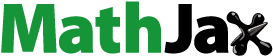
ABSTRACT
Several philosophers and psychologists have characterized belief in conspiracy theories as a product of irrational reasoning. Proponents of conspiracy theories apparently resist revising their beliefs given disconfirming evidence and tend to believe in more than one conspiracy, even when the relevant beliefs are mutually inconsistent. In this paper, we bring leading views on conspiracy theoretic beliefs closer together by exploring their rationality under a probabilistic framework. We question the claim that the irrationality of conspiracy theoretic beliefs stems from an inadequate response to disconfirming evidence and internal incoherence. Drawing analogies to Lakatosian research programs, we argue that maintaining a core conspiracy belief can be Bayes-rational when it is embedded in a network of auxiliary beliefs, which can be revised to protect the more central belief from disconfirmation. We propose that the (ir)rationality associated with conspiracy belief lies not in a flawed updating method, but in their failure to converge toward well-confirmed, stable belief networks in the long run. This approach not only reconciles previously disjointed views, but also points toward more specific hypotheses explaining why some agents may be prone to adopting beliefs in conspiracy theories.
1. Introduction
Over the course of the past decade, there has been an explosion of research on belief in conspiracy theories (henceforth CTs; see Goreis & Voracek, Citation2019 for an overview), reflecting an urgency to understand the phenomenon. This mounting pressure is motivated by the presence of conspiracy theorizing in public discourse, the potential of social media for spreading such beliefs, the associated erosion of trust in epistemic authorities, and the role that these factors play in spreading skepticism regarding the official narrative about the ongoing COVID-19 pandemic.
Despite the heightened interest, there remains little consensus about the nature and (ir)rationality of CT beliefs, e.g., what makes such beliefs intuitively “bad” or “good”, with existing attempts to explain key features of such beliefs being highly fragmented. Common points of contention concern the epistemic justification of such beliefs given their apparent resistance to counterevidence (Harris, Citation2018; Keeley, Citation1999; Napolitano et al., Citation2021), lack of falsifiability (Feldman, Citation2011) and truth-aptness (Cassam, Citation2019; but see Hagen, Citation2022), and the extent to which such theories, insofar as they belong to a general category (Stokes, Citation2016), are explanatory at all (Butter, Citation2021; Fenster, Citation2008). Attempts to understand the psychological factors that may contribute to people’s endorsement of beliefs in CTs are likewise inconclusive as to whether they might result from irrational reasoning (e.g., Cichocka et al., Citation2016; Douglas et al., Citation2019; van Prooijen & van Vugt, Citation2018). For example, CT beliefs tend to highly correlate (i.e., people who believe in one CT tend to believe in others), even when they are semantically and logically unrelated (T. Goertzel, Citation1994a), or mutually inconsistent (Wood et al., Citation2012). From these perspectives, the problem with beliefs in CTs is not their disregard for the evidence per se, but their monological nature, an aspect that has also been visible in recent analyses of COVID-19 CTs (Miller, Citation2020).
Contrary to these negative attitudes, some philosophers and psychologists tend to argue on diverse grounds in favor of certain epistemic and psychological benefits that might make such beliefs a source of rational reasoning. From these latter perspectives, belief in CTs is often responsive to the available evidence (Levy, Citation2021; Suthaharan et al., Citation2021), sometimes poses the best explanation of the events (Dentith, Citation2016), and may even lead to the truth (Dentith, Citation2019). Such views highlight the subjective importance of background beliefs as well as the relevance of sociocultural structures for evaluating the explanatory status of belief in CTs on a case-by-case basis (see also Basham, Citation2016).
Our aim in this paper is to explore the rationality of belief in conspiracy theories in more depth from the perspective of Bayesian cognitive science. Instead of preemptively accepting a view on whether such belief is rational or irrational, we begin by asking under what formal conditions such belief would become irrational in the first place.Footnote1 Our main aim is to clarify the debate by making these conditions more precise through the use of tools from Bayesian analysis in cognitive psychology and philosophy of science. Our hope is that this will offer a shared platform on which previously disjointed views can be brought closer together. Specifically, we focus on how agents incorporate new information to update their beliefs, and we argue that the implicit background structures can be seen as playing the role of auxiliary hypotheses which, under certain conditions, can be rightly discarded to protect core beliefs. Building on works from Strevens (Citation2001) and Gershman (Citation2019), we analyze the structure and rationality of belief in CTs in analogy to Lakatosian research programs and explain the robustness of high-probability beliefs to disconfirmation by counterevidence in reference to low-probability beliefs that can easily be discarded to protect core beliefs. We suggest that, if belief in conspiracy theories should be deemed irrational at all, it is not because of a failure to revise beliefs given disconfirming evidence. Rather, we consider the initial biases and assumptions that guide agents’ inferences as a crucial point of departure to make sense of the correlations between apparently incoherent belief systems and people’s apparent unresponsiveness to evidence contrary to what these systems entail.
Our treatment is, therefore, compatible with previous attempts to elucidate the rationality of CT belief in terms of their protective psychological nature. However, while several of these views have been presented as being “Bayes-like” or “Bayesian-compatible” (e.g., Dentith, Citation2016; Levy, Citation2019;Napolitano et al., Citation2021), none of them offers a formal analysis of the protective nature associated with belief in CTs.Footnote2 A further advantage of our account is that it is highly unifying; as we show, it reconciles the traditional and the higher-order framing of the monological belief view, bringing previously disjointed views on belief in CTs within a single formal framework. By unifying the two views, our aim is to provide a formal platform for proponents of either of these views to identify common factors deemed relevant to evaluate belief in CT. Finally, while Bayesian models in cognitive science have previously been appreciated for their high unificatory credentials (Colombo & Hartmann, Citation2017), this has not been shown for the domain of belief in CTs. We therefore think that this is also an interesting case study for proponents of Bayesian cognitive science.
Introductory remarks in place, we will begin our analysis by outlining the two contrastive views about the nature of belief in CTs in section 2. In section 3, we outline the Bayesian treatment of the relationship between networks of associated beliefs and disconfirmatory evidence. In section 4, we apply this analysis to a received negative characterization of conspiracy belief, while focusing on its evidence-responsiveness. Section 5 shows how our treatment unifies the two views, while section 6 clarifies the extent to which the Bayesian treatment provides a benchmark to identify and assess the degrees of epistemic rationality associated with belief in CTs. Section 7 illustrates this with a set of inductive biases that act as the possible sources of the formation of belief in CTs. We end with a brief conclusion concerning implications for future research in epistemology, psychology, and cognitive science.
2. (Ir)rationality of conspiracy belief and its sources
Negative characterizations of CT beliefs stress the doxastic structure of such beliefs and their epistemic support or lack thereof. The commonly alleged sources of irrationality associated with CT beliefs are:
the monological nature of CT beliefs, which mutually support one another to form a self-sustaining network (T. Goertzel, Citation1994a; B. Goertzel, Citation1994b); under some construals, this network might even contain mutually inconsistent beliefs that are individually supported by a broader higher-order belief (Wood et al., Citation2012); and
the insensitivity of CT beliefs to disconfirmation, which appeals either to the beliefs’ insensitivity to criticism (and even reframing it as supporting evidence, see Keeley, Citation1999Footnote3) or to the beliefs’ self-insulation, postulating that CT beliefs are isolated from disconfirming evidence and other doxastic states (Napolitano & Reuter, Citation2021).
The first feature, (1), assumes that belief in conspiracy theories is emblematic of a reasoning style in which a set of beliefs comprises a self-sustaining network of contents that mutually support each other in order to form a coherent explanation of contingent phenomena that could be otherwise difficult to explain or would threaten the cohesiveness of the existing belief system. Conspiracy theorists are said to represent a monological reasoning style because those who believe in one conspiracy theory are more likely to endorse beliefs in other conspiracies (T. Goertzel, Citation1994a), “even when they refer to completely unrelated events and protagonists” (Sutton and Douglas Citation2014, p. 255). As Benjamin Goertzel, who coined this term, explains, a monological belief system is “a belief system which speaks only to itself, ignoring its context in all but the shallowest respects” (Citation1994b, p. 186). Ted Goertzel adds that “in a monological belief system, each of the beliefs serves as evidence for each of the other beliefs” (Citation1994, p. 740). What is crucial for this account is that monological beliefs are opposed to dialogical ones in which evidence for different beliefs is examined in independent contexts.
