ABSTRACT
This study investigates how individual differences in 7- to 9-year-olds' curiosity relate to the inquiry-learning process and outcomes in environments differing in structure. The focus on curiosity as individual differences variable was motivated by the importance of curiosity in science education, and uncertainty being central to both the definition of curiosity and the inquiry-learning environment. Curiosity was assessed with the Underwater Exploration game (Jirout, J., & Klahr, D. (2012). Children's scientific curiosity: In search of an operational definition of an elusive concept. Developmental Review, 32, 125–160. doi:10.1016/j.dr.2012.04.002), and inquiry-based learning with the newly developed Scientific Discovery task, which focuses on the principle of designing informative experiments. Structure of the inquiry-learning environment was manipulated by explaining this principle or not. As intelligence relates to learning and possibly curiosity, it was taken into account. Results showed that children's curiosity was positively related to their knowledge acquisition, but not to their quality of exploration. For low intelligent children, environment structure positively affected their quality of exploration, but not their knowledge acquisition. There was no interaction between curiosity and environment structure. These results support the existence of two distinct inquiry-based learning processes – the designing of experiments, on the one hand, and the reflection on performed experiments, on the other – and link children's curiosity to the latter process.
1. Introduction
1.1. Individual differences and inquiry-based learning
Over the last decades, inquiry-based learning, also called discovery learning, has become a popular approach in science education policy and practice (De Jong, Citation2006; National Research Council, Citation2001; Osborne & Dillon, Citation2008). Even though the term ‘inquiry-based learning’ has been used to describe a wide range of interventions and consensus on a single definition is lacking, there is general agreement on the inductive, constructivist nature of the approach (Alfieri, Brooks, Aldrich, & Tenenbaum, Citation2011; Furtak, Seidel, Iverson, & Briggs, Citation2012; Klahr, Zimmerman, & Jirout, Citation2011; Zimmerman, Citation2007). In the approach, the child constructs its own knowledge by performing investigations in an information-rich environment (Alfieri et al., Citation2011; De Jong, Citation2006; Kirschner, Sweller, & Clark, Citation2006), often receiving some type of adult assistance in structuring this environment (Alfieri et al., Citation2011; Furtak et al., Citation2012).
A defining characteristic of inquiry-based learning is that it generates a high amount of uncertainty. This uncertainty is caused by the use of a complex environment in combination with the learner being given the freedom to determine her own strategy in investigating this environment. The environment generally mimics a real-world situation and offers not one but multiple variables to explore. The child is not given a fixed recipe to follow, but generally determines herself the type, number, and sequence of experiments. As a result of this uncertainty, individual differences between children, such as in their personality, prior knowledge, and motivation, are expected to result in a large variation in behaviour. That is, children will experiment and learn differently. Therefore, it is of crucial importance to further investigate individual differences in inquiry-based learning (De Jong, Citation2006).
A body of work on aptitude–treatment interactions has looked at how individual differences are related to children’s inquiry-based learning in environments with different degrees of structure (for reviews, see Clark, Citation1989; Cronbach & Snow, 1977 as cited in Kirschner et al., Citation2006; Kyllonen & Lajoie, Citation2003; Snow & Lohman, Citation1984). These studies examined individual differences in terms of learners’ personality (e.g. Leith, 1974 as cited in Eysenck, Citation1996; Leutner, Citation1993; Rowell & Renner, Citation1975; Shadbolt, Citation1978; Trown & Leith, Citation1975), prior knowledge and intelligence (e.g. Gijlers & De Jong, Citation2005; Snow & Lohman, Citation1984; Tuovinen & Sweller, Citation1999), and motivation (e.g. Hakkarainen, Lipponen, Järvelä, & Niemivirta, Citation1999; Veermans & Järvelä, Citation2004). In line with uncertainty being at the core of inquiry-based learning, a large part of these studies has been directed at investigating whether reducing uncertainty by providing structure to the environment selectively benefits students. In these studies, more unstructured, inductive approaches were compared with more structured, deductive approaches. Unstructured approaches for example included children participating in free inquiry-based learning with a low degree of teacher guidance, while structured approaches included children participating in inquiry-based learning with a high degree of teacher guidance, such as direct instruction or worked examples (Sweller & Cooper, Citation1985). Main findings are that introvert learners, high anxious learners, low intelligent learners, and learners with less prior knowledge tend to profit more from structured approaches, while extravert learners, low anxious learners, high intelligent learners, and learners with more prior knowledge tend to profit more from unstructured approaches (e.g. Leith, 1974 as cited in Eysenck, Citation1996; Rowell & Renner, Citation1975; Shadbolt, Citation1978; Snow & Lohman, Citation1984; Trown & Leith, Citation1975; Tuovinen & Sweller, Citation1999), although some studies also found opposite results (e.g. Leutner, Citation1993). Further work has looked at individual differences in terms of aptitude complexes, that is, combinations of learner characteristics that predict efficient learning (for an overview, see Ackerman, Citation2003).
The aptitude–treatment interaction studies have in common that they predominantly concern children of 10 years and older or adults (all above-mentioned studies), and computer-supported learning environments (Gijlers & De Jong, Citation2005; Hakkarainen et al., Citation1999; Leutner, Citation1993; Tuovinen & Sweller, Citation1999; Veermans & Järvelä, Citation2004). In addition, these studies often use outcome-based measures as opposed to process-based measures of inquiry-based learning, such as learners’ knowledge gains, delayed knowledge, and transfer of knowledge (Leutner, Citation1993; Rowell & Renner, Citation1975; Trown & Leith, Citation1975; Tuovinen & Sweller, Citation1999). The focus of these studies is in contrast with the noticed importance of hands-on experiences and skill learning in science education practices, and this especially being the case for the younger age group (Allen, Citation2004; Gelman & Brenneman, Citation2004; Kuhn, Citation2007; Osborne & Dillon, Citation2008; Stohr-Hunt, Citation1996). Even though there is a body of research addressing young children’s hands-on inquiry-based learning (e.g. Bonawitz, Van Schijndel, Friel, & Schulz, Citation2012; Chen & Klahr, Citation1999; Crowley et al., Citation2001; Klahr & Nigam, Citation2004; Peterson & French, Citation2008; Samarapungavan, Patrick, & Mantzicopoulos, Citation2011; Schauble, Citation1996; Van Schijndel, Franse, & and Raijmakers, Citation2010; Van Schijndel, Visser, van Bers, & Raijmakers, Citation2015), to our knowledge, few of these studies have investigated aptitude–treatment interactions (Arnone, Grabowski, & Rynd, Citation1994). Therefore, the present study addresses how individual differences are related to younger children’s hands-on inquiry-based learning in environments with different degrees of structure. To assess inquiry-learning performance, we will not only apply outcome-based measures (children’s knowledge acquisition) but also measures capturing the inquiry-learning process (quantity and quality of children’s exploration).
