.57.1.j463w79r56455411
- Shulman, L. S. (1986). Those who understand: Knowledge growth in teaching. Educational Researcher, 15(2), 4–14. doi: 10.3102/0013189X015002004
- Sommer, C., & Lücken, M. (2010). System competence – Are elementary students able to deal with a biological system? Nordic Studies in Science Education, 6(2), 125–143.
- Swiss Conference of Cantonal Directors of Education [EDK]. (2011). Grundkompetenzen für die Naturwissenschaften. Retrieved June 7, 2016, from http://edudoc.ch/record/96787/files/grundkomp_nawi_d.pdf
- Tatto, M. T., & Senk, S. (2011). The mathematics education of future primary and secondary teachers: Methods and findings from the teacher education and development study in mathematics. Journal of Teacher Education, 62, 121–137. doi: 10.1177/0022487110391807
- Tenorth, H. E. (2006). Professionalität im Lehrerberuf [Professionalism in the teaching profession]. Zeitschrift für Erziehungswissenschaft, 9(4), 580–597. doi: 10.1007/s11618-006-0169-y
- Van Driel, J. H., De Jong, O., & Verloop, N. (2002). The development of preservice chemistry teachers’ pedagogical content knowledge. Science Education, 86(4), 572–590. doi: 10.1002/sce.10010
- Van Driel, J. H., Verloop, N., & de Vos, W. (1998). Developing science teachers’ pedagogical content knowledge. Journal of Research in Science Teaching, 35(6), 673–695. doi: 10.1002/(SICI)1098-2736(199808)35:6<673::AID-TEA5>3.0.CO;2-J
- Verhoeff, R. P. (2003). Towards systems thinking in cell biology education (Doctoral dissertation). Utrecht: CD-b Press.
- Wainer, H., & Thissen, D. (1993). Combining multiple-choice and constructed response test scores: Toward a Marxist theory of test construction. Applied Measurement in Education, 6(2), 103–118. doi: 10.1207/s15324818ame0602_1
- Walstad, W., & Becker, W. (1994). Achievement differences on multiple-choice and essay tests in economics. American Economic Review, 84(2), 193–196.
- Warm, T. A. (1989). Weighted likelihood estimation of ability in item response theory. Psychometrika, 54, 427–450. doi: 10.1007/BF02294627
- Wilson, M., deBoeck, P., & Carstensen, C. (2008). Explanatory item response models: A brief introduction. In J. Hartig, E. Klieme, & D. Leutner (Eds.), Assessment of competencies in educational contexts: State of the art and future prospects (pp. 91–120). Göttingen: Hogefe & Huber.
- Wirtz, M. & Caspar, F. (2002). Beurteilerübereinstimmung und Beurteilerreliabilität [Intercoder aggreement and intercoder reliability]. Göttingen: Hogrefe.
- Wu, M. L., Adams, R. J., Wilson, M. R., & Haldane, S. A. (2007). ACER ConQuest (Version 2.0). Camberwell: ACER Press, Australian Council for Educational Research.
- Yin, Y., Vanides, J., Ruiz-Primo, M. A., Ayala, C. C., & Shavelson, R. J. (2005). Comparison of two concept-mapping techniques: Implications for scoring, interpretation, and use. Journal of Research in Science Teaching, 42(2), 166–184. doi: 10.1002/tea.20049
Appendix. Item examples
Teacher level (Großschedl et al., Citation2014)
Content knowledge (CK)
Which of the following statements relating to the shell structure of molluscs are correct?
□ The shells of molluscs are structured in three layers.
□ The shells of molluscs consist of a dense epithelial layer.
□ The shells of molluscs consist of an organic lipid layer and an inner nacre layer.
□ The shells of molluscs contain an organic glycoprotein layer, an outer prism layer, and an inner nacre layer.
Pedagogical content knowledge (PCK)
In the lesson before, 8th-grade students have learned that blue mussels need water to be able to breathe.
Please create a short outline for a problem-oriented beginning of a lesson with the topic ‘survival of the blue mussels during low tide’.
Curricular knowledge (CuK)
In the national educational standards for the intermediate school-leaving certificate in biology (KMK, Citation2010), three basic concepts indicate the substance dimension of the competences.
Please name the three basic concepts.
Student level (Brandstädter et al., Citation2012)
Structural system thinking
Why do mussels filtrate sea water?
□ to clean their bodies
□ to breathe
□ to filtrate food
□ to move
Procedural system thinking
Oysters do not feed on mussels and do not kill them. Why are they still so threat-generating for mussels?