However, the notion of monologicality at the center of this view is not as clear as its proponents tend to assume. Critics point out that there seems to be few concrete details about what monologicality consists in, other than the fact that believing in one conspiracy theory is predictive of belief in other conspiracy theories (Franks et al., Citation2017). Hagen (Citation2018, p. 316) evaluates this predictive feature as obvious and unsurprising, arguing that in many cases, the relevant conspiracy beliefs are in fact epistemically related via mediating beliefs (e.g., the belief that the authorities are deceptive). We generally agree with this criticism of the monological view, but also think that it misses a crucial point suggested by Goertzel’s initial contrast to dialogical systems. Goertzel’s distinction, as we understand it, is that in monological systems, evidence in favor of one claim is taken as indirect evidence for a separate claim, while in dialogical systems, the two claims are mutually independent, and so there is no such indirect evidence. Thus, the relevant difference between “monological” and “dialogical” belief systems, as originally presented, seems to lie in their internal structures. A consequence of the stronger dependencies in monological systems is that certain beliefs (e.g., that the UK authorities are deceptive) will be supportive of a diverse range of more specific beliefs (e.g., that Lady Diana faked her funeral, that there were two concurrent assassination attempts at JFK, etc.), providing room for wild or relatively unconstrained inferences. In contrast, dialogical belief systems consider a broader range of independent beliefs (e.g., that the UK authorities are deceptive, but this may have nothing to do with the deceptive character of other authorities). Together, these assumptions in their combination allow for fewer consequences, and so they are supportive of only a narrow range of specific beliefs (e.g., that Lady Diana faked her funeral, but not that there were two concurrent assassination attempts at JFK). Later (section 7), we discuss different inductive biases that may account for differences in the initial set-up of such belief systems. For instance, a strong preference for sparse and deterministic beliefs might foster the development of a monological belief system (in the sense discussed here).
To better capture the difference between the structures of different belief systems, proponents of the monological view have supplemented it with the “higher-order hypothesis” which postulates that conspiracy beliefs relate to each other to the extent that they cohere with a higher-order belief that indirectly provides their mutual support. Here, the focus is not on the direct evidential relationship between particular beliefs, but on the support they receive from a belief that entails their predictions. Wood et al. (Citation2012) suggest that even mutually inconsistent beliefs correlate positively in this way. For instance, they find that people are more likely to simultaneously agree that Osama Bin Laden was both dead and alive when the US forces arrived at the al-Qaida compound if they also believe that the related statements issued by the US government are suggestive of a cover-up operation.
Such findings have been taken to illustrate the seeming irrationality associated with belief in conspiracy theories, as it is commonly assumed that mutually inconsistent claims cannot be outrightly believed simultaneously (e.g., rational agents should not believe both that Princess Diana is alive and that she is dead). However, this interpretation of Wood et al.’s findings has also been called into question. Basham (Citation2018) and Hagen (Citation2018) question the judgment of irrationality passed onto the study subjects for endorsing seemingly mutually inconsistent positions by pointing out that such results do not conclusively show that subjects do, in fact, endorse the beliefs provided in the questionnaires.Footnote4 Furthermore, even if the results were to be taken at face value, subjects’ beliefs could still be rendered rational under the aforementioned assumption that they hold “a mediating belief that authorities are untrustworthy’’ (Hagen, Citation2018, p. 308). While we would like to avoid discussing issues of methodology in social psychology, we acknowledge the importance of the second concern about the role of background belief for making sense of this data in terms of rational inference. One of the advantages of the view which we will defend in this paper is that it can cast light on how such additional background assumptions relate to inconsistent beliefs. As we will argue, credibility is not assigned relative to either of the contrary options alone, but relative to these options and some further auxiliary assumptions. We elaborate on how these assumptions can be expected to change under probabilistic consistency constraints on degrees of belief in the Bayesian analysis in section 3.Footnote5
Unlike (1), (2) postulates that what is crucial for the (ir)rationality of beliefs in CTs is not their internal relationship, but the way in which their associated credences are (or rather are not) updated in light of novel evidence. The earliest version of this postulate can be found in Keeley (Citation1999), who claims that “all potentially falsifying evidence can be construed as supporting, or at worst as neutral evidence” (p. 121) of a CT. Napolitano and Reuter (Citation2021) restate that belief in CTs renders evidence probabilistically irrelevant, meaning that such evidence turns out equally likely when conditioned on the belief as when conditioned on its negation. While this suggests that CT beliefs are unfalsifiable, Napolitano questions whether this condition is sufficient to explain why an agent’s degree of belief in a CT would remain constant regardless of whether disconfirming observations bear on that belief.Footnote6 As she points out, under the irrelevance condition “a conspiratorial explanation can only be immune to being disconfirmed by any new evidence if it remains so general that it makes no specific predictions” (Napolitano & Reuter, Citation2021, p. 10), while also voicing skepticism about the possibility of agents acquiring such general beliefs without forming more specific beliefs that could be easily disconfirmed. Thus, in contrast to Keeley, Napolitano postulates that for CT beliefs to be maintained they need to be self-insulated, and the process of belief-updating cannot admit any disconfirming evidence.
One of the reasons why some epistemologists, such as Napolitano, are keen on postulating the insulation hypothesis is because they are engaging in conceptual engineering of the notion of (as well as belief in) a CT to cast it into a concept that is by definition epistemically suspect and derogatory (Napolitano & Reuter, Citation2021). In contrast, our analysis shows that beliefs about conspiracy theories might update in light of disconfirming evidence and belong to the same class as other kinds of doxastic states.
While the above summary is not exhaustive, the two views share some crucial features despite their many differences. Firstly, they all analyze conspiracy beliefs through the lens of flawed reasoning processes taken to be crucial for understanding the phenomenon. Secondly, they share the important assumption that the cognitive processes which give rise to belief in CTs are irrational and should be demarcated from rational reasoning in everyday as well as in scientific inquiry. Thirdly, they all place special importance on the notion of consistency and inconsistency, either between beliefs themselves (as in 1) or the beliefs and evidence (as in 2). Finally, despite the shared focus on the operations which produce and sustain beliefs in conspiracy theories, none of these views offers a detailed analysis or model of the process it describes.Footnote7 Although Napolitano does present her view in terms of conditional in-\dependence between beliefs and evidence, the Bayesian framing of the insulation of CT beliefs is only used for exposition and does not formalize why insulation happens. This is an important deficiency of these two competing views since it is not entirely clear that they are, in fact, incompatible.
We hope to clarify some of the questions that are left open by previous views. Why do some beliefs appear to be evidentially self-insulated? How can this process be understood conceptually, and in formal terms? Under what conditions is rejecting counterevidence acceptable? From the Bayesian perspective, there is nothing special to self-insulation per se, which is apparent in many forms of belief (e.g., scientific belief), but as a psychological feature, it can become pathological in extreme forms. We propose that our analysis reconciles positions (1) and (2), and though we question the claim that the belief-updating process is irrational, we agree with (2) that an adequate assessment of the rationality of conspiracy belief should take into account the way its associated credence is updated. We start by showing that resistance to counter evidence is principally compatible with Bayesian norms of rationality and, in and of itself, need not be a reasoning flaw.
3. The Bayesian treatment of auxiliary hypotheses
The outlined views place special focus on how conspiracy beliefs are evaluated in relation to other beliefs or the available evidence. This mimics some of the well-known problems in the philosophy of science such as the Quine-Duhem thesis according to which a scientific hypothesis cannot be empirically tested in isolation from additional background assumptions (Duhem et al., Citation1953).Footnote8 One of the results of this interdependence is the underdetermination of scientific prediction by the (confirming or disconfirming) evidence. Suppose we have a central belief h and an auxiliary hypothesis a, such that their conjunct ha entails prediction p, which h alone does not. If p is contradicted by evidence e, then e disconfirms ha. But this says nothing about which of the two conjuncts - a or h - is refuted. For illustration, consider the case of Princess Diana’s death.