1.2. Curiosity and inquiry-based learning
In science education practice and policy, curiosity is considered an important factor in children’s inquiry-based learning (Glogger-Frey, Fleischer, Grüny, Kappich, & Renkl, Citation2015; Jirout & Klahr, Citation2012; Klahr et al., Citation2011; Svinicki, Citation1998; Van Uum, Verhoeff, & Peeters, Citation2017). Curiosity is considered to drive learning as studies show a positive relation between curiosity and emotion control, deliberation (Lauriola et al., Citation2015), effective learning strategies (Muis et al., Citation2015), and learning outcomes (Trevors, Muis, Pekrun, Sinatra, & Muijselaar, Citation2017).
Recent scientific work provides a theoretical account for the relation between inquiry-based learning and curiosity by showing that uncertainty is not only a defining characteristic of the inquiry-learning environment but that children’s preference for uncertainty is also central to the definition of curiosity (Jirout & Klahr, Citation2012). Based on an extensive literature review (e.g. Berlyne, Citation1960; Litman & Jimerson, Citation2004; Loewenstein, Citation1994), Jirout and Klahr (Citation2012, p.150) defined children’s curiosity as: ‘The threshold of desired uncertainty in the environment which leads to exploratory behavior’. The foundation for the work of Jirout and Klahr was the information gap theory of Loewenstein (Citation1994). This theory states that one is motivated to solve a problem when one feels deprived of knowledge or, in other words, experiences an information gap. Litman (Citation2005) uses the term Deprivation-type curiosity when referring to this motivational state.
Given the insights on uncertainty being at the core of both the inquiry-learning environment and the definition of curiosity, it seems logical to focus on investigating curiosity as an individual differences variable in inquiry-based learning. Although a single study in science education did show that an inventing activity (which is in line with an inquiry-based learning environment) can increase children’s curiosity (Glogger-Frey et al., Citation2015), the question remains whether inquiry-based learning benefits children of all initial levels of curiosity. Arnone et al. (Citation1994) did investigate this question in the field of art education. The researchers let first and second graders interact with a computer-based learner environment featuring art videos and compared more (e.g. advisement to stop and think) with less structured conditions in children who were classified into different levels of curiosity. They found high curious children to learn more arts facts and concepts from inquiry-based learning than low curious children, but they did not find effects of condition nor an interaction between curiosity and condition (aptitude–treatment interaction). The researchers explained these findings by the fact that the learning environment was very different from normal classroom routine, which might have reduced differences between conditions. However, to the best of our knowledge, as yet no studies have investigated the relation between children’s curiosity and inquiry-based learning in the field of science education. This is the focus of the present study.
1.3. The present study
The present study investigates how individual differences in 7- to 9-year-olds’ curiosity are related to the inquiry-learning process and outcomes, in environments with different degrees of structure. Importantly, intelligence may also be a predictor of success in inquiry-based learning as problem-solving skills and intelligence are related, though not perfectly (Veenman & Spaans, Citation2005). Also, there may be a relation between curiosity and intelligence as the literature is inconclusive on this subject: some studies demonstrate a positive relation (e.g. Alberti & Witryol, Citation1994), while others show an absence of such a relation (e.g. Jirout & Klahr, Citation2012). In the present study, children’s intelligence is therefore taken into account in examining the relation between individual differences in curiosity and inquiry-learning performance.
Children’s Deprivation-type curiosity was measured with the Underwater Exploration Game (Jirout & Klahr, Citation2012). In contrast to curiosity instruments for adults where participants are usually asked to report knowledge deprivation (Litman, Citation2005; Loewenstein, Citation1994), knowledge deprivation is manipulated directly with this instrument. In the task, children are confronted with various levels of uncertainty after exploration. At the most certain level, children know exactly which information will follow after exploration, so there is no information gap. At the most uncertain level, information is minimal after exploration and there is a large information gap. The instrument assesses children’s preference for uncertainty, that is, for small or large information gaps. The Underwater Exploration Game has shown to be a reliable instrument for assessing individual differences in children’s curiosity. Correlations with convergent and divergent measures were in the expected direction, supporting the validity of the measure (Jirout & Klahr, Citation2012).
Children’s inquiry-based learning in the field of science education was measured with the Scientific Discovery task, which was developed within the context of the present study to allow for the inclusion of a relatively young age group, and to capture both inquiry-learning outcomes and process. In line with many inquiry-learning tasks in the field of science education (e.g. Dean & Kuhn, Citation2007; Klahr & Nigam, Citation2004), the task centres on the skill of designing unconfounded, informative experiments, that is experiments from which valid causal conclusions can be drawn. This skill is also called the Control of Variables Strategy, and it is at the core of children’s scientific reasoning (Chen & Klahr, Citation1999; Klahr & Nigam, Citation2004). The set-up of the Scientific Discovery task consists of a balance scale on which transparent balls containing coloured blocks can be weighed (see ). The child is given a set of balls, each of which contains three coloured blocks (red and/or green and/or blue), and is asked to figure out which colour block is the lightest and which colour block is the heaviest. To make the task suitable for a relatively young age group, the skill of designing informative experiments is stripped down to its basics in the task (see Method section), and a memory tool is provided to help children memorise the outcomes of consecutive experiments. To distinguish between inquiry-learning outcomes and process, outcomes are assessed by a post-test measuring children’s knowledge of the relative weights of the balls, while the process is assessed by the quality and quantity of children’s exploration. Two versions of the Scientific Discovery task were used, differing in the structure of the inquiry-learning environment. In the high structure condition (HSC), children were explained the principle of performing informative experiments by the use of examples, while in the low structure condition (LSC) they were not. To allow for uninterfered measurement of the quantity of children’s exploration, the explanation took place before the start of the exploration phase.
We hypothesise children’s curiosity to be positively related to the quantity of their exploration during inquiry-based learning, because curiosity is associated with a drive or motivation to explore (Bijvoet-van den Berg & Hoicka, Citation2014; Endsley, Hutcherson, Garner, & Martin, Citation1979; Jirout & Klahr, Citation2012). As a result of the hypothesised prolonged exploration, children’s curiosity is also expected to be positively related to their knowledge acquisition. As the structure manipulation in the Scientific Discovery task involves an explanation of the principle of designing informative experiments, we expect the quality of children’s exploration and, as a result of that, their knowledge acquisition, to be higher in the HSC than in the LSC. We expect that reducing uncertainty by providing structure will selectively benefit students. The structure will take away some of the uncertainty of the inquiry-learning environment, and therefore we expect low curious children to profit more from the provided structure than high curious children. We will investigate these hypotheses taking into account children’s intelligence.
2. Method
2.1. Participants
The final sample consisted of 139 children (77 girls and 62 boys): 77 from grade 2 (M = 95.35 months, SD = 4.90) and 62 from grade 3 (M = 105.76 months, SD = 5.83) who were recruited from four primary schools in Amsterdam, Netherlands. Thirty-nine other children were recruited, but excluded from the analyses due to experimental error (N = 16) or non-attendance of school at test days leading to one or more tasks not being administered (N = 23).
