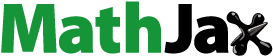
ABSTRACT
The use and the rapid growth of the cobot in industry are changing working conditions. New jobs can imply new advantages and inconveniences, which call for new occupational risk assessments. The aim here is to assess occupational risks in terms of mental stress, so as to determine whether a worker experiences greater stress when working in collaboration with a cobot rather than with another person while performing the same production-line process. The study involved a total of 32 volunteers of various ages, with no previous experience of cobots. An eye-tracker system that records a range of biometric data was used to quantify stress. Pupil diameter was mainly used in this investigation, as well as the number of gaze fixations by zones. The data registered were analyzed using the T-test method, with which data on two groups can be compared to test for significant differences. In addition, other secondary parameters were also analyzed, such as the time required to complete each test, and the number of errors that were committed. Among the most important conclusions, it was noted that working with cobots in no way increased stress levels, confirming one of the objectives for which these robots were designed.
1. Introduction
Industrial production is immersed in a continuous process of development and changes. The demand for both flexible and effective quality processes is nowadays incessant in manufacturing environments. In this context, although there have been some earlier references, the Industry 4.0 concept was first presented at the 2011 Hannover trade fair (Alcácer and Cruz-Machado Citation2019; Vogel-Heuser and Hess Citation2016; Wagner, Herrmann, and Thiede Citation2017; Xu, Xu, and Li Citation2018; Yang Citation2017). It was then officially announced in 2013, as a strategic German initiative to lead the new industrial revolution in which industrial processes are currently immersed (Xu, Xu, and Li Citation2018). Cobots or collaborative robots are robotic devices designed to share the workspace with operators in the absence of a protective barrier or with only limited protective equipment (Bauer, Wollherr, and Buss Citation2008a; Mariscal et al. Citation2019). A comparison between conventional industrial robots and cobots is shown in .
Table 1. Comparison between conventional industrial robots and cobots. Authors’ own assessments. Source: developed from (Djuric, Rickli, and Urbanic Citation2016; Villani et al. Citation2018.).
The fourth industrial revolution, also called Industry 4.0, is a change of paradigm that is based on the convergence in industrial processes of certain technologies. These technologies are: big data, cybersecurity, 3-D printing, collaborative robotics, and augmented reality, among others. In this paper, the technology of collaborative robotics will be studied, in particular their possible occupational risks in terms of occupational stress.
Human-Robot Collaboration (HRC) combines the flexibility of a worker with the accuracy and repeatability of a cobot (Faccio, Bottin, and Rosati Citation2019), in some cases both improving worker productivity and reducing fatigue (Villani et al. Citation2018). In many cases, these devices can be actively used with operators, either sharing the workspace or working together. Many researchers are focusing their work on balanced interaction between humans and cobots under safe working conditions. However, most HRC-related research work has been focused on human collaboration with the tasks of a cobot, rather than on industrial examples (El Zaatari et al. Citation2019).
The installation of a cobot implies some novelty that calls for investigation into the new working conditions that can arise and how those conditions can affect workers. New equipment in the production line will create jobs that are quite unlike traditional ones, which must be analyzed to assess their occupational risks. The range of tasks, options, and solutions for industry is practically infinite. Each specific example might well deserve individual study in accordance with all of the relevant details. Our objective here is therefore to analyze how proper workplace risk-assessment influences total productivity and the operator’s stress and mood, in both cases either positively or negatively, as for example in the study of Arai et al. (Citation2009).
The term HRC (Human-Robot Collaboration) will be used, understanding interaction as acting on someone or something else and in collaboration ‘with others’ or ‘working together with’ (Barbara Citation1996; Bauer, Wollherr, and Buss Citation2008b). The use of ‘co-worker’ for a cobot is considered an inappropriate term.
HRC is one of the characteristics of the new Industry 4.0 initiative, which is consolidating itself as a new paradigm in industrial production. The use of cobots is increasingly observed, especially in factories where cobots replace conventional industrial robots. In the following section, works related to HRC safety are reviewed: comparative models between HRC systems and traditional manual and robotic systems with no human collaboration, efficient collision detection methods for robot manipulators, measurement of electrical effects on the skin, and task ergonomics.
This paper starts with an introduction, after which there is a section on the State of the art that contains three sub-sections: ‘Studies carried out on safety working with collaborative robotics’, ‘Cobot safety and safety regulations’, and ‘Definition of stress and its measurement methods’. ‘Materials and methods’ are then presented, which is subdivided into three sub-sections: ‘Data collection and processing tools to detect signs of stress’, ‘Study variables’, and ‘Design of the experiment’. Then, the three types of results are considered in the results presentation: ‘Sociodemographic parameters of the sample’, ‘Results of pupil diameter and AOI’ and ‘Results of the NASA TLX TEST’. The four final sections are: ‘Discussion’, ‘Funding’, ‘Declaration of interest’, and the ‘Bibliography’.
2. State of art
2.1. Studies carried out on safety working with collaborative robotics
The state of the art in HRC has been analyzed in some recent articles; in safety, applications for industrial environments, examples from research, challenges and limitations, and possible future research and application areas. In 2017 (Robla-Gomez et al. Citation2017), presented a review of some standard regulations and the main safety systems applied to industrial robotic environments: methods using various sensors and sensor fusion systems and for visualization and monitoring of safety areas. An overview of collaborative industrial scenarios, cobot programming technologies categorized as optimization and learning, communication, and relevant research papers on similar aspects were presented in (El Zaatari et al. Citation2019). A review of the HRC-related literature over the 10 years between 2008 and 2017 can be found in (Hentout et al. Citation2019).
The papers that were consulted in preparation for this investigation are summarized in the following table. These papers cover cobot-related topics, most of which are on safety and workplace risks, but also on working models, and reviews.
2.2. Cobot safety regulations
It is necessary to establish classifications between the different safety methods to write the standard. The best way to prevent dangerous interaction between human operators and a cobot is to limit contact between them. However, contact is necessary in a few different operations, for which reason cobots are used. Safety-related research is therefore focused on multiple scenarios: seeking either to limit contact, or reacting to possible contacts, or responding in the safest way possible, once contact has occurred. In parallel, certain regulations provide recommendations, rules, and guides to try to evaluate this type of situation, in order to implement safe solutions. Some industries have implemented solutions with a greater or lesser degree of interaction between humans and cobot. At the same time, researchers are seeking solutions for real safety collaboration in HRC scenarios.
