ABSTRACT
This editorial is the introduction to a special issue of Economic Systems Research on Input–Output Virtual Laboratories (IO VLs). The main purpose of this editorial is to explain the rationale for dedicating a special issue to this evolving niche of IO research, highlighting its potential to contribute to debates on topical policy issues. The first two sections review recent developments in the field of multi-regional input–output database compilation, followed by a brief description of the concept and architecture behind IO VLs. The seven papers chosen for this issue are then introduced. The concluding section finally outlines current challenges and future research avenues for IO VLs.
1. Introduction to this special issue
This special issue is dedicated to the compilation and operation of large-scale multi-regional input–output (MRIO)Footnote1 databases within virtual laboratories (VLs). By definition, a VL is a shared digital workspace offering a high-performance computing (HPC) architecture that can be accessed from computers connected to the internet anywhere in the world (Lenzen, Citation2014). The compilation of MRIO databases within such a VL space fosters collaboration amongst a large number of input–output (IO) practitioners since all computational tools for the MRIO compilation process are assembled within a single, easy-to-access virtual environment. The notion of an MRIO VL is conceptually novel but is also still a work in progress, as exemplified in this editorial and the papers in this special issue. Its increasing sophistication and national and regional policy relevance make it a promising niche of IO research, as foreseen by field experts (Dietzenbacher et al., Citation2013a; Lenzen, Citation2014).
2. The advent of MRIOs models
The evolution of the field of IO analysis from its origins in Leontief’s economic IO model in the 1930s to the broadening spectrum of applications over the years is well documented (Hewings and Jensen, Citation1987; Rose and Miernyk, Citation1989; Lahr, Citation1993; Dietzenbacher and Lahr, Citation2004; Bjerkholt and Kurz, Citation2006; Miller and Blair, Citation2009). The diversity of themes in recent special issues aptly reflects the blossoming of IO as a key analytical framework in the field of economic systems research and its sub-disciplines, with relevance to numerous research fields (Forssell and Polenske, Citation1998; Suh and Kagawa, Citation2005; Timmer and Aulin-Ahmavaara, Citation2007; Debresson, Citation2008; Wiedmann, Citation2009; Los and Steenge, Citation2010; Duarte and Yang, Citation2011; Tukker and Dietzenbacher, Citation2013; Inomata and Owen, Citation2014; Okuyama and Santos, Citation2014). In addition, IO has established itself as an invaluable practical planning tool widely used by governments, industry and other national and international organisations (Eurostat, Citation2008; Meng et al., Citation2013; OECD, Citation2015; United Nations, Citation2017).
IO databases must thus increasingly cater to numerous academic questions along with satisfying the needs of a growing specialist and non-specialist audience. Doing so successfully represents a financial and human-resource challenge, especially for understaffed or under-funded national statistical agencies, or individual research groups. New incentives for compiling large-scale MRIO databases more efficiently and collaboratively can help to alleviate these issues and ensure that future developments in data quality and analytical tools are not biased towards specific applications but remain as inclusive as possible.
The ongoing innovation and refinement of MRIO methods and databases is an ongoing focus in MRIO research (Inomata and Owen, Citation2014; Owen, Citation2017), one that is driven by the unfolding possibilities of IO applications and research avenues. A key achievement in the recent evolution of the field has been the large-scale development and widespread availability of several MRIO databases, signalling a shift away from single-region models towards global MRIO models (Giljum et al., Citation2008; Wiedmann et al., Citation2011; Andrew and Peters, Citation2013; Dietzenbacher et al., Citation2013b; Lenzen et al., Citation2013; Tukker and Dietzenbacher, Citation2013; OECD, Citation2015). Databases offering harmonised national input–output tables (IOTs) for several countries along with bilateral trade information have, in truth, existed for several decades, with the most prominent examples being GTAP (Narayanan et al., Citation2012), OECD (Yamano and Ahmad, Citation2006) and the Asian International IOTs (Meng et al., Citation2013). However, the use of MRIOs has only proliferated in the last decade since truly global MRIOs have become available (Tukker and Dietzenbacher, Citation2013).
Unlike single-region IO models, global MRIOs are able to shed light on the complex interdependencies in a globalised word, such as the outsourcing of production and associated environmental impacts, vertical specialisation or value added embedded in trade and global value chains (Hummels et al., Citation2001; Hertwich and Peters, Citation2009; Lenzen et al., Citation2012; Wiebe et al., Citation2012; Timmer et al., Citation2014; Los et al., Citation2015). Nevertheless, with large-scale global MRIO databases, new computational and other technical challenges have also emerged.
