ABSTRACT
Designing and interpreting controlled experiments are important inquiry skills addressed in many current science curricula. The relevant skills associated with the design and interpretation of controlled experiments are summarised under the term control-of-variables strategy (CVS). Research on elementary school students’ CVS skills shows that they have basic conceptions of CVS, as they can correctly identify controlled experiments to test given hypotheses and can interpret the results of controlled experiments. However, they perform poorly when they have to plan experiments and interpret confounded experiments. Furthermore, previous research has identified eight misleading preconceptions regarding CVS that cause invalid experimental designs, called design errors. The current study investigates the occurrence and change of design errors during elementary school and their consistency over different tasks. Via a latent class analysis (LCA), we identified three distinct patterns of design errors: (1) correct CVS understanding, (2) change of too many variables and (3) non-contrastive experiments. Our results show that many students as low as grade two have a basic understanding of CVS and that occurring design errors are associated with limited metaconceptual knowledge about when and why to apply CVS. We discuss possible implications of our findings for teaching CVS and further research.
Introduction
Designing and interpreting controlled experiments is an important science process and inquiry skill because it enables students to conduct their own informative investigations. Furthermore, the logic behind controlled experiments is relevant to argumentation and reasoning about causality in both science and everyday life, as it includes an understanding of the lack of evidence from confounded experiments (or observations) and the importance of controlled conditions (Kuhn, Citation2005). Thus, many current science curricula and standards address skills related to design and interpretation of controlled experiments. For example, the Next Generation Science Standards (NGSS Lead States, Citation2013) mention science and engineering practices such as designing fair tests and interpreting evidence generated from controlled experiments at all levels of education from kindergarten to grade 12. The NRC’s Framework for K-12 Science Education proposes that even kindergarten students should be able to ‘plan and carry out investigations […] based on fair tests’ (National Research Council, Citation2012, p. 5), that middle school students should ‘identify independent and dependent variables and controls’ (p. 55) and that high school students should ‘construct and revise explanations based on valid and reliable evidence […] including students’ own investigations’ (p. 75). In the literature, these different skills associated with the design and interpretation of controlled experiments are summarised under the term control-of-variables strategy (CVS).
Evidence from intervention studies shows that teaching CVS is effective in elementary (Bohrmann, Citation2017; Chen & Klahr, Citation1999; Dean & Kuhn, Citation2007; Klahr & Nigam, Citation2004) as well as secondary school levels (Schwichow, Zimmerman, et al., Citation2016). This finding is in line with the results of a meta-analysis summarising 72 CVS intervention studies which illustrate that teaching CVS is effective with a mean effect size of 0.61 (Schwichow, Croker, et al., Citation2016). Although prior research leads to the conclusion that teaching CVS is basically effective, not all students benefit from CVS interventions to the same extent. For example, Chen and Klahr (Citation1999, p. 1114) report that students’ CVS performance increased substantially in consequence of an intervention, but still only reached 65%. In an intervention study by Klahr and Nigam (Citation2004), 75% of the students who got a direct CVS instruction solved three out of four posttest tasks successfully while 25% of the students solved only two or less out of four tasks. Lorch et al. (Citation2010) found that all students benefit from CVS training, but that low-achieving students gain less than higher-achieving students do. A potential reason for the restricted effect of CVS trainings might be that they address only some preconceptions regarding the design and interpretation of experiments while neglecting less dominant ones. Thus, students holding such preconceptions might not benefit from direct instruction because their misconceptions are not addressed.
Siler and Klahr (Citation2012) present a comprehensive overview of seven known CVS preconceptions and their manifestation in experimental design errors. However, in order to ground CVS instruction to a broader number of preconceptions, more fine-grained information about the occurrence and change of these preconceptions and the related design errors in elementary school is required. Such information can inform the design of CVS interventions that consider a broader variety of CVS preconceptions and adapt the instruction strategy to students’ individual preconceptions based on diagnostic tests. The aim of this study is to utilise a multiple-choice instrument that captures students’ experimental design errors based on the chosen answer option and thereby investigates the occurrence and change of experimental design errors in elementary school.
Controlling variables: theoretical relevance and elementary school students’ performance
The idea of causality in science is that a variable X has a causal effect on a variable Y if ‘(i) there is a possible intervention (…) that changes the value of X such that (ii) if this intervention and no other intervention were carried out, the value of Y (…) would change’ (Woodward, Citation2003, p. 45). Accordingly, controlling variables in experiments is directly related to causality because it guarantees that the intended variable and no other variable has a causal effect. Therefore, scientific experiments are based on conditions that solely differ with respect to the values of one variable in which causal status is under investigation. All other variables should be non-varying. If scientists observe variations in the values of dependent variables of such a controlled experiment, they can conclude that solely the investigated independent variable has an effect on the dependent variable. Siler and Klahr (Citation2012) derived from this idea of controlled comparison the four following consecutive rules for designing controlled experiments. First, identify the variable and its values for which a causal effect on a dependent variable should be investigated. Second, set up at least two conditions that differ in the values of this variable. Third, set all other variables on the same values. Fourth, run the experiment and observe differences on the dependent variable between the two conditions.
Apart from designing controlled experiments, controlling variables is important in many different operations regarding the interpretation and evaluation of experiments. In accordance with Chen and Klahr (Citation1999) the term CVS covers procedural and logical aspects relevant to the design and interpretation of controlled experiments. The procedural aspects comprise (a) the planning and creation of experiments, which conditions differ in just a single contrasting variable, and (b) the identification of confounded and non-confounded experiments. In addition, CVS requires the understanding of its logic, which involves the ability to (c) make appropriate inferences from the results of non-confounded experiments (e.g. the understanding that inferences about the causal status of the variable being tested are warranted) and the ability to (d) recognise the inherent indeterminacy of confounded experiments (Chen & Klahr, Citation1999). A full understanding of CVS thus includes four subskills: (1) planning and creating controlled experiments (PL), (2) identifying confounded and non-confounded experiments (ID), (3) interpreting the outcome of controlled experiments (IN), and (4) understanding that inferences drawn from uncontrolled experiments are invalid (UN).