Assume the central belief, h, is that Princess Diana is alive. Relevant empirical evidence for or against this claim may take the form of photographs and videos taken by the public media illustrating her official funeral. Call this e. Relevant to interpreting the evidence might be two auxiliary beliefs (for the sake of clarity, we keep the exposition simple). On the one hand, consider the belief that the public media is trustworthy and reports information reliably. Call this a. On the other hand, consider the belief that the government and its public institutions are involved in a cover-up and report unreliable information. Call this a’. In summary:
h: Princess Diana is alive.
a: The public media is trustworthy and reports information reliably.
a’: The government and its public institutions are involved in a cover-up and report unreliable information.
e: Photographs and videos taken by the public media illustrating her official funeral.
The example illustrates that the core belief can only be evaluated in terms of its empirical plausibility, if one additionally adopts (a coherent set of) auxiliary beliefs. Hence, in the following, we will focus on the confirmational import that e bears on pairings of h and a or a’.
The problem calls for a method of rationally distributing the blame between the central hypothesis and the auxiliary constructs. Clarke (Citation2002) has noticed that CT belief’s resistance to counter evidence mimics Lakatos (Citation1976) conception of degenerating research programs in which h is protected from revision by an ever changing set of auxiliary hypotheses A = {a1, a2 an} that can accommodate problematic evidence. However, as Clarke and others (Harris, Citation2018; Napolitano & Reuter, Citation2021) have pointed out, Lakatos did not provide a clear set of criteria that could elucidate at what point it becomes irrational to defend a degenerating research program. The search for these kinds of criteria has been taken up by Bayesian philosophers of science, most notably Urbach and Howson (Citation1993). Here, we focus on Strevens’ (Citation2001) addition to this tradition, which also offers an answer to both of the outlined problems.
Strevens starts with a set of assumptions. Firstly, the simplified assumption that e entails (ha), that is, that e affects h only in virtue of falsifying ha and, respectively, by supporting ha’. Secondly, that there is a limited range of alternatives to a, denoted a’, a’’, ... an, while each of them, together with h, assigns a well-defined probability to e. Thirdly, that h and a are not independent of each other, and that they are positively probabilistically dependent so that when Pr(a) increases Pr(a|h) will increase as well. In the same manner, h and a’ are positively probabilistically dependent when an alternative to a, denoted a’, is called to rescue h. In the Princess Diana case, the dynamics are such that when Pr(a’) increases Pr(a’|h) will also increase, and when Pr(a) decreases Pr(a|h) will decrease.Footnote9 In what follows, we accept these assumptions to allow for an elegant analysis of CT belief.Footnote10 On this basis, we can understand blame-shifting via analogy to Lakatosian research programs where auxiliary hypotheses form a “protective belt” that can absorb the evidential disconfirmation of a central hypothesis.
Let us apply this to the case of Princess Diana. Someone might maintain the core belief that Princess Diana is alive (h) after receiving evidence of the car crash under the auxiliary assumption that the government and its public institutions are involved in a cover-up story (a’), which would justify discarding the alternative auxiliary about the photographic evidence of her funeral being reliable (a). In this scenario, the assumption of a cover-up protects the central belief that Princess Diana is alive from refutation by the available funeral records, due to the expectation that the evidence is fake. Thus, the auxiliary hypothesis that the evidence source is trustworthy (a) is discarded to protect the central belief. In the following, we show that this process entirely conforms with Bayesian norms of rationality.Footnote11 Formally, we model the relationship between the degree of belief in the conjunct ha upon receiving evidence e with Bayes’ theorem:
where the posterior probability of ha given e is a function of the prior probability of ha regardless of e, Pr(ha), and the likelihood of observing the evidence if ha was true, Pr(e|ha). This is normalized relative to the sum of the likelihoods and priors associated with ha and those associated with its negation, (ha).
The first step to solving the problem of apportioning blame between the two hypotheses is to formally separate h from a. We can do this by marginalizing over a under the assumption that the probability of ha and ha sums up to 1 (following the sum ruleFootnote12). In our example, this means that the degree to which a rational agent believes that Princess Diana is alive and the media is trustworthy trades off with the agent’s degree of belief that she is alive and the government and its public institutions are involved in a cover-up. For instance, if the agent already assigns Pr=.7 to option ha, then the agent must assign Pr=.3 to h
a (as not doing so would violate the basic laws of probability). Thus, we obtain
and
Marginalization allows us to “extract” the influence of the central versus auxiliary hypothesis from the overall belief system. Gershman calls this the “crux” of the Bayesian answer to underdetermination: “A Bayesian scientist does not wholly credit either the central or auxiliary hypotheses, but rather distributes the credit according to the marginal posterior probabilities’’ (Gershman, Citation2019, p. 16). On this basis, it is possible to identify the impact of e on the posterior probability of h when ha is disconfirmed (i.e., when ha entails e but e is observed). Since e is observed, we can replace it by
(ha), such that
Pr((ha)|h) says that if h is true, then
(ha) can only be obtained if
a is the case. If we assume h, it follows that Pr(
(ha)|h) = Pr(
a|h) = 1
Pr(a|h). If we insert this into equation (IV) and under the product rule, we obtain
and we can derive
This model apportions the blame in proportion to the relative prior probabilities assigned to a and h. The higher the prior probability, Pr(h), the less h is blamed to the disfavor of a when ha is refuted. Conversely, if a is already highly probable, the blame is put on h, and so the negative impact of e on h increases relative to the certainty about a. In other words, as Pr(a|h) increases, the probability of the set of alternative auxiliaries multiplied with Pr(h) decreases. The robustness of a central hypothesis to disconfirmation can be summarized as the ratio Pr(h)/Pr(a|h), which is illustrated in . The interesting consequence of viewing the structure of CT belief in this way is that it might outwardly seem as if such beliefs are unresponsive to counterevidence, when in fact they follow consistent reasoning in which auxiliaries are rejected to protect the core belief from refutation.
Figure 1. The ratio of the posterior to the prior of h as a function of Pr(a|h) for different values of the prior. Adapted from Gershman (Citation2019, p. 15) and Strevens (Citation2001, p. 526).
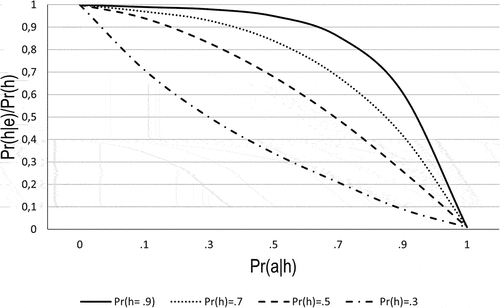
Let us consider the Watergate scandal as another illustration. Assume the central belief, h, is that there is a conspiracy surrounding the 1972 break-in at the Democratic National Committee headquarters at the Watergate Office Building. Relevant empirical evidence for or against this claim may take the form of criminal evidence concerning the break-in, testimony and actions of members of the Nixon party and the U.S. House Judiciary Committee. Call such pieces of evidence e. Relevant to interpreting the evidence are several auxiliary hypotheses: a says that testimony by members of the Nixon administration is reliable. a’ says that there is a systematic cover-up by the Nixon administration. (The inferences in this case might involve additional background beliefs, e.g., that the Nixon administration had a good reason for ordering the break in, but we ignore them for the sake of simplicity). In summary, the Watergate case illustrates the following inference according to our schema:
h: There is a conspiracy surrounding the 1972 break-in at the Democratic National Committee headquarters at the Watergate Office Building.
a: Testimony by the members of the Nixon administration is reliable.
e: The Nixon administration denies any involvement.
a’: There is a systematic cover-up by the Nixon administration.
e’: The Oval Office tapes reveal that Nixon conspired.
e’’: The impeachment articles are accepted by the House Judiciary Committee.
e’’’: Nixon resigned from his office in 1974.
In this case, a’ is called to rescue h. Specifically, a’ predicts e in conjunction with h, which would otherwise refute h if conjoined with a. With increasing novel evidence, e’, e’’, and e’’’, the conjunct of a and h receives increasing confirmation, while conjoining h with a would obtain increasing levels of disconfirmation.