3. Taking on the challenge of constructing global MRIOs
One of the main challenges is that reliable global MRIOs can only be constructed if input data from multiple disparate sources are reconciled. This ideally requires rigorous manual data checking to ensure the highest possible degree of quality and reliability. Nevertheless, budget and staff constraints often preclude equally meticulous manual data handling for each available source dataset. A faster and cheaper alternative is advanced automated data handling and processing during MRIO compilation (Lenzen et al., Citation2013). This approach does come with its own set of limitations. Key challenges with regard to data handling – whether or not it has a manual or an automated focus – are: (a) different data sources are often reported in different formats (such as sectoral detail, year and price level); (b) the reliability and hence uncertainty range of each data source is often unknown; and (c) the original compilation method may have been different for each data source.
A handful of groups and consortia took on the challenge to build global MRIO databases that were unprecedented in size and level of detail. The participants of each project decided on a fixed regional and sectoral structure tailored to the anticipated application focus of the user community (Tukker et al., Citation2009, Citation2013; Dietzenbacher et al., Citation2013b). Economic Systems Research dedicated the special issue ‘Global multiregional input–output frameworks’ to this development. Although all of these databases were developed as global MRIOs, they differ in their intended purpose and level of detail, as well as in the way raw data were handled and processed to create the final MRIOs (Dietzenbacher et al., Citation2013b; Lenzen et al., Citation2013; Tukker et al., Citation2013). Significantly different outcomes were obtained when using the different databases to calculate results for the same research questions (Moran and Wood, Citation2014; Owen et al., Citation2014; Steen-Olsen et al., Citation2014). Owen (Citation2017) highlighted the choice of underlying raw data as another key contributor to these differences. This suggested that differences would still be observed between MRIO databases, even if the compilation approaches used were identical.
The Economic Systems Research special issue, ‘Comparative evaluation of MRIO databases’, was dedicated to investigating the differences between global MRIO databases. In their editorial, Inomata and Owen (Citation2014) likened the cumulative process of compiling global MRIOs to that of a global value chain, highlighting the crucial role as well as the complexity of the data compilation stage. Given this focus on the data compilation stage, researchers involved in constructing the major global MRIOs discussed a few years back whether the combined knowledge of the various global MRIO consortia could be harnessed to make the entire compilation process more efficient and economical. This led to an initial joint meeting amongst representatives of the different research consortia at the International Input–Output Association (IIOA) conference in 2010 in Sydney. There, they discussed the possibility of an overarching collaboration to exploit synergies and to streamline the construction process of large-scale MRIO databases. Initial funding for this collaboration was provided by the University of Sydney’s International Program Development Fund, and the first official meeting was held on the island of Réunion in 2011, giving this project its original name: Project Réunion (Tukker and Dietzenbacher, Citation2013).
Further meetings at the IDE-JETRO conference in 2012, and a meeting at Kurokowa Onsen in Japan following the IIOA conference in Kitakyushu in 2013, helped in shaping the direction of the project. Independently, an Australian research consortium led by the University of Sydney received funding through the Australian Government’s NeCTAR eResearch scheme (www.nectar.org) to build what was at the time most detailed subnational Australian input–output database. The aim of this project was closely related to that of Project Réunion. Specifically, it aimed at including the expertise of as many Australian IO researchers as possible into one project, in order to construct a subnational Australian MRIO framework that brings together the respective in-depth knowledge of each participating IO researcher in a single MRIO framework. This project was named the Australian Industrial Ecology Virtual Laboratory (Australian IELab). During the Australian IELab project, fundamental concepts for such an undertaking were developed (Lenzen et al., Citation2014). Project Réunion received funding through a grant from the Australian Research Council, and it was soon realised that the computational infrastructure that was being developed for constructing the subnational MRIOs within the Australian IELab project could be extended to contribute to one of the outcomes of Project Réunion: The Global Industrial Ecology Virtual Laboratory (Global MRIO Lab; Lenzen et al., Citation2017a).
4. Topic of this special issue: IO VLs
This special issue focuses on IO VLs and at the same time marks the finalisation of Project Réunion with special attention on the IO VL that constitutes the project’s main outcome: the Global MRIO Lab. The Global MRIO Lab shares large parts of its software code with other IO VL projects, and the software suite for these projects is referred to as the MRIOLab suite.
