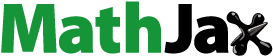
ABSTRACT
Human-robot collaboration (HRC) is putting humans back at the centre of manufacturing via tech-empowered workers. On human-robot assembly line (HRAL), humans and collaborative robots (cobots) can perform tasks collaboratively to improve flexibility and reconfigurability. Most previous work focuses on the production optimization of HRAL yet lacks systematic analysis of the implementation of HRAL. This study investigates factors influencing HRAL implementation: drivers, benefits and barriers, aiming to help practitioners realise suitable application scenarios and overcome implementation challenges. This study defines the features of three important resources on HRAL (humans, robots and cobots) via skillsets and discusses the contribution of these features to the benefits and limitations of HRAL. Furthermore, a novel Triple-I framework is proposed to summarise the implementation barriers of HRAL. The relative importance of these barriers is analysed by Best-Worst Method (BWM), and the impact on various HRAL configurations is assessed using Technique for Order of Preference by Similarity to Ideal Solution (TOPSIS). The results show that critical barriers revolve around the limitations in productivity and accuracy, resulting in safety-rated monitored stop-based HRAL configurations being the most appropriate choice for the automotive industry. Finally, this study offers practitioners with a barrier elimination plan to aid successful HRAL implementation.
1. Introduction
Automation helps companies significantly reduce costs while increasing production. However, as automation continues to grow in the assembly line area, manufacturers realise that human operators are still an integral part of the assembly systems (Faccio et al. Citation2022). Many technology-intensive and mass customisation industries still rely on the sensory mobility of workers for complex assembly tasks (Weckenborg and Spengler Citation2019). The new generation of automated systems is developing in the direction of the human-automation symbiosis (Romero et al. Citation2015) with human-robot collaboration (HRC) receiving increasing attention in recent years. Manufacturers want to bring this collaborative model to the workstations on the assembly line, thereby increasing the level of automation while retaining the advantages of human operators, known as the human-robot assembly line (HRAL). Yet collaboration is not a natural extension of traditional industrial robots as for safety reasons, industrial robots cannot work in the same workspace as humans. The emergence of collaborative robots (cobots) fills the gap between industrial robots and human operators in assembly lines. Cobots first appeared in manufacturing systems in the form of Intelligent assist devices (IADs) designed to improve the safety of industrial robots (Akella et al. Citation1999; Colgate et al. Citation1996). The definition of the HRC assembly mode (Krüger, Lien, and Verl Citation2009) and the risk assessment of the collaborative assembly robot (Matthias et al. Citation2011) allows the cobot to appear as an active resource in the workstation and cooperate with the workers to complete assembly tasks. When robots collaborate on repetitive tasks, humans can focus on processing intellectual and value-adding tasks. The novel collaboration model allows HRAL to have high flexibility and adaptability.
In the context of digital transformation, the integration of HRAL and Industry 4.0 technologies enables safer and more efficient HRC. Machine learning is used to predict worker movements to improve collaboration between cobots and humans (Liu and Wang Citation2017; Wang et al. Citation2018). Wang et al. (Citation2017) proposed a framework of HRAL based on Cyber-Physical Systems (CPS). The combination of cobots and software systems allows the system to assess the safe distance between human workers and cobots in real time (Nikolakis, Maratos, and Makris Citation2019). In addition, Bilberg and Malik (Citation2019) use digital twins to build a virtual model of HRAL for real-time production control.
As HRAL has received more and more attention, several studies on HRAL production optimization have been proposed in recent years (Li, Janardhanan, and Tang Citation2021; Stecke and Mokhtarzadeh Citation2022; Tsarouchi et al. Citation2017). Task allocation strategies in different collaborative assembly scenarios are proposed to achieve efficient utilization of heterogeneous assembly resources (Chen et al. Citation2014; Weckenborg et al. Citation2020). However, due to limited industrial application cases, most studies still use the production objective of the traditional assembly line balance problem. In this context, identifying and addressing critical issues affecting HRAL requires focusing on HRAL implementation cases.
However, there is a lack of research that comprehensively considers the factors influencing the implementation of HRAL. Limited research discusses the impact of introducing HRC technology in the industry, including human factors (Faccio et al. Citation2022), organizational factors (Charalambous, Fletcher, and Webb Citation2015), and success factors (Kopp, Baumgartner, and Kinkel Citation2021). However, whether the advantages of HRC technology can be fully utilized in assembly systems has not yet been investigated. Potential barriers to implementing and operating HRAL remain an open question. The decision problem of assembly line can be divided into Strategic Level, Tactical Level and Operation Level (Dolgui, Sgarbossa, and Simonetto Citation2022). The current research on HRAL is limited to Operation Level (HRC system design) and Tactical Level (assembly line balance problem). To the authors knowledge, there is no literature considering the barriers to the implementation and operation of HRAL, namely the Strategic Level.
From this perspective, the aim of this paper is to systematically investigate the factors that influence the implementation of HRAL and to propose a methodology for evaluating and alleviating the barriers. This will be achieved by addressing the following three research questions.
1.1. Research question 1: what factors currently impact the implementation of HRAL?
This study proposes a framework to define and analyse the different types of factors influencing HRAL implementation and explore ways to eliminate barriers. These factors can be divided into enablers and barriers, according to their positive or negative impact on implementation, while they can also be distinguished into pre-implementation and post-implementation factors, depending on the time of occurrence. As per the aforementioned categorisation, four categories of factors can be defined () as follows: Drivers are associated with the growth potential offered for HRAL from the manufacturing market developments. Benefits represent the positive impact of HRAL on production operations after implementation. The limitations reflect the gap between current technology and industrial needs leading to inadequacies. Both benefits and limitations reflect the technical features inherent in HRAL, referred to as intrinsic factors. In correspondence to this, drivers and challenges emerging from the industrial environment, form the extrinsic factors of the model. These external challenges along with the technical limitations can be considered as barriers to the implementation of HRAL.
1.2. Research question 2: what are the causes of the factors impacting the HRAL implementation and what relationships exist among these diverse factors?
This study proposes a framework based on heterogeneous assembly resources () to analyse the causes of HRAL benefits and limitations. This framework applies a cognitive map to summarise and visualise the causal relationships between component features and system features in HRAL (). This addresses two open questions, (1) how each component feature contribute to the benefits and limitations of HRAL? and (2) Do the benefits of HRAL lead to limitations (side effects)? Through a systematic literature analysis, the proposed framework will help industrial practitioners quickly clarify the correspondence between their overall goals and component resources when implementing HRAL.
For the causes of the barrier factors, an appropriate classification framework helps to analyse and trace implementation barriers to bridge the gap between failures and risk factors (Ranz et al. Citation2018). Malik and Bilberg (Citation2017) proposed a framework to evaluate the decision to deploy collaborative robots. This framework focuses on measuring cobot product selection and workstation design options across different engineering design cycles. Yanco and Drury (Citation2004) propose a descriptive taxonomy for general-purpose human-robot interaction (HRI) systems. On this basis, Ranz et al. (Citation2018) proposed the concept of HRC morphology to quantify HRC design alternatives from five aspects: economics, product, process, system, and safety. Kopp et al. (Citation2021) additionally consider the chronological order of cobot introduction and construct a two-dimensional framework to evaluate the success factors for implementing HRC. From the perspective of implementing HRAL, the existing frameworks and reference models for HRC introduction do not fully summarise the process of deploying assembly lines, because they do not consider factors such as the technology integration process and industrial environment. To fill this gap, this paper proposes a framework that allows practitioners to comprehensively consider barriers in various parts of HRAL implementation to support complex system planning. In the field of accident investigation, the HTO model is used to identify the cause of faults among human workers (H), technology (T) and organization (O). Similarly, HRAL is an industrial system developed around HRC technology. This system includes HRAL technology, human operators, and enterprises. This paper proposes a framework (Triple-I Framework) to consider barriers in various parts of HRAL implementation to support complex system planning comprehensively. The proposed framework defines three categories of the causes of barriers: technical limitations, technology integration, and industrial environments ().
1.3. Research question 3: within practical contexts, how to identify and alleviate key barriers while determining the most suitable HRAL configuration?
The study aims to develop an integrated multi-criteria decision-making model () to explore the key HRAL implementation barriers in the automotive industry sector and support industrial practitioners in selecting suitable HRAL configuration solutions. This is the first usage of the Multi-Criteria Decision-Making (MCDM) technique in the field of HRAL research. Previously, MCDM techniques had found extensive utility within barrier investigation studies spanning various fields. Malek and Desai (Citation2019) employed the Best-Worst Method (BWM) to assess the relative prioritization of barriers linked to sustainable manufacturing. Similarly, Marinelli and Janardhanan (Citation2022) utilized BWM to examine barriers related to green cement production. As the complexity of the problem grows, the integrated MCDM methods are increasingly capturing greater attention. James et al. (Citation2022) integrated BWM and DEMATEL (Decision-Making Trial and Evaluation Laboratory) to probe the challenges in human resource implementation within the context of Industry 4.0. Chang et al. (Citation2019) applied a novel FMEA (Failure Mode Effect Analysis) model based on BWM and TOPSIS (Technique for Order of Preference by Similarity to Ideal Solution) for product risk assessment.
This study aims to use the BWM technique to investigate the relative importance of the barriers to HRAL implementation and evaluate the implementation risks posed by the barriers to different HRAL configurations through the TOPSIS technique, translating industry experience from experts into a concrete plan to alleviate barriers to HRAL implementation.
The rest of this study is organised as follows: Section 2 presents a systematic analysis of the research in the field of HRAL, using a hierarchical structure to present the different types of factors influencing HRAL. The barriers to HRAL implementation will be analysed in Section 3 by an integrated MCDM methodology to explore the prioritisation of the individual barriers and assess the impact of the barriers in different HRAL configurations. Section 4 analyses and explains the implementation results of the proposed methodology in a specific field. Section 5 makes practical recommendations to alleviate the key barriers. Finally, Section 6 presents the conclusions and future scope of this work.
2. Literature Review
Section 2 will analyse the various factors affecting HRAL implementation through a systematic literature review and summarise the relationships of these factors in order to answer research questions 1 and 2.
2.1. Drivers of HRAL
As the manufacturing market diversifies, the assembly sector faces the challenges of shortened product cycles and increasing production. As a result, manufacturing companies need to introduce customised production on the assembly line, which requires good flexibility and variability (Asadi, Jackson, and Fundin Citation2017). However, traditional assembly lines cannot meet these demands. The emergence of HRAL fills this fast-growing niche in the current manufacturing industry.