As a final illustration, take the recent rise of belief in Bill Gates conspiracy theories, which might involve as a central belief the claim that Gates has manufactured the COVID-19 pandemic via long-term investments into the creation of vaccines that actually serve to implant microchips to manipulate and infect people with brain tumors. This core belief is surprising, for example, given the existence of photographic evidence of Gates receiving his first Moderna vaccine injection. However, a CT believer could discredit this piece of evidence by adding the auxiliary assumption that such evidence has been engineered, for example by Gates bribing healthcare workers to inject him with a saline solution instead of the vaccine. In terms of our analysis, h corresponds to the central belief that Gates manufactured the COVID-19 pandemic, and a corresponds to the belief that the photo of his vaccine injection is real. Insofar as h is very high a priori for our imaginary CT believer, it is less blamed to the disfavor of a when ha is undermined by the observation of the photograph.
This Bayesian treatment suggests that reasoning about conspiracies does not depend on singular, isolated, beliefs, but rather requires a system of interconnected beliefs that support each other. It is the internal coherence of the belief system that sets the norms governing changes of degree of belief in a conspiracy theory. That is, it is rational to protect h from refutation and maintain the core conspiracy belief, insofar as it does not violate the norms of probability calculus. From this subjectivist perspective, whether rejection of an auxiliary to the favor of the core belief should be deemed irrational depends entirely on the assignment of the prior probabilities to a and h. This raises the question of what the constraints on setting those priors might be, a version of a common worry within subjective versions of the Bayesian framework. We respond to this worry in section 4.1, where we suggest that, with enough counterevidence being available, belief in h should be rejected, and rational Bayesian reasoners should, in the long-run, converge to believing the hypothesis that obtains the best track record in terms of its overall evidential support.
4. Implications for the rationality of conspiracy belief
Let us highlight three general implications of our view for understanding belief in CT. Firstly, if a conjunction of auxiliary and central beliefs is falsified by the evidence, then the central belief can be rescued from refutation by replacing the auxiliary conjunct with an alternative that is not inconsistent with e. For example, the core belief that Princess Diana is still alive (h) seems to be refuted by photographic evidence showing her funeral, e, if one were not to discard the auxiliary assumption that the public media is trustworthy and transparent, and hence offers a reliable evidential source (a) to the favor of the alternative auxiliary hypothesis that Diana faked her death (a’). It is apparent that (ha) entails e,Footnote14 but h can be rescued from refutation by replacing ha with ha’, which is compatible with e.
Secondly, there is no principled difference between core and auxiliary beliefs in the way that probabilities are assigned to them given the evidence. The evidential impact on h increases relative to the certainty about a and vice versa; the important difference lies in their initial probabilities – the status of a “central” versus “auxiliary” hypothesis is purely identified based on their relative probabilities. For example, e has a great negative impact on Pr(a) but only a minor influence on Pr(h) when Pr(a) < Pr(h). Consequently, the probabilities associated with the hypotheses rivaling a will increase. When ha is falsified with Pr(h) < Pr(a), then h would instead be blamed (to the extent its prior is lower). Generally, auxiliary beliefs are more likely to absorb the blame and be readjusted given disconfirming evidence to the extent that they are more questionable to begin with. This contrasts with earlier approaches that see a principled distinction between conspiracy belief and other kinds of belief (such as Napolitano et al., Citation2021).
Thirdly, the apparent resistance to belief updating in light of disconfirming evidence complies with Bayesian norms of reasoning. When ha is disconfirmed by e, h can still be rescued by replacing ha with ha’ (EquationEquation 6(6)
(6) ). But it is not principally irrational to seek confirmation for h given e via a’ — shifting probability away from a is legitimate if the prior for h is sufficiently high and there is an alternative to a that is consistent with the evidence. In other words, it is not always irrational for a conspiracy theorist to shift probability away from auxiliary hypotheses to protect the central belief. The blame is on the protective belt, not on the updating process itself.
For another example, consider the core belief (corresponding to h in our model) that certain electromagnetic waves, including those of the recent 5 G technology, weaken our immune system and slowly damage our DNA. This belief has generated several novel predictions, for instance, that power lines cause cancer in children, that cell phones and high-speed networks cause brain tumors, autism, or Alzheimer’s disease, and that 5 G radio waves contribute to the spread of the COVID-19 pandemic. While this kind of reasoning may be diversely motivated (e.g., by a loss of agency and fear of lacking control), the reasoning itself does not have to be incoherent. It is entirely plausible that each of these predictions was initially formed under the auxiliary assumption that they would be empirically tested under trustworthy scientific standards (corresponding to a in our model). However, people might not always agree with how scientific testing works, and how the results of scientific studies are commonly interpreted or verified. Laboring under the influence of certain biases, e.g., confirmation bias and deterministic thinking, some reasoners may tend to ignore much of the established knowledge about electromagnetic waves and their influence on the human body. For instance, they might discount the observation that 5 G technologies use weak electromagnetic fields that have not been scientifically associated with a higher chance of developing brain tumors (corresponding to e in our model), based on the alternative auxiliary that wavelength weakness does not preclude long-term damaging effects. Attribution biases (suggested by Keeley, Citation1999) can also lead to adopting alternative auxiliaries postulating a malicious manipulative strategy behind the government’s installation of 5 G networks and the information communicated in scientific reports. We return to the effects of inductive biases on the formation of auxiliary beliefs in section 5.
From this perspective, the alleged irrationality of conspiracy belief does not necessarily reside in the dismissal of disconfirming evidence. For example, instead of changing the assumption that electromagnetic waves damage our immune systems, an agent could postulate additional hidden causes that could lead to the circulation of manufactured scientific evidence falsely showing a lack of correlation between wireless technology and the spread of the virus. Such additional hidden causes would allow for a consistent reinterpretation of the scientific evidence as irrelevant to the central hypothesis. This, in turn, would support ha, namely the belief that there is a conspiracy surrounding 5 G technology.
An important consequence of the analysis presented here is that the extent to which the evidence impacts, positively or negatively, some central belief depends on the auxiliary beliefs endorsed, such that a change in the field of auxiliary beliefs can produce a change in the interpretation of the data. The extent to which a belief is confirmed depends not only on the difference between the prior probability of that target belief and how probable it is given the available evidence, but also on the probabilities assigned to the protective belt (see ). Data that might be interpreted as supporting a belief, based on a set of auxiliary assumptions A, could be interpreted as defying that belief, based on the auxiliary set B. In the next section, we address some of the limits to probability shifting for belief protection.
4.1 Irrational belief as a desperate rescue
As Napolitano and Reuter (Citation2021) as well as others have argued, some CT beliefs appear to be irrational because they resist available evidence specifically when it has a negative bearing. If CT belief is principally compatible with Bayesian norms of rationality, then how can we account for the claimed irrationality of CT beliefs?
One idea is that CT belief is irrational because it builds on the endorsement of ad hoc assumptions that are motivated by personal desires or wishful thinking (Hahn et al., Citation2014; Kunda, Citation1990). We can say that an auxiliary belief is ad hoc when it entails unconfirmed claims while being specifically called to rescue a central belief by accommodating the disconfirmatory evidence. When a belief is well confirmed in a stable manner over time, it is well entrenched and not ad hoc. However, if the robustness to disconfirmation is conferred by a strong prior for the central belief, then the endorsement of an ad hoc auxiliary need not be due to motivated reasoning.
Strevens’ (Citation2001) example is the discovery of Neptune, the existence of which was initially postulated to explain away apparent deviations from the path that Newton’s theory of gravitation had predicted for the orbit of Uranus. Strevens characterizes this postulation as a “glorious rescue” because it correctly shifts most of the blame for a false prediction onto the auxiliary belief that there are seven planets in the solar system, and it generated new predictions that allowed the discovery of Neptune by Herschel’s telescopic observations. Analogously, Watergate might be a prime example of a glorious rescue of a CT belief. It was correct to replace the hypothesis that the Nixon administration is trustworthy by the assumption about a cover-up to protect the belief that the 1972 break-in at the Democratic National Committee headquarters involved a conspiratory act. This auxiliary assumption entailed novel predictions that allowed for its subsequent confirmation by the discovery of the Oval Office tapes, which revealed that Nixon’s office did in fact conspire. It is relevant for the explanation and evaluation of the Watergate case that a’ plays a protective role for h, and e’ constitutes a novel discovery. The novelty of e’ as well as the accumulating confirmational support in this case justifies, if only retrospectively, the adoption of a’ over a in conjunction with h as a rational choice.
