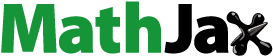
ABSTRACT
In behavioural contexts like fighting, eating, and playing, acoustically distinctive vocalisations are produced across many mammalian species. Such expressions may be conserved in evolution, pointing to the possibility of acoustic regularities in the vocalisations of phylogenetically related species. Here, we test this hypothesis by comparing the degree of acoustic similarity between human and chimpanzee vocalisations produced in 10 similar behavioural contexts. We use two complementary analysis methods: Pairwise acoustic distance measures and acoustic separability metrics based on unsupervised learning algorithms. Cross-context analysis revealed that acoustic features of vocalisations produced when threatening another individual were distinct from other types of vocalisations and highly similar across species. Using a multimethod approach, these findings demonstrate that human vocalisations produced when threatening another person are acoustically similar to chimpanzee vocalisations in the same situation as compared to other types of vocalisations, likely reflecting a phylogenetically ancient vocal signalling system.
Vocalisations described as ‘roars’ and ‘grunts’ occur across a wide range of species. Around 150 years ago, Darwin (Citation1872) hypothesised that such behaviours are phylogenetically continuous across mammalian species. Researchers have shown that many animal groups produce vocalisations in contexts that serve certain social and biological functions like play, threat and food (Morton Citation1977). For instance, low-frequency vocalisations with a wide frequency range and nonlinearities (e.g. the chaotic and noisy phonation in a rough or harsh voice) are produced when threatening another individual in many vertebrates (see Briefer Citation2012 for a review). Acoustic regularities across many taxa may reflect evolutionarily conserved vocal systems (Bryant Citation2021). In testing such preserved acoustic structures across animal groups, however, most of what we know comes from literature on non-human animals. Is there an evolutionary continuity between humans and other animals for nonverbal vocal expressions? In the current study, we test the existence of similarities in acoustic structures between vocalisations of humans (Homo sapiens) and one of our closest living relatives, chimpanzees (Pan troglodytes), produced in a wide range of behavioural contexts. We compare acoustic similarity between human and chimpanzee vocalisations produced in similar behavioural contexts to vocalisations produced in different contexts.
To date, only a handful of studies have tested acoustic similarities between humans and other animals for vocal expressions, and they mainly examined homologues of human laughter in other species (e.g. Davila Ross et al. Citation2009). This work shows that human laughter shares acoustic characteristics with play vocalisations in other great apes. A second line of research has demonstrated that human listeners can accurately infer affective information from heterospecific vocalisations, suggesting that this ability may draw on acoustic regularities that are conserved across related species (e.g. Scheumann et al. Citation2014; Filippi et al. Citation2017). Human listeners can accurately infer core affect dimensions like arousal (physiological alertness) and valence (positive or negative contexts) from vocalisations of different species. However, acoustic features of mammalian species systematically vary not only in terms of core affect dimensions but also in terms of production contexts that do not necessarily differ in terms of core affect dimensions (Morton Citation1977). For example, an individual attacking a conspecific and an individual facing off a dangerous predator are both in highly aroused and negative situations, but the situations and accompanying vocalisations are profoundly different. Moreover, perception studies may not fully capture the similarities in acoustic structures. These studies typically focus on the most salient or discriminative acoustic features that allow listeners to differentiate between vocalisations, while ignoring other subtle acoustic similarities or differences that may exist between vocalisations. Furthermore, human perception is influenced by a combination of factors, including the sensitivity of the human ear, auditory neurobiology, and cognitive processing. This is particularly true when comparing the acoustic structures of vocalisations across different species, where differences in these factors can lead to different perceptual abilities and limitations. Perception studies may therefore not fully capture the subtle acoustic differences or similarities that are beyond the limits of human perception. For instance, in a recent study, acoustic similarities between primate vocalisations did not predict human listeners’ perceptual judgements (Debracque et al. Citation2022). In order to assess evolutionary continuity in vocalisations, we thus need to directly compare acoustic structures of vocalisations mapping onto specific types of behavioural contexts across humans and other species.