2.2. Procedure
All children were administered the Scientific Discovery task (inquiry-based learning), Raven’s (Citation1965) Coloured Progressive Matrices (RCPM; intelligence), and the Underwater Exploration game (Jirout & Klahr, Citation2012; curiosity). Children were randomly assigned to the HSC or the LSC of the Scientific Discovery task, stratified by grade and sex.
The tests were administered in three fixed-order sessions. In the first session, children performed the Scientific Discovery task. This session was individual, took place outside the classroom in a quiet area of the child’s school, and lasted 15 minutes. In the second session, children performed the RCPM, as well as a number of other measures that are beyond the scope of this paper. This session took place in the child’s classroom and lasted 45 minutes, of which the first 10 were for the RCPM. In the third session, children performed the Underwater Exploration game. This session took place in a small group (each child using its own computer) outside the classroom in a quiet area of the child’s school, and lasted 15 minutes. The sessions were performed within a timeframe of one and a half month. As the tasks were substantially different from each other and were administered in separate sessions, we did not expect the fixed order of task administration to affect the results.
2.3. Materials
2.3.1. Scientific discovery task
The set-up of the task consists of a balance scale on which transparent balls containing coloured blocks can be weighed (see ). The core of the task consists of the child being given a set of balls, each of which contains three coloured blocks (red and/or green and/or blue), and being asked to figure out which colour block is the lightest and which colour block is the heaviest. Contrary to the large majority of tasks assessing the skill of designing informative experiments (e.g. Dean & Kuhn, Citation2007; Klahr & Nigam, Citation2004), the Scientific Discovery task only has one type of variable (colour), with three levels (red, blue, and green).
All children were first given an introduction to the task, after which they participated in an exploration- and post-test phase. Children in the HSC were additionally exposed to the structure manipulation which took place between the introduction phase and the exploration phase.
2.3.1.1. Introduction phase
The test leader explained the working of the balance scale. To this end, she used a set of three balls containing single white or brown blocks (relative weights white 2:1 brown). She hung a ball with a white block on one side of the scale and a ball with a brown block on the other side, and explained that the side with the ball containing the white block going down implied that the white block was heavier than the brown block. She demonstrated the workings of a simple memory tool. This tool consisted of a strip of paper with a ‘heavy’ symbol on one side and a ‘light’ symbol on the other side, and white and brown squares of paper. Putting the white square at the ‘heavy’ side and the brown square at the ‘light’ side of the strip could be of help in remembering the results of the experiment. Next, she hung a ball containing a white block on each side of the scale and explained that the scale being in balance implied that the white blocks had the same weight. She emphasised that blocks of the same colour always had the same weight.
2.3.1.2. Exploration phase
The child was given a set of eight balls, each of which contained three coloured blocks (red and/or green and/or blue, relative weights 1:2:3; see ). The test leader explained that the blocks were stuck in the balls and could not come out, and asked the child to figure out which colour block was the lightest and which colour block was the heaviest. Children also received a memory tool similar to the one demonstrated during the introduction phase. Recording of time started after the test leader gave a start sign (‘You can start now!’), and the exploration phase was ended after the child had played 4 minutes or when the child indicated that she was done exploring before that time. If she did so after having performed less than three experiments, she was encouraged to keep playing a bit longer. During this phase, the test leader noted the number of seconds the child played and the number of experiments she performed (quantity of exploration measures). After task administration, experiments were classified as being either unconfounded, informative or confounded, uninformative experiments. Experiments in which two balls were compared that were identical except for one colour change, such as red–blue–green versus red–blue–blue, were classified as informative. All other experiments, such as red–blue–blue versus red–red–green, were classified as uninformative (see ). Next, we calculated per child the number of performed unique informative experiments (0–3, comparing red–green, red–blue, or green–blue; quality of exploration measure).
The number of performed unique informative experiments, however, did not equal the units of information the child generated. Therefore, to check our choice for using this variable as a quality of exploration measure (see Results section), we calculated per child the number of generated units of information. A unit of information was defined as information on the rank order of two blocks. Depending on the order in which the child performed the experiments, a child could generate all three units of information (green > red, blue > red, blue > green) by either performing two or three unique informative experiments. For example, when a child first performed the experiment green–green–green versus green–red–red, she generated the information that green blocks are heavier than red blocks. When she subsequently performed the experiment red–blue–blue versus red–blue–green, she not only generated the information that blue blocks are heavier than green blocks but could also infer that blue blocks are heavier than red blocks. However, when, for example, a child chose to start with performing the informative experiment green–red–red versus red–blue–green, she would need three experiments to generate all necessary information to determine the rank order of the coloured blocks. Please note that generated information on a rank order does not equal knowledge on a rank order: a child might have generated information during play, but this does not mean that she knows the information. In other words, the number of generated units of information is considered a process measure (quality of exploration), while the knowledge on the rank orders of the blocks is considered an outcome measure (knowledge acquisition; see next paragraph).
2.3.1.3. Post-test phase
The test leader asked the child for the rank order of each possible pair of coloured blocks (red–green, red–blue, green–blue). Each time she asked: ‘Which block is heavier, this one or this one?’(knowledge acquisition measure; see ). A second set of questions was administered, concerning the relative weights of combinations of two balls. As these questions did not directly assess the child’s knowledge on the weight of the blocks, the answers were not included in the analyses.
2.3.1.4. Structure manipulation (HSC only)
The test leader explained the principle of performing unconfounded, informative experiments. To this end, she used a set of four balls, each of which contained three coloured blocks (pink and/or purple and/or yellow, relative weights 1:2:3). She first gave the example of an informative experiment (purple–purple–purple versus purple–pink–pink), and explained the general rules for recognising informative experiments:
You always compare two colors at the time; You then pick two balls; You check whether there are blocks of the same color in the two balls; If so, these blocks don’t count anymore; You then check whether the blocks that are left are of one color in one ball and of another color in the other ball; If so, you can put the balls on the scale and know which color block is the heaviest.
Next, the test leader discussed two additional examples, one of an uninformative experiment (purple–purple–purple versus yellow–yellow–pink) and one of an informative experiment (yellow–pink–yellow versus yellow–pink–purple). Finally, she repeated the general rules for recognising informative experiments (see above) ().
Figure 1. Scientific Discovery task.
Note: The photos were taken by VVBfoto during a day that the university was open to the public. The Scientific Discovery task that was used in this study is similar to the one that is shown on the photos, but the circumstances under which the task were administered are different: in the study, the task was administered individually outside the classroom in a quiet area of the child’s school, so no other children or adults were present. A colour version of this figure is available in the electronic version of the paper.