Studies with elementary students show that students’ performance on CVS tasks depends on the implemented subskills. For example, Bullock and Ziegler (Citation1999) conclude based on a longitudinal study that even elementary school students are able to distinguish between controlled and uncontrolled experiments to test a given hypothesis and to provide verbal justification for their decisions (corresponds to CVS subskill: identification). Nonetheless, they found that the majority of elementary school students are not able to plan and design controlled experiments (corresponds to CVS subskill: planning). Findings regarding elementary school students’ ability to interpret the outcome of experiments (corresponds to CVS subskill: interpreting) show that even six year-olds can draw correct causal inferences from experimental data if only a single variable is investigated, if the data shows unambiguous effects, and if the outcomes of the experiment is not conflicting with students’ preconceptions (Croker & Buchanan, Citation2011; Ruffman et al., Citation1993; Schulz & Gopnik, Citation2004; Tschirgi, Citation1980). However, when confronted with the outcome of a confounded experiment, only a few elementary students reject drawing causal inferences (corresponds to CVS subskill: understanding). This demonstrates that they do not understand that inferences drawn from uncontrolled experiments are invalid (Peteranderl & Edelsbrunner, Citation2020). Studies with secondary school students, which compare the difficulty of items implementing the CVS subskills, also found that the understanding subskill is particularly challenging for students and that only upper-secondary students solve these items (Schwichow, Christoph, et al., Citation2016; Schwichow et al., Citation2020; van Vo & Csapó, Citation2021).
In sum, these findings show that although elementary school students have some intuitive understanding of the identification and interpretation subskill, they perform poorly when planning controlled experiments and have no adequate understanding of confounded experiments. Thus, the question arises whether students’ poor performance on these tasks emerges from common systematic and misleading preconceptions regarding the design and interpretation of controlled experiments. In the following section, students’ performance of CVS tasks will be considered from the perspective of preconceptions guiding their cognitive process.
Students’ preconceptions regarding CVS
Students are no ‘tabula rasa’ when they enter formal science education. They rather hold ideas and intuitive models about many science phenomena. These preconceptions are ideas about natural phenomena that students develop based on their individual experiences, the use of language, or in consequence of inappropriate instruction. They are often quite coherent conceptions that have explanatory power with respect to multiple phenomena. Nonetheless, they are to some extent inconsistent or incompatible with scientific concepts and, therefore, cause erroneous responses compared to responses based on scientific concepts (Driver, Citation1989; Smith et al., Citation1994; Vosniadou, Citation2019). There are preconceptions with reference to scientific phenomena just as well as to inquiry skills. With regard to CVS, it is known that even elementary school students have an intuitive understanding of controlled experiments as ‘fair comparisons’. Many elementary school students are aware of the fact that experiments are used to test hypotheses or assumptions (for an overview see Zimmerman, Citation2007). Based on this intuitive understanding, even elementary school students can identify and interpret controlled experiments (Bullock et al., Citation2009).
However, misleading preconceptions dominate their CVS performance and cause mistakes when students plan experiments. Such mistakes violate the rules for producing controlled experiments (Siler & Klahr, Citation2012; see above). To take a deeper look into preconceptions and mistakes, Siler and Klahr (Citation2012) gathered various data in class, small groups, and one-on-one CVS instructions with fifth and six graders. In their study, they identified typical invalid experimental designs, called design errors, like designing confounded experiments (beside the investigated variable, one or more additional variables differ between conditions) or non-contrastive experiments (contrasting identical conditions). Based on students’ oral and written responses, they furthermore identified underlying preconceptions that produced the design errors. gives an overview of students’ design errors and the eight underlying preconceptions.
Table 1. Overview of CVS preconceptions, visible errors and misconceived aspects of the CVS according to Siler and Klahr (Citation2012) complemented by the hotat strategy described by Tschirgi (Citation1980).
The overview in shows that the preconceptions two to five cause the same design error which results in a contrast of confounded or multiply confounded conditions (more than one variable differs between conditions). With respect to the underlying conceptual idea, these preconceptions are quite different from each other but nonetheless all lead to the design of confounded experiments as a visible result. Reasons for designing confounded experiments are that students (1) believe that the additional varying variables have no causal effects, (2) just ignore them, (3) want to test multiple effects in the same experiment or (4) want to produce extreme differences on the dependent variable. The remaining three preconceptions cause unique design errors. Students who understand the logic of controlling variables but who have problems identifying the correct independent variable will compare controlled conditions but focus on the wrong independent variable (according to the given hypothesis). If students understand the importance of controlling variables, they might over-interpret this idea and thus build ‘totally fair conditions’ that differ in no variable and thus are non-informative. Students who do not understand why conditions are compared will eventually design an ‘experiment’ consisting of only one condition and neglect comparing at least two different conditions. Tschirgi (Citation1980) describes a further design error that she calls ‘hold-one-thing-at-a-time’ (hotat). In this case, students design experiments in which the independent variable is not varying between conditions, whereas all other variables differ. This design error is thus a special case of a confounded experiment. It is based on the idea that one has to find the one and only variable that has to be constant in order to get identical results under varying conditions. Overall the eight known preconceptions regarding CVS lead to five design errors in total: (1) designing (multiple) confounded experiments (ce), (2) designing controlled experiments for the wrong independent variable (cwv), (3) comparing identical conditions (non-contrastive experiments; nce), (4) designing only one experimental condition (ooe) and (5) designing confounded experiments in which only one independent variable is constant (hotat).
Siler and Klahr (Citation2012) and Tschirgi (Citation1980) identified these preconceptions and design errors while students worked on planning and designing choice tasks (corresponding to the planning and identification subskills). However, if preconceptions can be considered cross-situationally stable conceptions and not just task or context-specific mistakes, the corresponding design errors should emerge consistently in tasks implementing multiple CVS subskills. outlines the five known design errors and how they are manifested in tasks regarding identification, interpretation, and understanding CVS subskills.
Table 2. Overview of known CVS design errors and their manifest in identification, interpretation and understanding tasks.
Research questions
Research on elementary school students’ CVS skills illustrates that elementary school children have basic conceptions of controlled experiments, as they can correctly identify controlled experiments to test a given hypothesis and can interpret the results of controlled experiments. However, they perform poorly when they have to plan experiments and draw causal conclusions from confounded experiments. The typical experimental design errors that occur when they solve CVS tasks are related to eight misleading preconceptions regarding CVS. In order to increase the efficiency of CVS instructions and to adapt instruction to students’ individual design errors, information about the frequency of design errors in elementary school and changes in their occurrence during elementary school is required. Moreover, information about whether design errors depend on the addressed CVS subskill can inform the design of diagnostic tests. Thus, the current study investigates the occurrence and change of design errors during elementary school and their consistency over tasks regarding different CVS subskills. The research questions are:
Which design errors appear in the context of elementary school?
Which patterns of design errors can be identified over tasks regarding the interpretation, identification, and understanding CVS subskills?
Which of these patterns can be found in grades 2, 3, and 4?