As consumer demands become more diverse, enterprises need to diversify the assembly-line product while maintaining the advantages of mass production. From manual assembly line (MAL) to fully automated robotic assembly line (RAL), assembly lines are constantly upgraded in their automation level to take advantage of the mass production characteristics. However, fully automated RALs are unable to meet these needs due to their low flexibility. RALs have to adopt more complex production models to meet customisation requirements, which entails increased operating costs (Otto and Li Citation2020). Driven by market pressure, some assembly lines have begun to transform into flexible robotic assembly line (FRAL), such as the introduction of Computer Numerical Controlled machines (CNC) on the assembly line. Customised requirements can be met by pre-programming the CNC by the technician. However, the limitations of FRAL are as follows: (1) Operators are required to have specialised programming skills. (2) The long reconfiguration time of CNC makes it unsuitable for scenarios with diverse product portfolios. These constraints were the driving force behind the transition from FRAL to HRAL which is regarded as an excellent solution for the current trend of customisation. HRAL’s high flexibility simplifies the reconfiguration steps of the assembly line (Hashemi-Petroodi et al. Citation2021), allowing it to face dynamic market demands. presents the four assembly lines’ position on a flexibility-automation map and makes clear the comparative advantage of HRAL.
2.2. Benefits of HRAL
Both MAL and RAL are existing concepts, while HRAL has achieved great growth potential after combining the components of the other two options through collaboration. In theory, this collaboration is the utilization of heterogeneous assembly resources. HRAL’s collaborative model combines the resource skills of human operators and cobots, leading thus to good flexibility and reconfiguration capabilities. This section presents the theoretical background of resource heterogeneity (Castellucci and Costa Citation2012) and analyses the features and benefits of HRAL from the perspective of resource skills.
In early research, the assembly line was often assumed to be a homogeneous line (Malhotra et al. Citation1993), which means the active resources in the station have the same workload and features. This model simplifies the workload assignment problem. In order to adapt to the increasingly complex production requirements, heterogeneous lines begin to be considered (Ikemoto et al. Citation2005) and bring a new definition of workforce assignment. The diverse characteristics of different active resources build a skillset when they work collaboratively (Wittrock Citation1992). The HRAL’s collaborative model is also based on the idea of the heterogeneous assembly line. By adjusting operator-to-station assignment and task-to-station assignment, assembly lines can obtain unique system features. As shown in , a research framework based on heterogeneous resources is proposed. HRAL has two active resources, the human operator and the cobot. Subsection B lists the features of the three skillsets emerging from the active resources on HRAL. In subsection C, these component features are combined into the benefits and limitations of HRAL, namely the system features. A cognitive map () is also proposed to show the causal relationship between HRAL component features and HRAL system features.
2.2.1. Component features
depicts the three skillsets possessed by the two major active resources on HRAL, i.e. the humans and the cobots. The features of these components in HRAL will be discussed in this subsection. First, the skillset of human operators is mainly composed of four features: Cognition, Intelligence, Agility, and Stochasticity. Cognition (H1) makes human operators suitable for jobs requiring judgment and analytical skills. Intelligence (H2) is reflected in two aspects: action planning and learning ability. Planning actions in advance allow human operators to perform complex operations. Learning allows workers to gain experience from repetitive processes, translating into potential efficiency growth (Oberc et al. Citation2019). In addition, the human operator is the most agile (H3) component in the assembly system (ElMaraghy Citation2005) and can be flexibly assigned to different workstations. In the case of MAL, after a cycle time has elapsed, idle human operators move to unfinished workstations to assist, which reduces the bottleneck. However, human behaviour is inherently stochastic (H4). Although the effects of stochasticity can be minimised through training, human-induced bias still cannot be completely eliminated.
The features of cobots can be summarised into two skillsets: the robotic and the collaborative skillset. Previous studies have often overlooked that Cobots only have part of the features of traditional industrial robots because of their different positionings. This means that some features of industrial robots are not applicable in the HRC system. For example, cobots do not have the features of high output and high precision due to safety and collaboration requirements. The robotic skillset includes features that cobots inherit from industrial robots: Strength (R1), Tirelessness (R2), and Repeatability (R3). Robots have to deal with large payloads compared to human operators and can easily assemble large objects. Industrial robots do not feel fatigue and can perform highly repetitive operations around the clock (Hedelind and Jackson Citation2011).
The collaborative skillsets of cobots are designed to allow them to share workspaces with human operators safely. This collaborative skillset includes the following four features: Lightweight, Safe speed, Perception, and Usability. Compared with traditional industrial robots, cobots are smaller and lighter (C1). Under the scenario of the same payload, the maximum working radius of the cobot is only half of that of the industrial robot, and the maximum speed of a single joint is only one-third of that of the industrial robot (C2) (Kopp, Baumgartner, and Kinkel Citation2021). These features allow cobots to collaborate with humans at the right height and at a safe speed.
However, what really distinguishes collaborative robots from industrial robots is the control strategy designed specifically for collaboration. ISO/TS 15,066 defines four types of collaborative strategies for cobots: (1) Safety-rated monitored stop (2) Hand guiding (3) Speed and separation monitoring and (4) Power and force limiting (International Organization for Standardization Citation2016). Safety-rated monitored stop (SMS) means that the cobot will stop to ensure safety when humans enter the work area. Speed and separation monitoring (SSM) means that the cobot will adjust its speed according to the distance from the worker; when a human approaches, the cobot slows down until it reaches SMS. In addition, Power and force limiting (PFL) constrains the contact force threshold values of cobots to ensure safety when humans contact (intentionally or unintentionally) with the cobot arm. This allows the operator to use the Hand guiding (HG) control method to intuitively guide the robotic arm by hand, to plan the path of operation.
The realization of these control strategies is based on the strong perception (C3) of cobots (Wang et al. Citation2019). This is reflected in two aspects (Soter et al. Citation2018): (1) External perception of collaborative robots supporting SMS and SSM. The cobots constantly sense its surrounding environment, including the relative position of the human operator and the target workpiece. External perception allows cobots to intelligently adjust speed or trigger a stop to ensure a safe work environment. (2) Proprietary perception supporting HG and PFL. The proprietary perception is a feedback system for collaborative robots for their own actions. This is mainly reflected in the cobot’s control of its acceleration and force. For potential contact or impact with humans, the joints of the cobot need to feel the pressure and decelerate. In addition, the cooperation-based control strategy makes the collaborative robot have good usability (C4) (Kopp, Baumgartner, and Kinkel Citation2021). Compared with industrial robots, collaborative robots are simple to operate and easy to learn as they are designed to handle pre-set tasks by workers without programming skills. Action commands can be communicated directly to the system via end-of-arm tools or handheld devices (Matheson et al. Citation2019). This allows the collaborative robot to quickly switch presets according to the application scenario to achieve multi-variety production.
2.2.2. System features
Previous studies on HRC features are often limited to enumerating the features of humans, robots, and HRC workstations but lack discussion on the causal relations between these features. Causal relationships here can be divided into two types: (1) Causal relationships between component features and system features, i.e. the contribution of a component’s skillset to HRAL strengths and limitations. (2) Causal relationships between system features, i.e. links between strengths and limitations. This subsection presents a cognitive map to summarise and visualise these two types of causal relationships and discusses the benefits of HRAL in detail based on causal logic. The limitations of HRAL will be analysed in a comprehensive manner in Section 2.3 along with the barriers.
For the first type of causality, as shown in , the main column lists the six system features of HRAL i.e. three benefits (L1, L2, and L3) and three limitations (L4, L5, and L6) while the header row presents the HRAL component skillsets, which were discussed in the previous section. shows the causal relationships between the component features and the system features of HRAL. A tick in a cell indicates that the corresponding component feature supports or affects the system feature.
Table 1. Interrelationship between component features and system features of HRAL.
In addition, the second type of causality, the relationship between the benefits and limitations of HRAL, is also important. Specifically, are there limitations that are side effects accompanying the benefits? However, previous studies have lacked consideration of these side effects. For example, while a collaborative robot gains flexibility and safety, it pays the price in the form of strict limitations in speed and force. These limitations cause collaborative robots to lose many advantages of robotics, such as accuracy, repeatability, and productivity.
In order to analyse these two types of causality comprehensively, this study utilises a cognitive map to visualise the causal relationships between HRAL’s diverse factors and enables HRAL system features to be represented as a combination of the component features. The cognitive map containing the causal relationships of HRAL’s features is presented in . The 11 nodes in the outer circle correspond to the 11 component features of HRAL. Six nodes in the inner circle corresponds to the six system features of HRAL. Where different coloured arrows indicate positive and negative causality. Causal links between component features and system features are indicated by solid arrows, and causal links between system features (between benefits and limitations) are indicated by dashed arrows. Following this subsection, the causal links in will be analysed in detail through the literature analysis. For easier reading and indexing, each causal link is represented below in formula form. For example, H1 + H2 → L1 represents the influence of Cognition (H1) and Intelligence (H2) in the human skillset to improve the flexibility and reconfigurability (L1) of HRAL.
Figure 5. Cognitive map showing the causal relationships between component features and system features of HRAL.
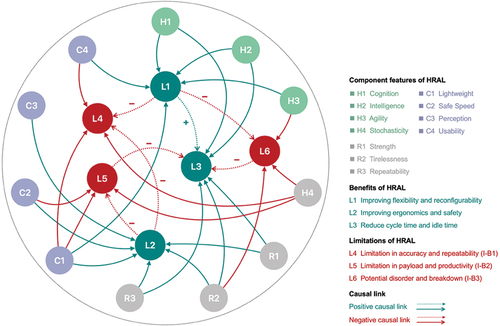
2.2.2.1. L1: Improving flexibility and reconfigurability
As the product portfolio becomes more complex, the industry calls for a flexible manufacturing system. Two concepts have emerged around this issue: flexibility and reconfigurability. Flexible manufacturing is defined as a system that can change its own function and state according to changing requirements with minimal cost (Kara and Kayis Citation2004). On this basis, the reconfigurability of an assembly line refers to the ability of a product line to adjust function and output (Prasad and Jayswal Citation2019). The collaboration of robots and humans represents an exciting next step in flexible manufacturing (Liu et al. Citation2022). The human workers can take on tasks that require high flexibility while robots can handle tasks that require the best use of their speed and strength (Wang et al. Citation2019). Many studies have pointed out the high flexibility of HRAL, but the cause of HRAL flexibility is still poorly discussed (Silva, Simões, and Blanc Citation2022). This paper systematically analyses the contribution of individual characteristics on HRAL to flexibility. The results show that the flexibility and reconfigurability of HRAL come from three aspects: (1) human-centered production; (2) efficient control methods; (3) lightweight assembly resources.