Throughout Project Reúnion and its associated projects, a number of VL types were defined, each with a unique function or purpose for a given project. Certain denominations are useful to distinguish different parts of the projects, and they are used interchangeably throughout this editorial and in the articles featured in this special issue. For the sake of clarity, these denominations are listed and defined in , which may serve as a reference to readers unfamiliar with this increasingly complex and ever-evolving nomenclature. The versatility of the MRIOLab suite is showcased in a number of articles that focus on IO VLs which, despite sharing the MRIOLab suite with the Global MRIO Lab, were developed independently from the Global MRIO Lab (Faturay et al., Citation2017; Lenzen et al., Citation2017b; Reyes et al., Citation2017; Wang Citation2017).
TABLE 1. IO VL denominations used in this special issue.
The MRIOLab suite was developed with two key Project Réunion principles in mind: (a) the ability to process arbitrary input data (provided these were available in the correct format) and (b) the ability to process conflicting data source in a fully automated one-step reconciliation process. Since the researchers who developed the MRIOLab suite developed the AISHA tool during the Eora project (Geschke et al., Citation2011; Lenzen et al., Citation2013), and because the tool fulfilled the two above-mentioned requirements, AISHA was chosen as a data processing engine for the MRIOLab suite. Due to the selection of AISHA as the data processing engine for the MRIOLab suite, it was impossible to fully replicate the diversity of MRIO construction processes that were used and developed for the other global MRIOs. Hence, whilst the Global MRIO Lab encompasses the collaborative ideas for the compilations of global MRIOs as they were envisaged in Project Réunion, it can neither be regarded nor was it ever intended to be a replacement for the different global MRIOs. It should therefore be considered as an academic experiment that offers one possible realisation of the initial motivations for Project Réunion. The Global MRIO Lab project is not the only new global MRIO project currently under development or nearing completion. At the time of writing, the DESIREFootnote2 project is being finalised and a special issue on DESIRE is being prepared for the Journal of Industrial Ecology.
In the remainder of this editorial, we offer a general overview of the MRIOLab suite approach, present each of the papers in the special issue and conclude with what are the most significant limitations and future research avenues emerging from the current phase of the MRIOLab suite development.
5. Building and operating a virtual laboratory for input–output analysis
During the initial concept phases of the MRIOLab suite, the initial MRIOLab suite development team (consisting of the researchers participating in the Australian subnational MRIO project, led by the ISA research group at the University of Sydney and the Sustainability Assessment Program at UNSW Sydney) defined a number of key features for the MRIOLab suite, which were in line with the aims of Project Réunion.
The key features of the MRIOLab suite are the following:
Streamlined raw data management. As discussed in the introduction, the different source datasets used in the construction of MRIOs usually come in vastly different data storage formats. In order for the MRIOLab suite to be able include a data source for the construction of an MRIO database, the data source must first be translated into an MRIOLab suite-compliant format. The function that carries out this data preparation is called a data feed.
Flexible MRIO structure. Once a data source is processed using a data feed, the MRIOLab suite is able to produce MRIOs in user-specified structures in terms of both regional and sectoral resolution without further source data preparation.Footnote3 The MRIOLab suite uses a unique approach in which each MRIO is compiled at the selected level of detail, rather than being an aggregation of a larger feedstock table, thus allowing additional constraints to be imposed during the table building process. The construction of each MRIO is subject to the choices that the MRIOLab suite user makes with regard to sectoral and regional detail, time series, and the raw data inputs to be used as constraints during the data compilation process. Having this level of control over the compilation process of a global MRIO allows users to tailor their MRIO to their specific needs. This has been identified as one of the most attractive features for users, enabling novel research of a wide policy relevance (Wiedmann et al., Citation2016).
High degree of automation. Following the preparation of raw data and the specification of the MRIO structure, the MRIOLab suite is able to produce an MRIO in a fully automated way without further user input (see illustration of the workflow in Figure ). The major advantage of this is saving time and resources and allowing quick updates of the database (as demonstrated by Lenzen et al. Citation2017b). However, the absence of manual data checks poses a challenge when it comes to quality control. For this reason, any further enhancements of this feature will require the development of techniques to improve quality assurance.
FIGURE 1. Schematic of the basic IELab workflow for global MRIOs.