Method
Sample
Students from 17 elementary schools in Southern Germany (in the state of Baden-Württemberg) participated in this study. The German elementary school covers grades 1 to 4 (age 6 to 10). Students from grades 2 to 4 participated in this study. We collected an initial sample of 570 students. Apart from gender, no demographic information referring to e.g. ethnicity, socioeconomic status, or school achievement was collected (for data privacy reasons). However, the sample can be considered representative because these schools are located in rural, urban, and suburban areas that represent typical neighbourhoods in the state of Baden-Wuerttemberg. We excluded 15 students from our analysis because they failed to complete more than 50% of the test. We excluded these students since we are interested in the consistency of design errors over tasks regarding different CVS subskills, and because these students did not answer items regarding at least two different CVS subskills. Moreover, we excluded 59 students because they failed to answer at least one item type twice. We excluded these students because we need a complete data sample for a planned latent class analysis and, as it is only reasonable to simulate missing data for individual students when only one of two possible answers is missing (for further information regarding the method see Data Scaling, Reliability Estimation and Statistical Models). The final sample of our analyses is N = 496 (48% female, 51% male, see for distribution of students over grade). The social and ethnic background of the students from the respective area of Germany can be described as predominantly middle class and of German origin.
Table 3. Distribution of students over grade (N = 496).
Assessment instruments
To assess students’ CVS skills, we adopted and expanded the CVS Inventory by Schwichow, Christoph, et al. (Citation2016) to an elementary school level. The original CVSI consists of 23 multiple-choice items embedded in secondary school physics contexts of heat and temperature, and electricity and electromagnetism. The CVSI instrument covers the identification (ID), interpretation (IN), and understanding (UN) of CVS subskills. We adapted the original version of the CVSI because (1) the contexts are not suitable for elementary school level, (2) we wanted to evaluate design errors systematically, (3) the items had to be adapted to the lower reading comprehension of elementary students and (4) we wanted to tackle a problem of ‘too easy’ (IN) and ‘too difficult’ (UN) items (see Schwichow, Christoph, et al., Citation2016) with more sophisticated short stories and answer options.
To adapt the experimental contexts for elementary students, we chose four contexts fitting to elementary school curricula: dropping of parachutes, floating of boats, scaling of lemonade, crawling of snails. The items for the contexts dropping of parachutes and floating of boats are based on items from the instrument by Peteranderl and Edelsbrunner (Citation2020). For each context, we created four items: two items for the identification subskill and one each for the interpretation and understanding subskill. We used two items for identification in order to collect a broader variety of design errors. We did not create items for the CVS subskill planning because this subskill is very challenging for primary school students (Bullock & Ziegler, Citation1999; Peteranderl & Edelsbrunner, Citation2020) and we wanted to keep a reasonable test length. Every item presents a short story and a hypothesis about a causal relationship. To meet the limited reading comprehension of elementary school children we used graphic representations for all experiments and independent variables used within the text. To ensure that our adapted version meets the requirements of elementary school children, we did two rounds of pilot testing and consulted experienced elementary school teachers.
In the case of items regarding the interpretation and understanding subskills, the original version of CVSI asked students to interpret results of experiments. As opposed to this, our interpretation and understanding items present information about whether or not the experiment in question is valid (interpretation subskill) or invalid (understanding subskill). Based on multiple given arguments, the students have to reason why the experiment was valid (interpretation items) or invalid (understanding items). The results of a pilot testing showed that after these changes we did not have ground or ceiling effects on the interpretation and understanding items anymore. shows a classification and short description of the items. The adapted version of the CVSI includes 16 multiple choice/multiple select items out of four contexts.
Table 4. Classification and description of the elementary school CVS items.
Evidence for the validity of measures from our test comes from a study by Brandenburger et al. (Citation2020, Citation2021) who utilised similar items in a CVS test with primary school children (grade 2 to 4). They found an increase in students’ CVS skills from grade 2 to 4 similar to the one reported in an interview study conducted by Bullock and Ziegler (Citation1999). They furthermore reported that their CVS items are sensitive to intervention effects and, in addition, the item difficulty depends on the applied CVS subskills and not on the item contexts, which is consistent with results of similar studies with different domains and contexts (Brandenburger & Mikelskis-Seifert, Citation2019). Thus, this is a strong indication in terms of construct validity because, theoretically, CVS is a domain general experimental strategy that does not depend on any domain specific knowledge.
Procedure
The data collection took place during a regular school lesson of 45 min. All data collection was done via paper-pencil tests. The items of the CVS test were presented in a fixed order, starting for every context with identification (ID1), followed by interpretation (IN), understanding (UN), and the second identification item (ID2). All items had the same context (e.g. snails). We used a test booklet design shown in (design based on Youden square design; Frey et al., Citation2009), so that we had a variety of contexts and a reasonable test time for the individual students. Every student worked on two contexts comprising four items each. Every context appears in first and second position with equal frequency over all booklets in order to control positioning effects between the contexts.
Table 5. Distribution of experimental contexts over test booklets.
Data scaling, reliability estimation, and statistical models
To explore students’ design errors, we assigned every answer option (ID1 and ID2 items) and answer pattern (IN and UN items) to the represented design error (see ). shows the design errors represented in the different item types.
Table 6. Possible design errors within an item type.
In the next step, we combined items of the same item type based on different contexts so that every student can be characterised by his/her two answers within one of the four item types. Based on this categorisation, we calculated the frequencies of design errors for every item type.
To find patterns in design errors, we used a latent class analysis (LCA). This analysis searches for subgroups of students who show similar answer patterns across the four item types. The classes can be viewed as the categories of a categorical latent variable. LCA is used when a dichotomous or polytomous response is observed for every person (Skrondal & Rabe-Hesketh, Citation2004). For the analysis, we used the software WINMIRA (Davier, Citation1997a).
To avoid the exclusion of cases due to single missing answers (overall 108 of 3968 answers were missing (< 3%); for details see ), we utilised ‘hot deck imputation’ to impute values for missing answers for the LCA (Rubin, Citation1987). This technique selects a (weighted) random value out of the distribution of given answers. With this method, we generated a similar variation in the missing data as in our complete data. This non-parametric method has several advantages, i.e. any type of variable can be replaced and no strong distributional assumptions are required (Pérez et al., Citation2002).
Table 7. Frequency of students’ design errors in the different item types (N = 992).
Results
Findings for research question 1 – frequency of the design errors
In the first step, we counted the design errors for every item type (see , research question 1). All expected design errors described in occur in a significant frequency (4% – 33% of the answers in a specific item type). In line with the results reported in previous studies (e.g. Bullock & Ziegler, Citation1999; Croker & Buchanan, Citation2011; Peteranderl & Edelsbrunner, Citation2020; Schwichow, Christoph, et al., Citation2016; Schwichow et al., Citation2020), the three CVS subskills differed in their difficulty for elementary school students.
About 70% of the students correctly applied CVS in the two item types of the identification (ID) subskill. The majority of design errors were made by choosing controlled experiments for the wrong variable (15%) or confounded experiments with two (10%) and three variables changed (10%). Only a small number of students identified non-contrastive experiments (6–8%) or only one experiment, without comparison, (4%) as suitable experimental settings.