During recent years, some standard regulations related to collaborative robots have been published. ISO/TS 15,066, 2016 (‘ISO/TS 15,066, 2016 Robots and robotic devices – Collaborative robots’ Citation2016), a technical specification published in 2016, is nowadays the reference standard on collaborative robots. The above standard supplements the knowledge on industrial robot safety standards ISO 10,218–1:2008 (ISO/TR 20,218–1 Citation2018) and 10,218–2:2017 (ISO/TR 20,218–2 Citation2017) and complements the four collaborative operating modes described in ISO 10,218:2008 (Mariscal et al. Citation2019).
Standard 15,066 also defines maximum force and pressure values for various body areas both for quasi-static contact (with probability of being clamped) and transient contact (no chance of being clamped). ISO/DIS 21,260, 2018 (21260), Draft International Standard, provides mechanical safety data for physical contacts among active machinery or moving parts of machinery and operators. ISO/TR 20,218–1, 2018 (ISO/TR 20218–1 Citation2018) a technical report published in 2018 provides guidance on the safe design and the integration of robot end-effectors.
During HRC, contact with the skull, ears, eyes, forehead, larynx or face is not allowed (‘ISO/TR 20,218–1, 2018 Robots and robotic devices – Collaborative robots’ Citation2016). Depending on the contact scenario for a HRC risk evaluation, pressure and force values must be estimated and taken into consideration, in order to identify which one should be the limiting factor (‘ISO/TS 15,066, 2016 Robots and robotic devices – Collaborative robots’ Citation2016). In this study some aspects have been taken into account: the potentially most dangerous contact geometry between human and robot, the mass of the end effectors and workpieces, the mass of the moving parts of the robot, and the parts of the human body that can be hit, to establish the maximum programming speed of the robot in accordance with the provisions of ISO/TS 15,066:2016 and considering transient contact in all cases.
2.3. Definition of stress and its measurement methods
Stress is, in medical terms, the response of the body to either physical, mental, or emotional pressures, or combinations thereof. Stress produces chemical changes that speed up the heart rate, raise blood sugar levels, and heighten arterial blood pressure. Stress also produces feelings of frustration, anxiety, anger, and depression. The activities of daily life or certain situations, such as a traumatic event or an illness, can all produce stress. Intense stress or stress that lasts a long time, also referred to as emotional, nervous, and psychic stress, may provoke physical, and mental health problems (National Cancer Institute of the United States Cáncer, Instituto Nacional del, and de los Institutos Nacionales de la Salud de EE. UU Citation2022).
Biologically, stress is the interaction between damage and defense, comparable in Physics to the pressure that represents a reciprocal action between a force and the resistance to that force. In psychological terms stress is an object of study, as it is considered to be a physiological, endocrinal, or psychological response to events or situations that oppress or that are perceived as threatening, provoking symptoms of exhaustion, loss of appetite, weight loss, and other non-specific symptoms (Suárez and Humberto Ramírez-Díaz Citation2020).
Studying the stress levels in the workplace is important to be able to reduce them as far as possible and thereby improve the health of the workers. When novel technologies are implemented, such as cobots, it is not clear what the mental consequences of the human operatives alongside them might be, which can often be damaging in the long term due to mental stress. Through the study, valuable conclusions may be drawn, to design the collaborative work stations in a more beneficial way for the health of the workers, without them undergoing inconvenience, due to a lack of information and experience. There are ergonomic studies on work stations, time cycles, implementation of cobot systems, and stress measurement systems. However, studies on the influence of mental stress at a work station and the variables that may cause it are very scarce. Hence the interest in pursuing research in that area.
The study of mental workload has been explored by many authors in very different settings and through numerous variables. Before talking about the relationship between cognitive workload and pupil size, some examples from the literature are mentioned where the mental workload has been associated with multiple physiological variables.
Researchers have used Heart Rate Variability (HRV) for measuring mental stress, as it has been shown that stress and cognitive processing influences heart rate and HRV (Acharya et al. Citation2006; Landi et al. Citation2018; Taelman et al. Citation2009; Thayer et al. Citation2012). In addition to HRV analysis, other authors have also used cognitive signals, cardiorespiratory measures, brain electrical activity, skin conductance, skin potential (amplitude and number of responses), pupil size, and facial expressions to measure mental stress (Grassmann et al. Citation2017; Ryu and Myung Citation2005; Wilson and Russell Citation2003).
From among the many peripheral physiological measures of neuronal activity, pupil size is probably the easiest to acquire. It is perceptible to the naked eye (also possible with corrective lenses) of the observer and can certainly be measured with eye-tracking technologies (Einhäuser Citation2017; Pedrotti et al. Citation2014). Pupil size can reveal data on cognitive load, degree of attention, arousal, and anxiety among others (Einhäuser Citation2017). Since the early 1960s, it has been known that pupil size is related to cognitive load. Dilation of the pupils has been observed in some studies such as (Boersma et al. Citation1970; Hess and Polt Citation1964) when participants were solving mathematical problems of increasing difficulty. The relationship between pupil size and stress has been experimentally tested in recent studies, all confirming how pupil size increases in stressful situations (Hirt, Eckard, and Kunz Citation2020; Pedrotti et al. Citation2014).
The work presented in (Hirt, Eckard, and Kunz Citation2020) consisted of non-intrusively measuring mental workload using eye-tracking technologies during virtual reality tasks.
3. Materials and methods
The experiment was designed in the context of assembly-disassembly tasks in two different situations: one for a human operator and another for a cobot. One situation between a human operator and a cobot, and the other between two human operators. For each of these situations, a risk evaluation was carried out, in accordance with the ISO/TS 15,066, 2016 (ISO/TS 15066 Citation2016), to program the cobot accordingly, analyzing such factors as the geometries of the workpieces, the possible areas of collision between the moving parts of the cobot and workpiece and the operator. With these considerations in mind, the differences in terms of productivity between each of the different scenarios (parts/hour) and the stress that each worker suffers in each situation were studied.