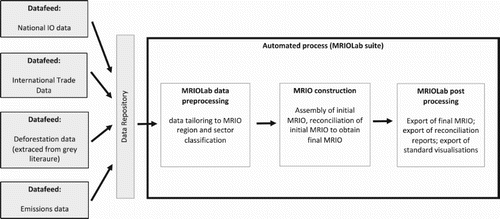
The computational demands of the single-step reconciliation approach require HPC architecture. But striving for full automation also means that the nuances entailed in the construction process in different available global MRIO databases could not be fully replicated within the MRIOLab suite (see, e.g., Reyes et al. Citation2017). An MRIO construction process consists of two main steps: (1) the assembly of an initial table (often referred to by its mathematical term initial estimate) and (2) the reconciliation (or balancing) of this table to adhere to pre-set conditions such as balancing conditions (see Figure ). The first of these two steps contains more intricate data analysis and as such, introduces a more project-specific ‘flavour’ to the MRIO. Within the MRIOLab suite’s workflow, the initial assembly of the final MRIO is part of the data preparation, and hence the different approaches for this step could – to the extent possible – be replicated within the MRIOLab structure (often referred to as initial estimate feed or IE feed). This initial assembly process tends to be significantly different between MRIOs.
The second step, reconciliation, is usually centred around a large-scale optimisation problem, and due to the nature of how this task is approached within the MRIOLab suite, HPC architecture has proven to be essential. This step, which is part of the automated construction process in the MRIOLab, requires an automated approach that does not require further user inputs once it has commenced. At the time of writing, the one-step reconciliation approach that is used within the AISHA tool was implemented in the MRIOLab, but other reconciliation routines such as SUT-RAS or commercial software packages would be suitable for this task as well.
The experiences during the Australian IELab and Global MRIO Lab have shown that this high level of automation is both a blessing and a curse. On the one hand, the vision of a full-scale collaboration was partly hindered by the complexity of the MRIOLab suite. In an ideal IO VL project, each set of raw data would be independently added to the project by the researcher who has the best knowledge of the particular dataset or relevant research field (e.g. trade, value added, employment, water, land use, greenhouse gases, etc.). Due to the complexity of the MRIOLab suite itself, newly developed data feed code must ensure specific data formats for the processed raw data. Otherwise, the MRIOLab suite cannot process these data (see box on the right in Figure ). Developing data feed code that adheres to these stipulations requires a good understanding of the workflow of the MRIOLab suite and advanced programming and computer knowledge.Footnote4 Wiedmann (Citation2017) elaborates on this as well as other challenges posed by the reliance on inputs from multiple individuals necessary for developing and continuously updating the data within the MRIOLab. On the other hand, Lenzen et al. (Citation2017b) demonstrate how readily data feeds can be implemented, thus highlighting the virtues of the MRIOLab suite workflow where the collaborative ethos is present.
At the same time, the data feed concept is one of the most successful and potent features of the MRIOLab suite by allowing a raw data integration standard to be defined. This, in turn, facilitates the integration of a diverse range of raw data types, including some from previously untapped sources. In the case of the Australian IELab especially, there has been an integration of a large number of data feeds based on data from the ‘grey literature’ (this may, e.g., provide valuable inputs to create a highly detailed or specialised satellite account such as the deforestation example shown in Figure ), in addition to the more ‘traditional’ data sources from the Australian Bureau of Statistics. The guidelines that the MRIOLab suite sets with regard to the integration of any raw data often require complex data feed codes, and the integration of these data codes also often requires a substantial level of support for the programmer. A major point of concern is how these data feeds will be maintained, documented and updated.Footnote5 The development of robust quality assurance measures and data updating strategies will become necessary to keep the global and the national MRIO Labs up to date and to ensure their long-term reliability.
Owing to the complexity of the MRIOLab suite, users must be assigned different roles depending on their level of contribution to the project. Standard users obtain basic access to the MRIOLab suite and can use the MRIOLab suite’s MRIO building capabilities, but do not contribute any input data. The support for standard users is usually limited to general input–output support if no previous input–output experience exists, but MRIO Lab-specific training is usually minimal. Data feed contributors gain access to the code repositories in order to contribute new data feed code, and they often require substantial support. Finally, MRIOLab administrators have full access to all MRIOLab suite and the hardware that houses the MRIOLab suite. Currently, only users with intimate knowledge of the MRIOLab suite can exercise this role, often supported by expert HPC administrators. While this classification of users is relevant to the MRIOLab suite, it would be preferable, in an ideal world, if a collaboration project in the spirit of Project Réunion would require less training and support for participating researchers, and hence allow for a more even distribution of MRIOLab-related work.
The various MRIO Labs currently in operation have highlighted the variety of different, often project-specific experiences and challenges that researchers encounter during development. The selection of papers in this special issue aptly demonstrates the variety of foci, aims and experiences associated with each individual MRIO Lab.
6. Articles in this special issue
This section briefly introduces each of the contributions to this special issue. The first paper is an overview of the Global MRIO Lab, followed by papers on the development and application of other MRIOLabs. The final two papers highlight the research outputs and policy relevance offered by the Australian IELab.