H1 + H2 → L1: HRAL’s flexibility comes primarily from its human-centred work philosophy. Human cognition and intelligence make workers the most flexible resource on the assembly line (Bilberg and Malik Citation2019). Through learning and experience, human operators can quickly adapt to the rapidly changing product mix on the assembly line (Hashemi-Petroodi et al. Citation2020). In contrast, industrial robots need to be reprogrammed and reconfigured to switch to new tasks, which increases the time cost and difficulty of reconfiguration. HRAL utilises human cognition and action planning, making it suitable for complex, highly mixed assembly tasks.
C4 → L1: Furthermore, the intuitive and efficient control of the cobot also provides HRAL with more flexibility. Cobots are much simpler to reconfigure than industrial robots and do not require specialised programming skills. Cobots are designed to operate under the guidance of the operator’s hand, from simple grabbing to complex circuit soldering (Safeea, Bearee, and Neto Citation2017). The guided control method allows the cobot to rely on the operator’s cognition to complete the operation more intuitively, without formulating the operation like the CNC equipment. With human guidance, cobots can perform many complex tasks. Intuitive controls reduce assembly line reconfiguration time and reduce learning costs for training employees.
H3 + C1 → L1: In addition to the above-mentioned functional features, the physical shape of the human and cobot also helps HRAL increase flexibility. The agility, mobility of humans and the small size, lightweight of cobots allow both to be easily assigned and reassigned to any workstation on an assembly line. In addition, the robotic arm of the cobot can quickly assemble different kinds of robotic end-of-arm tooling (EOAT). These unique EOATs enable workstations to perform more tasks that bring value-added attributes to the user. Compatible with multiple types of EOATs, the HRC workstation has a wide range of multitasking capabilities, further enhancing HRAL’s flexibility and reconfiguration capabilities.
2.2.2.2. L2: Improving ergonomics and safety
There are a lot of human and machine interactions in HRAL’s work scenarios. Therefore, the design goal of HRAL is not only to increase the output but also to make the equipment operation and working environment more adaptable to the operator’s needs according to the operator’s physiological and psychological factors (Tan et al. Citation2009).
R3 + R4 → L2: The high number of repetitive assembly tasks on the assembly line exposes workers to significant ergonomic risks. Assembly lines that lack ergonomics can add substantial additional costs to manufacturers. One-third of absenteeism in factories is caused by poor ergonomics each year, and the impact of these injuries is $15 billion to $20 billion (Occupational Safety and Health Administration Citation2000). The robotic features provided by cobots in HRAL, such as Tirelessness and Repeatability, can support human operators to perform highly repetitive and heavy-load assembly tasks, increasing efficiency while reducing the physical burden on humans (Marinelli Citation2022).
C2 + C3 → L2: The collaborative features of cobots fully consider the ergonomics and personal safety of human operators, and they can be divided into two aspects: the safety mechanism at the software level and the appearance design at the hardware level. Safety mechanisms in HRAL ensure that cobots and humans can safely share workstation space. These safety mechanisms are based on the powerful perception of cobots, and the integrated safety system monitors the robot’s internal state, body trajectory, and surrounding environment in real-time (Michalos et al. Citation2015). At the same time, the joints of the cobot limit the speed and force of the robotic arm to a safe range. These joints are fitted with reducers to reduce load inertia or achieve a safe stop.
C1 → L2: Thanks to the lightweight of cobots, the reconfiguration of cobots is very simple compared to traditional robots. This allows cobots to adjust according to human operators’ characteristics and operating habits to create an optimal working environment. For example, cobots should refer to the operator’s height and dominant hand when delivering objects to a human operator. They can help to move materials/parts to the appropriate location and deliver it to human operators with the appropriate force. In addition, the anthropomorphic design of cobots can also help them improve the perceived safety (You et al. Citation2018). Most cobots are close to human size, with sleek joints and skin-friendly surface materials. This friendly appearance makes the cobot appear more like a helper than a competitor, reducing worker distrust and resistance.
2.2.2.3. L3: Reduce cycle time and idle time
H2 + R1 + R3 + R4 → L3: The combination of human intelligence and the characteristics of tirelessness, repeatability, and usability of cobots enables HRAL to compress idle time and reduce cycle time. In the collaboration between cobots and humans, cobots are responsible for increasing workstations’ productivity, allowing human operators to focus on complex assembly tasks that require intelligence. In a survey of HRAL usage within the automotive industry (Olsen and Tomlin Citation2020), BMW manufacturer reduced the idle time of assembly line workers by 85% after introducing HRC to its production lines. Cobots and human operators can be responsible for different tasks simultaneously, and workers’ idle time is effectively reduced (Ren et al. Citation2022). By reducing the physical workload of tasks, these cobots allow human operators to do more work that adds value (Faccio, Bottin, and Rosati Citation2019).
2.3. Barriers of HRAL
The benefits of HRAL are in line with the development trend of the current market. However, the implementation status of HRAL is not optimistic, which forms a clear contrast with its associated benefits (Matheson et al. Citation2019). Therefore, it is meaningful to discuss the barriers to the HRAL implementation.
As shown in , this paper defines three categories of the causes of barriers: technical limitations, technology integration, and industrial environments. Correspondingly, this study proposes a new research framework (Triple-I Framework) for qualitative and quantitative analysis of these barriers. Triple-I refers to Intrinsic, Integration, and Industrial, respectively, correspond to the three causes of barriers to HRAL implementation.
More specifically, (1) Intrinsic barriers refer to the technical limitations of HRAL. These technical limitations will affect the performance of HRAL after implementation, and affect the willingness of enterprises to adopt HRAL. (2) Integration barriers appear in the process of integrating HRAL with existing resources in the factory, including two levels: hardware and software integration. (3) Industrial barriers refer to the impact of the industrial environment on HRAL implementation. Based on the Triple-I framework, 10 identified barriers to HRAL implementation are presented in and will be analysed according to the hierarchical structure.
Table 2. Barriers of HRAL implementation based on the Triple-I framework.
2.3.1. Intrinsic barriers
According to the Triple-I framework, intrinsic barriers arise from the technical limitations of HRAL. Intrinsic barriers can be divided into three categories: (1) The first is the weaknesses of collaborative robots, such as limitations in accuracy and repeatability. The analysis of these barriers helps HRAL to find suitable application scenarios. (2) The second category is derived from HRC safety regulations, such as limitations in payload and productivity. These discussions can help HRAL find the optimal trade-off between competing factors. (3) The third is the limitation of random human behaviour on collaborations, such as potential disorder and breakdown. The analysis of these barriers can point the way for the development and improvement of HRC workstations in the future. This subsection will analyse the different types of intrinsic barriers based on the productivity and flexibility requirements of the assembly system.
2.3.1.1. I-B1: Limitations in accuracy and repeatability
Cobots have undergone many improvements in design to accommodate collaborative operations, but this at the expense of repeatability, which is lower than when traditional industrial robots are employed. In practice, this trade-off is mainly reflected in the choice of the cobot reducer. The reducer is the core component of the robot arm, which determines the precision and load of robots. Most cobots use harmonic reducers which compared with other reducers, they have a small outline, fewer parts and high transmission efficiency. Harmonic reducers allow cobots to have a small joint size and weight and achieve safety requirements of suitable size and low inertia. However, the harmonic reducer’s joint rotational stiffness is low, leading to a low motion accuracy during operation (Hu et al. Citation2022). In addition, the hand-guided controls of cobots allow for intuitive interactions with workers. However, this comes at the cost of not being able to preset exact operations (Safeea, Bearee, and Neto Citation2017; Wang et al. Citation2018). This makes HRAL less repeatable and less accurate than fully automated RAL.
2.3.1.2. I-B2: Limitations in payload and productivity
Since cobots and human operators share a workspace on HRAL, cobots are limited in power and speed to ensure safety. But these limitations also prevent cobots from reaching the level of load and productivity typifying traditional robots. ISO/TS 15,066 (International Organization for Standardization Citation2016) recommends that the impact force of the cobot on the human body should be less than 150N. Therefore, cobots must limit the maximum load to reduce inertia for fast motor response. The limitation of HRAL productivity is mainly due to the safe speed limit of cobots (Mazzocchi et al. Citation2015). Furthermore, HRAL cannot work independently 24/7 like RAL. Although cobots theoretically have the ability to work independently, most tasks on HRAL still rely on the help or supervision of human operators. As a result, the productivity of HRAL is limited by the productivity of human workers and cannot fully utilise the advantages of robots (Bänziger, Kunz, and Wegener Citation2020).
2.3.1.3. I-B3: Potential disorder and breakdown
Collaborative work requires a high degree of collaboration between human operators and cobots. But the randomness of human operation creates potential disorder in HRAL (Stadnicka and Antonelli Citation2019). HRAL’s tasks require great collaboration between the two, so any deviation from the standardised steps by the human operator could lead to disorder in the cobot (Faccio et al. Citation2022). When cooperating with humans, cobots need to have a certain fault tolerance rate, which challenges the robot’s perception and intelligence level. In addition, it is difficult to completely avoid the errors in accuracy and time of human operation, which often makes the operation’s cycle time, unstable (Casalino et al. Citation2019; Kopp, Baumgartner, and Kinkel Citation2021). This instability also adds difficulty to the cobot configuration and the assembly line’s overall balance (Zhang et al. Citation2022).
2.3.2. Integration barriers
HRAL transforms the cobot from an independent workstation into part of a large-scale production process, so the implementation needs to consider integrating existing resources. The integration process includes two parts: (1) Hardware integration: the integration of HRAL with the existing equipment and operators in the factory, involving barrier II-B1 and II-B2. (2) Software integration: the integration of HRAL and existing assembly line balancing algorithms, involving barrier II-B3 and II-B4.
2.3.2.1. II-B1: Lack of corresponding line feeding subsystem
In hardware integration, HRAL first needs to consider integrating the factory’s infrastructure. An assembly line system consists of an assembly subsystem and a feeding subsystem (Battini et al. Citation2009). The role of the assembly line feeding subsystem covers transportation, preparation and item management (Schmid and Limère Citation2019), ensuring that the workstations of the assembly system can accurately receive specific items. The collaboration between the two determines the overall performance of an assembly line system. However, existing feeding policies are not adapted to the way human-robot collaboration works (Aaltonen and Salmi Citation2019). HRAL cannot play its true performance without upgrading the matching assembly line feeding system.
2.3.2.2. II-B2: Lack of universal interaction interface
The operator is an important element on the assembly line. This subsection will discuss the barriers arising in integrating HRAL with the operator. Many collaborative tasks in HRAL have high requirements for the interactive interface between the operator and the cobot. Existing HRC workstations have many interactive interfaces, such as gestures, voice and programming (Gustavsson et al. Citation2018; Tsarouchi, Makris, and Chryssolouris Citation2016). Although all designs are geared towards better collaboration between human operators and cobots, these interactive interfaces are not universal due to differences in language and gestures among individuals. The variety of HRC interactions increases the cost of training employees. This has a significant impact on the introduction of new technology. The industry expects a universal interaction interface to make HRAL operational skills transferable (Kildal et al. Citation2018).