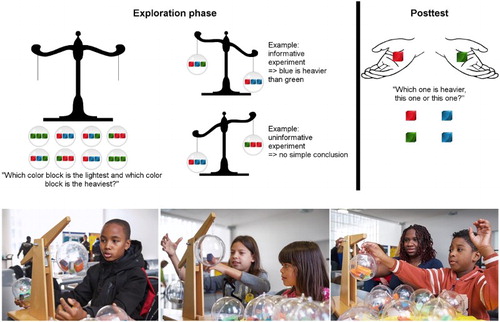
2.3.2. Raven’s Coloured Progressive Matrices
To assess children’s intelligence, we used Raven’s (Citation1965) Coloured Progressive Matrices. The RCPM consisted of 36 items, divided over three sets of 12 (A, AB, and B). The test is nonverbal in nature: for each item, the child was confronted with an incomplete picture and asked to select, from six options, the missing element that completes the picture. The test was printed in black and white and administered via paper and pencil. Children were given a maximum of 10 minutes to perform the test (Hamel & Schmittmann, Citation2006). Raw scores were standardised by grade, using Z-scores.
2.3.3. Underwater exploration game
To assess children’s curiosity, we translated Jirout and Klahr’s (Citation2012) Underwater Exploration game. The game was administered on a computer and consisted of 18 items. The child was told that she would see many different kinds of fish by looking out of the windows of a submarine. For each item, she was shown information at the sides of the screen about what might be outside each of two windows and was asked to open one of the windows. The information was varied to manipulate the level of uncertainty of the item: a minimal information gap was represented by one fish (the child knew the type of fish she would see when opening the window; see (A)), a medium information gap was represented by two to six fish (the child knew she would see one of the presented types of fish when opening the window; see (B)), and a maximum information gap was represented by a question mark (the child had no information on what type of fish she would see when opening the window; see (A)). The task was adaptive: it presented children with combinations of information gaps based on their choice of exploration at the previous three items (Jirout & Klahr, Citation2012). The child’s level of curiosity was calculated by taking the sum over items of the number of fish outside the chosen windows. This total uncertainty score was shown to have the greatest variability and precision by Jirout and Klahr.
Figure 2. Underwater Exploration game (Jirout & Klahr, Citation2012).
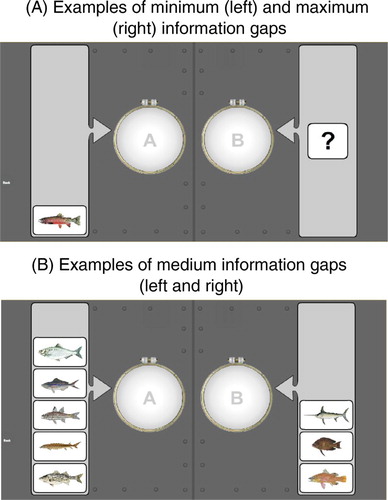
3. Results
3.1. General analyses
Prior to analysing the research questions, we examined whether the independent variables, curiosity and structure of the inquiry-learning environment, were related to children’s sex, grade, and school. Significant relations would be a reason to include sex, grade, or school variables in the main analyses.
For the analyses involving curiosity, we used non-parametric tests as the total uncertainty score was not distributed normally. Mann–Whitney tests showed that the total uncertainty score on the Underwater Exploration game did not differ for girls and boys, U = 2329.00, z = −.25, p = .81 (mean rank girls = 69.25, mean rank boys = 70.94), or for children from grade 2 and grade 3, U = 2281.00, z = −.45, p = .65 (mean rank grade 2 = 68.62, mean rank grade 3 = 71.71). A Kruskal–Wallis test showed that the total uncertainty score did not differ for the four schools, H(3) = 7.20, p = .07 (mean rank school 1 = 62.87, school 2 = 62.57, school 3 = 82.36, school 4 = 65.18).
Next, we checked whether the HSC and LSC of the Scientific Discovery task differed in terms of sex and grade distributions, and curiosity levels. Sixty-seven children were assigned to the HSC and 72 children to the LSC (see Procedure section). Chi-square tests showed that the conditions did not differ in proportions girls and boys, χ2(1) = .09, p = .76 (HSC: 38 girls and 29 boys, LSC: 39 girls and 33 boys), in proportions children from different grades, χ2(1) = .09, p = .76 (HSC: 38 children grade 2 and 29 children grade 3, LSC: 39 children grade 2 and 33 children grade 3), or in proportions children from different schools, χ2(3) = .26, p = .97, (HSC: 10 children school 1, 24 children school 2, 23 children school 3, 10 children school 4; LSC: 9 children school 1, 25 children school 2, 26 children school 3, 12 children school 4). A Mann–Whitney test showed that the conditions did not differ in average total uncertainty score on the Underwater Exploration game, U = 2356.00, z = −.24, p = .81 (mean rank HSC = 70.84, mean rank LSC = 69.22).
To conclude, the above analyses show that curiosity and structure of the inquiry-learning environment were unrelated to children’s sex, grade, and school, and we therefore did not include these variables in our main analyses (see next section). Supporting this choice was the fact that inquiry-learning performance did not differ for children from different sexes, grades, and schools. As the inquiry-learning performance variables were not distributed normally, we used non-parametric tests. Mann–Whitney tests showed that performance on the Scientific Discovery task did not differ for girls and boys: quantity of exploration in terms of number of played seconds, U = 2379.00, z = −.03, p = .97; quantity of exploration in terms of number of performed experiments, U = 2357.50, z = −.13, p = .90; quality of exploration, U = .2364.50, z = −.11, p = .92; learning, U = 2134.00, z = −1.21, p = .23 (see for the mean ranks). Mann–Whitney tests showed that performance on the Scientific Discovery task did not differ for children from grade 2 and 3: quantity of exploration in terms of number of played seconds, U = 2242.50, z = −.62, p = .53; quantity of exploration in terms of number of performed experiments, U = 2216.50, z = −.75, p = .45; quality of exploration, U = 2270.50, z = −.55, p = .58; learning, U = 2230.50, z = −.75, p = .46 (see for the mean ranks). Kruskal–Wallis tests showed that performance on the Scientific Discovery task did not differ between the four schools: quantity of exploration in terms of number of played seconds, H(3) = 6.45, p = .09; quantity of exploration in terms of number of performed experiments, H(3) = 5.54, p = .14; quality of exploration, H(3) = .19, p = .98; learning, H(3) = 3.57, p = .31 (see for the mean ranks).
Table 1. Mean ranks for performance on the Scientific Discovery task (quantity and quality of exploration, knowledge acquisition) by sex, grade, and school.
3.2. Curiosity and inquiry-based learning
To investigate how individual differences in curiosity were related to children’s inquiry-learning performance (process and outcomes) in environments with different degrees of structure, we conducted four multiple regression analyses while bootstrapping the confidence intervals of the regression coefficients (because of a non-normality of residuals; Field, Miles, & Field, Citation2012, p. 954): a set of multiple regression analyses with the two quantity of exploration measures (number of played seconds and number of performed experiments in Exploration phase of Scientific Discovery task) as dependent variables, a multiple regression analysis with the quality of exploration measure (number of performed unique informative experiments in Exploration phase of Scientific Discovery task) as a dependent variable, and a multiple regression analysis with the knowledge acquisition measure (number of correctly answered questions in Post-test phase of Scientific Discovery task) as a dependent variable. In all analyses, we included structure of the inquiry-learning environment (HSC and LSC Scientific Discovery task) as an independent variable, and curiosity (centred total uncertainty score Underwater Exploration game) and intelligence (centered standardised per grade RCPM score) as covariates (see Method section). All main effects and two-way interactions were included in the models, as these covered the scope of our hypotheses.