However, not all rescues lead to glorious discoveries. Some attempts to protect a central belief wrongly blame the auxiliaries for a failed prediction. Strevens characterizes such cases as “desperate” because researchers merely “cling to the central hypothesis and discard the evidently superior auxiliary” (ibid.). In this kind of rescue, the blame is not rationally apportioned between a and h. Therefore, desperate rescues can be treated as a form of irrational reasoning according to the Bayesian standard.
An example of a desperate rescue in the case of CT belief might be the controversy about Bill Gates’ investments into vaccine production. Upon observing photographic evidence reporting Gates’ receiving the vaccine injection, the central belief, that Gates has funded and planned the COVID-19 pandemic to implant controlling microchips into people (h), and the initial auxiliary supplement, that healthcare workers are trustworthy (a), are disconfirmed. Following the analysis in section 3, h can be protected from refutation by doubting a. Then the unexpected event, his reception of the vaccine injection, can be explained by postulating, for example, that he faked it by bribing the nurse(s) into replacing the vaccine with a saline solution (corresponding to a’). However, following the analysis in section 3, this rescue is desperate, if the initial faith in the trustworthiness of healthcare workers was very high to begin with (being equivalent to Pr(a) in the model being very high). Then the shift to believing that Gates faked his vaccine reception is unwarranted, since the belief that he has manufactured the pandemic to implant microchips loses most of its credibility (), and so trust in healthcare workers would be wrongly discarded.
Of course, labeling this explanation as “desperate” is appropriate only if the belief that healthcare workers are reliable is evidentially superior to the belief that Gates manufactured the spread of the virus. The analogy with scientific discoveries, such as the Neptune case, suggests that whether a belief is evidentially superior depends on its historical track record. In this case, we may take “historical track record” to be the past record associated with measurable outputs of the health care system, personal experience, and third-person reports. In analogy to Strevens’ example, the universal law of gravitation had accumulated a much greater degree of confirmation over time than the competing alternatives, so its superior track record provided reasons for assigning to it a much stronger prior belief. If agents have set these priors in correspondence with the historical track record, and if this prior turns out to be lower than the priors for available alternatives, then clinging on to that belief (to the disfavor of a better alternative) can be considered desperate, or simply irrational. In the Gates example, the auxiliary that he controls public servants is specifically called to protect the belief that there is a conspiracy surrounding his investments into vaccine production. The conjunction of these hypotheses might be internally coherent and even generate a new prediction that Gates aims to control the world’s population. However, the central belief about the conspiracy is not well entrenched, since little confirmation in favor of such belief has accumulated over time. Thus, the Gates vaccination conspiracy is a likely candidate for being labeled as a “desperate rescue”.
This brings us to an important implication of the track-record constraint, which is that identifying whether a given case of belief protection counts as “glorious” or “desperate” in terms of its overall evidential support can often be determined only in the long run. Thus, as the examples of the conspiracy beliefs surrounding Gates’ investments and the Watergate scandal illustrate, the distinction between “glorious” and “desperate” rescues might itself be a matter of degree.
5. Reconciling monological and self-insulated systems
We will now view the monological and self-insulation approaches outlined in section 3 through the Bayesian lens, and show that they can be seen as two ways of describing the same kind of belief system.
On the Bayesian view, both central and auxiliary beliefs mutually constrain one another and follow the same principles for belief revision. For instance, how likely an auxiliary hypothesis is to be rejected directly depends on how well it is entrenched compared to its rivals. This fits with the common characterization of CT belief in terms of a monological system, that is, a system where different beliefs form a self-sustaining network that can absorb varying kinds of evidence. As elucidated in section 3, as Pr(a|h) increases, the probability of the alternative auxiliaries multiplied with Pr(h) decreases (). For instance, the probability that Gates faked his COVID-19 vaccine injection given that he planned the spread of the virus is considerably higher than the probability that the photograph of him getting the first vaccine is real given that he is trying to control the world population. On the one hand, these auxiliaries mutually constrain each other; if the agent is highly confident that one of them is true, they should find the truth of the alternative highly dubious. On the other hand, conflicting hypotheses that share content, e.g., in being about Gates’ evil plans, are part of the same hypotheses space; they are tied to each other by playing the role of arguments in a probability function that is distributed across all of them. Since, following the probability axioms, the probabilities associated with the individual hypotheses must sum up to 1, raising credence in one hypothesis affects credence in the other ones. By the same manner, stipulating h (e.g., that Gates has planned the pandemic) directly raises the probability of certain auxiliary hypotheses (e.g., that Gates faked his photograph) to the disfavor of others (e.g., that the public media is transparent). This also holds for the introduction of new auxiliaries to the hypothesis space, insofar as they are compatible with the central hypothesis, that is, if Pr(a|h)/Pr(h) is sufficiently high. In this sense, the Bayesian view on offer allows us to model how even mutually inconsistent conspiratorial beliefs are interconnected and can support one another as long as they share content with a central hypothesis, thus accommodating the framing of monologicity in terms of higher-order beliefs proposed by Douglas et al. (Citation2019). We expect that beliefs central to any particular CT will be more general in scope, not only because they offer hypotheses that better reconcile mutually inconsistent auxiliaries (thus being better entrenched and less prone to disconfirmation), but also because Bayesian methods favor hypotheses that are more general and have a higher chance of generalizing to new data (a feature which we discuss in the next section).
Our treatment likewise captures aspects of the apparent insensitivity to disconfirmation highlighted by Napolitano and Reuter (Citation2021) as well as Keeley (Citation1999), which we understand as a matter of belief protection (as opposed to ignorance or insulation). In agreement with these positions, our view suggests that to evaluate the (ir)rationality associated with CT belief from a normative perspective, we should not only study the internal relationship among such beliefs, but also to their (dis)confirmatory relationships with the evidence, if only in the long run. Correspondingly, our analysis allows for belief networks to show monologicality and apparent self-insulation to the extent that they are self-sustaining (at least over short periods of time) and exemplify desperate rescues (in which auxiliary hypotheses are changed to accommodate novel evidence).
However, unlike these views, our approach does not analyze CT beliefs through the lens of flawed reasoning processes, and instead renders some cases of CT belief – especially the “glorious”’ rescues – akin to rational reasoning in everyday as well as in scientific inquiry.
6. A benchmark of rationality
So far, we have shown how the Bayesian treatment can unify the monological and higher-order views as well as account for the seeming insensitivity of conspiratorial beliefs to disconfirmatory evidence. On our view, beliefs about conspiracy theories might update in light of disconfirming evidence and hence belong to fundamentally the same class as other kinds of doxastic states. This, together with the fact that Bayesian probability theory is often taken as a normative standard for rationality, might suggest a view in which believing in conspiracy theories is always rational after all. However, we do not endorse such a view.
Our claim is not that all conspiracy beliefs are rational, but rather that beliefs about conspiracy theories are not fundamentally different from any other kind of belief. Furthermore, given a multitude of definitions and a lively debate over the notion of conspiracy theories, we do not wish to engage in any project of engineering the concept. In our view, a belief in a conspiracy is not epistemically different from a belief in a conspiracy theory. After all, there are well-recognized cases, such as the aforementioned Watergate scandal, where conspiratorial beliefs turned out to be true. However, this does not mean that the difference between a true belief about an actual conspiracy and a false belief about an outlandish conspiracy lies only in their truth value. In fact, our account provides a benchmark for tracking the credibility of a central hypothesis and whether or not it should be abandoned. We can make this explicit by returning to Strevens’ distinction between glorious and desperate rescues.