2.3.2.3. II-B3: Lack of datasets to balance HRAL
HRAL is in the early stages of development, and as a result, assembly lines balancing is a challenging task due to the lack of relevant data (Li, Janardhanan, and Tang Citation2021). Especially in the early stage of production, i.e. several months before its start, manufacturers face great uncertainty when conducting assembly line planning (Boysen, Schulze, and Scholl Citation2021). Product design and assembly line planning are carried out simultaneously at this stage. Even if the production time of each task is determined, precise precedence relations are still unavailable, forcing manufacturers to rely on past experience to complete line balancing. With the development of the digital industry though, digital twin technology provides potential solutions to HRAL’s data problems (Kousi et al. Citation2021).
2.3.2.4. II-B4: Complexity in integrating with existing line
The complexity of balancing HRAL was further increased during the integration of HRAL with the existing traditional assembly systems. The reason is that most manufacturers do not want to completely replace existing assembly lines with HRAL to save on upgrade costs. They prefer to adopt the hybrid model, i.e. by introducing part of the HRAL in the existing assembly line or adding HRC workstations to the RAL. This hybrid model meets its need for flexibility and customisation while reducing upgrade costs (Malik and Bilberg Citation2019; Sadrfaridpour, Saeidi, and Wang Citation2016). However, this hybrid HRAL increases the difficulty of configuration, reconfiguration and balancing of the assembly line (Sadrfaridpour, Saeidi, and Wang Citation2016). The resource skills on the assembly line are further complicated, making it more challenging to design operator-to-station and task-to-station allocation strategies.
2.3.3. Industrial barriers
The successful implementation of technology requires a lot of external support. Enterprises need to invest a lot of resources to assist the implementation of HRAL, including intellectual property resources, human resources and financial resources. This section will analyse the external barriers from these three perspectives.
2.3.3.1. III-B1: Lack of standardisation and metrics
Standards are an essential means of technology extension. Technical standards can help manufacturers regulate the working environment and adjust the parameters of the production line. Therefore, it is necessary to develop appropriate standardisation to ensure that HRAL operates compliantly in each and every operational circumstance of different manufacturing industries. Human operators need to rely on new safety standards and regulations in the new collaborative work environment to ensure their safety and avoid potential disorder (Grahn et al. Citation2018). However, it takes much time to re-assess the risks of different scenarios, which will make HRAL unable to play its advantages in adapting to various tasks (Nimawat and Gidwani Citation2021). The lack of standards is also partly due to lengthy regulatory approval processes. A large number of human-robot interactions lead to a protracted approval process for HRAL-related standards, increasing the time cost of HRAL implementation.
2.3.3.2. III-B2: Lack of knowledge and trust among workers
The internal personnel management of companies also influences the implementation of HRAL. Referring to the previous automation implementation cases, lack of consideration of human factors is the main reason for the failure of new technology promotion. A survey showed that more than half of advanced manufacturing technologies (AMT) implementation failures are due to management challenges rather than technical problems of AMT itself (Lewis and Boyer Citation2002). In the process of HRAL implementation, the negative tendencies mainly stem from employees’ unfamiliarity and distrust of new technologies (Kumar, Savur, and Sahin Citation2021), as well as the consequent fear of unemployment and insecurity at work. This negative emotion is exacerbated when there is a lack of communication within the company or when transformation goals are unclear, ultimately leading to personnel management dilemmas such as lower job satisfaction and higher turnover rates (Charalambous, Fletcher, and Webb Citation2015).
2.3.3.3. III-B3: High acquisition and maintenance costs
HRAL is a suitable automation solution for SMEs, but the current cost of acquiring and operating an HRAL system is still too high (Guerin et al. Citation2015). A feature of cobots is low cost, but this is for a single HRC workstation. If the cobot is used in HRAL on a large scale, enterprises must consider not only the cost of large-scale purchases but also the considerable integration costs, accessory costs, and long-term maintenance costs (Cohen, Shoval, and Faccio Citation2019). These additional initial costs play a negative role in the decision to adopt HRAL (Silva, Simões, and Blanc Citation2022). Companies capable of implementing HRAL are mainly from regions with high-cost manufacturing environments. A recent survey titled ‘Global Collaborative Robot (Cobot) Market: Focus on Payloads, Applications and Industries – Analysis and Forecast, 2019–2024’ revealed that 47% of the global cobot market is concentrated in Europe (Sherwani, Asad, and Ibrahim Citation2020). This point is also verified in the case study of HRAL deployment. The manufacturer emphasized that the motivation for investing in HRAL is mainly to enhance the company’s competitiveness with offshore manufacturing (De Backer et al. Citation2018). However, for enterprises outside the high-cost production environment, the premise of these companies introducing HRAL is that the cost after the payback period cannot exceed the equivalent labour cost. If humans can do the same job at a lower price, then introducing HRAL is entirely unattractive. Knudsen and Kaivo-Oja (Citation2020) discuss the path for the cobot market to break through the current geographic distribution. Low-cost collaborative robots may be the key to unlocking labour-intensive markets in the future.
3. Methodology
The vast potential and benefits of HRAL do not match the progress of its implementation in the industry, which shows that there are many unexplored barriers to the implementation of HRAL. However, to the authors’ knowledge, there are no studies addressing this issue. After the systematic literature review, the barriers to HRAL implementation have been subdivided into different scenarios. However, the extent of the impact of different implementation barriers remains opaque. Multi-criteria decision-making (MCDM) technique is designed to evaluate a set of criteria to find optimal alternatives for complex decision problems (Jato-Espino et al. Citation2014). This study aims to utilise MCDM techniques to help industrial practitioners identify key barriers to HRAL implementation and select appropriate HRAL configuration options. By using the HRAL implementation barriers as decision criteria, the proposed integrated MCDM methodology will be applied to evaluate the implementation risk of different HRAL configuration alternatives under the barriers. This will enable industrial practitioners to select the HRAL configuration with the lowest implementation risk.
As shown in , the proposed methodological framework consists of 3 stages. (1) The first stage is to identify the barriers to HRAL implementation and construct a classification framework. This is done through a systematic literature review. (2) in the second stage, BWM analysis will be used to specify the relative importance of potential implementation barriers and TOPSIS analysis will be used to assess the potential risks of implementing HRAL configuration alternatives based on the weights of the barriers. Since the relative importance of barriers is different in different scenarios, the evaluation matrix for the TOPSIS analysis will consider the weights of the barriers provided by BWM in the previous step. (3) In the third stage, this study will propose a strategy to improve and mitigate the key barriers.
3.1. BWM-based ranking of barriers
This study utilises the BWM technique to prioritise a large number of barriers in HRAL implementation. The evaluation will be based on the Triple-I framework proposed earlier: 10 barriers from three categories will be analysed. Considering the large number of barriers involved, this study chose the BWM which compared to other MCDM methods can reduce the number of pairwise comparisons and obtain more consistent results (Rezaei Citation2015). In this study, the BWM method will be implemented through the following structured approach:
3.1.1. Step 1.1: Determine a set of decision criteria
Conduct a systematic literature analysis of studies involving HRAL technology to collect the barriers to its implementation. As shown in , this paper proposes the Triple-I framework to organise the classification of barriers found in the literature. The purpose of classification is to allow barriers that appear in similar scenarios to be analysed under the same category to maintain the independence of factors between different categories.
3.1.2. Step 1.2: Determine a decision-making group
In order to implement the proposed MCDM methodology, experts with extensive relevant experience will be selected to form the decision-making team in this study. This study selected experts with extensive experience in assembly line operation to participate in the MCDM evaluation. The selection of the expert team is based on the purposive snowball sampling method. Respondents were screened by work field and experience to ensure the accuracy and practical value of their opinions. After extensive selection and communication, a decision-making group consisting of six experts with an average of 14 years of relevant experience was established and participated in the study. The details are shown in .
Table 3. Details of experts.
3.1.3. Step 1.3: Determining ranking rounds for multi-criteria
A BWM ranking document is designed and sent to experts for their professional opinion. The authors personally participated in the investigation, briefing experts on the purpose of the study and details of the barriers. Expert opinions were collected through four rounds of evaluation according to the Triple-I framework. The purpose was to limit the ranking criteria for each round to between 3 and 9 so as to reduce the generation of multiple optimal solutions. In the first round of evaluation, experts were asked to rank three main categories of barriers to HRAL implementation. In the subsequent three rounds of evaluation, experts made pairwise comparisons of sub-barriers contained in each of the three main categories of barriers.
3.1.4. Step 1.4: Determine the preference of each criteria
In each round of evaluation, experts were asked to perform pairwise comparisons of each criterion to determine the importance of each barrier. First, the experts identify the barriers that are considered the best (most important) and worst (least important). Next, pair-wise comparisons are made between the other barriers and the best and worst barriers. The comparison process is as follows:
Experts determine the degree to which the most important (best) barrier is superior (more impactful) to other barriers, according to the 1–9 grading scale. The result is represented as a best-to-others (BO) vector, as in EquationEquation (1)
(1)
(1) , where
represents the preference of the best criterion over criterion j (
).
(1)
(1)
Experts determine the degree to which the other barriers are superior to the worst (least important) barriers, according to a grading scale of 1–9. The result is represented as a worst-to-others (WO) vector, as in EquationEquation (2)
(2)
(2) , where
represents the preference of the other criteria over the worst criterion (
).
(2)
(2)
Step 1.5: Find the optimal weights. The condition for optimal weights to appear is for each pair /
or
/
, there exists
/
or
/
. The optimal weights of each criterion can be obtained by solving the equivalent linear programming problem (LPP) (Rezaei Citation2016), shown in EquationEquation (3)
(3)
(3) :
can be seen as an indicator of the consistency of the comparison matrices. When the value of
tends to zero, it indicates that this round of evaluation is more consistent and reliable (Marinelli and Janardhanan Citation2022). The final ranking results were submitted to the decision-making group to explore ways to eliminate barriers to HRAL implementation.
3.2. TOPSIS-based evaluation of barriers under different scenarios
TOPSIS analysis is an MCDM methodology based on compensatory aggregation that allows a set of alternatives to be compared based on multiple criteria (Behzadian et al. Citation2012). This method weighs the impacts of multiple criteria on alternatives. In this paper, TOPSIS analysis is utilised to help industrial practitioners evaluate the impact of each implementation barrier on the different HRAL configuration alternatives. In this approach, defined barriers to HRAL implementation are considered as decision criteria for evaluating the implementation risk of configuration alternatives. In order to synthesise the impacts of the many barriers to implementation, the TOPSIS approach was adopted for this study to achieve a comprehensive evaluation.