The operator wore glasses with eye-tracking technology to record pupil diameter and to analyze whether there were significant variations that might indicate lower/higher levels of stress and cognitive load. Pupil size can reveal data on cognitive load, degree of attention, arousal, and anxiety among others. The glasses also record the area where the person places visual attention, in order to know what is attracting greater attention or what is of concern. All the components of stress and emotional impact were reinforced with a questionnaire that was later administered to the participants. In our experiment, we administered the NASA TLX questionnaire (National Aeronautics and Space Administration, Nasa, Citation2020) at the end of each session, to ascertain the subjective perceptions of each student with regard to mental workload.
3.1. Data collection and processing tools to detect signs of stress
Different indicators that can help us to quantify stress were used, in order to assess the possible stress level of each worker.
Pupil diameter. Using an eye-tracker (see ). the pupil diameters of both eyes were tracked during the experiments at a sampling rate of 10 ms. Pupil diameter is one of the main indicators of mental stress and it is a somewhat unintrusive measurement method (Landi et al. Citation2018). The eye-tracker is an electronic device in the form of glasses, equipped with multiple cameras to detect eye-related biometric data. The participant must wear the glasses while participating in the experiment.
Area Of Interest (AOI). Using the same device, the eye-tracker Tobii Pro Glasses 2, it is possible to observe whether a person is fixating on a particular zone of the workplace, as a register (spreadsheet) is obtained in which a fixation on some of the previously established areas of interest is shown with a 1. There will be moments where there is no fixation on any of the areas and moments at which there will be fixations on some of them, but never on more than one at a time.
Heat maps. A heat map highlights the points on an image of the work zone where the visual fixation takes place and at what intensity. It is a useful way to convey where and how often the visual gaze of the subject is directed at an object in an instant. This image is generated from data collected by the eye-tracker.
Maps of gaze routes. All movements of the gaze point are scanned to represent all the points where the gaze is directed with a circle on an image of the work place. These points are consecutively linked by a line along the route and are larger whenever the gaze time on each one is longer. Each point is chronologically numbered (Peruzzini, Grandi, and Pellicciari Citation2018). The image is generated with data by the eye-tracker.
NASA TLX TEST. A subjective test that the participant involved in an experiment has to complete in parts. First, the person is shown some pairs of variables and has to choose the one that generates the highest levels of stress in a job. Then the task on which the experiment is focused is completed. On completion of that task, the participant is administered a questionnaire, in which the presence of each one of the above-mentioned variables is evaluated from the participant’s own point of view in the recently completed tasks. If the experiment is to be repeated on various occasions, in different situations, as our experiment was, then the quantitative questionnaire must be filled in after each situation. Performed in quite a different way, the comparison of variables is only done once and before completing the experiments.
3.2. Study variables
The study variables for our study were obtained from the eye-tracker, which provides a wide range of metrics. Moreover, a better analysis of eye behavior and gaze fixation within each different AOI (see ) was defined, to calculate quantitative eye movement variables (note that the AOIs encompass the map of gaze routes).
Statistical tests were performed to compare measured variables within an AOI for both sample groups. The list of variables considered in this study appears below, which were linked to each participant’s level of cognitive load and visual distraction (Bednarik Citation2005; Murray Citation2000; Tatler et al. Citation2014):
Average fixation duration (total fixation duration divided by interval duration).
Number of fixations.
Average pupil diameter for left and right eyes.
Total time spent in each scenario (task completion duration) in minutes.
Number of errors in each test.
A review of the literature (Barassi and Zhou Citation2012; Fagerland Citation2012; Sedgwick Citation2015) indicated that various parametric and non-parametric tests can be used to contrast several samples. In this experiment, the Mann – Whitney U test, a non-parametric test, was applied to compare the Human-Human and Human-Cobot samples:
Assumptions of both normality and variance are not required.
Observations of both groups are independent of each other.
The Mann-Whitney U test is used to determine whether there are differences in either the distributions or the medians of two samples, respectively dependent on the similarity or the dissimilarity of the shape of the overall distribution. Hence, the research hypothesis for comparing the proposed samples is given below and the test was run at the two-sided significance level, α = 0.05.
H0:
The sample medians are identical (μ1 = μ2).
H1:
The sample medians are different (μ1 ≠ μ2).
The Mann – Whitney U test uses the rank of the ranks of the data rather than their raw values to calculate the statistic for the test. The test statistic for the Mann Whitney U Test is denoted as follows:
where U1 and U2 are defined in the following way:
The critical U value (UCritical) based on sample sizes (n1=n2 = 32) and a two-sided significance level (α = 0.05) is 2. That is, the null hypothesis would be rejected only if Umin ≤2. The Mann – Whitney U test was performed using MATLAB software.
Pupil diameter and heart rate were measured and evaluated against self-reported stress levels using a series of questionnaires. Statistically significant correlations were noted between both variables and the self-reported stress level. Paired-sample t-tests at a significance level of α = 0.05were used, in order to determine whether the changes of the mean values for pupil diameter were significant. Before applying the t-test, the Anderson-Darling test result at a degree of significance of α = 0.05 confirmed that the samples followed a normal distribution. The data were once again analyzed with MATLAB statistical software and it was determined with the help of an algorithm whether there were relevant variations within the samples of both groups that were studied. The initial data registered by the eye-tracker were presented for each experiment in a data column for the diameter of the left eye, another column for the right eye and another column for time. This MATLAB algorithm applied the T-Test to test the level of coincidence of the two data series and to determine whether there were significant or at least significative differences between each one. To do so, a coefficient between 0 and 1 was generated, which if closer to 0 indicated that the data series were very different and if closer to one, indicated that they were very similar. The limit to affirm whether significant differences exist or not was 0.05. The algorithm also generated graphs for the data on pupil diameter. The following shows part of the code:
[h_r,p_r] = ttest2(Pdr_robot,Pdr_human);
[h_l,p_l] = ttest2(Pdl_robot,Pdl_human);
% Show results on screen
disp(‘Right pupil’)
disp(Pdr_robot); disp(Pdr_human)
disp(‘Left pupil’)
disp(Pdl_robot); disp(Pdl_human)
In addition, productivity was studied, which was measured in terms of the time required to complete the task and the error rate in the assembly/disassembly of parts/blocks. In this way, it was possible to determine the speed of a cobot in comparison with a human and whether there was any significant variation in the error rates between both situations.