3.2.1. Quantity of exploration
A regression model with the quantity of children’s exploration in terms of the number of played seconds as dependent variable showed that the predictors explained a significant proportion of the variance, F(6,132) = 2.37, p = .033, R2 = .097. First, the structure of the inquiry-learning environment was related to the quantity of children’s exploration during inquiry-based learning, standardised β = −.20, p = .022; children in the HSC played longer during the Exploration phase than children in the LSC (HSC: M = 193.87, SD = 48.32, LSC: M = 173.32, SD = 56.41). Second, children’s curiosity was related to their quantity of exploration, standardised β = −.24, p = .038: the number of played seconds in the Exploration phase decreased with curiosity. A second regression model showed that the variance in the number of performed experiments was not explained significantly by the predictors in the regression model, F(5,133) = 1.87, p = .10.
3.2.2. Quality of exploration
A regression model with the number of performed unique informative experiments as dependent variable showed that the predictors explained a significant proportion of the variance, F(6,132) = 2.42, p = .030, R2 = .099. An interaction effect of structure of the inquiry-learning environment and intelligence on children’s quality of exploration during inquiry-based learning was found, β = −.40, p = .002: to illustrate the effect, it appears that among low intelligent children (median split), children in the HSC performed more unique informative experiments in the Exploration phase than children in the LSC, mean difference = −.44, bootstrapped p = .025, while among high intelligent children structure of the inquiry-learning environment had no effect on the performance of these type of experiments, mean difference = .04 (see ).
Figure 3. Quality of exploration: Average number of performed unique informative experiments by the structure of the inquiry-learning environment and intelligence.
Note: Error bars represent standard errors. Low (N = 74) and high (N = 65) intelligence groups were created by a median split. NB the median split was performed only for graphical clarity, in the analyses intelligence was included as a covariate.
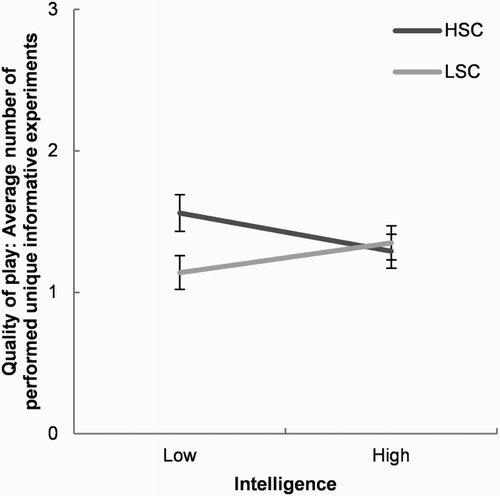
In the set-up of the Scientific Discovery task, performing unique informative experiments did not equal generating the necessary information to determine the rank order of the three coloured blocks, and therefore we repeated the analysis of variance with the number of generated units of information as an outcome variable (see Method section). This analysis rendered a similar interaction effect of structure of the inquiry-learning environment and intelligence on children’s quality of exploration, F(6,132) = 2.32, p = .037, R2 = .095, β = −.41, p = .005, in addition to an interaction between intelligence and curiosity, β = −.15, p = .04,
3.2.3. Knowledge acquisition
Before performing the regression analysis, we checked whether children in all conditions performed above chance level on the post-test (1.5). This was the case: HSC M = 2.37, p = .001, and LSC M = 2.53, p = .001. A regression model with the number of correctly answered questions as a dependent variable including all main and two-way interactions explained a significant amount of variance, F(6,132) = 3.48, p = 003, R2 = .14. However, the unique contribution of individual factors was not significant. A regression model with only main effects included also explained a significant amount of variance, F(3,135) = 6.60, p < .001, R2 = .13. Both children’s curiosity, β = .24, p = .002, and their intelligence, β = .20, p = .003, were found to be related to their knowledge acquisition from inquiry-based learning: the number of correctly answered questions in the Post-test phase increased with curiosity and with intelligence.
The correlations between curiosity and quantity of exploration and between curiosity and knowledge acquisition gave rise to a further investigation of the relations between the three variables. We conducted a mediation analysis with curiosity as the independent variable, quantity of exploration (number of played seconds in Exploration phase) as the mediating variable, and knowledge acquisition as the dependent variable. The total and direct effects were determined by performing a hierarchical regression analysis (Jansen et al., Citation2013), and the indirect effect was determined by using the method of Preacher and Hayes (Citation2004). In step 1 of a hierarchical regression analysis, we performed an analysis estimating the total effect of curiosity on knowledge acquisition. The results of step 1 were compared to the results of step 2, in which we performed a second analysis estimating the direct effect of curiosity on knowledge acquisition, after controlling for quantity of exploration. The explained variance of the regression model in step 1 was significant, R2 = .07, F(3, 137) = 10.54, p = .001. The inclusion of quantity of exploration as a predictor in step 2 explained a significant additional proportion of variance, ΔR2 = .08, ΔF(1, 136) = 12.09, p = .001. The bootstrap test of Preacher and Hayes (Citation2004) indicated that the indirect effect, similar to the difference between the total and the direct effect of curiosity, was estimated at .0017, and differed significantly from zero (bootstrapped 95% confidence interval ranged from .0002 to .044).
shows that the estimated beta parameter for curiosity was significant in the total effect model, and smaller but also significant in the direct effect model, signalling a positive relation between curiosity and knowledge acquisition. The significant parameter associated with the path from the independent variable to the mediating variable (estimated with a regression analysis with quantity of exploration as a dependent variable and curiosity as a predictor) signalled a negative relation between curiosity and quantity of exploration, B = −.516, SE B = .206, β = −.209, p = .014. The significant parameter associated with the path from the mediating variable to the dependent variable (estimated with a regression analysis with knowledge acquisition as a dependent variable and quantity of exploration as a predictor) signalled a negative relation between quantity of exploration and knowledge acquisition, B = −.004, SE B = .001, β = −.325, p < .001. Hence, these results suggest partial mediation of the relation between curiosity and knowledge acquisition by quantity of exploration: curiosity was associated with a lower quantity of play, which was associated with more learning.
Table 2. Results of hierarchical regression analyses estimating total and direct effects of curiosity on knowledge acquisition.
4. Discussion
The present study investigated how individual differences in 7- to 9-year-olds’ curiosity are related to the inquiry-learning process and outcomes, in environments with different degrees of structure. Below we will first discuss the results concerning individual differences in children’s curiosity, followed by the results concerning the effects of the manipulation of the structure of the inquiry-learning environment. Last, we will discuss the results concerning the interaction between curiosity and structure of the environment. Note that the effects we found were robust, but effect sizes were small.