In the opening paper, Lenzen et al. (Citation2017a) introduce the application of the Virtual Laboratory concept to MRIO building, by emphasising the collaborative, wiki-style nature of the Global MRIO Lab as an alternative to tying global MRIO projects to specific institutions or consortia, thus avoiding potential duplication and saving resources. The authors highlight that the Global MRIO Lab allows for the construction of tailored MRIO tables, breaking from the norm of fixed regional and sectoral structures in past global MRIO projects. They offer an overview of the MRIO Lab data processing concepts, and discuss the role of the highly detailed root classification that acts as a feedstock for deriving MRIO tables tailored to specific research questions. The authors also explain the divergence of MRIO Lab workflows from those of fixed-structure MRIOs. In their results section, the authors present a number of global MRIOs in various classifications, generated for the year 2015 using the Global MRIO Lab using the Global MRIO Lab. The final sections of the paper provide a unique account of the perspectives, experiences and concerns of Project Réunion’s founding members.
Abd Rahman et al. (Citation2017) offer a detailed account on the logistics and challenges involved in transferring the WIOD database into the Global MRIO Lab environment. This Global MRIO Lab-based version is referred to as WIOD-Lab. The authors first provide an overview of the WIOD database and describe the workflow of the WIOD-Lab. By transferring the WIOD construction approach into the MRIOLab suite, the authors achieve a number of enhancements specific to the WIOD database that go beyond the standard features that the MRIOLab suite offers. The original WIOD database is constructed according to a multi-step process that considers a number of different data sources at different points in the workflow. This process was reconstructed within the Global MRIO Lab. Particular focus was placed on the construction of the initial estimate. In addition to carefully reconstructing the WIOD workflow, the authors introduced a number of data quality enhancements to WIOD-Lab compared to WIOD. To achieve this, the authors introduced standard deviations to the raw data, allowing the MRIOLab suite’s reconciliation engine to place more importance on more reliable data sources. Superior data from National Accounts were also introduced in the reconciliation process. The article includes a detailed comparison between the WIOD and WIOD-Lab databases and a discussion highlighting the steps in the WIOD construction process that could not be replicated in the WIOD-Lab as well as their respective influence on the differences between WIOD-Lab and the original WIOD database. The authors close with a discussion on how WIOD-Lab could extend the use of WIOD by allowing for flexible industry and geographical resolutions through time- and cost-efficient updates of the original WIOD database.
Reyes et al. (Citation2017) discuss the integration of the EXIOBASE2 database into the Global MRIO Lab, as a special case and a proof of concept. Bringing EXIOBASE2 into the Lab setting enables flexible compilation of EXIOBASE2-based MRIOs (EB2MRIO) without being constrained to the original recipe. The authors present a simplified compilation process of the more complex original procedure where the database’s most labour-intensive country detailing/harmonising segment of construction is skipped and the final EXIOBASE2 database is used as the starting point of the MRIO ‘replication’ in the Global MRIO Lab. They apply the MRIOLab suite’s compilation technique to two sets of underlying input data: pre-processed input data provided by the EXIOBASE2 team and final EXIOBASE2 tables. Following the construction of EB2MRIOs using the MRIOLab suite’s one-step reconciliation, these are compared to the original EXIOBASE2 tables using a number of matrix distance measures. Standard footprint calculations are also carried out using final EXIOBASE2 satellite data on all MRIOs. A detailed analysis of the adherences of the two different datasets to the constraints (or superior) data shows that the multi-step data reconciliation process employed for the original database yields a very good adherence to balancing constraints and country SUT data. The EB2MRIO exhibits better adherence to the various datasets but at the expense of adherence to the balancing constraints when compared to the original tables. The footprint results show that for the carbon, water and material footprints, the results are comparable across the EB2MRIOs and original EXIOBASE2 tables. A notable exception is the land footprint, which tends to differ significantly. The authors cite a number of reasons for this, with the main one being the concentration of direct land use to very few (mostly agricultural) sectors. The article highlights these discrepancies as an important area of further research.