One reason for the lack of comparative research between human and other animals’ vocalisations is that human vocalisations are often collected based on the emotional state of the expresser (e.g. feeling angry, afraid or amused: Laukka et al. Citation2013), while vocalisations of other species are categorised based on the behavioural context (e.g. play, food and threat) in which they were produced. There is a clear risk of anthropomorphism if we try to map the vocalisations of other species onto human emotion categories, but it is possible to obtain human vocalisations produced in behavioural contexts similar to other species. This would allow us to directly compare the acoustic structure of human vocalisations to those produced by other species in parallel situations (e.g. being attacked). Here, we employ human and chimpanzee vocalisations produced in a wide range of real-life situations.
In the present study, we test the hypothesis that human and chimpanzee vocalisations that are produced in parallel behavioural contexts are acoustically more similar in comparison to cross-species vocalisations produced in other contexts. We used two different approaches to assess acoustic similarity: 1) pairwise acoustic distance measures and 2) acoustic separability metrics based on unsupervised learning. Acoustic distance measures are widely used for computations of acoustic similarity and produce an average acoustic distance matrix demonstrating the (dis)similarity between cross-species vocalisations produced in different contexts. Additionally, we employed unsupervised analyses, which perform well in classification of vocal signals across species, and are especially well suited to high-dimensional data (Keen et al. Citation2021).
Method
Materials
Stimuli
We obtained human and chimpanzee vocalisations that were produced in 10 types of behavioural contexts: being attacked by another person/chimpanzee, being refused access to food, being separated from mother, being tickled, copulation/having sex, discovering a large food source, discovering something scary, eating high value/higher preference food, eating low-value/lower preference food, threatening an aggressive individual. Vocalisations produced in these contexts have been shown to serve specific biological and social functions in primates and other mammals (Townsend and Manser Citation2013).
Spontaneous human nonverbal vocalisations from each behavioural context were collected from Youtube.com by three naive research assistants, excluding videos with acted performances, like movies. These human nonverbal vocalisations were introduced in a previous study (Kamiloǧlu and Sauter Citation2022). In total, 200 vocalisations (20 for each context) were collected, each produced by a unique speaker (average duration (sec): 1.73; Sd = 0.82). Inclusion of vocalisations was based exclusively on 1) the eliciting situation matching the target behavioural context; 2) the presence of a nonverbal vocalisation that was clearly audible; and 3) only one person vocalising. The research assistants also considered suddenness, clarity, and certainty (see Anikin and Persson Citation2017): Sudden events offer minimal time for conscious posing or impression management, clear (unambiguous) situations minimise the risk of misunderstanding the target context, and the assistants selected videos that they were maximally certain reflected the target contexts. Chimpanzee vocalisations were taken from a previous study and included 155 recordings produced by 66 individual chimpanzees (average duration (sec): 1.45, Sd = 0.50) in the 10 behavioural contexts (Kamiloǧlu et al. Citation2020). The behavioural contexts were determined by author K.E.S., who is an expert on chimpanzee vocal communication. The behaviour of the caller, the response of individuals in the group or party, and the general behavioural context were noted for each vocalisation. Only vocalisations from single individuals whose identities were known used in this study. Details of the recording set-ups and dataset compilation procedures for the collection of chimpanzee vocalisations can be found in the Supplementary Material, Text 1S. A representative vocalisation for each context can be found for humans at https://emotionwaves.github.io/BehaviouralContexts/, and chimpanzees at https://emotionwaves.github.io/chimp/.
Human vocalisations included audio from YouTube which are typically in AAC (Advanced Audio Coding) format at bit rates between 128 and 384 kbps (kilobits per second) depending on the quality of the video (from 360p to 4k, respectively). Chimpanzee vocalisations were digitised at a sampling rate of 44.1 kHz, as mono files with 16 bits accuracy before the analysis. We subjected original chimpanzee recordings to AAC compression at 128 kbps, mimicking the YouTube audio compression, and compared acoustic features between these and the uncompressed versions. There were no significant differences in any features, indicating that the compression likely used in our YouTube data did not distort our analysis (see Supplementary Analysis Table S1). The number of female and male expressors per context for each species for the raw and final datasets (see Acoustic feature extraction and pre-processing section for data exclusion rules) is presented in .
Table 1. Number of tokens produced by female and male expressers per context for raw and final datasets.