Recall from section 4.1 that the two kinds of revisions to auxiliary beliefs differ in how well the central belief is supported by the available evidence. The discovery of Neptune is an example of glorious rescue because, at the time of the adoption of the auxiliary hypothesis about the planet’s existence, Newton’s theory had been much better confirmed than any competing theory, which in turn justified adopting surprising auxiliary hypotheses to account for the anomalous disconfirmatory evidence. It is this condition that is violated in what our account clearly stigmatizes as a desperate rescue, where the central hypothesis is held onto despite its bad track record and given few sources of evidence and predictions that repeatedly fail to be confirmed.
What is crucial to the view presented here is that the differences between the two kinds of belief revision, glorious and desperate, can be compared in terms of the relative probabilities of the beliefs in question. Thus, as Strevens points out, one is only justified in revising the auxiliary beliefs when the probability of the central hypothesis, Pr(h), is higher than that of the auxiliary, Pr(a), while the degree of justification (or “glory” of the rescue in Strevens’ terms) is inversely proportional to the prior probability of the auxiliary hypothesis. While this does not offer, as some philosophers may wish, an a priori distinction between legitimate and illegitimate beliefs in conspiracies, it puts us on track for a comparison of different conspiratorial explanations. While this position does not clearly adjudicate whether the belief in CIA’s involvement in the US crack epidemic is a case of glorious or desperate rescue, it does state that beliefs in conspiracy theories which are poorly entrenched and can only be maintained through regular adoption of new auxiliary hypotheses, such as the belief that the Earth is flat or the QAnon conspiracy, are desperate and irrational.
7. Possible effects of inductive biases on the formation of belief in conspiracy theories
In the penultimate section of this paper, we explore some additional insights that contemporary Bayesian cognitive science offers for explaining conspiracy belief formation. Specifically, we focus on the initial parameters of the belief system and their constraining role in the inductive process. Such “inductive biases” decide which auxiliary beliefs will be considered as “good” explanations for observations in the first place, by weighing the posteriors and priors computed for individual beliefs (Tenenbaum et al., Citation2006). Inductive biases can take multiple forms, but here we concentrate on two examples that seem helpful for characterizing cognitive constraints on the formation of CT beliefs.
The first example is a bias for sparse beliefs, which, following Gershman (Citation2019), encodes a preference for auxiliaries that generate narrow predictions consistent with the evidence. In the extreme, these are auxiliaries that predict all and only the observed data. Evidence suggesting that people might endorse such biases comes from studies of concept learning. For example, when people infer animal categories, they seem to prefer subordinate (DALMATIAN) or basic-level (DOG), as opposed to superordinate level predictions (ANIMAL), even if the available evidence (e.g., a spotted dog that resembles a Dalmatian) underdetermines which of these predictions representing the category’s intension is correct (Xu & Tenenbaum, Citation2007). From the perspective of philosophy of science, sparse beliefs are valuable because their low initial probability makes them highly informationally relevant to acquired empirical evidence (Bar-Hillel, Citation1955; Popper, Citation1954). Sparse beliefs are verifiable by sparse evidence.Footnote15
Under the assumption of agents’ bias toward forming sparse belief systems, we can expect them to be also veer toward doxastic determinism. Here, “determinism” refers to a preference for ascribing high credences to only a few beliefs consistent with the data (Gershman, Citation2019).Footnote16 In the extreme, this means endorsing only auxiliaries that perfectly predict the observed data. The rationale for this is that, in belief networks that predict only a few possible events, each of the predictions will be assigned with a relatively high probability (since the axioms of probability theory require probability assignments to sum up to 1). Together, a joint bias for sparsity and determinism can lead the system to single out one hypothesis as “the only true cause” for a given set of observations.
The second example is a bias toward simple belief systems. This bias is driven by concern for predictive accuracy and avoidance of overfitting hypotheses to the available data, which can be illustrated by the problem of model selection in Bayesian statistics (see Griffiths & Yuille, Citation2006). The problem in question is choosing, based on the observations, among a set of hypotheses of varying complexities. Complex hypotheses are more flexible and can be better fitted to the available data. This means that they can make better predictions, provided that future observations follow tendencies present in the existing data. However, complex hypotheses can also lead to worse predictions if the available data is anomalous. Thus, on average, simpler hypotheses will generalize better across a broad range of scenarios and possible observations. This feature has been labeled as Bayesian Occam’s Razor (BOR): beliefs that are too sparse or fixed are unlikely to generate future observations; beliefs that are too flexible can generate many possible data sets, while also being unlikely to generate a particular data set at random. Interestingly, a recent study by Blanchard, Lombrozo, and Nichols (Citation2018) has shown that when confronted with simple narrative tasks “people’s intuitive judgments follow the prescriptions of BOR, whether making estimates of the probability of a hypothesis or evaluating how well the hypothesis explains the data” (Blanchard et al., Citation2018, p. 1355).
These examples illustrate that inductive biases can pull the updating process in opposite directions. An optimal agent should achieve a balance to avoid overfitting (making hypotheses too precise) or underfitting (making them too general) (see Forster & Sober, Citation1994 in the context of scientific inferences). Depending on which inductive biases are present, different inferences can become plausible in light of the same evidential observation. For example, a bias toward sparse hypotheses could explain why “conspiracy theorists use a large set of auxiliary hypotheses that perfectly (i.e., deterministically) predict the observed data and only the observed data (sparsity)’’ (Gershman, Citation2019, p. 23). However, such agents would be unable to generalize toward novel cases, violating a bias toward simplicity. Thus, as Gershman (Citation2019, p. 23) himself suggests, there may be significant individual differences in how strong particular biases are in different individuals. This may be tied to certain personality traits (e.g., “epistemic vices”, see Cassam, Citation2019 and Sunstein & Vermeule, Citation2008; but see Pidgen, Citation2016 for a rebuttal) which could predispose some people to have a propensity for forming belief networks more prone to hijacking by CT narratives. Due to the relatively young age of this research domain and space constraints, we leave discussion of the exact ways in which such biases might influence inferential processes as a topic for future investigation.
8. Conclusion
In this paper, we have used the Bayesian framework to analyze beliefs about conspiracy theories and present the implications of framing such beliefs in this way for two prominent proposals regarding their nature. As we have shown, the Bayesian framing not only offers helpful insight for the existing accounts, but also allows to unify them under a single formal umbrella. What is intuitively appealing in our analysis is that instead of focusing on changes in isolated beliefs, it focuses on changes in belief systems (as involving auxiliary claims). We regard this perspective as more plausible, not only for scientific inference but also for psychological inference. Secondly, our analysis predicts that belief systems with repeatedly disconfirmed hypotheses must eventually fall. Even if a central belief can be protected from refutation to the disfavor of an auxiliary belief (at a given moment of disconfirmation), its associated credence must nevertheless decrease in that moment. Thus, by showing that the apparent resistance to counter evidence is compatible with Bayesian norms of inference, our analysis also opposes the idea that people who believe conspiracy theories exhibit a reasoning style which is fundamentally at odds with widely accepted norms of reasoning, since they do apportion the blame for a failed prediction according to their prior beliefs.
Where the Bayesian approach departs from the previous proposals is that it does not principally rule out that conspiracy beliefs can be rational. Some previous accounts suggest that conspiracy beliefs, which tend to fail to update in response to novel contrary information, are irrational on that account. Our analysis, in contrast, suggests that such behavior is not necessarily irrational. It is only irrational in cases in which there is a desperate attempt to rescue badly confirmed hypotheses by introducing only weakly grounded ad hoc auxiliary beliefs. A tendency to do so may be enhanced by strong individual inductive biases. However, since many of the glorious rescues in science might have at one point in time seemed desperate, an important consequence of our view is that part of the (ir)rationality of conspiracy beliefs might depend on the wider context in which they are formed and independent means of their verification. Thus, we suggest that more attention should be given to aspects of conspiracy belief other than updating, for example, the role that social factors play in their acquisition.
Disclosure statement
No potential conflict of interest was reported by the author(s).
Correction Statement
This article has been corrected with minor changes. These changes do not impact the academic content of the article.
Notes
1. For the same reasons, we do not assume that there is a principled difference between beliefs in conspiracies and beliefs in conspiracy theories. If any such difference exists, it should emerge as a conclusion rather than constitute a starting point of our inquiry.