As a complementary method, each participant was administered the NASA TLX TEST, a subjective test designed to assess workload in relation to the other tests that the participant had completed. Before starting the experiment, the participant was asked to complete the first part of test, then complete the first test with human or robotic assistance, the order of which was changed on each occasion. When the first test was over, the participant was asked to assess the different variables that were involved in the process, and then complete the second test, and once again assess the variables used in the second test. Mental load is not the same as stress, although the results of the NASA TLC test were in that respect used to draw conclusions on the basis of subjective assessments.
3.3. Design of the experiment
In this experiment, a UR3 cobot device from Universal Robots (Universal Robots, Citation2020) was used. It had a payload of 6.6 lbs (3 kg), 6 degrees of freedom with 360-degree rotation on all wrist joints. Its reach was up to 19.7 ins (500 mm). According to ISO/TS 15,066:2016 (‘ISO/TS 15,066, 2016 Robots and robotic devices – Collaborative robots’ Citation2016), there are four main collaborative operations: Safety-Rated Monitored Stop; Speed and Separation Monitoring; Hand-Guided; and Power and Force Limited. It is also common to use these modes together. However, the cobot was programmed to operate in limited power and force mode. Moreover, the cycle time of the cobot had been adjusted in such a way that the worker could perform the task in a timely manner. In this experiment, the cobot was used with a power and force sensor and an electric gripper (see ).
In this research, ultralight (45 g) wearable eye-tracking technology was employed. The eye-tracker was equipped with two cameras and had a sampling rate of 100 Hz. Data recorded by the eye-tracker were analyzed using software, to estimate the participant’s gaze-fixation duration and other parameters.
The experiment consisted of 10 assembly and disassembly tasks (see ), in which the participants were provided with a set of colored parts and were asked to add/remove the parts to/from a base board, closely following the work schedule (list of instructions) on the worktable (see ). As shown in , the experiment was conducted in the workspace (see ) under the two following conditions:
Human-Human cooperation:Tobii Pro Glasses 2 wearable eye-tracker glasses, a worktable, a 45º work platform (Base board) with previously positioned colored parts and a work schedule (list of instructions) placed in front of the worker for use in the test The participants were asked to use the colored parts placed in position and the complementary parts available from an assistant worker.
Human-Cobot interaction test: a test consisting of the cobot and two feeder platforms for supplemental parts that the cobot delivers, as well as Tobii Pro Glasses 2, a work table, a 45º work platform (Base board) with previously assembled colored parts, and a work schedule (list of instructions) placed in front of the worker. The participants were asked to use the colored parts already in position and complementary parts provided by the UR3 robot arm with the power and force sensor and an electric gripper. The cobot clutches the part, positions it near the worker and waits until it is removed, at which moment it detects that the part is no longer held in the gripper and moves away to fetch another part. The most hazardous/critical event is when the cobot delivers a part to the worker. Hence, in the interests of safety, the minimum distance between the workers’ eyes and the cobot gripper was set at no less than 450 mm throughout the experiment (see ). The moment at which the part is removed from the gripper can be in different ways, which can influence the results. So, that part of the programming code where the part is taken from the cobot is detailed below. As we can see in Figure X, the cobot arrives at a point where it will stop, wait until it detects that the part has been transferred (it can do so, because when the part is removed, the gripper is no longer constrained). When the part is removed, the cobot waits 1 second and it then opens the gripper to pick up another part. It waits 1 second so that the removal of the part will not create an alarming situation where the robot reacts immediately and the hand of the operator is close to the device.
In total, 32 people participated in this study. Upon their arrival, the participants received instructions and followed a 5-minute practice session. After the training, participants were asked to wear the Tobii Pro Glasses 2 wearable eye-tracker whilst completing the experiments.
4. Results
4.1. Sociodemographic parameters of the sample
shows a review of papers related to the research topic. A total of 32 volunteers participated in this research, most of whom were engineering teachers and students. The participants had no prior experience of working with cobot and assembly task procedures. A within-users design was adopted for this experiment with each participant carrying out all tasks. The age of the participants ranged from 18 years old, the age of majority, up to 70 years old, an age at which most workers will probably have retired. In , the characteristics of the volunteers can be observed. The sample was divided into age groups for data processing and demographic data were recorded that could be studied as variables that could influence the results.
Table 2. Review of Articles.
Table 3. Participants.
No significant differences were found after having examined the sample of 32 participants. More than 20 participants in an experimental sample is usually a good rule of thumb for interesting results. In addition, it was suggested from a review of the literature that a sample of between 19 and 45 participants was likely to yield significant results (Anita et al. Citation2020; Azimian et al. Citation2021; Bethel et al. Citation2007; Catalina et al. Citation2020).
Aspects such as age, sex, and ambient lighting were overlooked as influential elements, because the same person was tested on each occasion in two different scenarios, though neither sex, nor age, nor ambient lighting changed. When carrying out the experiments with volunteers, each participant performed two different tests: one with human assistance and another with a UR3 as a cobot. The order of the tests was repeatedly changed, in such a way that the initial learning effect was not always on the same test and was uniformly distributed, so that it had no influence on the results. The number of participants was therefore set as a multiple of 2.
4.2. Results of pupil diameter and AOI
When assessing the significant differences, in addition to seeing if the code indicated whether they existed or not, it is worth looking at the coefficient that indicates the intensity of the difference. It is done by evaluating the p> value on the basis of which the null hypothesis may be rejected. The value of alpha is set at the commonly used value of 0.05. If p is greater than alpha, then no relevant variances exist. If p is less than alpha then considerable divergences do exist. It is worth seeing whether p is close to this value of 0.05 before drawing any conclusions.
These values in Matlab coding were p_l and p_r corresponding to the left and to the right pupil, respectively.
Running the code created a graph () for each eye (right and left), in which pupil diameter was represented for all participants and time of each experiment (human-human and human-cobot). In this way, the person-to-person differences for each test could be rapidly observed.
Significant differences can be detected between the two situations with the MATLAB algorithm. The MATLAB algorithm (explained in section 3.2. Study Variables) indicated the absence of any significant differences for pupil diameter data with or without cobot. In other words, collaborating with a cobot rather than another operator had no influence on the stress that was endured.
It is very useful to see a diagram of gaze-fixation zones for a better understanding of gaze behavior, including the gaze-fixation time outside those zones for more complete information. It provides an idea of which zones are given more attention in each of the tests, as may be seen in .