Faturay et al. (Citation2017) present the construction of the Indonesian MRIOLab (IndoLab), the first subnational MRIO framework for Indonesia. As a large archipelago, Indonesia features a large economic and environmental diversity across its territory, thus any investigation of socio-economic and environmental policy questions must account for this diversity. The IndoLab presents a major leap forward in socio-economic data modelling for Indonesia, since the vast majority of studies in the past were based on national IO data that do not offer any regional specificity. Studies that did employ a regional distinction did so at a comparatively crude level compared to the IndoLab. In order to achieve this high level of detail, the author’s labour survey data, which were available in the IndoLab’s most detailed classification, served as the main proxy database for the regionalisation. The authors showcase the completed IndoLab by compiling a subnational MRIO for Indonesia providing detailed information about the Indonesian domestic economy, a full set of financial flows between nine sectors across eight regions, and their economic interdependencies. The article concludes with a discussion on potential applications of the IndoLab by businesses and policy-makers, including the need for quantifying the impacts of regional policy incentives on the rest of the country.
Wang (Citation2017) offers an insider’s view into the development of a Chinese IELab. China is perhaps the finest example of a country where the enormous interregional diversity in economic structure creates an urgent need for a highly disaggregated subnational table. Wang argues that the level of sectoral and regional disaggregation in the official provincial single-region input–output table series released every five years by the National Bureau of Statistics of China does not cater to specific regional policy applications. This is cited as the reason behind the fact that the majority of existing studies concentrate mostly on environmental issues at the provincial or national level. Furthermore, the country’s rapid economic growth necessitates an annually updated time series. The author describes how the Australian IELab workflow was adapted to the Chinese context, with employment data used as a proxy for regionalisation. The article concludes with a case study of a topical regional policy issue, namely the potential supply-chain impacts of a relocation of Beijing’s noncapital functions to neighbouring cities, as envisaged in the Beijing–Tianjin–Hebei Coordinated Development Strategy. Using the Chinese MRIO Lab, the author generates a tailored Beijing–Tianjin–Hebei MR SUT at the city level for 2014 and employs structural path analysis to identify and rank the most significant regional structural paths with regard to embodied employment. The results highlight the importance of considering both direct and indirect employment generation at a highly disaggregated regional and sectoral level in order to ensure that sectors generating large employment multipliers are prioritised during the regional policy implementation process.
Lenzen et al. (Citation2017b) offer a detailed account on how recent economic and environmental datasets released by the Australian Bureau of Statistics towards the end of 2016 were used to provide a timely update of the Australian IELab. The authors emphasise how the capability of rolling out updates swiftly and efficiently makes the MRIOLab suite suitable for conducting timely and flexible regional and national impact analysis to support evidence-based policy on topical issues, using a suite of available economic, environmental and social indicators. The article presents results from sensitivity analyses aiming to, firstly, test the Australian IELab’s ability to regionalise official IO data and to, secondly, ascertain the usefulness of the flexible regionalisation option across different indicators. The authors also present a topical regional case study by calculating the direct and indirect value added employment effects (including inter-state spillovers) associated with the boom and subsequent slowdown of mining in Western Australia during 2008–2015. The case study supports previous findings that value added and employment multipliers are mostly restricted to WA sectors, with some spillovers to sectors such as trade, transport, finance and technical services in the larger states.
Wiedmann (Citation2017) reviews published applications of the Australian IELab to establish the extent to which the Australian IELab has truly enabled new IO-related research not possible using previously available tools and datasets. The article begins with a nontechnical introduction to the collaborative ethos of the Australian IELab as well as its unique analytical features (amongst these are the high degree of regional and sectoral disaggregation and flexibility, balancing, heatmaps, diagnostic tools and uncertainty estimates). The main empirical section of the article presents the results of a survey sent out to the first authors of 30 studies published in peer-reviewed journals and conference proceedings. The author finds that two-thirds (20) of the studies were truly enabled by the Australian IELab with the majority of other respondents (6) admitting that while their studies would have been possible without the Australian IELab, considerable additional resources would have been required. The article concludes by stressing the enormous potential of the Australian IELab to enable wider collaboration between diverse disciplines but also by reiterating some of the challenges highlighted in this editorial, namely that the Australian IELab is still currently a tool for expert users and that its success ultimately relies on the willingness and generous time and intellectual contribution of its community.
In addition to the articles featured in this issue, various MRIOLabs have already seen a strong uptake by the research community. Wiedmann (Citation2017) presents a review of all MRIOLab-related publication (as of January 2017 including the articles featured in this special issue) and counts 30 articles in total.