In the interpretation (IN) and understanding (UN) subskill 30% resp. 38% of the students could correctly apply CVS. As mentioned above in previous studies, items of those two subskills struggled with being ‘too easy’ (IN) and ‘too difficult’ (UN) (Schwichow, Christoph, et al., Citation2016). With our adapted instrument, we could tackle that problem and provide items fitting for the design errors of elementary students without ceiling or floor effects. Overall, the most common misconception in IN as well as in UN was the application of confounded experiments with two variables changed (33% / 19%), followed by confounded experiments with three variables changed (12% / 7%) and non-contrastive experiments (8% / 14%). The least common design error was ‘hold-one-thing-at-a-time’ (5% / 6%).
Even if the proportions of correct answers and design errors differ between the measured subskills, we cannot investigate from descriptive results if students’ design errors are inconsistent over tasks or instead follow specific patterns. Therefore, we follow up the descriptive analysis with a latent class analysis.
Findings for research question 2 – identifying patterns of design errors
We conducted a latent class analysis (LCA) in order to identify patterns of design errors across different item types. For choosing a latent class model based on goodness-of-fit statistics, we drew bootstrap samples from the given LCA model and refit a new LCA model for each sample (performing the bootstrap first suggested by Aitkin et al., Citation1981; also see Davier, Citation1997b; Rost, Citation2004). We compared the test statistic (χ² and CRFootnote1) of our found LCA model with the distribution of simulated test statistics. Our model fits if the test statistic does not differ significantly from the simulated test statistics (p > .05). Furthermore, we relied on the AIC and BIC criterion and a likelihood ratio test. shows the results for the 1-class to 4-class model.
Table 8. Summary of estimated LCA models.
Looking at the empirical p-values of the bootstrap fit, we clearly need to reject a 1-class model (p < .05). The 2-, 3- and 4-class models show adequate fit for the χ² test statistic (p > .05). The 2-class and 4-class models barely meet the requirements for the Cressie Read test statistic (p > .05).
While the 2-class solution shows a mildly better BIC than the 3-class model, AIC and the likelihood ratio test show a better fit of the 3-class model (χ²(41) = 123.88; p = .000). The 4-class model is not of interest because it has a worse fit (AIC, BIC, Log-Likelihood). Overall, our model comparisons show that the 3-class solution is the best model to find students with distinct error patterns across the four item types.
To describe the three classes and connect them to possible underlying students’ preconceptions (research question 2), we counted the frequencies of design errors for every item type within every class ().
Figure 1. Distribution of design errors over classes. Note. CVS: correct application of the control-of-variables strategy; cwf: CVS for wrong variable, nce: Noncontrastive target variable, ooe: Single-condition experiment, ce1 & ce2: Confounded experiments, hotat: Hold-one-thing-at-a-time.
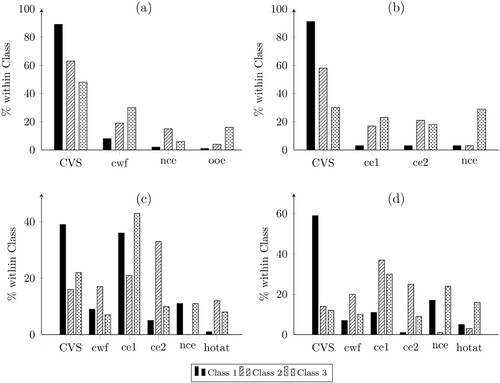
Class 1 (correct CVS understanding, 57.8% of the sample): Students in class 1 are able to apply CVS in items regarding the identification, interpretation, and understanding subskills. 89% (ID2) or 91% (ID1) of their answers to identification items were correct. In the subskill ‘interpretation’ they still have the best results of all classes (39% CVS). If they make errors, it is mostly the choice of a confounded experiment (two variables change) (36% ce1). Nevertheless, class 1 students understand the problem of confounded experiments as they solve 59% of the understanding items by stating that the experiments are poorly designed because too many variables differ between conditions.
Class 2 (change of too many variables, 22.2% of the sample): Students in class 2 basically understand that variables need to differ between contrasted conditions (CVS rules 1 & 2). Nonetheless, they do not comprehend why all non-investigated variables should be equal (violation of rule 3). Accordingly, their answers represent design errors associated with the choice or justification of confounded experiments (ce1, ce2). In particular, such design errors appear in interpretation and understanding items. In interpretation items, students do not state that in a controlled experiment some variables need to be constant (21% ce1, 32% ce2 within IN items). In understanding items, they state that a purely planned experiment has too few changed variables (37% ce1, 25% ce2 within UN items).
Class 3 (non-contrastive experiments, 19.9% of the sample): Students in class 3 basically understand that it is part of CSV to keep variables unchanged (CVS rule 3). However, they tend to over-generalise this concept (violating CVS rules 1 and 2). In the subskill ‘identification’, they show the error ‘only one experiment’ more often than any other class (16% within ID1) and stick to this concept even if the non-contrastive experiment is the only choice with unchanging variables (29% within ID2). Furthermore, they show these design errors in understanding items when they state that the experiment is flawed because all variables are changed (nce 24%, ncv 16%). However, in interpretation items, the most dominant design errors of class 3 students are associated with confounded experiments.
Our results show that dominant design errors as well as aspects of correct CVS understanding prove to be consistent to some degree in all three classes and over items regarding different CVS subskills. However, in all classes students also give answers not associated with the dominant design error. For example, in identification items, class 2 and 3 students most frequently choose a correctly designed controlled experiment. Nonetheless, if students out of class 2 and 3 make mistakes they are mostly associated with the dominant design error characterising their class. Moreover, students in class 1 show a nearly perfect CVS understanding in identification items, yet solve less than 50% of the interpretation items and only 60% of the understanding items. Nonetheless, they are the most successful class in solving these types of items.
Findings for research question 3 – patterns of design errors in grades 2, 3, and 4
To verify the identified classes with the expected development of CVS from grade 2 to 3 (research question 3), we looked at the distribution of classes over grade ().
Table 9. Contingency table class X grade (N = 496).
There was a significant association between the grade and the class found in the LCA (χ²(4) = 24.73, p = .000; Cramer’s V = .158, p = .000, small to medium effect). To break down the results of the χ² test, we looked at the standardised residuals. We can conclude that significantly less students than expected out of grade 2 are in class 1 (correct CVS understanding) (p = .021) and significantly more students than expected are in class 2 (change too many variables) (p = .028). For grade 4, this effect is reversed – more students than expected are in class 1 (p = .028) and less students than expected are in class 2 (p = .028). For class 3 (non-contrastive experiments), we found no significant difference between expected and observed counts in grades 2 and 4. Nonetheless, we can see a similar tendency for standardised residuals as in class 2. In grade 2, more students show a flawed understanding of CVS than in grade 4. As expected, we can summarise that a student is more likely to have a better understanding of CVS if she/he is in a higher grade.