In line with our hypotheses, children’s curiosity was found to be positively related to their knowledge acquisition: high curious children learned more from inquiry-based learning than low curious children. Importantly, even though children’s intelligence was also positively related to their knowledge acquisition, curiosity had an added positive effect on children’s learning. The relation between curiosity and knowledge acquisition was in part explained by the quantity of children’s exploration. Against our hypotheses, high curious children were found to play shorter, which was associated with more learning. Thus, being curious was not positively, but negatively associated with playtime, and it was not associated with the number of performed (unique informative) experiments. Nevertheless, in line with Arnone et al.'s (Citation1994) results, being curious was associated with more knowledge acquisition, and this effect could not be explained by intelligence. Even though the shorter play time for the high curious group might suggest that these results can be explained by this group being bored or uninterested in the inquiry-learning task, this is not the case as the number of performed informative experiments did not differ between the high and low curious group and the high curious group learned more than the low curious group. A possible explanation for these results is that high curious children’s preference for uncertainty motivated them to close the information gap (Jirout & Klahr, Citation2012; Piotrowski, Litman, & Valkenburg, Citation2014) by faster and better reflection on the experiments they performed. The distinction between exploration on the one hand and reflection and learning on the other hand has also been demonstrated in previous work on the topic of inquiry-based learning (e.g. Dunbar & Klahr, Citation1989; Schauble, Citation1990). This work showed differences between groups of participants in terms of the formulation of hypotheses and drawing of conclusions from experiments, but not in terms of the performance of experiments. For example, Dunbar and Klahr (Citation1989) compared 8- to 11-year-old’s performance to adults’ performance on a robot programming task. They found children’s learning to be limited compared to that of adults, but both groups performed similar experiments. Children, however, came up with less efficient hypotheses than adults and misevaluated evidence by ignoring negative evidence and only interpreting the results of their most recent experiment. We consider the hypothesis that high curious children’s motivation to close the information gap is shown in the efficiency of their reflection on experiments an interesting point of departure for future research.
Next, we investigated the effects of the manipulation of the structure of the inquiry-learning environment. In line with our hypothesis, we found the structure of the environment to positively affect the quality of children’s exploration, but this was only the case for the low intelligent children. The structure of the environment also positively affected the quantity of children’s exploration, but contrary to our expectations did not have an effect on children’s knowledge acquisition. Thus, adding structure to the environment led all children to play longer, without making more experiments. Adding structure to the environment also led low intelligent children to perform more unique informative experiments, but these changes did not result in more learning. Possibly, the rules for performing informative experiments that children were given in the high structure condition made them think longer during exploration on the procedure for performing experiments. The added structure might have made low intelligent children to understand the principle of performing informative experiments on a procedural level, but not on a conceptual level. That is, they learned to follow the rules or procedure for performing these types of experiments, but might not have understood the results of the experiments on a conceptual level.
Last, contrary to our expectations, individual differences in curiosity were not related to the extent to which children profited from the structure of the environment during inquiry-based learning. This result is in line with Arnone et al.’s (Citation1994) results, but contrasts with the results from aptitude–treatment interaction studies showing that reducing uncertainty selectively benefits introvert and high anxious learners and learners with less prior knowledge (e.g. Cronbach & Snow, 1977 as cited in Kirschner et al., Citation2006; Leith, 1974 as cited in Eysenck, Citation1996; Rowell & Renner, Citation1975; Shadbolt, Citation1978; Trown & Leith, Citation1975; Tuovinen & Sweller, Citation1999). The absence of an interaction effect between curiosity and structure of the environment might in the present study be explained by curiosity mainly being associated with the inquiry-learning outcomes (knowledge acquisition), while the structure manipulation affected the inquiry-learning process (quantity and quality of exploration).
The Scientific Discovery task was developed within the context of this study to measure children’s inquiry-based learning. The task focuses on the skill of designing informative experiments. As several studies have demonstrated primary school-aged children’s difficulty with the learning of this skill (e.g. Klahr & Nigam, Citation2004; Kuhn, Garcia-Mila, Zohar, & Andersen, Citation1995) and the present study focused on a relatively young age group (7- to 9-year-olds), the Scientific Discovery task was designed to present children with a relatively simple environment for performing informative experiments. The task therefore only has one type of variable (colour), with three levels (red, blue, and green). In line with this aim, the study’s results showed that children in all conditions performed above chance level and children from grade 2 and 3 did not differ in their performance on the task. In addition, the task was designed to not only assess the inquiry-learning outcomes but also the inquiry-learning process. Even though the task successfully distinguished between children’s knowledge acquisition and their exploration, a suggestion for improvement of the task concerns the reliability of one of the quantity of exploration measures: the number of played seconds. In the present version of the task, children were asked to sit down if they were done exploring, but informal, unsystematic observations during test administration showed that children’s motivation to stop exploring was not solely dependent on their certainty about the relative weights of the blocks. Some children explored shorter because they reasoned it was best to solve the task as fast as possible, while other children explored longer because their shyness prevented them from indicating they were done.
To conclude, the results of the present study bear relevance for the practice of (informal) science education. First, by investigating how curiosity relates to the inquiry-learning process as well as how curiosity relates to inquiry-learning outcomes, the results shed light on the manner in which curiosity impacts on inquiry-based learning. The results showing that curiosity is positively related to learning outcomes but not positively related to the learning process, tentatively suggest the need for educators to provide structure to the low curious group’s inquiry-learning environment by focusing on reflection on performed experiments. However, future research is needed to replicate and further look into these results. Second, the present study showed that the structure manipulation did not differ in effectiveness for low and high curious children, but did so for low and high intelligent children. That is, the added structure improved the quality of the inquiry-learning process for low, but not high, intelligent children. The results therefore tentatively suggest the need for educators to adapt the structure of the inquiry-learning environment to individual children.
Acknowledgements
We thank children and primary schools for their participation. We thank students Bo Baesjou, Zinzi Boonstra, Hilde Hogendoorn, Peggy Kornman, Romy ten Nijenhuis, Anne van Steijn, Eline Tan, Hester van Trommel, and Jacob Zwaan for their help in data collection. We thank Dennis Smit for his help in figure design. Finally, we are very grateful to Jamie Jirout (Rhodes College) and Kevin Willows (Carnegie Mellon University) for their willingness to share the Underwater Exploration game, and their help in making the Dutch version of the game. The Underwater Exploration game was developed with support, in part, from the Institute of Education Sciences, U.S. Department of Education through grant (R305B040063) to Carnegie Mellon University.
Disclosure statement
No potential conflict of interest was reported by the authors.