7. Conclusions, future research and outlook
The currently operational MRIOLabs presented in this special issue, along with the growing number of applications based on the Australian IELab, demonstrate that the VL concept can make a meaningful contribution to the field of large-scale MRIO compilation (Lenzen, Citation2014; Wiedmann, Citation2017). The standardisation of the raw data integration process, in combination with the highly automated workflow for MRIOs, reduces the labour-intensity involved in constructing large-scale MRIOs. This makes it easier to share the efforts that would normally arise from raw data preparation across a number of MRIOs. At the same time, VLs remain flexible to incremental improvements by users who see IO-based VLs as engines to create reliable and highly disaggregated (spatially, sectorally and temporally) MRIO databases along with a complete set of economic, environmental and social extensions. As already discussed, the MRIOLab suite presents one of several possible ways forward in the spirit of Project Réunion. Nevertheless, the focus on high automation also poses certain limitations on the current MRIOLab suite that require more research in the future.
TABLE 2. List of all MRIOLab projects as of March 2017.
Many of the aforementioned advantages of the MRIOLab suite pose significant practical challenges. The MRIOLab suite as a whole is currently too complex to allow a full distribution of data work amongst participating researchers. Core tasks such as administrative supervision and data feed/MRIOLab suite integration are currently handled by a handful of expert users. Identifying the training needs of new data feed developers will allow the MRIOLab to become less dependent on a handful of expert MRIOLab users that currently fill this role. As of April 2017, projects are well advanced in their planning stage to build the first MRIOLab that is mainly supported by a former data feed developer who has now gained the knowledge and experience to act in a support role (see Malaysia MRIO in Table ). provides a brief global overview of the MRIOLab projects at different stages of development as of April 2017. The future will show if this is sufficient, or whether the concepts for MRIOLab data feeds will need to be revised to offer a more comprehensive and structured introduction to data feed development.
At present, there is no standardised framework for quality assurance. Although quality assurance has been carried out (and as of April 2017 is still being carried out) for the data feeds of the Australian IELab (Lenzen et al. Citation2017b) and the Global MRIO Lab (Abd Rahman et al. Citation2017), there is currently no defined strategy on this issue. Only rigorous quality assurance and testing can ensure that the MRIOLab approach will continue to build confidence amongst the research community, and the strategies to undertake this quality assurance must be sufficient and transparent. This becomes especially important when input data include grey literature or other data sources not subjected to the scrutiny of official statistical offices.
The avoidance of collective memory loss must also be addressed in the future. How will the MRIOLab community handle data feed updates and maintenance if researchers leave an MRIOLab project? Is written documentation enough, or should other technologies such as ‘IELab Wikipedia’ be employed? And finally, it should be thoroughly examined how other approaches for harmonising MRIO construction that do not rely on such a high degree of automation as the MRIOLab suite compare in terms of performance and accuracy. This could take place in a similar vein to previous comparisons between MRIO databases in the recent past (Inomata and Owen, Citation2014). As with any new technology – such as VLs – there comes a new set of research possibilities but also challenges, especially with regard to quality assurance and safeguarding the collaborative ethos that VLs rely on for updates and improvements.
Future plans of the MRIOLab suite include a number of MRIOLab incentives, mainly aimed at highly detailed national MRIOLabs with great potential to readily inform topical issues in regional policy-making and state-level decision-making, as illustrated in the papers by Wang (Citation2017), Lenzen et al. (Citation2017b) and Faturay et al. (Citation2017). While there is still a long road ahead in terms of streamlining and quality testing, we believe that the contributions in this special issue aptly demonstrate that VLs offer a promising research niche within the field of IO. Their main advantages are a high degree of automation and flexibility allowing for regular updating and tailoring of IO databases. This allows tackling diverse topical research and policy issues, especially where resources and datasets would otherwise be scarce.
Acknowledgements
We would like to thank all the contributing authors as well as Manfred Lenzen, Bart Los, Michael Lahr and Thomas Wiedmann for their comments on an early draft of this editorial.
Disclosure statement
No potential conflict of interest was reported by the authors.
ORCID
Michalis Hadjikakou http://orcid.org/0000-0002-3667-3982
Arne Geschke http://orcid.org/0000-0001-9193-5829
Notes
1 We adopt the term MRIO here to encompass both multi-regional input–output tables and multi-regional supply-use tables.
3 The TERM model (Wittwer and Horridge, Citation2010), developed prior to the MRIOLab, is a subnational Australian MRIO that also offers a variable and highly disaggregated sector structure. TERM is static, however, as researchers need to aggregate to the level of detail suitable for their research question.
4 Experience has shown that even experienced programmers require a certain amount of support by the MRIOLab suite development team to successfully incorporate new data feed code into the MRIOLab suite.
5 The current strategy is that each data feed provider is asked to supply a detailed manual which covers the workflow of the data feed as well as instructions on how to update it to consider newly available data.