Discussion
Summary
The aim of our study is to investigate the occurrence (research question 1) and change of experimental design errors during elementary school (research question 3) and their consistency over tasks regarding the identification, interpretation, and understanding CVS subskills (research question 2). Concerning our first research question, we found all five design errors reported in the literature on items regarding all three CVS subskills. The frequency of occurrence varies between 4% (only on experiment, no comparison) and 33% (confounded experiment – two variables changed). However, for both types of identification items (ID1: 73%; ID2: 70%) and the understanding items (UN: 38%) the most frequent observed responses are associated with controlled experiments. Only for interpretation items did more students choose answers associated with the design error of confounded experiments (45%) than answers associated with controlled experiments (30%).
To investigate the consistency of experimental design errors over tasks regarding different CVS subskills (research question 2) we conducted a latent class analyses (LCA). By this analysis, we identified three subgroups of students (classes) who show similar answer patterns across the four item types (ID1, ID2, IN, UN). Class 1 is the largest class (57.8% of the students are in this class) and contains students who show a correct CVS understanding across nearly all items. Only on interpretation items, they choose 30% of answer options representing the design error of confounded experiments. As mentioned before, this is because they did not state that it is important to hold non-investigated variables equal between experimental conditions. In sum, class 1 students know that they have to compare two conditions (CVS rules 1 & 2) that differ only regarding one variable (CVS rule 3) and that they have to observe variations in the dependent variable (CVS rule 4). However, they cannot explicitly justify when to apply these rules.
The remaining two classes both contain about 20% of the students. The second class represents students who choose answers associated with the design error of confounded experiments. However, on both identification items, they prevalently choose answers representing a correct CVS understanding. As opposed to class 1 students, they not only ignore the relevance of equal conditions in interpretation items but also do not state that the experiments presented in the understanding items are invalid, because non-investigated variables differ between conditions. In sum, it seems that class 2 students know that they have to compare conditions in experiments (CVS rule 2 & 4), and prefer controlled experiments when confronted with different experimental designs but cannot explicitly state the relevance of controlling variables (violation of CVS rule 3).
Class 3 students show a less clear pattern of design errors. Surprisingly, they exhibit design errors that represent a lack of understanding as to why they should compare conditions (design errors nce: of non-contrastive experiments, ooe: only one experimental condition) or that investigated variables should differ (hotat: hold one thing at a time). These design errors hardly ever occur in the case of class 1 or class 2 students. Accordingly, class 3 students display unique design errors that are associated with an incorrect manipulation or identification of independent variables. However, they also show the design errors of confounded experiments and the lowest frequencies of answers that are associated with a correct CVS understanding. A prevalent correct CVS understanding and only few design errors can only be found in identification type 1 items. In sum, it seems that class 3 students do not understand that they have to compare conditions in experiments (violation of CVS rules 1 and 2) and in consequence do not see the relevance of controlling variables between conditions (violation of CVS rule 3).
To answer our third research question we looked at the distribution of the identified classes over grades. Our results () show that the percentage of students who are in classes associated with an erroneous CVS understanding (class 2 and 3) decreases from grade two to four, while the percentage of students in class 1 increases from 37.7% to 72.1%. Thus, we found a clear trend from erroneous to sufficient understandings of CVS in the course of elementary school. The distribution of class 2 and class 3 students over grades is nearly identical so that we have no evidence for an order or developmental trajectory from one class of erroneous CVS understanding to another. It seems that both classes of errors occur in all grades so that both should be addressed by instructional interventions.
The question remains unaddressed as to what enhances CVS understanding from grade two up to grade four. A straightforward hypothesis would be that CVS skills increase because teachers address them explicitly in science classes. Although the elementary science curriculum aims at skills like designing and conducting controlled experiments (Ministerium für Kultus, Jugend und Sport, Citation2016), this can hardly be the decisive factor. A video study focusing on lab work in science classes demonstrated that teachers rarely address procedural skills like CVS explicitly (Nehring et al., Citation2016). However, even if CVS skills are not addressed explicitly they can increase in consequence of an instruction that focuses on science content. Schalk et al. (Citation2019) compared a regular science curriculum to a curriculum using a high proportion of experiments to introduce concepts like floating and sinking or air and atmospheric pressure, but without any explicit introduction of CVS. They found that students who learn with the experimental curriculum exhibit a higher increase in conceptual knowledge as well as in their CVS skills compared to students in regular classes. However, this does not imply that learning CVS implicitly is as effective as or even more effective than an explicit introduction. Instead, more students improve their CVS skills if they learn explicitly (Strand-Cary & Klahr, Citation2008), in particular when they are low achieving students (Lorch et al., Citation2010). As understanding CVS and understanding science content knowledge positively influence each other (Edelsbrunner et al., Citation2018; Koerber & Osterhaus, Citation2019; Schwichow et al., Citation2020; Stender et al., Citation2018), such direct instructions of CVS might not only enhance students’ CVS skills but also have positive effects on their conceptual understanding. Our findings can guide the design of such explicit instructions as they offer new insights into what students already know and what they have to learn about CVS.
Findings regarding the interpretation and understanding subskill
The percentage of correctly solved identification items has the same magnitude as in previous studies (Bullock & Ziegler, Citation1999; Schwichow et al., Citation2020). However, our findings regarding the interpretation and understanding items differ from previous studies employing these subskills. Schwichow, Christoph, et al. (Citation2016) reported that most secondary school students are able to solve interpretation items, whereas only very few solve understanding items. The students in Schwichow, Christoph, et al. (Citation2016) study are older and in higher grades than the students in this study, although a comparison with these findings is interesting. We found better performance on understanding tasks although our students are younger. At first sight, this outcome is rather counterintuitive. One reason for this might be the amount of information given by the item. Schwichow, Christoph, et al. (Citation2016) presented students with the results of a confounded experiment and asked them to draw conclusions (i.e. choosing between various causal interpretations and the additional statement that the experiment was invalid). As opposed to this, we informed the students that the experiment was invalid and asked for reasons why. The same differences apply to the interpretation items in which Schwichow, Christoph, et al. (Citation2016) just asked for an interpretation of the presented outcome of a controlled experiment while we presented students with the outcome plus an interpretation and asked them to give reasons why the experiment was valid. Thus, many elementary students are aware that confounded variables are the reason why an experiment is invalid. However, without an explicit hint, they do not search for potential confounding variables. Likewise, the lower performance on our interpretation items might reflect that students do not search for confounded variables when interpreting experimental outcome. When prompted to assess the validity of an experiment, they tend to deny the importance of equal conditions. Thus, it seems that the reason why students ignore confounding variables is not a wrong conception of the four CVS rules (a cognitive aspect) but rather insufficient metacognitive knowledge about when to apply rule 3 to search for confounding variables (Zohar & Peled, Citation2008a). In general, metacognitive knowledge comprises (1) knowledge about strategies referring to a task and (2) criteria to select the strategy that applies to the solution of the task (Kuhn, Citation1999). Accordingly, metacognitive knowledge regarding CVS tasks is comprised of knowing that when testing or evaluating over causal hypothesis one has to vary the focal variable and hold non-investigated variables constant between conditions. A sufficient strategy to achieve this is to set (or to check that) the values of all non-investigated variables are equal between conditions and that only if this is the case one can draw causal inferences from experiments (rule 3). Zohar and Peled (Citation2008a) demonstrated that students (fifth graders) can achieve this metacognitive knowledge during classroom discourses in which they discuss why and when to control variables.