3.2.1. Step 2.1 Determine a set of HRAL configuration alternatives
According to ISO/TS 15,066:2016, four types of control strategies for collaborative robots are defined as (1) safety level monitoring for stopping (2) hand guidance (3) speed and separation monitoring and (4) power and force limitation (International Organization for Standardization Citation2016). Based on the consultation and discussion with the expert team, the proposed model will assess four HRAL configuration alternatives containing different collaborative robot control strategies as outlined in .
Table 4. Alternatives of HRAL configuration.
3.2.2. Step 2.2 Obtain an initial evaluation matrix of configuration alternatives
Based on the application scenarios, a set of HRAL configurations are identified as decision alternatives. For each alternative, the expert team will be asked to assess under each barrier, ‘What potential challenges will the barrier cause to the implementation of the alternative?’. The evaluation consists of a numerical value on a scale of 1–10, with larger values representing the greater challenge the barrier poses to the implementation of the current alternative. Referring to Lo et al. (Citation2019)‘s definition of failure severity, lists the linguistic terms for the rating. This creates the initial evaluation matrix of the HRAL configuration alternatives. This evaluation matrix contains four alternatives (M = 4) and 10 criteria (N = 10). The intersection of each alternative and criterion is denoted as , resulting in an initial evaluation matrix
.
Table 5. Linguistic terms and rating (Lo et al. Citation2019).
3.2.3. Step 2.3 Form a weighted normalised decision matrix for configuration alternatives
The evaluation matrix is normalised to obtain the normalisation matrix
. The computation of the normalisation vector
is shown in Equationequation (8)
(8)
(8) . In this study, the relative importance of the different barriers was considered by multiplying the normalisation matrix with the barrier weight
(provided by the BWM analysis) in order to obtain a weighted normalised decision matrix
as shown in EquationEquation (9)
(9)
(9) .
3.2.4. Step 2.4 Calculate the separation distance for each alternative
Based on the weighted normalised decision matrix, the ideal alternative () and the anti-ideal alternative (
) under each barrier criterion (
) can be determined. These reference points serve as benchmarks for evaluating the alternatives’ performance. The distances of alternatives from these points provide a measure of how well an alternative satisfies the criteria relative to these benchmarks. Since the barrier criteria in the proposed model are all negative criteria, this process can be simplified as Equation (10, 11). Separation distances are the distances from each alternative to the ideal alternative
and the anti-ideal alternative
, denoted as
and
. It is obtained by calculating the n-dimensional Euclidean distance as shown in Equations (12, 13).
3.2.5. Step 2.5 Calculate the relative closeness to determine the ranking of the alternatives
Each alternative will be ranked according to its relative proximity . The relative closeness to the anti-ideal alternative is calculated based on Equations (14). The greater the relative proximity, the more desirable the conditions of the alternative,
when and only when the alternative has the best conditions. Finally, the ranking of each HRAL configuration alternative under the barrier criteria can be obtained.
4. Results and discussion
Following the steps in the methodology, this section will delve into the interpretation and significance of the results obtained from the implementation of BWM analysis and TOPSIS analysis.
4.1. BWM implementation results for barrier criteria
Following the structured BWM approach described earlier, the decision group ranked 10 barriers to HRAL implementation from three main categories. In the first round of evaluation, the scores of the six experts for the three categories are presented as the best-to-others matrix () and the others-to-worst matrix (
). Similarly, in the subsequent three rounds of evaluation, the evaluation results of the experts on the 10 sub-barriers are presented as matrices
and
.
After the processing of step 5, the optimal weights of the first round of evaluation are shown in , where the superscript (B) or (W) on the data indicates that the specific criterion is selected as the Best (Worst) criterion by the corresponding expert. The category weights are calculated by aggregating the optimal weights of the six experts. The results show that among the three types of barriers to implementation, Intrinsic barriers (0.510) representing the technical limitations of HRAL are the most important; the second is Integration barriers (0.311) that appear in the process of integrating with existing resources; Industrial barriers caused by the industrial environment barriers (0.180) have relatively low priority. The difference of each category weight is obvious, which shows the rationality of the Triple-I classification framework. At the same time, this study examined the consistency of the data provided by each expert. The mean consistency of this round of evaluations (0.077) is close to 0, demonstrating the reliability of the results.
Table 6. The weights of main categories.
As shown in , in the next three rounds of evaluation, the decision-making team evaluated the sub-barriers under the three categories. After repeating the same BWM approach, the local weights of each sub-barrier in the category class (round) are obtained. The category weights obtained in the first round of evaluation will be used as coefficients to assign each local weight to obtain the global weight of all sub-barriers. Finally, the priority ranking of key sub-barriers to HRAL implementation is shown in .
Table 7. The local weights of barriers.
Table 8. Final ranking of the sub-barriers for implementing HRAL.
The results show that the two barriers that experts consider to be the most important are Limitations in payload and productivity (I-B2: 0.233) and Limitations in accuracy and repeatability (I-B1: 0.212) from the category of Intrinsic barriers. During the evaluation process, four experts from different manufacturing locations flagged Barrier I-B2 as the most important barrier. This shows that HRAL’s productivity limitations have affected its development at multiple levels. Load limitations make it difficult to fully apply HRAL to large assembly tasks. It has become the most significant barrier for HRAL to enter the automobile manufacturing industry, which is currently the largest assembly line market. The interaction analysis of HRAL’s intrinsic features in Section 2.2 shows that the benefits and limitations of HRAL are inherently linked to each other, i.e. component features bring benefits to HRAL and also lead to limitations. These intrinsic technical weaknesses of HRAL are the most fundamental barriers to HRAL implementation, which stem from the design orientation of HRAL. For example, cobots gain flexibility and safety at the cost of severe limitations in speed and strength. These limitations make cobots lose many of the advantages of robots, such as precision, repeatability, and productivity. Since these side effects accompany the benefits of HRAL, the intrinsic barriers are also the most difficult to overcome. However, previous studies lacked consideration of these limitations.
In third and fourth place are Complexity in integrating with existing line (II-B4: 0.119) and Lack of datasets to balance HRAL (II-B3: 0.102) from the category of Integration barriers. At this level, experts from academia and HRC technical departments rated Barrier II-B4 higher, while experts from industry chose Barrier II-B3 as more important. Both of these barriers belong to the software integration level (assembly line balancing problem). The cobots in HRAL are no longer independent individuals, and they are deployed to complete different tasks of the assembly task jointly. Therefore, the assembly line scheduling and balancing algorithms will directly affect the final performance of HRAL.
Fifth place is High acquisition and maintenance costs from Industrial barriers (III-B3: 0.087). A significant reason for the high acquisition cost is that the upstream cobot market is still not fully competitive. Leading companies’ monopoly of technology has increased premiums for high-end cobots. Cobot joints are highly integrated and have many innovative components, such as joint torque sensors, direct-drive motors, and modular decoders. The premium pricing brought by the technical barriers of these core parts eventually leads to an increase in the overall cost of the HRC workstation.
4.2. TOPSIS implementation results for various HRAL configurations
The purpose of implementing TOPSIS is to rank four potential HRAL configurations using implementation barriers as decision criteria. In step 2.1, potential HRAL configurations are identified according to the four categories of collaboration strategies defined by ISO/TS 15,066:2016 as shown in . Following step 2.2, a team of experts are invited to assess the impact of the implementation barriers for the different configuration scenarios. The average evaluation ratings of the six experts will result in an initial evaluation matrix as shown in .
Table 9. Initial evaluation matrix for HRAL configuration alternatives.
Following step 2.3, the initial evaluation matrix was normalised using Equationequation (8)(8)
(8) , which allowed each score to be normalised between 0 and 1. Next, the impact of the evaluation criteria weights on the assessment values will be calculated using EquationEquation (9)
(9)
(9) with reference to the barrier priority weights provided by the BWM analysis. shows the resulting weighted normalised decision matrix. On this basis, the ideal and anti-ideal alternatives under each barrier criterion can be determined through EquationEquations (10
(10)
(10) -Equation11
(11)
(11) ). In , ideal alternatives and anti-ideal alternatives are marked by superscripts (+) or (–) on the data.
Table 10. Weighted normalised decision matrix.
According to step 2.4, the separation distance of each alternative from the ideal and anti-ideal alternatives is calculated using EquationEquations (12(12)
(12) -Equation13
(13)
(13) ). Finally, the relative proximity of each alternative to the anti-ideal alternative was calculated using EquationEquation (14)
(14)
(14) which allows the final ranking to be determined as shown in .
Table 11. Separation distance and relative closeness of alternatives.
The results show that among the four types of HRAL configurations, Configuration 1 Safety-rated monitored stop is the least affected by implementation barriers in the proposed implementation scenario ( = 0.888). The weighted normalised decision matrix in shows that Configuration 1 is considered to be the ideal alternative under the intrinsic barriers (I-B1, I-B2, I-B3) that carry the major weights. This is because the SMS-based configuration chooses the latter in balancing interaction flexibility and power performance, which is valued in the engine and automotive industries. The SMS-based configuration requires the collaborative robot to stop running when a worker enters the workspace, which would allow the adoption of collaborative robots with high loads and low speed limits. At the same time, the traditional monitoring stop mechanism is more stable, guaranteeing high work accuracy and reducing potential breakdowns.
In second place was Configuration 2 Speed and separation monitoring ( = 0.413). The results show that although the SSM configuration performs well under the criteria of intrinsic barriers and industrial environment barriers, SSM is considered as the anti-ideal alternative under the integration barriers (II-B1, II-B2, II-B3 and II-B4). Collaborative robots based on SSM configurations are able to adjust their speed according to the worker’s proximity to ensure safe collaboration. This is a balanced option between performance and safety in many workshop production scenarios. However, in the proposed engine flow production, the varying work speed of the cobot is considered to cause challenges to the integration effort by the experts. This increases the difficulty of balancing assembly line tasks and planning material replenishment, making it difficult to integrate SSM-based workstations with existing assembly lines.
In third and fourth place are Configuration 3 Power and force limiting ( = 0.255) and Configuration 4 Hand guiding (
= 0.161). The results show that the implementation of these two configurations in the proposed scenario will face significant obstacles. Although the PFL configuration and the HG configuration can allow human robots to perform close interactions with high flexibility, the accompanying power and speed limitations also limit the feasible tasks for both configurations in this engine assembly. Along with high acquisition costs, both the PFL configuration and the HG configuration face high implementation risks in the proposed scenario.
The ranking results were resubmitted to the decision-making group for discussion. Following this, specific barrier alleviation plans were developed, based on the importance of each barrier, as discussed in the following section.