ORCID
Tessa J. P. van Schijndel http://orcid.org/0000-0002-9818-0796
Brenda R. J. Jansen http://orcid.org/0000-0001-9262-933X
Maartje E. J. Raijmakers http://orcid.org/0000-0003-1843-6462
Additional information
Funding
References
- Ackerman, P. L. (2003). Aptitude complexes and trait complexes. Educational Psychologist, 38(2), 85–93. doi: 10.1207/S15326985EP3802_3
- Alberti, E. T., & Witryol, S. L. (1994). The relationship between curiosity and cognitive ability in third- and fifth-grade children. The Journal of Genetic Psychology, 155(2), 129–145. doi: 10.1080/00221325.1994.9914767
- Alfieri, L., Brooks, P. J., Aldrich, N. J., & Tenenbaum, H. R. (2011). Does discovery-based instruction enhance learning? Journal of Educational Psychology, 103(1), 1–18. doi: 10.1037/a0021017
- Allen, S. (2004). Designs for learning: Studying science museum exhibits that do more than entertain. Science Education, 88(1), S17–S33. doi: 10.1002/sce.20016
- Arnone, M. P., Grabowski, B. L., & Rynd, C. P. (1994). Curiosity as a personality variable influencing learning in a learner controlled lesson with and without advisement. Educational Technology Research and Development, 42(1), 5–20. doi: 10.1007/BF02298167
- Berlyne, D. E. (1960). Conflict, arousal and curiosity. New York: McGraw-Hill.
- Bijvoet-van den Berg, S., & Hoicka, E. (2014). Individual differences and age-related changes in divergent thinking in toddlers and preschoolers. Developmental Psychology, 50(6), 1629–1639. doi: 10.1037/a0036131
- Bonawitz, E. B., Van Schijndel, T. J., Friel, D., & Schulz, L. (2012). Children balance theories and evidence in exploration, explanation, and learning. Cognitive Psychology, 64(4), 215–234. doi: 10.1016/j.cogpsych.2011.12.002
- Chen, Z., & Klahr, D. (1999). All other things being equal: Acquisition and transfer of the control of variables strategy. Child Development, 70(5), 1098–1120. doi: 10.1111/1467-8624.00081
- Clark, R. E. (1989). When teaching kills learning: Research on mathemathantics. In H. N. Mandl, N. Bennett, E. de Corte, & H. F. Freidrich (Eds.), Learning and instruction: European research in an international context (Vol. 2, pp. 1–22). London: Pergamon.
- Crowley, K., Callanan, M. A., Jipson, J. L., Galco, J., Topping, K., & Shrager, J. (2001). Shared scientific thinking in everyday parent-child activity. Science Education, 85(6), 712–732. doi: 10.1002/sce.1035
- Dean, D., & Kuhn, D. (2007). Direct instruction vs. discovery: The long view. Science Education, 91(3), 384–397. doi: 10.1002/sce.20194
- De Jong, T. (2006). COMPUTER SIMULATIONS: Technological advances in inquiry learning. Science, 312, 532–533. doi: 10.1126/science.1127750
- Dunbar, K., & Klahr, D. (1989). Developmental differences in scientific inquiry-based. In D. Klahr & K. Kotovsky (Eds.), Complex information processing: The impact of Herbert A. Simon (pp. 109–143). Hillsdale, NJ: Erlbaum.
- Endsley, R. C., Hutcherson, M. A., Garner, A. P., & Martin, M. J. (1979). Interrelationships among selected maternal behaviors, authoritarianism, and preschool children's verbal and nonverbal curiosity. Child Development, 50, 331–339. doi: 10.2307/1129407
- Eysenck, H. J. (1996). Personality and the experimental study of education. European Journal of Personality, 10(5), 427–439. doi:10.1002/(SICI)1099-0984(199612)10:5<427::AID-PER254>3.0.CO;2-H
- Field, A., Miles, J., & Field, Z. (2012). Discovering statistics using R. London: Sage Publications.
- Furtak, E., Seidel, T., Iverson, H., & Briggs, D. C. (2012). Experimental and quasi-experimental studies of inquiry-based science teaching: A meta-analysis. Review of Educational Research, 82(3), 300–329. doi: 10.3102/0034654312457206
- Gelman, R., & Brenneman, K. (2004). Science learning pathways for young children. Early Childhood Research Quarterly, 19, 150–158. doi: 10.1016/j.ecresq.2004.01.009
- Gijlers, H., & De Jong, T. (2005). The relation between prior knowledge and students’ collaborative discovery learning processes. Journal of Research in Science Teaching, 42(3), 264–282. doi: 10.1002/tea.20056
- Glogger-Frey, I., Fleischer, C., Grüny, L., Kappich, J., & Renkl, A. (2015). Inventing a solution and studying a worked solution prepare differently for learning from direct instruction. Learning and Instruction, 39, 72–87. doi: 10.1016/j.learninstruc.2015.05.001
- Hakkarainen, K., Lipponen, L., Järvelä, S., & Niemivirta, M. (1999). The interaction of motivational orientation and knowledge-seeking inquiry in computer-supported collaborative learning. Journal of Educational Computing Research, 21(3), 263–281. doi: 10.2190/C525-TDYQ-WWKY-87CB
- Hamel, R., & Schmittmann, V. D. (2006). The 20-minute version as a predictor of the Raven advanced progressive matrices test. Educational and Psychological Measurement, 66(6), 1039–1046. doi: 10.1177/0013164406288169
- Jansen, B. R., Louwerse, J., Straatemeier, M., Van der Ven, S. H., Klinkenberg, S., & Van der Maas, H. L. (2013). The influence of experiencing success in math on math anxiety, perceived math competence, and math performance. Learning and Individual Differences, 24, 190–197. doi: 10.1016/j.lindif.2012.12.014
- Jirout, J., & Klahr, D. (2012). Children’s scientific curiosity: In search of an operational definition of an elusive concept. Developmental Review, 32, 125–160. doi: 10.1016/j.dr.2012.04.002
- Kirschner, P. A., Sweller, J., & Clark, R. E. (2006). Why minimal guidance during instruction does not work: An analysis of the failure of constructivist, discovery, problem-based, experiential, and inquiry-based teaching. Educational Psychologist, 41(2), 75–86. doi: 10.1207/s15326985ep4102_1
- Klahr, D., & Nigam, M. (2004). The equivalence of learning paths in early science instruction: Effects of direct instruction and discovery learning. Psychological Science, 15(10), 661–667. doi: 10.1111/j.0956-7976.2004.00737.x
- Klahr, D., Zimmerman, C., & Jirout, J. (2011). Educational interventions to advance children’s scientific thinking. Science, 333(6045), 971–975. doi: 10.1126/science.1204528
- Kuhn, D. (2007). Is direct instruction an answer to the right question? Educational Psychologist, 42(2), 109–113. doi: 10.1080/00461520701263376
- Kuhn, D., Garcia-Mila, M., Zohar, A., & Andersen, C. (1995). Strategies of knowledge acquisition. Monographs of the Society for Research in Child Development, 60(4, Serial No. 245), i. doi: 10.2307/1166059
- Kyllonen, P. C., & Lajoie, S. P. (2003). Reassessing aptitude: Introduction to a special issue in honor of Richard E. Snow. Educational Psychologist, 38(2), 79–83. doi: 10.1207/S15326985EP3802_2
- Lauriola, M., Litman, J. A., Mussel, P., De Santis, R., Crowson, H. M., & Hoffman, R. R. (2015). Epistemic curiosity and self-regulation. Personality and Individual Differences, 83, 202–207. doi: 10.1016/j.paid.2015.04.017
- Leutner, D. (1993). Guided discovery learning with computer-based simulation games: Effects of adaptive and non-adaptive instructional support. Learning and Instruction, 3(2), 113–132. doi: 10.1016/0959-4752(93)90011-N
- Litman, J. A. (2005). Curiosity and the pleasures of learning: Wanting and liking new information. Cognition & Emotion, 19(6), 793–814. doi: 10.1080/02699930541000101
- Litman, J. A., & Jimerson, T. L. (2004). The measurement of curiosity as a feeling of deprivation. Journal of Personality Assessment, 82(2), 147–157. doi: 10.1207/s15327752jpa8202_3
- Loewenstein, G. (1994). The psychology of curiosity: A review and reinterpretation. Psychological Bulletin, 116(1), 75–98. doi: 10.1037/0033-2909.116.1.75
- Muis, K. R., Pekrun, R., Sinatra, G. M., Azevedo, R., Trevors, G., Meier, E., & Heddy, B. C. (2015). The curious case of climate change: Testing a theoretical model of epistemic beliefs, epistemic emotions, and complex learning. Learning and Instruction, 39, 168–183. doi: 10.1016/j.learninstruc.2015.06.003
- National Research Council. (2001). Inquiry and the national science education standards. Washington, DC: National Academies Press.