However, as changes in item formulation dramatically changes the difficulty of items of both items both with respect to interpretation and understanding items we research should investigate the relation between item formulations and item difficulty. To do this, new instruments should combine items that ask for reasons for or against the validity of experimental outcomes and items that only ask students to interpret the outcome of confounded and controlled experiments.
Limitations
A limitation of our test instrument is that we cannot differentiate between different preconceptions that cause design errors associated with the comparison of multiple confounded experiments, as we only trace design errors and do not ask for justifications in order to get insight into the different underlying preconceptions that cause these errors. Furthermore, the results of the LCA show that the design errors, which are associated with unique preconceptions, are less frequent. In consequence, our instrument insufficiently identifies specific preconception regarding CVS. However, based on our instrument we are able to identify patterns or classes of design errors that seem to cluster, and that offer more nuanced information about elementary students’ CVS skills and their errors than traditional test instruments that only differentiate between correct and incorrect CVS performance (e.g. Bullock & Ziegler, Citation1999; Edelsbrunner et al., Citation2018; Klahr & Nigam, Citation2004).
To measure elementary students CVS skills, we adopted the existing CVS instrument by Schwichow, Christoph, et al. (Citation2016) to meet the curriculum and students’ reading abilities in elementary school. Like the original instrument, we did not include items on the planning subskill, because this subskill is particularly challenging for elementary students (Bullock & Ziegler, Citation1999; Peteranderl & Edelsbrunner, Citation2020). Therefore, our findings are limited, as an important CVS subskill is not implemented in our test instrument. However, we succeed in adopting the difficulty of interpretation and understanding items to meet the abilities of elementary students. Further studies should complement our item pool and construct planning items that can be utilised in elementary school.
Implications for teaching CVS skills
Our findings offer a nuanced view on students’ CVS skills. Even though many students as low as grade two show a basic understanding of CVS, we found two types of common design errors that are rather stable over items addressing different subskills. The first design error is associated with changing too many variables between experimental conditions (students in class 2) and the second with the incorrect manipulation or identification of the independent variable (students in class 3). Accordingly, interventions should address both types explicitly in order to reach as many students as possible. Most interventions focus on the relevance of holding control variables constant between conditions but do not address the importance of changing the investigated variable. This might be an explanation for the restricted effect of CVS interventions (Chen & Klahr, Citation1999; Klahr & Nigam, Citation2004; Lorch et al., Citation2010), as the percentage of students who did not benefit from these interventions has roughly the same value as class 3 students in our study (about 20%).
Moreover, teachers and researchers can utilise our results and test instruments to implement CVS interventions that match the design errors of individual students. By using our test instrument to diagnose the dominant design errors of individual students teachers can allocate students to individual instructions that either focus on the relevance of controlling variables or why and how to manipulate the independent variable. However, to design and give instructions that consider students’ design errors, teachers need both a reasonable understanding of CVS themselves along with knowledge about students’ preconceptions and design errors. Further studies should address teachers’ knowledge about these two aspects and how their understanding can be improved.
Both classes of identified design errors are not only restricted to flawed CVS conceptions but are associated with limited metacognitive knowledge about when and why to apply the four CVS rules. As teaching metacognitive knowledge regarding CVS is known to be effective with older students (Zohar & Peled, Citation2008a, Citation2008b), a straightforward implication of this finding is that CVS interventions in elementary school should focus on metacognitive knowledge about CVS, too. In order to enable students to apply the four CVS rules in tasks addressing different CVS subskills, it seems promising to teach students how to apply the CVS strategy in different CVS tasks and to identify the subskills required in specific CVS tasks. This might help the student to overcome a production deficit, i.e. failing to apply available CVS skills in relevant situations (Thillmann et al., Citation2013), which might be one reason why younger students fail to transfer CVS in new situations (Chen & Klahr, Citation1999).
A possible option to facilitate metacognitive knowledge is to confront students with confounded experiments in order to induce negative knowledge about what is wrong and what is to be avoided when planning and interpreting experiments (Gartmeier et al., Citation2008). Similarly, to address the design errors of class 3 students (problems manipulating or identifying the independent variables) one could confront students with non-contrastive experiments or experiments that vary the wrong variable. Thereby, it is an open-ended question whether or not teachers should just confront students with the described experiments, apply some form of direct instruction, or offer guidance during the analyses and discussion of these experiments. Investigating the best way for teaching metacognitive knowledge regarding CVS could be the focus of future intervention studies.
Implications for testing CVS skills
Compared to instruments that rate items just as correct or incorrect, our test instrument can provide detailed information about students’ design errors. This is of particular relevance to research on CVS as a meta-analysis by Schwichow, Croker, et al. (Citation2016) showing that the utilised test instrument has a strong influence on intervention effects. Our instrument, which is available as an online supplement to this paper, can help draw a more detailed picture of the effect of intervention studies or developmental trajectories. For example, further intervention studies can utilise the instrument to investigate how students’ pre-instructional design errors influence the effect and transfer of CVS skills in consequence of an intervention. Investigating such aptitude treatment interactions can foster our understanding of students’ design errors (students in different classes) in relation to different teaching strategies. Applying the instrument in longitudinal studies can answer questions regarding developmental changes in the conceptual understanding of CVS (e.g. by analyzing the data via latent transition analysis, Collins & Lanza, Citation2010). Moreover, teachers can utilise our instrument for a more detailed assessment of their students’ CVS skills in order to identify the dominant design error of individual students and to offer instructional aids addressing these design errors. Even if further studies and teachers cannot utilise our instrument directly because they need items embedded in different contexts due to different curricula, they can adapt our instrument to their requirements because of the systematic item construction.
Supplemental Material
Download MS Word (596.6 KB)Disclosure statement
No potential conflict of interest was reported by the author(s).
Additional information
Funding
Notes
1 CR (Cressie Read) is an alternative test statistic suggested in Cressie and Read (Citation1984) that is less liberal than χ², but both test statistics can be used as bootstrapping goodness-of-fit statistics Davier (Citation1997b).