2. Some approaches have used Bayesian tools explicitly to analyze the protective nature of delusional beliefs (e.g., McKay, Citation2012). Although the two kinds of phenomena are oftentimes considered to be related (see Bortolotti et al., Citation2021, for a comparative analysis), explicit Bayesian analysis of belief in CTs is still widely lacking. To fill this gap, we concentrate our present efforts only on the case of belief in CTs. Our analysis might be applicable to other cognitive phenomena such as delusional beliefs, but such applications remain beyond the scope of this paper.
3. It should be noted that sometimes, reframing apparent counterevidence can be the rational thing to do. For example, suspicion concerning the Nixon administration’s initial defense during the Watergate scandal was justified (Buenting & Taylor Citation2010). We are grateful to an anonymous reviewer for pointing us to this possibility.
4. We focus on degree of belief, i.e., credences, and our discussion remains neutral with regards to debates about how to interpret Wood et al.’s findings for outright belief.
5. Our novel suggestion for this debate is to consider, contra Hagen and Basham, that even if the given positions were to be interpreted as outrightly held beliefs (as was initially suggested by Wood et al.), it would not suggest that holding them is irrational. We argue that what is important to deciding on the status of (ir)rationality of such beliefs is the way in which they are probabilistically related, specifically, the way in which they are conditionally dependent on each other. Insofar as belief in the “is alive” option does not directly depend on belief in the “is dead” option, there is nothing strange about increasing one’s credence in either of such propositions, if it is also highly probable that authorities are covering up. Our analysis thus responds to the quest to understand “how beliefs and attitudes hold together” (Hagen Citation2018, p. 310, see also Sutton & Douglas Citation2014).
6. It is important to note that this is not the same as the belief being probabilistically independent from the evidence. Independence corresponds to: Pr(belief|evidence) = Pr(belief), while irrelevance, as Napolitano uses the term, corresponds to: Pr(evidence|belief) = Pr(evidence|¬belief).
7. An exception is Benjamin Goertzel’s (Citation1994b) application of complex systems theory to distinguish open from closed minds. Despite little impact for the topic of belief in CTs, it has inspired work formally distinguishing conspiracy narratives from conspiracy theories on the internet (Tangherlini et al., Citation2020).
8. It is important to note that our analysis builds on an analogy, as opposed to an identity, between psychological and scientific reasoning.
9. Whether a or a’ is selected as being probabilistically dependent on h may change based on the available evidence. In the Diana case, after receiving evidence of the car crash, h may co-depend more with a’ while prior to receiving this evidence, h may co-depend more with a.
10. A detailed discussion of these assumptions can be found in the exchange between Fitelson and Waterman (Citation2005, Citation2007) and Strevens (Citation2005).
11. Our analysis does not consider the effects of ordering beliefs, but focuses on the rational relations between beliefs independently of their order. From this perspective, it makes no difference to the protection of the core belief that Princess Diana is still alive (h) whether the agent initially endorses the auxiliary that the photographic evidence of her funeral is reliable (a) and then evaluates the evidence with the alternative auxiliary that the government and its public institutions are involved in a cover-up story (a’), or vice versa. We are interested in why, from the idealized perspective of Bayesian inference, it makes sense to resist the evidence, if one were to adopt a’ instead of a as a means to interpret the evidence. For what reasons an agent does in fact adopt either a or a’ is interesting but a broader question outside the scope of this paper. Our rationale for calling the auxiliaries “alternatives” is not because one is endorsed subsequent to the other but that they cannot simultaneously obtain Pr = 1. The idealizations present in our analysis (since it diverges from reasoning in real-world agents whose memory is affected by ordering effects) support our aim of making a contribution that can clarify aspects of modeling belief protection mechanisms, since simpler models are easier to understand and investigate. We thank an anonymous reviewer for asking us to clarify this perspective.
12. The sum rule is a foundational assumption of Bayesianism. It expresses the relationship between a proposition, a, and its negation, ¬a, given some fixed background assumptions, as a sum of their conditional probabilities: Pr(a|b) + Pr(¬a|b) = 1. For an excellent introduction, readers are referred to Stone (Citation2013, pp. 32–33).
13. Cf. Gershman (Citation2019, p. 15).
14. We closely follow Strevens’ understanding of entailment, where `ha entails e’ means that ha is to some extent compatible or confirmed by the occurrence of e.
15. Xu and Tenenbaum appeal to the size principle, which is used in computational psychology as a heuristic to explain why people may inductively generalize in the way they do, beyond the data they see. According to this principle, when people make inferences about what category an exemplar belongs to, they are in part guided by a bias to weigh categories with a narrower intension higher than those with wide intension (the size of the intension of a category is defined by the similarity of possible things falling under the corresponding word, e.g., the similarity among Dalmatians is assumably on average greater than the average similarity among things falling under “dog”). If people preferred to infer ”Dalmatian” as the correct category, they illustrate a preference for inferring a more specific hypothesis about the correct category intension, even though the observation of a white spotted dog is also compatible with the hypothesis that this example has been generated from the much larger categories of dogs, white spotted things or things in the universe. Sparse beliefs can thus be understood as propositions with narrow content. One reason for why it has been considered rational to rely on such propositions in scientific inferences is that they are a priori highly improbable but highly likely when confirmed, if confirmation is assessed in terms of the overlap of the content of the prediction and the content of the evidential statement. This inductive inference principle has been repeatedly used in computational models to explain how people can learn so much so quickly even from a limited set of everyday observations.
16. Gershman does not explain how consistency can be quantified, but Strevens (Citation2001, p. 529) assumes that some measure of the degree of confirmation is appropriate.
References
- Bar-Hillel, Y. (1955). An examination of information theory. Philosophy of Science, 22(2), 86–105. https://doi.org/10.1086/287407
- Basham, L. (2016). The need for accountable witnesses: A reply to dentith. Social Epistemology Review and Reply Collective, 5(7), 6–13.
- Basham, L. (2018). Social Scientists and Pathologizing Conspiracy Theorizing. In M. R. X. Dentith (Ed.), Taking Conspiracy Theories Seriously (pp. 95–107). London: Rowman and Littleʨield.
- Blanchard, T., Lombrozo, T., & Nichols, S. (2018). Bayesian Occam's razor is a razor of the people. Cognitive science, 42(4), 1345–1359. https://doi.org/10.1111/cogs.12573
- Bortolotti, L., Ichino, A., & Mameli, M. (2021). Conspiracy theories and delusions. Reti, saperi, linguaggi, 8(2), 183–200.
- Buenting, J., & Taylor, J. (2010). Conspiracy Theories and Fortuitous Data. Philosophy of the Social Sciences, 40(4), 567–578. https://doi.org/10.1177/0048393109350750
- Butter, M. (2021). Conspiracy theories–conspiracy narratives. Diegesis Interdisciplinary E-Journal for Narrative Research/Interdisziplinäres E-Journal für Erzählforschung, 10(1), 97–100.
- Cassam, Q. (2019). Conspiracy theories. Polity Press.
- Cichocka, A., Marchlewska, M., & de Zavala, A. G. (2016). Does self-love or self-hate predict conspiracy beliefs? Narcissism, self-esteem, and the endorsement of conspiracy theories. Social Psychological and Personality Science, 7(2), 157–166. https://doi.org/10.1177/1948550615616170
- Clarke, S. (2002). Conspiracy theories and conspiracy theorizing. Philosophy of the Social Sciences, 32(2), 131–150. https://doi.org/10.1177/004931032002001
- Colombo, M., & Hartmann, S. (2017). Bayesian cognitive science, unification, and explanation. The British Journal for the Philosophy of Science. https://doi.org/10.1093/bjps/axv036
- Dentith, M. R. X. (2016). When inferring to a conspiracy might be the best explanation. Social Epistemology, 30(5–6), 572–591. https://doi.org/10.1080/02691728.2016.1172362
- Dentith, M. R. X. (2019). Conspiracy theories on the basis of the evidence. Synthese, 196(6), 2243–2261. https://doi.org/10.1007/s11229-017-1532-7
- Douglas, K. M., Uscinski, J. E., Sutton, R. M., Cichocka, A., Nefes, T., Ang, C. S., & Deravi, F. (2019). Understanding conspiracy theories. Political Psychology, 40(S1), 3–35. https://doi.org/10.1111/pops.12568
- Duhem, P. (1953). Physical theory and experiment. In H. F & M. Brodbeck (Eds.), Readings in the Philosophy of Science (pp. 235–252). Appleton-Century-Crofts, Inc.