- Osborne, J., & Dillon, J. (2008). Science education in Europe: Critical reflections (Vol. 13). London: The Nuffield Foundation.
- Peterson, S. M., & French, L. (2008). Supporting young children's explanations through inquiry science in preschool. Early Childhood Research Quarterly, 23(3), 395–408. doi: 10.1016/j.ecresq.2008.01.003
- Piotrowski, J. T., Litman, J. A., & Valkenburg, P. (2014). Measuring epistemic curiosity in young children. Infant and Child Development, 23(5), 542–553. doi: 10.1002/icd.1847
- Preacher, K. J., & Hayes, A. F. (2004). SPSS and SAS procedures for estimating indirect effects in simple mediation models. Behavior Research Methods, Instruments, & Computers, 36(4), 717–731. doi: 10.3758/BF03206553
- Raven, J. C. (1965). Guide to using the coloured progressive matrices. London: H.K. Lewis & Co.
- Rowell, J. A., & Renner, V. J. (1975). Personality, mode of assessment and student achievement. British Journal of Educational Psychology, 45(2), 232–236. doi: 10.1111/j.2044-8279.1975.tb03248.x
- Samarapungavan, A., Patrick, H., & Mantzicopoulos, P. (2011). What kindergarten students learn in inquiry-based science classrooms. Cognition and Instruction, 29(4), 416–470. doi: 10.1080/07370008.2011.608027
- Schauble, L. (1990). Belief revision in children: The role of prior knowledge and strategies for generating evidence. Journal of Experimental Child Psychology, 49, 31–57. doi: 10.1016/0022-0965(90)90048-D
- Schauble, L. (1996). The development of scientific reasoning in knowledge-rich contexts. Developmental Psychology, 32(1), 102–119. doi: 10.1037/0012-1649.32.1.102
- Shadbolt, D. R. (1978). Interactive relationships between measured personality and teaching strategy variables. British Journal of Educational Psychology, 48(2), 227–232. doi: 10.1111/j.2044-8279.1978.tb02389.x
- Snow, R. E., & Lohman, D. F. (1984). Toward a theory of cognitive aptitude for learning from instruction. Journal of Educational Psychology, 76, 347–376. doi: 10.1037/0022-0663.76.3.347
- Stohr-Hunt, P. M. (1996). An analysis of frequency of hands-on experience and science achievement. Journal of Research in Science Teaching, 33(1), 101–109. doi:10.1002/(SICI)1098-2736(199601)33:1<101::AID-TEA6>3.0.CO;2-Z
- Svinicki, M. D. (1998). A theoretical foundation for inquiry-based learning. American Journal of Physiology, 275, S4–S7.
- Sweller, J., & Cooper, G. A. (1985). The use of worked examples as a substitute for problem solving in learning algebra. Cognition and Instruction, 2(1), 59–89. doi: 10.1207/s1532690xci0201_3
- Trevors, G. J., Muis, K. R., Pekrun, R., Sinatra, G. M., & Muijselaar, M. M. (2017). Exploring the relations between epistemic beliefs, emotions, and learning from texts. Contemporary Educational Psychology, 48, 116–132. doi: 10.1016/j.cedpsych.2016.10.001
- Trown, E., & Leith, G. O. M. (1975). Decision rules for teaching strategies in primary schools: Personality-treatment interactions. British Journal of Educational Psychology, 45(2), 130–140. doi: 10.1111/j.2044-8279.1975.tb03238.x
- Tuovinen, J. E., & Sweller, J. (1999). A comparison of cognitive load associated with discovery learning and worked examples. Journal of Educational Psychology, 91(2), 334–341. doi: 10.1037/0022-0663.91.2.334
- Van Schijndel, T. J. P., Franse, R. K., & and Raijmakers, M. E. J. (2010). The Exploratory Behavior Scale: Assessing young visitors’ hands-on behavior in science museums. Science Education, 94, 794–809. doi: 10.1002/sce.20394
- Van Schijndel, T. J. P., Visser, I., van Bers, B. M. C. W., & Raijmakers, M. E. J. (2015). Preschoolers perform more informative experiments after observing theory-violating evidence. Journal of Experimental Child Psychology, 131, 104–119. doi: 10.1016/j.jecp.2014.11.008
- Van Uum, M. S., Verhoeff, R. P., & Peeters, M. (2017). Inquiry-based science education: Scaffolding pupils’ self-directed learning in open inquiry. International Journal of Science Education, 39(18), 2461–2481. doi: 10.1080/09500693.2017.1388940
- Veenman, M. V., & Spaans, M. A. (2005). Relation between intellectual and metacognitive skills: Age and task differences. Learning and Individual Differences, 15(2), 159–176. doi: 10.1016/j.lindif.2004.12.001
- Veermans, M., & Järvelä, S. (2004). Generalized achievement goals and situational coping in inquiry learning. Instructional Science, 32(4), 269–291. doi: 10.1023/B:TRUC.0000026465.74406.47
- Zimmerman, C. (2007). The development of scientific thinking skills in elementary and middle school. Developmental Review, 27(2), 172–223. doi: 10.1016/j.dr.2006.12.001