References
- Aitkin, M., Anderson, D., & Hinde, J. (1981). Statistical modelling of data on teaching styles. Journal of the Royal Statistical Society. Series a (General), 144(4), 419. https://doi.org/https://doi.org/10.2307/2981826
- Bohrmann, M. (2017). Zur Förderung des Verständnisses der Variablenkontrolle im naturwissenschaftlichen Sachunterricht [Facilitating the understanding of controlling variables in science eduaction]. Logos Verlag.
- Brandenburger, M., & Mikelskis-Seifert, S. (2019). Facetten experimenteller Kompetenz in den Naturwissenschaften [Facets of experimental competence in science]. In C. Maurer (Hrsg.), Naturwissenschaftliche Bildung als Grundlage für berufliche und gesellschaftliche Teilhabe. Gesellschaft für Didaktik der Chemie und Physik, Jahrestagung in Kiel 2018. (S. 77). Universität Regensburg. https://gdcp-ev.de/wpcontent/tb2019/TB2019_77_Brandenburger.pdf
- Brandenburger, M., Mikelskis-Seifert, S., Schwichow, M., & Wilbers, J. (2020). Variablenkontrollstrategien in der Grundschule [Control of variables strategy (CVS) in elementary school]. In S. Habig (Hrsg.), Naturwissenschaftliche Kompetenzen in der Gesellschaft von morgen. Gesellschaft für Didaktik der Chemie und Physik, Jahrestagung in Wien 2019. (S. 130). Universität Duisburg-Essen. https://gdcp-ev.de/?p=3714
- Brandenburger, M., Salim, C. A., Schwichow, M., & Wilbers, J. (2021). Modellierung der Struktur der Variablenkontrollstrategie und Abbildung von Veränderungen in der Grundschule [Modelling the structure of the control of variables strategy (CVS) and mapping changes in CVS through elementary school]. Zeitschrift für Didaktik der Naturwissenschaften.
- Bullock, M., Sodian, B., & Koerber, S. (2009). Doing experiments and understanding science: Development of scientific reasoning from childhood to adulthood. In W. Schneider & M. Bullock (Eds.), Human development from early childhood to early adulthood. Findings from the Munich longitudinal study (pp. 173–197). Lawrence Erlbaum.
- Bullock, M., & Ziegler, A. (1999). Scientific reasoning: Developmental and individual differences. In F. E. Weinert & W. Schneider (Eds.), Individual development from 3 to 12: Findings from the Munich longitudinal study (pp. 38–54). Cambridge University Press.
- Chen, Z., & Klahr, D. (1999). All other things being equal: Acquisition and transfer of the control of variables strategy. Child Development, 70(5), 1098–1120. https://doi.org/https://doi.org/10.1111/1467-8624.00081
- Collins, L. M., & Lanza, S. T. (2010). Latent class and latent transition analysis: With applications in the social, behavioral, and health sciences. John Wiley & Sons, Inc.
- Cressie, N., & Read, T. R. C. (1984). Multinomial goodness-of-fit tests. Journal of the Royal Statistical Society: Series B (Methodological), 46(3), 440–464. https://doi.org/https://doi.org/10.1111/j.2517-6161.1984.tb01318.x
- Croker, S., & Buchanan, H. (2011). Scientific reasoning in a real-world context: The effect of prior belief and outcome on children’s hypothesis-testing strategies. British Journal of Developmental Psychology, 29(3), 409–424. https://doi.org/https://doi.org/10.1348/026151010X496906
- Davier, M. (1997a). WINMIRA – Program description and recent enhancements. Methods of Psychological Research Online, 2(2), 25–28.
- Davier, M. (1997b). Bootstrapping goodness-of-fit statistics for sparse categorical data: Results of a monte carlo study. Methods of Psychological Research Online, 2(2), 29–48.
- Dean, D., & Kuhn, D. (2007). Direct instruction vs. discovery: The long view. Science Education, 91(3), 384–397. https://doi.org/https://doi.org/10.1002/sce.20194
- Driver, R. (1989). Students’ conceptions and the learning of science. International Journal of Science Education, 11(5), 481–490. https://doi.org/https://doi.org/10.1080/0950069890110501
- Edelsbrunner, P. A., Schalk, L., Schumacher, R., & Stern, E. (2018). Variable control and conceptual change: A large-scale quantitative study in elementary school. Learning and Individual Differences, 66, 38–53. https://doi.org/https://doi.org/10.1016/j.lindif.2018.02.003
- Frey, A., Hartig, J., & Rupp, A. A. (2009). An NCME instructional module on booklet designs in large-scale assessments of student achievement: Theory and practice. Educational Measurement: Issues and Practice, 28(3), 39–53. https://doi.org/https://doi.org/10.1111/j.1745-3992.2009.00154.x
- Gartmeier, M., Bauer, J., Gruber, H., & Heid, H. (2008). Negative knowledge: Understanding professional learning and expertise. Vocations and Learning, 1(2), 87–103. https://doi.org/https://doi.org/10.1007/s12186-008-9006-1
- Klahr, D., & Nigam, M. (2004). The equivalence of learning paths in early science instruction: Effects of direct instruction and discovery learning. Psychological Science, 15(10), 661–667. https://doi.org/https://doi.org/10.1111/j.0956-7976.2004.00737.x
- Koerber, S., & Osterhaus, C. (2019). Individual differences in early scientific thinking: Assessment, cognitive influences, and their relevance for science learning. Journal of Cognition and Development, 20(4), 510–533. https://doi.org/https://doi.org/10.1080/15248372.2019.1620232
- Kuhn, D. (1999). Metacognitive development. In L. Balter & C. S. Tamis-LeMonda (Eds.), Child psychology: A handbook of contemporary issues (pp. 259–286). Taylor and Francis.
- Kuhn, D. (2005). Education for thinking. Harvard University Press.
- Lorch, R. F., Lorch, E. P., Calderhead, W. J., Dunlap, E. E., Hodell, E. C., & Freer, B. D. (2010). Learning the control of variables strategy in higher and lower achieving classrooms: Contributions of explicit instruction and experimentation. Journal of Educational Psychology, 102(1), 90–101. https://doi.org/https://doi.org/10.1037/a0017972
- Ministerium für Kultus, Jugend und Sport. (2016). Bildungsplan der Grundschule. Sachunterricht. [Elementary curriculum. Science]. Retrieved February 17, 2021, from http://www.bildungsplaene-bw.de/site/bildungsplan/get/documents/lsbw/export-pdf/depot-pdf/ALLG/BP2016BW_ALLG_GS_SU.pdf
- National Research Council. (2012). A framework for K-12 science education: Practices, crosscutting concepts, and core ideas. The National Academies.