- Feldman, S. (2011). Counterfact conspiracy theories. The International Journal of Applied Philosophy, 25(1), 15–24.
- Fenster, M. (2008). Conspiracy theories: Secrecy and power in American culture. University of Minnesota Press.
- Fitelson, B., & Waterman, A. (2005). Bayesian confirmation and auxiliary hypotheses revisited: A reply to Strevens. The British Journal for the Philosophy of Science, 56(2), 293–302. https://doi.org/10.1093/bjps/axi117
- Fitelson, B., & Waterman, A. (2007). Comparative Bayesian confirmation and the Quine–Duhem problem: A rejoinder to Strevens. The British Journal for the Philosophy of Science, 58(2), 333–338. https://doi.org/10.1093/bjps/axm012
- Forster, M., & Sober, E. (1994). How to tell when simpler, more unified, or less ad hoc theories will provide more accurate predictions. The British Journal for the Philosophy of Science, 45(1), 1–35. https://doi.org/10.1093/bjps/45.1.1
- Franks, B., Bangerter, A., Bauer, M. W., Hall, M., & Noort, M. C. (2017). Beyond “monologicality”? Exploring conspiracist worldviews. Frontiers in Psychology, 8, 861. https://doi.org/10.3389/fpsyg.2017.00861
- Gershman, S. J. (2019). How to never be wrong. Psychonomic Bulletin & Review, 26(1), 13–28. https://doi.org/10.3758/s13423-018-1488-8
- Goertzel, T. (1994a). Belief in conspiracy theories. Political Psychology, 15(4), 731–742. https://doi.org/10.2307/3791630
- Goertzel, B. (1994b). Chaotic logic. Plenum.
- Goreis, A., & Voracek, M. (2019). A systematic review and meta-analysis of psychological research on conspiracy beliefs: Field characteristics, measurement instruments, and associations with personality traits. Frontiers in Psychology, 10, 205. https://doi.org/10.3389/fpsyg.2019.00205
- Griffiths, T. L., & Yuille, A. L. (2006). Technical introduction: A primer on probabilistic inference. UCLA. Department of Statistics Papers no. 2006010103. UCLA.
- Hagen, K. (2018). Conspiracy theorists and monological belief systems. Argumentation, 3 2 , 303–326.
- Hagen, K. (2022). Are ‘conspiracy theories’ so unlikely to be true? A critique of Quassim Cassam’s concept of ‘conspiracy theories’. Social Epistemology, 36(3), 1–15. https://doi.org/10.1080/02691728.2021.2009930
- Hahn, U., & Harris, A. J. (2014). What does it mean to be biased: Motivated reasoning and rationality. In B. Ross (Ed.), Psychology of learning and motivation (Vol. 61, pp. 41–102). Academic Press.
- Harris, K. (2018). What’s epistemically wrong with conspiracy theorising? Royal Institute of Philosophy Supplements, 84, 235–257. https://doi.org/10.1017/S1358246118000619
- Keeley, B. L. (1999). Of conspiracy theories. The Journal of Philosophy, 96(3), 109–126. https://doi.org/10.2307/2564659
- Kunda, Z. (1990). The case for motivated reasoning. Psychological Bulletin, 108(3), 480. https://doi.org/10.1037/0033-2909.108.3.480
- Lakatos, I. (1976). The methodology of scientific research programmes. Cambridge University Press.
- Levy, N. (2019). Due deference to denialism: Explaining ordinary people’s rejection of established scientific findings. Synthese, 196, 313–327. https://doi.org/10.1007/s11229-
- Levy, N. (2021). Echoes of covid misinformation. Philosophical Psychology, 1–17. https://doi.org/10.1080/09515089.2021.2009452
- McKay, R. (2012). Delusional inference. Mind & Language, 27(3), 330–355. https://doi.org/10.1111/j.1468-0017.2012.01447.x
- Miller, J. M. (2020). Do COVID-19 conspiracy theory beliefs form a monological belief system? Canadian Journal of Political Science/Revue Canadienne de Science Politique, 53(2), 319–326. https://doi.org/10.1017/S0008423920000517
- Napolitano, G. (2021). Conspiracy theories and evidential self-insulation. In S. Bernecker, A. Flowerree, & T. Grundmann (Eds.), The epistemology of fake news (pp. 82–106). Oxford University Press.
- Napolitano, G., & Reuter, K. (2021). What is a conspiracy theory? Erkenntnis. https://doi.org/10.1007/s10670-021-00441-6
- Pigden, C. R. (2016). Are conspiracy theorists epistemically vicious? In K. Lippert-Rasmussen, K. Brownlee, & D. Coady (Eds.), A companion to applied philosophy (pp. 120–132). John Wiley & Sons, Ltd.
- Popper, K. R. (1954). Degree of confirmation. The British Journal for the Philosophy of Science, 5(18), 143–149.
- Stokes, P. (2016). Between generalism and particularism about conspiracy theory: A response to Basham and Dentith. Social Epistemology Review and Reply Collective, 5(10), 34–39.
- Stone, J. V. (2013). Bayes’ rule: A tutorial introduction to Bayesian analysis. Sebtel Press.
- Strevens, M. (2001). The Bayesian treatment of auxiliary hypotheses. The British Journal for the Philosophy of Science, 52(3), 515–537. https://doi.org/10.1093/bjps/52.3.515
- Strevens, M. (2005). The Bayesian treatment of auxiliary hypotheses: Reply to Fitelson and Waterman. The British Journal for the Philosophy of Science, 56(4), 913–918. https://doi.org/10.1093/bjps/axi133
- Sunstein C R and Vermeule A. (2009). Conspiracy Theories: Causes and Cures*. Journal of Political Philosophy, 17(2), 202–227. 10.1111/j.1467-9760.2008.00325.x
- Suthaharan, P., Reed, E. J., Leptourgos, P., Kenney, J. G., Uddenberg, S., Mathys, C. D., Litman, L., Robinson, J., Moss, A. J., Taylor, J. R., Groman, S. M., & Corlett, P. R. (2021). Paranoia and belief updating during the COVID-19 crisis. Nature Human Behaviour, 5(9), 1190–1202. https://doi.org/10.1038/s41562-021-01176-8
- Sutton, R., & Douglas, K. (2014). Examining the monological nature of conspiracy theories. In J. Prooijen & P. Lange (Eds.), Power, Politics, and Paranoia: Why People are Suspicious of their Leaders (pp. 254–272). Cambridge: Cambridge University Press. doi:10.1017/CBO9781139565417.018
- Tangherlini, T. R., Shahsavari, S., Shahbazi, B., Ebrahimzadeh, E., Roychowdhury, V., & Lin, Y. -R. (2020). An automated pipeline for the discovery of conspiracy and conspiracy theory narrative frameworks: Bridgegate, pizzagate and storytelling on the web. Plos One, 15(6), e0233879. https://doi.org/10.1371/journal.pone.0233879
- Tenenbaum, J. B., Griffiths, T. L., & Kemp, C. (2006). Theory-based Bayesian models of inductive learning and reasoning. Trends in Cognitive Sciences, 10(7), 309–318. https://doi.org/10.1016/j.tics.2006.05.009
- Urbach, P., & Howson, C. (1993). Scientific reasoning: The Bayesian approach. Open Court, Second Edition.
- van Prooijen, J. W., & van Vugt, M. (2018). Conspiracy theories: Evolved functions and psychological mechanisms. Perspectives on Psychological Science, 13(6), 770–788. https://doi.org/10.1177/1745691618774270
- Wood, M. J., Douglas, K. M., & Sutton, R. M. (2012). Dead and alive: Beliefs in contradictory conspiracy theories. Social Psychological and Personality Science, 3(6), 767–773. https://doi.org/10.1177/1948550611434786
- Xu, F., & Tenenbaum, J. B. (2007). Word learning as Bayesian inference. Psychological Review, 114(2), 245. https://doi.org/10.1037/0033-295X.114.2.245