A predominance of ‘No fixations detected’ or ‘Another zone’ can be appreciated.
In the following image (), the distribution and the gaze concentrations may be seen in a graph that is called a ‘heat map’. The greater part are focused on the work instructions, in second place, they are grouped within the zone of the panel and the available parts (the positions of the points on the background image are a little misplaced), in third place in the reception area of the parts that have been supplied and on the cobot itself.
The variation of attention within the different zones was not very high (at around 30%), except within the panel zone where it almost doubled, and in the zone occupied by the assistant, whether the robot or the person’s arm, within which attention was almost multiplied 6 times over. It is interesting to see the changes between both situations; results that are visible in .
The percentage attention within the AOI that circumscribes the robot is reflected in . The fixation percentages of all the AOIs for each participant are shown. The distribution of fixations can therefore be seen in a general way between the zones for all the participants, so that any great variations for different people can be seen.
The number of gazes-fixations per unit of time is something that can reveal tendencies as a function of the type of collaboration. These results are presented in and are commented upon in greater detail in the ‘Discussion’ section.
When the robot intervened, the number of gazes increased by 17%.
In a similar way to the above, tendencies relating to the average duration of fixations can be seen. These results are presented in .
The differences between series were 1.6%, which was not a significant value.
As has been mentioned, one of the main disadvantages of these devices is that they are slower, which is the reason why the data on task duration is presented in .
It was observed that Human-Human collaboration completed the test more rapidly than Human-Robot collaboration. Working with a cobot meant a delay of 9.6% more time.
The errors were manually quantified for one person throughout the experiments. The errors when changing human collaboration for robot collaboration would in principle be of the same type, although the quantity could vary. The errors that were usually committed were ‘not following the work instructions properly’ (placing a part in an incorrect place, forgetting to move a part). The interest in analyzing that aspect is that it might imply poor assembly or quality defects. To do so, the collaboration-related errors are shown in .
The errors increased by 38% when substituting human collaboration for robotic collaboration, which is a relevant percentage that might be of great interest in industry.
4.3. Results of the NASA TLX TEST
With regard to the NASA TLX TEST results, a greater mental load was noted among participants when working with a human, from their point of view. However, rather than a direct quantification of stress, this test quantified mental load, which depended on other factors such as mental demand, physical demand, effort, time demands, performance, and frustration. The NASA TLX TEST results are presented in .
When completing the experiment, some people made it known that they felt under greater pressure when keeping a person rather than a cobot waiting. It might be due to the more impersonal nature of working with machines. Cobots are designed to help humans with the tasks, while humans can develop feelings of frustration and tiredness arising from wait times. Empathy that is a human feeling can reflect the feelings between workers and can influence the stress of a worker causing the delay, given the pressure that the worker may be under.
Age and task duration can be determining factors behind the errors, which is why the results were analyzed as a function of age. The following shows a set of graphs on errors () and the durations of tests according to age ranges.()
An analysis of errors committed as a function of holding university studies also yielded the following result:
average errors per person with university studies: 1.24
Average errors per person with no university studies: 1.25
5. Discussion
In this section, there are three clearly different parts on working with cobots: its influence on the stress of the worker; its influence on productivity; and future lines of research on the basis of the results.
In the first place, the variation of worker stress was measured together with cobots with different tools (pupil diameter, AOI, quantity of gazes, duration in gaze fixations and the NASA TLX TEST), although pupil diameter was the most important.
The Mann-Whitney U test applied to the measurement of pupil diameter yielded results that showed no significant differences with regard to the mental stress that a worker endures when either working collaboratively with another human or working in collaboration with a cobot. A conclusion that was drawn from the most relevant stress indicator of the study (pupil diameter).
According to the AOI analysis, greater attention on the robot than on another person was observed, even though it was not a source of stress. This hypothesis was supported by the graph of ‘Quantity of gazes-fixations by unit of time’, in which the quantity of fixations was greater when working with the robot. It was consistent with the principal theory, because only slight changes were detected.
The quantity of gazes during each test is a parameter that can reveal information on the degree to which nervousness is affecting an individual. The gaze-point of a nervous person will usually change more frequently, although it may also be due to lending greater attention to the robot, without it being a source of stress. The quantity increased with the cobot by 17%, which was a significant value and supported the theory.
A longer duration in the gaze fixations can point to a higher mental load for which reason the mental processing time might increase. In contrast, maintaining the gaze longer on one spot can mean that the volunteers are more tranquil and that they feel more secure carrying out the task. In any case, gaze duration when working with the robot remained practically constant.
It was concluded from the NASA TLX TEST results that working with collaborative robots generated no greater mental load than working with humans, as was found for the other results. There was a 6.7% variation in mental load, which was not a significant value. In addition, stress was only one part of all the factors that constituted mental load, for which reason it was an even less relevant value for mental stress.
In second place, with regard to productivity when working with cobots, it can be seen that the use of cobots somewhat increases the time of a cycle, which is one of the biggest barriers when expanding the use of cobots within industry. It was found that the work time increased when working with a cobot by around 10%, which pointed to an appreciable difference. Data that could be taken into account for production purposes, given the substantial variations and their cost implications for a firm. This result was in accordance with the results of (Andronas et al. Citation2023), who noted a slight increase in cycle times of 1.4%. The percentile variation depends a lot on the work station, though the trend remains.
According to this investigation, more mistakes were made by people working collaboratively with a cobot than by people working with another person. The errors increased significantly (38%) in this case, although the indicator was expected to drop in the case of experiments of longer duration or with greater worker training. Although stress only slightly increased, this point must be highlighted, as to install a cobot in a production process is a very important factor that deserves careful assessment.
In this study, the recommendation of Arai et al. (Citation2009), was followed, who proposed further investigation as a future line of research on factors that affect operator fatigue during assembly processes. Their study affirmed that semi-automation can lead to higher productivity, improving total productivity (50%), and reducing human error. Findings unlike the results of our study, as productivity diminished in our case and the human errors increased with the introduction of HRC, although it was due to the specific design of the experiment.
With regard to future lines of research, four interesting proposals were raised as a function of the results.