Data analysis
Overview
Our analysis framework included three main steps (see for a detailed illustration of the framework), which were set up to allow us to reveal acoustically similar vocalisations produced by humans and chimpanzees in similar behavioural contexts. First, we extracted a large number of acoustic features from the human and chimpanzee vocalisations. Second, the dataset was subjected to pre-processing before similarity analysis: normalisation, exclusion of outliers, and balancing sex of expressers of the two species for each behavioural context. Third, we used two different methods for determining acoustic similarity of human and chimpanzee vocalisations: pairwise distance measures and separability metrics based on unsupervised learning. We considered vocalisations acoustically similar for a specific behavioural context across the two species if they were closer in terms of acoustic distance and acoustically less separable based on unsupervised clustering as compared to vocalisations from other contexts.
Figure 1. The analysis framework followed three main steps: 1) extraction of acoustic features from human and chimpanzee vocalisations that were produced in 10 matching behavioural contexts; 2) pre-processing of the audio data, and 3) testing acoustic differentiability of vocalisations for specific contexts between species using two techniques; vocalisations produced in specific contexts were identified as acoustically similar between the two species if verified by both methods.
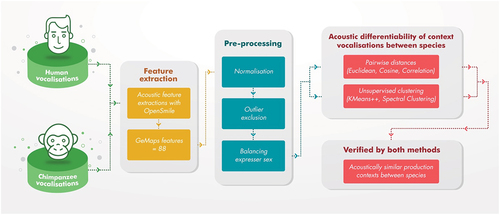
Acoustic feature extraction and pre-processing
We extracted 88 acoustic features for each audio recording from the extended version of the Geneva Minimalistic Acoustic Parameter Set (Eyben et al. Citation2016). GeMAPs is a standardised, open-source approach for assessing acoustic characteristics in emotional voice analysis. The acoustic elements encompass frequency, energy/amplitude, spectral balance, and temporal domains. Frequency domain features involve fundamental frequency components (associated with perceived pitch) as well as formant frequencies and bandwidths. Energy/amplitude characteristics pertain to the air pressure within the sound wave, which is perceived as volume. Spectral balance parameters, influenced by laryngeal and supralaryngeal movements, relate to the perceived voice quality. Finally, temporal domain features represent the duration and rate of voiced and unvoiced speech portions. In total, we extracted 88 acoustic attributes from these four domains. For each stimulus, the feature vector comprised the average of the entire audio clip.
Given that acoustic features are measured in a variety of units and ranges, we applied a 0–1 normalisation. Within each species, normalised data were then subjected to five multi-dimensional outlier detection algorithms using the Python PyOD library (Zhao et al. Citation2019): Angle-Based Outlier Detection (probabilistic), Fully Connected Auto Encoder (neural networks), Isolation Forest (outlier ensembles), k-nearest Neighbours (proximity-based) and One-Class Support Vector Machines (linear). Vocalisations flagged by three or more outlier detection algorithms were considered outliers (i.e. max. voting principle), resulting in the exclusion of 11 vocalisations (nhuman = 195, nchimp = 149; ntotal = 344). We also balanced the sex classes between chimpanzees and humans, because there are natural differences in the acoustics of vocalisations across sexes in both humans (e.g. Pisanski et al. Citation2016) and chimpanzees (e.g. Slocombe and Zuberbühler Citation2005). Given that the sex of the expressor was not balanced for chimpanzee vocalisations (see for details), we balanced sex classes (female and male) for pairs of species for each context such that the count difference was not more than 5 per context between human and chimpanzee expressors (see Figure S1 in Supplementary Materials). The final dataset included 313 vocalisations (nhuman = 165, nchimp = 148). Data exclusion and balancing sex classes allowed us to account for natural differences, and to focus on a subset of vocalisations that were representative of the population. We conducted the analysis not only on the final dataset but also on the raw dataset in order to make sure that data exclusion did not have an influence on the results. The number of tokens produced by female and male expressers per context is provided in . The acoustic characteristics of the vocalisations used in this study (duration, intensity, F0 mean, F0 minimum, F0 maximum, F0 Sd, Spectral Center of Gravity (SC0G) mean, and SCoG Sd; extracted using Praat (Boersma and Weenink Citation2011): are presented in for illustration purposes.