5. Practical recommendations
Following the ranking results and expert inputs, this study proposes a three-tiered barrier alleviation plan. The first step is intrinsic balance: a Dynamic Safety Configuration strategy is proposed to address HRAL’s most significant safety-productivity balance conflict. The second step is smart integration, which will discuss how to use Industry 4.0 technologies to help HRAL integrate with existing resources. The third step is external support, which will explore how companies can gain more financial support while exploring the social value of HRAL.
5.1. Intrinsic balance: dynamic safety configuration
The results show that the barriers to HRAL implementation mainly come from intrinsic barriers. These limitations (I-B1, I-B2) make HRAL unable to meet the requirements of some production scenarios, which fundamentally affects the implementation of HRAL. On this issue, the expert panel’s recommendation is as follows: internal balance. HRAL needs to flexibly balance its productivity, flexibility and security according to different usage scenarios. Following this suggestion, this study proposes a dynamic safety configuration strategy for HRAL to solve the existing productivity dilemma. This dynamic safety configuration merges existing collaboration policies (International Organization for Standardization Citation2016) into two workstation modes. Each operating mode has a unique safety configuration, namely Safety-Rated Suspension (SRS) and Distance-Rated Operation (DRO). With the help of the cobot’s perception, the safety configuration of the workstation can be dynamically switched according to the collaboration needs of the current task on the HRAL. The dynamic operation mode enables the HRC workstation to properly release the force and speed restrictions under the premise of ensuring safety and minimise the occurrence of safe stops to balance the safety and productivity of the workstation.
In SRS mode, the cobot will pause as long as a human operator enters the collaborative workspace. In this mode, cobots and humans do not actively move around the workspace at the same time. SRS mode will be triggered in the following two scenarios. The first is the scenario where the worker manually guides the operation of the cobot. At this point, the worker will be in direct contact with the robotic arm. The cobot suspends active operation and is passively guided by the worker to preset or operate. The second is the scenario where the cobot operates independently. At this time, cobots are more like traditional industrial robots. After the worker exits the workspace, the cobot will remove speed and force limitations and be used to move heavy objects or perform repetitive operations at high speed.
DRO mode should be the most common mode for HRAL, as DRO mode allows cobots and human operators to actively move simultaneously in the work area. At this point, the system will limit and adjust the speed of the robotic arm according to the distance. In the specific implementation, the area around the cobot will be divided into different safety areas according to the radius from the robot. When performing cooperative operations, the cobot monitors the relative distance of the human operator and adjusts the running speed according to the location of the worker. When the worker is outside its working radius, the cobot will run at full or preset speed; however, as the worker approaches, the arm speed will gradually decrease to a lower safe speed. With the help of distance-rated monitoring, cobots can properly increase the operating speed while ensuring the safety of workers. This approach helps address the current HRAL dilemma of balancing safety and productivity.
5.2. Smart integration: industry 4.0 technology
The two main barriers in the integration phase are Complexity in integrating with existing line (II-B4) and Lack of datasets to balance HRAL (II-B3). In response to this problem, experts recommend using the support of Industry 4.0 technologies in the integration process of HRAL. With the help of IoT, computer vision and cloud computing, HRAL will be able to solve complex balancing problems (Da Silva et al. Citation2020). The core of Industry 4.0 is data. Various sensors of the HRC workstation provide HRAL with a large amount of raw production data. Thanks to advancements in sensors, there has been a considerable increase in the ways and types of data that can be collected. For example, computer vision and machine learning techniques convert large amounts of real-time visual information into production data or error information (Coupeté, Moutarde, and Manitsaris Citation2015). IoT is built on a data network formed by connecting sensors of different devices. The data will be analysed in real-time by cloud computing and shared across devices on the assembly line. The cobots that join the IoT network can dynamically adjust to the needs of the current assembly line. IoT cobots allow the assembly line to be balanced and planned synchronously so that the assembly and feeding systems can reach the optimal working state of synchronisation.
5.3. External support: discover the social value
The third step in the alleviation plan is to find external support. Although experts consider Industrial barriers a lower priority than the other two categories, there is still one barrier that ranks high, High acquisition and maintenance costs (III-B3). The financial pressure on enterprises is mainly due to the high pricing of upstream cobot manufacturers. Enterprises not only have to bear the acquisition cost but also bear the cost of long-term maintenance and supporting software. Therefore, enterprises generally face difficulties such as long investment return period and large initial financial pressure in the implementation of HRAL. Experts suggest that enterprises explore their own corporate social responsibility and actively seek collaboration with local governments when introducing HRAL technology. Unlike the introduction of a fully automatic assembly line, HRAL’s unique collaboration model avoids a lot of layoffs on the assembly line. The usability of cobots also indirectly reduces barriers to employment and provides more local employment opportunities. The employment value that HRAL brings to the local society makes it an option which can potentially be favoured by the government. Experts believe that companies should work with the government and seek financial incentives from this point. At the same time, strong political commitment also increases the availability of loans. Such benign collaboration can greatly reduce the financial pressure of HRAL in the early stage of implementation.
6. Conclusion
This study provides a systematic investigation of the drivers, benefits and barriers of the HRAL implementation, a highly relevant topic, as the collaborative mode of HRAL can achieve high flexibility and ergonomics, leveraging heterogeneous assembly components. In this paper, distinct skillsets associated with the HRAL features are defined for each one of the assembly components (humans, robots, and cobots). A cognitive map is also developed to effectively represent the links between the assembly line performance features and the individual components’ characteristics, allowing practitioners to understand the role of the various resources on the benefits and limitations of HRAL. Furthermore, a classification framework (Triple-I framework) was proposed to analyse the barriers to HRAL implementation from three different perspectives: technological limitations, technological integration, and industrial environment. This study analyses the input of experts from academia and industry using the integrated MCDM approach to determine the relative importance of different barriers and to assess the impact of these barriers on different HRAL configuration scenarios. The results show that the most significant barriers to HRAL implementation arise from intrinsic limitations associated with payload and productivity as well as accuracy and repeatability, followed by integration barriers and external barriers. The results also indicate that in the automotive industry, the configuration based on Safety-rated monitored stop is the least risky option for implementation, followed by the configuration based on speed and separation monitoring. Configurations based on power and force limiting and hand guiding have a higher implementation risk. This study also proposes a commensurate alleviation plan for the key implementation barriers to provide theoretical support for the successful implementation of HRAL.
The implementation of MCDM on HRAL is a promising and emerging area of research that can help practitioners make efficient scientific decisions on mass production targets. Beyond the prioritisation of barriers addressed in this paper, there are many operational research questions that deserve to be investigated. The implementation of structured analytical evaluations based on subjective assessments is a valuable direction. Especially for scenario-specific factors such as financial and human factors, further implementation of case studies in specific scenarios will expand HRAL implementation research to different industrial sectors. Furthermore, with the different features of HRAL presenting complex, potentially causal interactions, future research could analyse these links using an integrated approach incorporating MCDM with the Interpretive Structural Modelling (ISM) technique, to effectively map the relationships between the various components’ skills and the overall benefits of the HRAL.
Acknowledgments
The authors wish to thank the experts for providing their valuable opinions during the project. The authors would also like to express their appreciation to anonymous referees for their constructive comments. This work is supported in part by China Scholarship Council (CSC) under Grant No. 202106060016.
Disclosure statement
No potential conflict of interest was reported by the author(s).
References
- Aaltonen, I., and T. Salmi. 2019. “Experiences and Expectations of Collaborative Robots in Industry and Academia: Barriers and Development Needs.” Procedia Manufacturing 38:1151–1158. https://doi.org/10.1016/j.promfg.2020.01.204.
- Akella, P., M. Peshkin, E. Colgate, W. Wannasuphoprasit, N. Nagesh, J. Wells, S. Holland, T. Pearson, and B. Peacock 1999, “Cobots for the Automobile Assembly Line”, in Proceedings 1999 IEEE International Conference on Robotics and Automation (Cat. No. 99CH36288C), Vol. 1, pp. 728–733. Detroit, MI, USA.
- Asadi, N., M. Jackson, and A. Fundin. 2017. “Linking Product Design to Flexibility in an Assembly System: A Case Study.” Journal of Manufacturing Technology Management 28 (5): 610–630. https://doi.org/10.1108/JMTM-10-2016-0144.
- Bänziger, T., A. Kunz, and K. Wegener. 2020. “Optimizing Human–Robot Task Allocation Using a Simulation Tool Based on Standardized Work Descriptions.” Journal of Intelligent Manufacturing 31 (7): 1635–1648. https://doi.org/10.1007/s10845-018-1411-1.
- Battini, D., M. Faccio, A. Persona, and F. Sgarbossa. 2009. “Design of the Optimal Feeding Policy in an Assembly System.” International Journal of Production Economics 121 (1): 233–254. https://doi.org/10.1016/j.ijpe.2009.05.016.
- Behzadian, M., S. K. Otaghsara, M. Yazdani, and J. Ignatius. 2012. “A State-Of The-Art Survey of TOPSIS Applications.” Expert Systems with Applications 39 (17): 13051–13069. https://doi.org/10.1016/j.eswa.2012.05.056.
- Bilberg, A., and A. A. Malik. 2019. “Digital Twin Driven Human–Robot Collaborative Assembly.” CIRP Annals 68 (1): 499–502. https://doi.org/10.1016/j.cirp.2019.04.011.
- Boysen, N., P. Schulze, and A. Scholl. 2021. “Assembly Line Balancing: What Happened in the Last Fifteen Years?.” European Journal of Operational Research 301 (3): 797–814. https://doi.org/10.1016/j.ejor.2021.11.043.
- Casalino, A., E. Mazzocca, M. G. Di Giorgio, A. M. Zanchettin, and P. Rocco 2019, “Task Scheduling for Human-Robot Collaboration with Uncertain Duration of Tasks: A Fuzzy Approach”, in 2019 7th International Conference on Control, Mechatronics and Automation (ICCMA), pp. 90–97. Delft, Netherlands.
- Castellucci, P. B., and A. M. Costa. 2012. “Simulation Model for Assembly Lines with Heterogeneous Workers.” arXiv Preprint arXiv: 1211 6406.
- Chang, T.-W., H.-W. Lo, K.-Y. Chen, and J. J. H. Liou. 2019. “A Novel FMEA Model Based on Rough BWM and Rough TOPSIS-AL for Risk Assessment.” Mathematics 7 (10): 874. https://doi.org/10.3390/math7100874.
- Charalambous, G., S. Fletcher, and P. Webb. 2015. “Identifying the Key Organisational Human Factors for Introducing Human-Robot Collaboration in Industry: An Exploratory Study.” The International Journal of Advanced Manufacturing Technology 81 (9): 2143–2155. https://doi.org/10.1007/s00170-015-7335-4.