In this experiment, the robot was programmed in such a way that it waited at the pick-up point until the part was taken. The fact that the robot waited for the person might be a source of stress. Another alternative situation could be for the robot to wait at another place further away and only when the worker requests the part with an order (for example, with a wifi bracelet) could it be placed at the pick-up point. If this method truly reduces stress, it will be a very interesting development for the industrial sector.
The work of humans with various cobots and even with cobots of various sizes remains to be investigated, to see whether the stress of the worker really increases in those situations.
In reality, a cobot is composed of two main parts: the robotic arm and the end effector. Both parts are likely to influence the worker, for which reason simulation of a work station with different tools has been proposed as a future line of research.
In this experiment, pupil diameter has been used as a principal indicator of stress. It is quite an effective indicator, although others are equally effective that could, if measured, contribute extra reliability. To do so, new measurement instruments can be included in future investigations with which more relevant data may be collected, such as for example pulsations per minute and the electrical charge of the skin. With these two new variables, it will be possible to increase the quality of the conclusions.
Disclosure statement
The authors wish to confirm that there are no known conflicts of interest associated with this publication.
The authors confirm that the manuscript has been read and approved by all named authors and that there are no other persons who satisfied the criteria for authorship but are not listed. The authors further confirm that they have all approved the order of the authors listed in the manuscript.
The authors confirm that they have given due consideration to the protection of intellectual property associated with this work and that there are no impediments to publication, including the timing of publication, with respect to intellectual property. By doing so, they confirm that they have followed the regulations of their institutions concerning intellectual property.
Additional information
Funding
References
- Acharya, U. R., K. P. Joseph, N. Kannathal, C. M. Lim, and J. S. Suri. 2006. “Heart Rate Variability: A Review.” Medical & Biological Engineering & Computing 44 (12): 1031–1051. https://doi.org/10.1007/s11517-006-0119-0.
- Alcácer, V., and V. Cruz-Machado. 2019. “Scanning the Industry 4.0: A Literature Review on Technologies for Manufacturing Systems.” Engineering Science and Technology, an International Journal 22 (3): 899–919. https://doi.org/10.1016/j.jestch.2019.01.006.
- Andrea, R., C. Di Marino, A. Pasquariello, F. Vitolo, S. Patalano, A. Zanella, and A. Lanzotti. 2021. Collaborative Workplace Design: A Knowledge-Based Approach to Promote Human-Robot Integration and Multi-Objective Layout Optimization.
- Andronas, D., E. Kampourakis, G. Papadopoulos, K. Bakopoulou, G. Michalos, P. Stylianos Kotsaris, and S. Makris. 2023. “Towards Seamless Collaboration of Humans and High-Payload Robots: An Automotive Case Study.” Robotics and Computer-Integrated Manufacturing 83:102544. https://doi.org/10.1016/j.rcim.2023.102544.
- Anita, P., M. Paliga, M. M. Pulopulos, B. Kozusznik, and M. W. Kozusznik. 2020. “Stress in Manual and Autonomous Modes of Collaboration with a Cobot.” Computers in Human Behavior 112: 106469. https://doi.org/10.1016/j.chb.2020.106469.
- Arai, T., F. Duan, R. Kato, J. T. C. Tan, M. Fujita, M. Morioka, and S. Sakakibara. 2009. “A New Cell Production Assembly System with Twin Manipulators on Mobile Base.” In Paper presented at the 2009 IEEE International Symposium on Assembly and Manufacturing, Seoul, Korea (South), ISAM.
- Azimian, A., C. A. Catalina Ortega, J. M. Espinosa, M. Á. Mariscal, and S. García-Herrero. 2021. “Analysis of Drivers’ Eye Movements on Roundabouts: A Driving Simulator Study.” Sustainability 13 (13): 7463. https://doi.org/10.3390/su13137463.
- Barassi, M. R., and Y. Zhou. 2012. “The Effect of Corruption on FDI: A Parametric and Non-Parametric Analysis.” European Journal of Political Economy 28 (3): 302–312.
- Barbara, J. G. 1996. “Collaborative Systems AAAI-94 Presidential Address.” AI Magazine 17 (2). https://doi.org/10.1609/aimag.v17i2.1223.
- Barbazza, L., M. Faccio, F. Oscari, and G. Rosati. 2017. “Agility in Assembly Systems: A Comparison Model.” Assembly Automation 37 (4): 411–421. https://doi.org/10.1108/AA-10-2016-128.
- Bascetta, L., G. Ferretti, P. Rocco, H. Ardö, H. Bruyninckx, E. Demeester, and E. Di Lello. 2011. “Towards Safe Human-Robot Interaction in Robotic Cells: An Approach Based on Visual Tracking and Intention Estimation.” In Paper presented at the IEEE International Conference on Intelligent Robots and Systems, San Francisco, CA, USA.
- Bauer, A., D. Wollherr, and M. Buss. 2008a. “Human-Robot Collaboration: A Survey.” International Journal of Humanoid Robotics 5 (1): 47–66. https://doi.org/10.1142/s0219843608001303.
- Bauer, A., D. Wollherr, and M. Buss. 2008b. “Human-Robot Collaboration: A Survey.” International Journal Humanoid Robotics 5:47–66. https://doi.org/10.1142/S0219843608001303.
- Bednarik, R. 2005. Jeliot 3-Program Visualization Tool—Evaluation Using Eye-Movement Tracking.
- Bethel, C. L., K. Salomon, R. M. Robin, and L. B. Jennifer 2007. “Survey of Psychophysiology Measurements Applied to Human-Robot Interaction.” In 16th IEEE International Conference on Robot & Human Interactive Communication, Jeju, Korea (South).
- Bi, Z., Y. Liu, J. Krider, J. Buckland, A. Whiteman, D. Beachy, and J. Smith. 2018. “Real-Time Force Monitoring of Smart Grippers for Internet of Things (IoT) Applications.” Journal of Industrial Information Integration 11:19–28. https://doi.org/10.1016/j.jii.2018.02.004.
- Boersma, F., K. Wilton, R. Barham, and W. Muir. 1970. “Effects of Arithmetic Problem Difficulty on Pupillary Dilation in Normals and Educable Retardates.” Journal of Experimental Child Psychology 9 (2): 142–155. https://doi.org/10.1016/0022-0965(70)90079-2.