Pairwise distance comparisons
We computed average pairwise similarity across different contexts and between the two species employing three common distance measures: Euclidean, Cosine and Correlation distances. Using multiple distance measures can increase the robustness of results and provide confidence in the findings, especially if the same pattern is observed across different measures. Also, different distance measures have their own strengths and weaknesses, and using a combination of them can provide a more complete understanding of the data. For instance, Euclidean distance is simple but may not be appropriate for high-dimensional or outlier data, whereas Cosine distance is better suited for high-dimensional data but not for negative values. Correlation distance can be useful for different scales or outlier data, but assumes normal distribution. If the acoustic distance across the three measures is less between similar contexts produced by the two species then all other contexts, vocalisations of these contexts are considered as similar. For example, if the acoustic distance between vocalisations produced by humans when being separated and vocalisations produced in the same context by chimpanzees is less than the acoustic distance between the same human separation vocalisations and vocalisations produced in each of the other nine contexts by chimpanzees, then separation vocalisations are considered acoustically similar between the two species based on the acoustic distance measures. This was formalised as:
where i represents individuals, j is a particular context, and k are all contexts other than j.
Pairwise unsupervised clustering methods
We used two unsupervised clustering algorithms, KMeans++ (Arthur and Vassilvitskii Citation2006) and Spectral Clustering (Ng et al. Citation2001), to test the acoustic separability of context vocalisations between the two species. These clustering algorithms reveal to what extent pairs of contexts can be clustered based on the acoustic features of vocalisations produced in those contexts. The quality of pairwise clusters is determined by three well-accepted measures: Adjusted Rand Index (Hubert and Arabie Citation1985), Homogeneity Scores (Rosenberg and Hirschberg Citation2007), and Mutual Information (Strehl and Ghosh Citation2002). The Adjusted Rand Index measures the similarity between the pairwise clusters obtained from the clustering algorithm and the ground truth clusters, if available. The Homogeneity Scores measure how pure the pairwise clusters are, i.e. how well they contain only samples from one category. The Mutual Information measures how much information about the ground truth clustering is provided by the pairwise clusters obtained from the clustering algorithm. By using these three measures, we can provide a more comprehensive evaluation of the quality of the pairwise clusters obtained from the clustering algorithms. For all three measures, we evaluated the following comparison:
where k is a particular context and l are all contexts other than k.
For example, if vocalisations when being tickled are less separate between the two species than being tickled vocalisations of humans and the vocalisations produced by chimpanzees in other contexts, being tickled vocalisations were considered as acoustically similar.
Results
Pairwise distance comparisons: Euclidean, Cosine, and Correlation distances
The results revealed that vocalisations from two behavioural contexts were more similar to each other between the two species than vocalisations from all other contexts: Eating low-value food, and Threatening another. The results were highly consistent across the three distance measures, Euclidean, Cosine, and Correlation. Acoustic distances between human and chimpanzee vocalisations by Euclidean distance measure are illustrated in . Supplementary Materials Figure S2 illustrates Cosine and Correlation distance matrices; Figure S3 illustrates within-species distances assessed by the three distance measures in order to demonstrate (dis)similarity of acoustic structures between contexts for each species, separately.
Figure 3. Acoustic similarity evaluations. (a) pairwise Euclidean distance. Warmer (red) colours indicate smaller distance (i.e. greater similarity), and colder (blue) colours indicate larger distance (i.e. low similarity); (b) unsupervised clustering quality assessed by Adjusted Rand Index. Darker colours indicate better separability. In both measures, boxes with black borders show similar vocalisations produced in similar contexts by the two species.
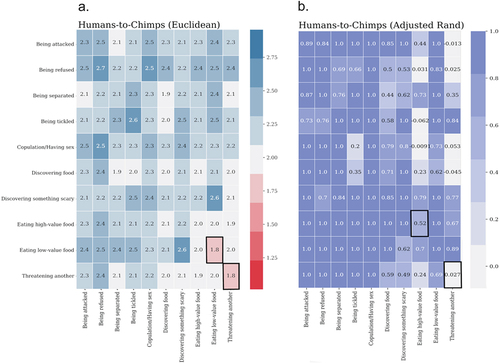
Unsupervised learning with two clusters
The three cluster quality algorithms showed that both KMeans++ and Spectral Clustering unsupervised clustering methods were consistent with each other (see Supplementary Materials Table S2). Between-species comparisons revealed that vocalisations produced in two contexts were less separable as compared to other contexts across the species: Eating high-value food and Threatening another (see ). These results were confirmed by the other quality indices, Homogeneity Index and Mutual Information (see Supplementary Materials Figure S4).