- Nehring, A., Stiller, J., Nowak, K. H., Upmeier zu Belzen, A., & Tiemann, R. (2016). Naturwissenschaftliche Denk- und Arbeitsweisen im Chemieunterricht – eine modellbasierte Videostudie zu Lerngelegenheiten für den Kompetenzbereich der Erkenntnisgewinnung. [Inquiry methods and scientific reasoning in chemistry education – A model-based video study on learning opportunities in the field of scientific inquiry]. Zeitschrift für Didaktik der Naturwissenschaften, 22(1), 77–96. https://doi.org/https://doi.org/10.1007/s40573-016-0043-2
- NGSS Lead States. (2013). Next generation science standards: For states, by states. The National Academies Press.
- Pérez, A., Dennis, R. J., Gil, J. F. A., Rondón, M. A., & López, A. (2002). Use of the mean, hot deck and multiple imputation techniques to predict outcome in intensive care unit patients in Colombia. Statistics in Medicine, 21(24), 3885–3896. https://doi.org/https://doi.org/10.1002/sim.1391
- Peteranderl, S., & Edelsbrunner, P. A. (2020). The predictive value of the understanding of inconclusiveness and confounding for later mastery of the control-of-variables strategy. Frontiers in Psychology, 11, 531565. https://doi.org/https://doi.org/10.3389/fpsyg.2020.531565
- Rost, J. (2004). Lehrbuch Testtheorie – Testkonstruktion [Textbook test theory and test construction]. Huber.
- Rubin, D. B. (1987). Multiple imputation for nonresponse in surveys. Wiley series in probability and mathematical statistics Applied probability and statistics. Wiley. https://doi.org/https://doi.org/10.1002/9780470316696
- Ruffman, T., Perner, J., Olson, D. R., & Doherty, M. (1993). Reflecting on scientific thinking: Children’s understanding of the hypothesis-evidence relation. Child Development, 64(6), 1617–1636. https://doi.org/https://doi.org/10.1111/j.1467-8624.1993.tb04203.x
- Schalk, L., Edelsbrunner, P. A., Deiglmayr, A., Schumacher, R., & Stern, E. (2019). Improved application of the control-of-variables strategy as a collateral benefit of inquiry-based physics education in elementary school. Learning and Instruction, 59, 34–45. https://doi.org/https://doi.org/10.1016/j.learninstruc.2018.09.006
- Schulz, L. E., & Gopnik, A. (2004). Causal learning across domains. Developmental Psychology, 40(2), 162–176. https://doi.org/https://doi.org/10.1037/0012-1649.40.2.162
- Schwichow, M., Christoph, S., Boone, W. J., & Härtig, H. (2016). The impact of sub-skills and item content on students’ skills with regard to the control-of-variables-strategy. International Journal of Science Education, 38(2), 216–237. https://doi.org/https://doi.org/10.1080/09500693.2015.1137651
- Schwichow, M., Croker, S., Zimmerman, C., Höffler, T., & Härtig, H. (2016). Teaching the control-of-variables strategy: A meta analysis. Developmental Review, 39, 37–63. https://doi.org/https://doi.org/10.1016/j.dr.2015.12.001
- Schwichow, M., Osterhaus, C., & Edelsbrunner, P. A. (2020). The relation between the control-of-variables strategy and content knowledge in physics in secondary school. Contemporary Educational Psychology, 63, 101923. https://doi.org/https://doi.org/10.1016/j.cedpsych.2020.101923
- Schwichow, M., Zimmerman, C., Croker, S., & Härtig, H. (2016). What students learn from hands-on activities. Journal of Research in Science Teaching, 53(7), 980–1002. https://doi.org/https://doi.org/10.1002/tea.21320
- Siler, S. A., & Klahr, D. (2012). Detecting, classifying and remediating: Children’s explicit and implicit misconceptions about experimental design. In R. W. Proctor & E. J. Capaldi (Eds.), Psychology of science: Implicit and explicit processes (pp. 137–180). Oxford University Press.
- Skrondal, A., & Rabe-Hesketh, S. (2004). Generalized latent variable modeling: Multilevel, longitudinal, and structural equation models. Chapman & Hall/CRC interdisciplinary statistics series. Chapman & Hall/CRC. http://search.ebscohost.com/login.aspx?direct=true&scope=site&db=nlebk&db=nlabk&AN=111068
- Smith, J. P., Disessa, A. A., & Roschelle, J. (1994). Misconceptions reconceived: A constructivist analysis of knowledge in transition. Journal of the Learning Sciences, 3(2), 115–163. https://doi.org/https://doi.org/10.1207/s15327809jls0302_1
- Stender, A., Schwichow, M., Zimmerman, C., & Härtig, H. (2018). Making inquiry-based science learning visible: The influence of CVS and cognitive skills on content knowledge learning in guided inquiry. International Journal of Science Education, 72(7), 1–20. https://doi.org/https://doi.org/10.1080/09500693.2018.1504346
- Strand-Cary, M., & Klahr, D. (2008). Developing elementary science skills: Instructional effectiveness and path independence. Cognitive Development, 23(4), 488–511. https://doi.org/https://doi.org/10.1016/j.cogdev.2008.09.005
- Thillmann, H., Gößling, J., Marschner, J., Wirth, J., & Leutner, D. (2013). Metacognitive knowledge about and metacognitive regulation of strategy use in self-regulated scientific discovery learning: New methods of assessment in computer-based learning environments. In R. Azevedo & V. Aleven (Eds.), International handbook of metacognition and learning technologies (pp. 575–588). Springer.
- Tschirgi, J. E. (1980). Sensible reasoning: A hypothesis about hypotheses. Child Development, 51(11), 1–10. https://doi.org/https://doi.org/10.2307/1129583
- van Vo, D., & Csapó, B. (2021). Development of scientific reasoning test measuring control of variables strategy in physics for high school students: Evidence of validity and latent predictors of item difficulty. International Journal of Science Education, 14(2), 1–21. https://doi.org/https://doi.org/10.1080/09500693.2021.1957515
- Vosniadou, S. (2019). The development of students’ understanding of science. Frontiers in Education, 4, 2368. https://doi.org/https://doi.org/10.3389/feduc.2019.00032
- Woodward, J. (2003). Making things happen: A theory of causal explanation. Oxford studies in philosophy of science. Oxford University Press.
- Zimmerman, C. (2007). The development of scientific thinking skills in elementary and middle school. Developmental Review, 27(2), 172–223. https://doi.org/https://doi.org/10.1016/j.dr.2006.12.001
- Zohar, A., & David, A. B. (2008b). Explicit teaching of meta-strategic knowledge in authentic classroom situations. Metacognition and Learning, 3(1), 59–82. https://doi.org/https://doi.org/10.1007/s11409-007-9019-4
- Zohar, A., & Peled, B. (2008a). The effects of explicit teaching of metastrategic knowledge on low- and high-achieving students. Learning and Instruction, 18(4), 337–353. https://doi.org/https://doi.org/10.1016/j.learninstruc.2007.07.001