- Catalina, C. A., S. García-Herrero, E. Cabrerizo, S. Herrera, F. Mohamadi Santiago García-Pineda, and M. A. Mariscal. 2020. “Music Distraction Among Young Drivers: Analysis by Gender and Experience.” Journal of Advanced Transportation 2020: 6039762. https://doi.org/10.1155/2020/6039762.
- Chandrasekaran, B., and J. M. Conrad. 2015. “Human-Robot Collaboration: A Survey.” Paper presented at the SoutheastCon 2015, April 9-12, 2015.
- Corrales, J. A., F. A. Candelas, and F. Torres. 2011. “Safe Human-Robot Interaction Based on Dynamic Sphere-Swept Line Bounding Volumes.” Robotics and Computer-Integrated Manufacturing 27 (1): 177–185. https://doi.org/10.1016/j.rcim.2010.07.005.
- De Luca, A., and F. Flacco. 2012. “Integrated Control for pHri: Collision Avoidance, Detection, Reaction and Collaboration.” In Paper presented at the Proceedings of the IEEE RAS and EMBS International Conference on Biomedical Robotics and Biomechatronics, Rome, Italy.
- Djuric, A. M., J. L. Rickli, and R. J. Urbanic. 2016. “A Framework for Collaborative Robot (CoBot) Integration in Advanced Manufacturing Systems.” SAE International Journal of Materials and Manufacturing 9 (2): 457–464. https://doi.org/10.4271/2016-01-0337.
- Einhäuser, W. 2017. “The Pupil as Marker of Cognitive Processes.” Cognitive Science and Technology 141–169. https://doi.org/10.1007/978-981-10-0213-7.
- El Zaatari, S., M. Marei, W. Li, and Z. Usman. 2019. “Cobot Programming for Collaborative Industrial Tasks: An Overview.” Robotics and Autonomous Systems 116:162–180. https://doi.org/10.1016/j.robot.2019.03.003.
- Fabrizio, F., and A. De Luca. 2017. “Real-Time Computation of Distance to Dynamic Obstacles with Multiple Depth Sensors.” IEEE Robotics and Automation Letters 2 (1): 56–63. https://doi.org/10.1109/LRA.2016.2535859.
- Faccio, M., M. Bottin, and G. Rosati. 2019. “Collaborative and Traditional Robotic Assembly: A Comparison Model.” International Journal of Advanced Manufacturing Technology 102 (5–8): 1355–1372. https://doi.org/10.1007/s00170-018-03247-z.
- Fagerland, M. W. 2012. “T-Tests, Non-Parametric Tests, and Large Studies—A Paradox of Statistical Practice?” BMC Medical Research Methodology 12 (1): 78.
- Flacco, F., T. Kroeger, A. De Luca, and O. Khatib. 2015. “A Depth Space Approach for Evaluating Distance to Objects.” Journal of Intelligent & Robotic Systems 80 (1): 7–22. https://doi.org/10.1007/s10846-014-0146-2.
- Flacco, F., T. Kröger, A. De Luca, and O. Khatib. 2012. “A Depth Space Approach to Human-Robot Collision Avoidance.” In Paper presented at the 2012 IEEE International Conference on Robotics and Automation, Saint Paul, MN, USA, May 14-18, 2012.
- Grassmann, M., E. Vlemincx, A. von Leupoldt, and O. Van den Bergh. 2017. “Individual Differences in Cardiorespiratory Measures of Mental Workload: An Investigation of Negative Affectivity and Cognitive Avoidant Coping in Pilot Candidates.” Applied Ergonomics 59:274–282. https://doi.org/10.1016/j.apergo.2016.09.006.
- Hentout, A., M. Aouache, A. Maoudj, and I. Akli. 2019. “Human–Robot Interaction in Industrial Collaborative Robotics: A Literature Review of the Decade 2008–2017.” Advanced Robotics 33 (15–16): 764–799. https://doi.org/10.1080/01691864.2019.1636714.
- Hess, E. H., and J. M. Polt. 1964. “Pupil Size in Relation to Mental Activity During Simple Problem-Solving.” Science 143 (3611): 1190–1192. https://doi.org/10.1126/science.143.3611.1190.
- Hirt, C., M. Eckard, and A. Kunz. 2020. “Stress Generation and Non-Intrusive Measurement in Virtual Environments Using Eye Tracking.” Journal of Ambient Intelligence and Humanized Computing. https://doi.org/10.1007/s12652-020-01845-y.
- ISO/TR 20218-1. 2018. “ISO/TR 20218-1: 2018 Robotics – Safety Design for Industrial Robot Systems – Part 1: End-Effectors.” International Organization for Standardization.
- ISO/TR 20218-2. 2017. “ISO/TR 20218-2_2017 Robotics – Safety Design for Industrial Robot Systems – Part 2: Manual Load/Unload Stations.” International Organization for Standardization.
- ISO/TS 15066. 2016. “Robots and Robotic Devices - Collaborative Robots.” 2016 edited by International Organization for Standardization. Geneva, Switzerland: International Organization for Standardization.
- Landi, C. T., V. Villani, F. Ferraguti, L. Sabattini, C. Secchi, and C. Fantuzzi. 2018. “Relieving operators’ Workload: Towards Affective Robotics in Industrial Scenarios.” Mechatronics 54:144–154. https://doi.org/10.1016/j.mechatronics.2018.07.012.
- Lu, L., Z. Xie, H. Wang, L. Li, and X. Xu. 2022. “Mental Stress and Safety Awareness During Human-Robot Collaboration- Review.” Applied Ergonomics 105: 103832. https://doi.org/10.1016/j.apergo.2022.103832.
- Mariscal, M. A., J. González-Pérez, A. Khalid, J. M. Gutierrez-Llorente, and S. García-Herrero. 2019. “Risks Management and Cobots. Identifying Critical Variables.” In Paper presented at the Proceedings of the 29th European Safety and Reliability Conference, Hannover, Germany.
- Murray, N. P. 2000. An Assessment of the Efficiency and Effectiveness of Simulated Auto Racing Performance: Psychophysiological Evidence for the Processing Efficiency Theory as Indexed Through Visual Search Characteristics and P300 Reciprocity. Florida, USA: State University System of Florida.
- National Aeronautics and Space Administration, Nasa. “NASA TLX Task Load Index.” https://humansystems.arc.nasa.gov/groups/TLX/.