Vocalisations produced in one behavioural context were found to be acoustically similar using both types of analyses methods: Threatening another individual. A 2D representation based on the acoustic features of vocalisations produced in this context is shown in together with other contexts, using Principle Component Analysis (PCA). The 2D maps illustrate that vocalisations produced while threatening another individual are acoustically similar between the two species. We provide eGemaps features for human and chimpanzee vocalisations produced in the threat context in Supplementary Materials Table S3 for further inspection. The observed inter-specific acoustic similarity in threat vocalisations was consistent for the dataset without data exclusion, indicating the robustness of the findings. Pairwise Euclidean distance and Adjusted Rand Index matrices as well as 2D acoustics maps for the raw dataset are provided in Supplementary Materials Figure S5.
Figure 4. 2D acoustic maps of human and chimpanzee vocalisations produced while threatening another individual, constructed using Principal Component analysis (PCA). Blue data points are human vocalisations, orange data points are chimpanzee vocalisations. Vocalisations produced in threatening another context are acoustically similar between the two species. This similarity is illustrated with two other dimension reduction techniques (Spectral Embedding and t-SNE) with 2D acoustic maps (see Supplementary Materials Figure S6).
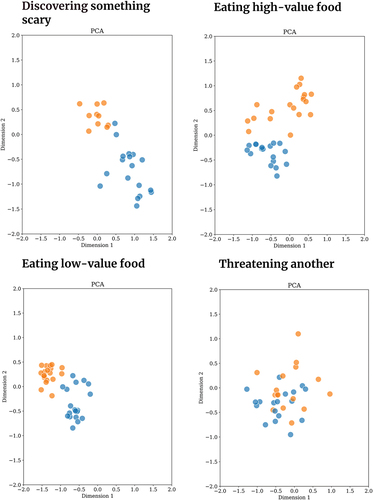
Discussion
We found that humans and chimpanzees produce acoustically similar vocalisations when they are threatening another individual. These results are robust across two different kinds of assessment methods, and offer clear evidence for preserved acoustic regularities in this type of nonverbal vocalisations. These results demonstrate acoustic similarity in threat vocalisations between humans and our nearest living relatives, providing evidence consistent with a shared origin in our last common ancestor.
Our multi-method analysis reveals robust acoustic similarity between human and chimpanzee vocalisations produced when threatening another individual. Harsh, low-frequency sounds with nonlinearities are used in threat contexts by many non-human species, contrasting with higher, more tonal frequencies often associated with affiliative contexts (Morton Citation1977). It has been suggested that threat vocalisations might convey the impression of larger body size, and therefore alter the level of perceived threat (Briefer Citation2012). Due to their inherently nonlinear nature, threat vocalisations also have the advantage that they prevent habituation (Karp et al. Citation2014). Our results reveal that key acoustic regularities of threat vocalisations are conserved across species, which is consistent with the idea that there is phylogenetic continuity in threat vocalisations. Future work could additionally examine whether equivalent acoustic patterns for threat vocalisations exist with bonobos. As chimpanzees and bonobos are equally phylogenetically distant from humans, such an examination would help to validate the phylogenetic continuity argument by ruling out an alternative convergent evolutionary scenario between humans and chimpanzees.
It is worth noting that our results do not mean that there is no acoustic similarity between human and chimpanzee vocalisations produced in other situations as there are several possible explanations for the null results for the other contexts. Firstly, one possibility is that vocalisations produced in some of the behavioural contexts included in our study might not be acoustically distinct from other within-species vocalisations. For instance, human vocalisations produced while eating high- and low-value foods were acoustically not well differentiated from each other (see Supplementary Materials Figures S3 and S7). Indeed, a recent study found that listeners often confuse these two contexts when asked to identify production context from human vocalisations (Kamiloǧlu and Sauter Citation2022). While listeners could accurately recognise the food context in general, they could not differentiate between high versus low value food. It is thus possible that human vocalisations show little differentiation based on the degree of food preference. Secondly, a closer look at the information communicated via particular contexts might be useful. For example, the same listening study found that listeners frequently confused vocalisations produced in situations where individuals were separated versus refused access to food, indicating that these situations may share similarities in the way they are expressed through vocalisations. In our results, these two contexts were least separable based on unsupervised clustering quality assessment (see ). It is thus possible that vocalisations produced in these situations might have common characteristics like loss of opportunity.