- Chen, F., K. Sekiyama, F. Cannella, and T. Fukuda. 2014. “Optimal Subtask Allocation for Human and Robot Collaboration within Hybrid Assembly System.” IEEE Transactions on Automation Science and Engineering 11 (4): 1065–1075. https://doi.org/10.1109/TASE.2013.2274099.
- Cohen, Y., S. Shoval, and M. Faccio. 2019. “Strategic View on Cobot Deployment in Assembly 4.0 Systems.” IFAC-Papersonline 52 (13): 1519–1524.
- Colgate, J. E., J. Edward, M. A. Peshkin, and W. Wannasuphoprasit. 1996. “Cobots: Robots for Collaboration with Human Operators.” In ASME international mechanical engineering congress and exposition (Vol. 15281, pp. 433-439). American Society of Mechanical Engineers.
- Coupeté, E., F. Moutarde, and S. Manitsaris. 2015. “Gesture Recognition Using a Depth Camera for Human Robot Collaboration on Assembly Line.” Procedia Manufacturing 3:518–525. https://doi.org/10.1016/j.promfg.2015.07.216.
- Da Silva, V. L., J. L. Kovaleski, R. N. Pagani, J. D. M. Silva, and A. Corsi. 2020. “Implementation of Industry 4.0 concept in companies: empirical evidences.” International Journal of Computer Integrated Manufacturing 33 (4): 325–342.
- De Backer, K., T. DeStefano, C. Menon, and J. R. Suh. 2018. “Industrial Robotics and the Global Organisation of Production .” OECD Science, Technology and Industry Working Papers, No. 2018/03, OECD Publishing, Paris. https://doi.org/10.1787/dd98ff58-en.
- Dolgui, A., F. Sgarbossa, and M. Simonetto. 2022. “Design and Management of Assembly Systems 4.0: Systematic Literature Review and Research Agenda.” International Journal of Production Research 60 (1): 184–210.
- ElMaraghy, H. A. 2005. “Flexible and reconfigurable manufacturing systems paradigms.” International Journal of Flexible Manufacturing Systems 17 (4): 261–276. https://doi.org/10.1007/s10696-006-9028-7.
- Faccio, M., M. Bottin, and G. Rosati. 2019. “Collaborative and traditional robotic assembly: a comparison model.” The International Journal of Advanced Manufacturing Technology 102 (5): 1355–1372. https://doi.org/10.1007/s00170-018-03247-z.
- Faccio, M., I. Granata, A. Menini, M. Milanese, C. Rossato, M. Bottin, R. Minto, P. Pluchino, L. Gamberini, G. Boschetti, and G. Rosati. 2022. “Human Factors in Cobot Era: A Review of Modern Production Systems Features.” Journal of Intelligent Manufacturing 34 (1): 1–22. https://doi.org/10.1007/s10845-022-01953-w.
- Grahn, S., V. Gopinath, X. V. Wang, and K. Johansen. 2018. “Exploring a Model for Production System Design to Utilize Large Robots in Human-Robot Collaborative Assembly Cells.” Procedia Manufacturing 25:612–619. https://doi.org/10.1016/j.promfg.2018.06.094.
- Guerin, K. R., C. Lea, C. Paxton, and G. D. Hager 2015, “A Framework for End-User Instruction of a Robot Assistant for Manufacturing”, in 2015 IEEE international conference on robotics and automation (ICRA), pp. 6167–6174. Seattle, WA, USA.
- Gustavsson, P., M. Holm, A. Syberfeldt, and L. Wang. 2018. “Human-Robot Collaboration–Towards New Metrics for Selection of Communication Technologies.” Procedia CIRP 72:123–128. https://doi.org/10.1016/j.procir.2018.03.156.
- Hashemi-Petroodi, S. E., A. Dolgui, S. Kovalev, M. Y. Kovalyov, and S. Thevenin. 2021. “Workforce Reconfiguration Strategies in Manufacturing Systems: A State of the Art.” International Journal of Production Research 59 (22): 6721–6744. https://doi.org/10.1080/00207543.2020.1823028.
- Hashemi-Petroodi, S. E., S. Thevenin, S. Kovalev, and A. Dolgui. 2020. “Operations Management Issues in Design and Control of Hybrid Human-Robot Collaborative Manufacturing Systems: A Survey.” Annual Reviews in Control 49:264–276. https://doi.org/10.1016/j.arcontrol.2020.04.009.
- Hedelind, M., and M. Jackson. 2011. “How to Improve the Use of Industrial Robots in Lean Manufacturing Systems.” Journal of Manufacturing Technology Management 22 (7): 891–905. https://doi.org/10.1108/17410381111160951.
- Hu, M., H. Sun, L. Liao, and J. He. 2022. “FESM-based approach for stiffness modeling, identification and updating of collaborative robots.” Industrial Robot: The International Journal of Robotics Research and Application, Vol Ahead-Of-Print No Ahead-Of-Print 50 (1): 35–44. https://doi.org/10.1108/IR-02-2022-0042.
- Ikemoto, Y., Y. Hasegawa, T. Fukuda, and K. Matsuda. 2005. “Gradual Spatial Pattern Formation of Homogeneous Robot Group.” Information Sciences 171 (4): 431–445. https://doi.org/10.1016/j.ins.2004.09.013.
- International Organization for Standardization. 2016. “ISO/TS 15066: 2016.” Robots and Robotic Devices—Collaborative Robots.
- James, A. T., G. Kumar, P. Tayal, A. Chauhan, C. Wadhawa, and J. Panchal. 2022. “Analysis of Human Resource Management Challenges in Implementation of Industry 4.0 in Indian Automobile Industry.” Technological Forecasting & Social Change 176:121483. https://doi.org/10.1016/j.techfore.2022.121483.
- Jato-Espino, D., E. Castillo-Lopez, J. Rodriguez-Hernandez, and J. C. Canteras-Jordana. 2014. “A Review of Application of Multi-Criteria Decision Making Methods in Construction.” Automation in Construction 45:151–162. https://doi.org/10.1016/j.autcon.2014.05.013.
- Kara, S., and B. Kayis. 2004. “Manufacturing Flexibility and Variability: An Overview.” Journal of Manufacturing Technology Management 15 (6): 466–478. https://doi.org/10.1108/17410380410547870.
- Kildal, J., A. Tellaeche, I. Fernández, and I. Maurtua. 2018. “Potential users’ Key Concerns and Expectations for the Adoption of Cobots.” Procedia CIRP 72:21–26. https://doi.org/10.1016/j.procir.2018.03.104.
- Knudsen, M., and J. Kaivo-Oja. 2020. “Collaborative Robots: Frontiers of Current Literature.” Journal of Intelligent Systems: Theory and Applications 3 (2): 13–20. https://doi.org/10.38016/jista.682479.
- Kopp, T., M. Baumgartner, and S. Kinkel. 2021. “Success Factors for Introducing Industrial Human-Robot Interaction in Practice: An Empirically Driven Framework.” The International Journal of Advanced Manufacturing Technology 112 (3): 685–704. https://doi.org/10.1007/s00170-020-06398-0.
- Kousi, N., C. Gkournelos, S. Aivaliotis, K. Lotsaris, A. C. Bavelos, P. Baris, G. Michalos, and S. Makris. 2021. “Digital Twin for Designing and Reconfiguring Human–Robot Collaborative Assembly Lines.” Applied Sciences 11 (10): 4620. https://doi.org/10.3390/app11104620.
- Krüger, J., T. K. Lien, and A. Verl. 2009. “Cooperation of Human and Machines in Assembly Lines.” CIRP Annals 58 (2): 628–646. https://doi.org/10.1016/j.cirp.2009.09.009.
- Kumar, S., C. Savur, and F. Sahin. 2021. “Survey of Human–Robot Collaboration in Industrial Settings: Awareness, Intelligence, and Compliance.” IEEE Transactions on Systems, Man, and Cybernetics: Systems 51 (1): 280–297. https://doi.org/10.1109/TSMC.2020.3041231.
- Lewis, M. W., and K. K. Boyer. 2002. “Factors Impacting AMT Implementation: An Integrative and Controlled Study.” Journal of Engineering and Technology Management 19 (2): 111–130. https://doi.org/10.1016/S0923-4748(02)00005-X.
- Li, Z., M. N. Janardhanan, and Q. Tang. 2021. “Multi-Objective Migrating Bird Optimization Algorithm for Cost-Oriented Assembly Line Balancing Problem with Collaborative Robots.” Neural Computing and Applications 33 (14): 8575–8596. https://doi.org/10.1007/s00521-020-05610-2.
- Liu, L., F. Guo, Z. Zou, and V. G. Duffy. 2022. “Application, Development and Future Opportunities of Collaborative Robots (Cobots) in Manufacturing: A Literature Review.” International Journal of Human–Computer Interaction 1–18. https://doi.org/10.1080/10447318.2022.2041907.
- Liu, H., and L. Wang. 2017. “Human Motion Prediction for Human-Robot Collaboration.” Journal of Manufacturing Systems 44:287–294. https://doi.org/10.1016/j.jmsy.2017.04.009.
- Lo, H.-W., J. J. Liou, C.-N. Huang, and Y.-C. Chuang. 2019. “A Novel Failure Mode and Effect Analysis Model for Machine Tool Risk Analysis.” Reliability Engineering & System Safety 183:173–183. https://doi.org/10.1016/j.ress.2018.11.018.
- Malek, J., and T. N. Desai. 2019. “Prioritization of Sustainable Manufacturing Barriers Using Best Worst Method.” Journal of Cleaner Production 226:589–600. https://doi.org/10.1016/j.jclepro.2019.04.056.
- Malhotra, M. K., T. D. Fry, H. V. Kher, and J. M. Donohue. 1993. “The Impact of Learning and Labor Attrition on Worker Flexibility in Dual Resource Constrained Job Shops.” Decision Sciences 24 (3): 641–664. https://doi.org/10.1111/j.1540-5915.1993.tb01296.x.
- Malik, A. A., and A. Bilberg 2017, “Framework to Implement Collaborative Robots in Manual Assembly: A Lean Automation Approach”, Annals of DAAAM & Proceedings, 28th DAAAM International Symposium on Intelligent Manufacturing & Automation - Zadar, Croatia.
- Malik, A. A., and A. Bilberg. 2019. “Collaborative Robots in Assembly: A Practical Approach for Tasks Distribution.” Procedia CIRP 81:665–670. https://doi.org/10.1016/j.procir.2019.03.173.
- Marinelli, M. 2022. “Human–Robot Collaboration and Lean Waste Elimination: Conceptual Analogies and Practical Synergies in Industrialized Construction.” Buildings 12 (12): 2057. https://doi.org/10.3390/buildings12122057.