- National Cancer Institute of the U.S. Cáncer, Instituto Nacional del, and de los Institutos Nacionales de la Salud de EE. UU. 2022. “NCI Dictionaries.” Accessed 3 of June. Definition of stress - NCI Dictionary of Cancer Terms - NCI.
- Pedrotti, M., M. A. Mirzaei, A. Tedesco, J. R. Chardonnet, F. Mérienne, S. Benedetto, and T. Baccino. 2014. “Automatic Stress Classification with Pupil Diameter Analysis.” International Journal of Human-Computer Interaction 30 (3): 220–236. https://doi.org/10.1080/10447318.2013.848320.
- Peruzzini, M., F. Grandi, and M. Pellicciari. 2018. “How to Analyse the workers’ Experience in Integrated Product-Process Design.” Journal of Industrial Information Integration 12:31–46. https://doi.org/10.1016/j.jii.2018.06.002.
- Ragaglia, M., L. Bascetta, and P. Rocco. 2015. “Detecting, Tracking and Predicting Human Motion Inside an Industrial Robotic Cell Using a Map-Based Particle Filtering Strategy.” In Paper presented at the Proceedings of the 17th International Conference on Advanced Robotics, Istanbul, Turkey, ICAR.
- Robla-Gomez, S., V. M. Becerra, J. R. Llata, E. Gonzalez-Sarabia, C. Torre-Ferrero, and J. Perez-Oria. 2017. “Working Together: A Review on Safe Human-Robot Collaboration in Industrial Environments.” IEEE Access 5:26754–26773. https://doi.org/10.1109/ACCESS.2017.2773127.
- Ryu, K., and R. Myung. 2005. “Evaluation of Mental Workload with a Combined Measure Based on Physiological Indices During a Dual Task of Tracking and Mental Arithmetic.” International Journal of Industrial Ergonomics 35 (11): 991–1009. https://doi.org/10.1016/j.ergon.2005.04.005.
- Sedgwick, P. 2015. “A Comparison of Parametric and Non-Parametric Statistical Tests.” BMJ 350: h2053. https://doi.org/10.1136/bmj.h2053
- Suárez, O. J., and M. Humberto Ramírez-Díaz. 2020. “Estrés académico en estudiantes que cursan asignaturas de Física en ingeniería: dos casos diferenciados en Colombia y México.” Revista científica 39:341–352. https://doi.org/10.14483/23448350.15989.
- Taelman, J., S. Vandeput, A. Spaepen, and S. Van Huffel. 2009. “Influence of Mental Stress on Heart Rate and Heart Rate Variability.” In Paper presented at the 4th European conference of the International Federation For Medical And Biological Engineering, Antwerp, Belgium.
- Tan, J. T. C., Y. Zhang, F. Duan, K. Watanabe, R. Kato, and T. Arai. 2009. “Human Factors Studies in Information Support Development for Human-Robot Collaborative Cellular Manufacturing System.” In Paper presented at the Proceedings - IEEE International Workshop on Robot and Human Interactive Communication, Toyama, Japan.
- Tatler, B. W., C. Kirtley, R. G. Macdonald, K. M. Mitchell, and S. W. Savage. 2014. “The Active Eye: Perspectives on Eye Movement Research.” In Current Trends in Eye Tracking Research, 3–16. Berlin, Germany: Springer.
- Thayer, J. F., F. Åhs, M. Fredrikson, J. J. Sollers, and T. D. Wager. 2012. “A Meta-Analysis of Heart Rate Variability and Neuroimaging Studies: Implications for Heart Rate Variability as a Marker of Stress and Health.” Neuroscience & Biobehavioral Reviews 36 (2): 747–756. https://doi.org/10.1016/j.neubiorev.2011.11.009.
- Universal Robots. “UR3 Collaborative Robot Technical Details.” https://www.universal-robots.com/.
- Villani, V., F. Pini, F. Leali, and C. Secchi. 2018. “Survey on Human–Robot Collaboration in Industrial Settings: Safety, Intuitive Interfaces and Applications.” Mechatronics 55:248–266. https://doi.org/10.1016/j.mechatronics.2018.02.009.
- Vogel-Heuser, B., and D. Hess. 2016. “Guest Editorial Industry 4.0–Prerequisites and Visions.” IEEE Transactions on Automation Science and Engineering 13 (2): 411–413. https://doi.org/10.1109/TASE.2016.2523639.
- Wagner, T., C. Herrmann, and S. Thiede. 2017. “Industry 4.0 Impacts on Lean Production Systems.” Procedia CIRP 63:125–131. https://doi.org/10.1016/j.procir.2017.02.041.
- Wang, L., R. Gao, J. Váncza, J. Krüger, X. V. Wang, S. Makris, and G. Chryssolouris. 2019. “Symbiotic Human-Robot Collaborative Assembly.” Manufacturing Technology 68 (2): 701–726. https://doi.org/10.1016/j.cirp.2019.05.002.
- Wilson, G. F., and C. A. Russell. 2003. “Real-Time Assessment of Mental Workload Using Psychophysiological Measures and Artificial Neural Networks.” Human Factors: The Journal of the Human Factors & Ergonomics Society 45 (4): 635–644. https://doi.org/10.1518/hfes.45.4.635.27088.
- Xu, L. D., E. L. Xu, and L. Li. 2018. “Industry 4.0: State of the Art and Future Trends.” International Journal of Production Research 56 (8): 2941–2962. https://doi.org/10.1080/00207543.2018.1444806.
- Yang, L. 2017. “Industry 4.0: A Survey on Technologies, Applications and Open Research Issues.” Journal of Industrial Information Integration 6:1–10. https://doi.org/10.1016/j.jii.2017.04.005.
- Yu, X. B., S. Zhang, L. Sun, Y. Wang, C. Q. Xue, and B. Li. 2020. “Cooperative Control of Dual-Arm Robots in Different Human-Robot Collaborative Tasks.” Assembly Automation 40 (1): 95–104. https://doi.org/10.1108/aa-12-2018-0264.
- Zacharaki, A., I. Kostavelis, A. Gasteratos, and I. Dokas. 2020. “Safety Bounds in Human Robot Interaction: A Survey.” Safety Science 127. https://doi.org/10.1016/j.ssci.2020.104667.