Thirdly, in detecting acoustically similar vocalisations between species, we applied a strict criterion of positive evidence from two different analyses approaches. There were differences between the results of different methods, and vocalisations produced in only one particular context satisfied this strict criterion. While distance measures do well with low-dimensional data, clustering algorithms work well with high-dimensional data, and they often require larger datasets (Keen et al. Citation2021). Given that our dataset is high-dimensional and not very big (n = 313), we opted to apply a strict criterion in order to make sure that our findings are not due to a specific analysis approach. In future studies, replication of our findings with a larger stimulus set would be useful since applying unsupervised analyses to a larger-scale empirical dataset might allow researchers to better capture acoustic diversity and similarity. Furthermore, given that online recordings often differ in audio quality due to variations in recording equipment and the presence of background noise, the use of larger datasets would help safeguard against the undue influence of any particular ‘noisy’ audio extraction on the overall analysis. This approach will allow us to better discern the systematic structure of acoustic data from diverse online sources, reducing the potential bias or confounding effects of specific, low-quality audio extractions.
In conclusion, our study reveals an intriguing acoustic similarity between human and chimpanzee threat vocalisations, yet such similarities were not discerned in the other contexts that we investigated. The reasons behind this could be due to our limited sample sizes hindering us from unearthing their distinct features. Thus, this study provides preliminary support for the evolutionary continuity hypothesis in terms of threat vocalisations. Its applicability to a broader spectrum of behavioural contexts remains a topic for future exploration. In the current study, we demonstrate that the acoustic form of human nonverbal vocalisations produced while threatening another individual is shared with chimpanzees, suggesting that threat vocalisations developed based on a phylogenetically ancient vocal signalling system.
Ethical statement
Collection of human vocalisations was approved by the Faculty Ethics Review Board of the University of Amsterdam, the Netherlands (project no. 2020-SP-11883), and the School of Psychology Ethics Committee, University of St Andrews gave ethical clearance for the non-invasive, behavioural studies that included the recording of chimpanzee vocalisations.
Data and script availability
Data and analysis scripts are available from https://osf.io/8xnd9/?view_only=5439fd42c6e94fe78a62f0c61985154e.
Supplemental Material
Download PDF (2.1 MB)Disclosure statement
No potential conflict of interest was reported by the author(s).
Supplementary material
Supplemental data for this article can be accessed online at https://doi.org/10.1080/09524622.2023.2250320.
Additional information
Funding
References
- Anikin A, Persson T. 2017. Nonlinguistic vocalisations from online amateur videos for emotion research: A validated corpus. doi: 10.3758/s13428-016-0736-y.
- Arthur D, Vassilvitskii S. 2006. K-means++: the advantages of careful seeding, stanford. https://theory.stanford.edu/~sergei/papers/kMeansPP-soda.pdf.
- Boersma P, Weenink D. 2011. Praat: doing phonetics by computer. http://www.praat. org/.
- Briefer EF. 2012. Vocal expression of emotions in mammals: mechanisms of production and evidence. J Zool. 288(1):1–20. doi: 10.1111/j.1469-7998.2012.00920.x.
- Bryant GA. 2021. The evolution of human vocal emotion. Emot Rev. 13(1):25–33. doi: 10.1177/1754073920930791.
- Darwin C. 1872. The expression of the emotions in man and animals. London: John Murray. doi: 10.1037/10001-000.
- Davila Ross M, Owren MJ, Zimmermann E. 2009. Reconstructing the evolution of laughter in great apes and humans. Curr Biol. 19(13):1106–1111. doi: 10.1016/j.cub.2009.05.028.
- Debracque C, Clay Z, Grandjean D, Gruber T. 2022. Humans recognize affective cues in primate vocalizations: acoustic and phylogenetic perspectives. bioRxiv. 2022.01.26.477864. doi:10.1101/2022.01.26.477864
- Eyben F, Scherer KR, Schuller BW, Sundberg J, André E, Busso C, Devillers LY, Epps J, Laukka P, Narayanan SS, et al. 2016. The Geneva Minimalistic Acoustic Parameter Set (GeMAPS) for voice research and affective computing. IEEE Trans Affective Comput. 7(2):190–202. doi: 10.1109/TAFFC.2015.2457417.