- Marinelli, M., and M. Janardhanan. 2022. “Green Cement Production in India: Prioritization and Alleviation of Barriers Using the Best–Worst Method.” Environmental Science and Pollution Research 29 (42): 63988–64003. https://doi.org/10.1007/s11356-022-20217-x.
- Matheson, E., R. Minto, E. G. Zampieri, M. Faccio, and G. Rosati. 2019. “Human–Robot Collaboration in Manufacturing Applications: A Review.” Robotics 8 (4): 100. https://doi.org/10.3390/robotics8040100.
- Matthias, B., S. Kock, H. Jerregard, M. Källman, and I. Lundberg 2011, “Safety of Collaborative Industrial Robots: Certification Possibilities for a Collaborative Assembly Robot Concept”, in Proceedings - 2011 IEEE International Symposium on Assembly and Manufacturing, ISAM 2011. Tampere, Finland.
- Mazzocchi, T., A. Diodato, G. Ciuti, D. M. De Micheli, and A. Menciassi 2015, “Smart Sensorized Polymeric Skin for Safe Robot Collision and Environmental Interaction”, in 2015 IEEE/RSJ International Conference on Intelligent Robots and Systems (IROS), pp. 837–843. Hamburg, Germany.
- Michalos, G., S. Makris, P. Tsarouchi, T. Guasch, D. Kontovrakis, and G. Chryssolouris. 2015. “Design Considerations for Safe Human-Robot Collaborative Workplaces.” Procedia CIRP 37:248–253. https://doi.org/10.1016/j.procir.2015.08.014.
- Nikolakis, N., V. Maratos, and S. Makris. 2019. “A Cyber Physical System (CPS) Approach for Safe Human-Robot Collaboration in a Shared Workplace.” Robotics and Computer-Integrated Manufacturing 56:233–243. https://doi.org/10.1016/j.rcim.2018.10.003.
- Nimawat, D., and B. D. Gidwani. 2021. “Prioritization of Barriers for Industry 4.0 Adoption in the Context of Indian Manufacturing Industries Using AHP and ANP Analysis.” International Journal of Computer Integrated Manufacturing 34 (11): 1139–1161.
- Oberc, H., C. Prinz, P. Glogowski, K. Lemmerz, and B. Kuhlenkötter. 2019. “Human Robot Interaction–Learning How to Integrate Collaborative Robots into Manual Assembly Lines.” Procedia Manufacturing 31:26–31. https://doi.org/10.1016/j.promfg.2019.03.005.
- Occupational Safety and Health Administration. 2000. “Ergonomics Program”, Office of the Federal Register. National Archives and Records Administration. United States.
- Olsen, T. L., and B. Tomlin. 2020. “Industry 4.0: Opportunities and Challenges for Operations Management.” Manufacturing & Service Operations Management 22 (1): 113–122.
- Otto, A., and X. Li. 2020. “Product Sequencing in Multiple-Piece-Flow Assembly Lines.” Omega 91:102055. https://doi.org/10.1016/j.omega.2019.03.013.
- Prasad, D., and S. C. Jayswal. 2019. “A Review on Flexibility and Reconfigurability in Manufacturing System.” In: Chattopadhyay, J., Singh, R., Prakash, O. (eds) Innovation in Materials Science and Engineering. Springer, Singapore. https://doi.org/10.1007/978-981-13-2944-9_19
- Ranz, F., T. Komenda, G. Reisinger, P. Hold, V. Hummel, and W. Sihn. 2018. “A Morphology of Human Robot Collaboration Systems for Industrial Assembly.” Procedia CIRP 72:99–104. https://doi.org/10.1016/j.procir.2018.03.011.
- Ren, W., X. Yang, Y. Yan, and Y. Hu. 2022. “The Decision-Making Framework for Assembly Tasks Planning in Human–Robot Collaborated Manufacturing System.” International Journal of Computer Integrated Manufacturing 36 (2): 1–19. https://doi.org/10.1080/0951192X.2022.2081359
- Rezaei, J. 2015. “Best-Worst Multi-Criteria Decision-Making Method.” Omega 53:49–57. https://doi.org/10.1016/j.omega.2014.11.009.
- Rezaei, J. 2016. “Best-Worst Multi-Criteria Decision-Making Method: Some Properties and a Linear Model.” Omega 64:126–130. https://doi.org/10.1016/j.omega.2015.12.001.
- Romero, D., O. Noran, J. Stahre, P. Bernus, and Å. Fast-Berglund. 2015. “Towards a Human-Centred Reference Architecture for Next Generation Balanced Automation Systems: Human-Automation Symbiosis.” In Advances in Production Management Systems: Innovative Production Management Towards Sustainable Growth, edited by S. Umeda, M. Nakano, H. Mizuyama, H. Hibino, D. Kiritsis, and G. von Cieminski, 556–566. Cham: Springer.
- Sadrfaridpour, B., H. Saeidi, and Y. Wang 2016, “An Integrated Framework for Human-Robot Collaborative Assembly in Hybrid Manufacturing Cells”, in 2016 IEEE international conference on automation science and engineering (CASE), pp. 462–467. Fort Worth, TX, USA.
- Safeea, M., R. Bearee, and P. Neto 2017, “End-Effector Precise Hand-Guiding for Collaborative Robots”, in Iberian Robotics conference, pp. 595–605. Sevilla, Spain.
- Schmid, N. A., and V. Limère. 2019. “A Classification of Tactical Assembly Line Feeding Problems.” International Journal of Production Research 57 (24): 7586–7609. https://doi.org/10.1080/00207543.2019.1581957.
- Sherwani, F., M. M. Asad, and B. S. K. K. Ibrahim 2020, “Collaborative Robots and Industrial Revolution 4.0 (IR 4.0)”, in 2020 International Conference on Emerging Trends in Smart Technologies (ICETST), pp. 1–5. Karachi, Pakistan.
- Silva, A., A. C. Simões, and R. Blanc 2022, “Criteria to consider in a decision model for collaborative robot (cobot) adoption: A literature review”, in 2022 IEEE 20th International Conference on Industrial Informatics (INDIN), pp. 477–482. Perth, Australia.
- Soter, G., A. Conn, H. Hauser, and J. Rossiter 2018, “Bodily Aware Soft Robots: Integration of Proprioceptive and Exteroceptive Sensors”, in 2018 IEEE International Conference on Robotics and Automation (ICRA), pp. 2448–2453. Brisbane, QLD, Australia.
- Stadnicka, D., and D. Antonelli. 2019. “Human-Robot Collaborative Work Cell Implementation Through Lean Thinking.” International Journal of Computer Integrated Manufacturing 32 (6): 580–595. https://doi.org/10.1080/0951192X.2019.1599437.
- Stecke, K. E., and M. Mokhtarzadeh. 2022. “Balancing Collaborative Human–Robot Assembly Lines to Optimise Cycle Time and Ergonomic Risk.” International Journal of Production Research 60 (1): 25–47. https://doi.org/10.1080/00207543.2021.1989077.
- Tan, J. T. C., Y. Zhang, F. Duan, K. Watanabe, R. Kato, and T. Arai 2009, “Human Factors Studies in Information Support Development for Human-Robot Collaborative Cellular Manufacturing System”, in RO-MAN 2009-The 18th IEEE International Symposium on Robot and Human Interactive Communication, pp. 334–339. Toyama, Japan.
- Tsarouchi, P., S. Makris, and G. Chryssolouris. 2016. “Human–Robot Interaction Review and Challenges on Task Planning and Programming.” International Journal of Computer Integrated Manufacturing 29 (8): 916–931. https://doi.org/10.1080/0951192X.2015.1130251.
- Tsarouchi, P., A. S. Matthaiakis, S. Makris, and G. Chryssolouris. 2017. “On a Human-Robot Collaboration in an Assembly Cell.” International Journal of Computer Integrated Manufacturing 30 (6): 580–589. https://doi.org/10.1080/0951192X.2016.1187297.
- Wang, L., R. Gao, J. Váncza, J. Krüger, X. V. Wang, S. Makris, and G. Chryssolouris. 2019. “Symbiotic Human-Robot Collaborative Assembly.” CIRP Annals 68 (2): 701–726. https://doi.org/10.1016/j.cirp.2019.05.002.
- Wang, X. V., Z. Kemény, J. Váncza, and L. Wang. 2017. “Human–Robot Collaborative Assembly in Cyber-Physical Production: Classification Framework and Implementation.” CIRP Annals - Manufacturing Technology 66 (1): 5–8. https://doi.org/10.1016/j.cirp.2017.04.101.
- Wang, W., R. Li, Z. M. Diekel, Y. Chen, Z. Zhang, and Y. Jia. 2018. “Controlling Object Hand-Over in Human–Robot Collaboration via Natural Wearable Sensing.” IEEE Transactions on Human-Machine Systems 49 (1): 59–71. https://doi.org/10.1109/THMS.2018.2883176.
- Wang, P., H. Liu, L. Wang, and R. X. Gao. 2018. “Deep Learning-Based Human Motion Recognition for Predictive Context-Aware Human-Robot Collaboration.” CIRP Annals 67 (1): 17–20. https://doi.org/10.1016/j.cirp.2018.04.066.
- Weckenborg, C., K. Kieckhäfer, C. Müller, M. Grunewald, and T. S. Spengler. 2020. “Balancing of Assembly Lines with Collaborative Robots.” Business Research 13 (1): 93–132. https://doi.org/10.1007/s40685-019-0101-y.
- Weckenborg, C., and T. S. Spengler. 2019. “Assembly Line Balancing with Collaborative Robots Under Consideration of Ergonomics: A Cost-Oriented Approach.” IFAC-Papersonline 52 (13): 1860–1865. https://doi.org/10.1016/j.ifacol.2019.11.473.
- Wittrock, R. J. 1992. “Note—Operator Assignment and the Parametric Preflow Algorithm.” Management Science 38 (9): 1354–1359. https://doi.org/10.1287/mnsc.38.9.1354.
- Yanco, H. A., and J. Drury 2004, “Classifying Human-Robot Interaction: An Updated Taxonomy”, in 2004 IEEE international conference on systems, man and cybernetics (IEEE Cat. No. 04CH37583), Vol. 3, pp. 2841–2846. The Hague, Netherlands.
- You, S., J.-H. Kim, S. Lee, V. Kamat, and L. P. Robert Jr. 2018. “Enhancing Perceived Safety in Human–Robot Collaborative Construction Using Immersive Virtual Environments.” Automation in Construction 96:161–170. https://doi.org/10.1016/j.autcon.2018.09.008.
- Zhang, R., Q. Lv, J. Li, J. Bao, T. Liu, and S. Liu. 2022. “A Reinforcement Learning Method for Human-Robot Collaboration in Assembly Tasks.” Robotics and Computer-Integrated Manufacturing 73:102227. https://doi.org/10.1016/j.rcim.2021.102227.