- Filippi P, Congdon JV, Hoang J, Bowling DL, Reber SA, Pašukonis A, Hoeschele M, Ocklenburg S, de Boer B, Sturdy CB, et al. 2017. Humans recognize emotional arousal in vocalizations across all classes of terrestrial vertebrates: evidence for acoustic universals. Proc R Soc B Biol Sci. 284(1859):20170990–20170999. doi: 10.1098/rspb.2017.0990.
- Hubert L, Arabie P. 1985. Comparing partitions. J Classif. 2(1):193–218. doi: 10.1007/BF01908075.
- Kamiloǧlu RG, Sauter D. 2022. Sounds like a fight: listeners can infer behavioural contexts from spontaneous nonverbal vocalisations. doi: 10.31219/osf.io/rt49v.
- Kamiloǧlu RG, Slocombe KE, Haun DBM, Sauter DA. 2020. Human listeners’ perception of behavioural context and core affect dimensions in chimpanzee vocalizations: perception of chimpanzee vocalisations. Proc R Soc B Biol Sci. 287(1929):20201148. doi: 10.1098/rspb.2020.1148.
- Karp D, Manser MB, Wiley EM, Townsend SW, Fusani L. 2014. Nonlinearities in meerkat alarm calls prevent receivers from habituating. Ethology. 120(2):189–196. doi: 10.1111/eth.12195.
- Keen SC, Odom KJ, Webster MS, Kohn GM, Wright TF, Araya-Salas M. 2021. A machine learning approach for classifying and quantifying acoustic diversity. Methods Ecol Evol. 2021(7):1213–1225. doi: 10.1111/2041-210x.13599.
- Laukka P, Elfenbein HA, Söder N, Nordström H, Althoff J, Chui W, Iraki FK, Rockstuhl T, Thingujam NS. 2013. Cross-cultural decoding of positive and negative non-linguistic emotion vocalizations. Front Psychol. 4(JUL):1–8. doi: 10.3389/fpsyg.2013.00353.
- Morton ES. 1977. On the occurrence and significance of motivation-structural rules in some bird and mammal sounds. Am Nat. 111(981):855–869. doi: 10.1086/283219.
- Ng A, Jordan M, Weiss Y. 2001. On spectral clustering: analysis and an algorithm. Adv Neural Inf Process Syst. 14. https://proceedings.neurips.cc/paper/2001/hash/801272ee79cfde7fa5960571fee36b9b-Abstract.html.
- Pisanski K, Cartei V, McGetrick J, Raine J, Reby D, Feinberg DR. 2016. Voice modulation: a window into the origins of human vocal control? Trends Cogn Sci. 20(4):304–318. doi: 10.1016/j.tics.2016.01.002.
- Rosenberg A, Hirschberg J. 2007. V-Measure: a conditional entropy-based external cluster evaluation measure. Proceedings of the 2007 Joint Conference on Empirical Methods in Natural Language Processing and Computational Natural Language Learning (EMNLP-CoNLL). p. 410–420. https://aclanthology.org/D07-1043.
- Scheumann M, Hasting AS, Kotz SA, Zimmermann E. 2014. The voice of emotion across species: how do human listeners recognize animals’ affective states? PLoS One. 9(3):1–10. doi: 10.1371/journal.pone.0091192.
- Slocombe KE, Zuberbühler K. 2005. Functionally referential communication in a chimpanzee. Curr Biol. 15(19):1779–1784. doi: 10.1016/j.cub.2005.08.068.
- Strehl A, Ghosh J. 2002. Cluster ensembles – a knowledge reuse framework for combining multiple partitions. J Mach Learn Res. 3:583–617.
- Townsend SW, Manser MB. 2013. Functionally referential communication in mammals: the past, present and the future. Ethology. 119(1):11. doi: 10.1111/eth.12015.
- Zhao Y, Nasrullah Z, Li Z. 2019. Pyod: a python toolbox for scalable outlier detection. arXiv Preprint arXiv: 1901.01588.