References
- Abd Rahman M.D., B. Los, A. Geschke, Y. Xiao, K. Kanemoto and M. Lenzen (2017) A Flexible Adaptation of the WIOD database in a Virtual Laboratory. Economic Systems Research, 29, 187–208.
- Andrew, R.M. and G.P. Peters (2013) A Multi-Region Input–Output Table Based on the Global Trade Analysis Project Database (GTAP-MRIO). Economic Systems Research, 25, 99–121. doi: 10.1080/09535314.2012.761953
- Bjerkholt, O. and H.D. Kurz (2006) Introduction: The History of Input–Output Analysis, Leontief’s Path and Alternative Tracks. Economic Systems Research, 18, 331–333. doi: 10.1080/09535310601020850
- Debresson, C. (2008) China’s Growing Pains – Recent Input–Output Research in China on China: Foreword. Economic Systems Research, 20, 135–138. doi: 10.1080/09535310802075257
- Dietzenbacher, E. and M.L. Lahr (2004) Wassily Leontief and Input–Output Economics. Cambridge, Cambridge University Press.
- Dietzenbacher, E., M. Lenzen, B. Los, D. Guan, M.L. Lahr, F. Sancho, S. Suh and C. Yang (2013a) Input–Output Analysis: The Next 25 Years. Economic Systems Research, 25, 369–389. doi: 10.1080/09535314.2013.846902
- Dietzenbacher, E., B. Los, R. Stehrer, M. Timmer and G. de Vries (2013b) The Construction of World Input–Output Tables in the WIOD Project. Economic Systems Research, 25, 71–98. doi: 10.1080/09535314.2012.761180
- Duarte, R. and H. Yang (2011) Input–Output and Water: Introduction to the Special Issue. Economic Systems Research, 23, 341–351. doi: 10.1080/09535314.2011.638277
- Eurostat (2008) Eurostat Manual of Supply, Use and Input–Output Tables, 2008 Edition. Luxembourg, Office for Official Publications of the European Communities.
- Faturay, F., M. Lenzen and K. Nugraha (2017) A New Sub-National Multi-Region Input–Output Database for Indonesia. Economic Systems Research, 29, 234–251.
- Forssell, O. and K.R. Polenske (1998) Introduction: Input–Output and the Environment. Economic Systems Research, 10, 91–97. doi: 10.1080/09535319808565468
- Geschke, A., D. Moran, K. Kanemoto and M. Lenzen (2011) AISHA: A Tool for Constructing Time Series and Large Environmental and Social Accounting Matrices Using Constrained Optimisation. 19th International Input–Output Conference, Alexandria, USA. http://www.iioa.org/Conference/19th-downablepaper.htm
- Giljum, S., C. Lutz and A. Jungnitz (2008) The Global Resource Accounting Model (GRAM): A Methodological Concept Paper. Vienna, Sustainable Europe Research Institute.
- Hertwich, E.G. and G.P. Peters (2009) Carbon Footprint of Nations: A Global, Trade-Linked Analysis. Environmental Science & Technology, 43, 6414–6420. doi: 10.1021/es803496a
- Hewings, G.J.D. and R.C. Jensen (1987) Chapter 8 Regional, Interregional and Multiregional Input–Output Analysis. Handbook of Regional and Urban Economics, 1, 295–355. doi: 10.1016/S1574-0080(00)80011-5
- Hummels, D., J. Ishii and K.-M. Yi (2001) The Nature and Growth of Vertical Specialization in World Trade. Journal of International Economics, 54, 75–96. doi: 10.1016/S0022-1996(00)00093-3
- Inomata, S. and A. Owen (2014) Comparative Evaluation of MRIO Databases. Economic Systems Research, 26, 239–244. doi: 10.1080/09535314.2014.940856
- Lahr, M.L. (1993) A Review of the Literature Supporting the Hybrid Approach to Constructing Regional Input–Output Models. Economic Systems Research, 5, 277–293. doi: 10.1080/09535319300000023
- Lenzen, M. (2014) An Outlook into a Possible Future of Footprint Research. Journal of Industrial Ecology, 18, 4–6. doi: 10.1111/jiec.12080
- Lenzen, M., D. Moran, K. Kanemoto, B. Foran, L. Lobefaro and A. Geschke (2012) International Trade Drives Biodiversity Threats in Developing Nations. Nature, 486, 109–112. doi: 10.1038/nature11145
- Lenzen, M., D. Moran, K. Kanemoto and A. Geschke (2013) Building Eora: A Global Multi-Region Input–Output Database at High Country and Sector Resolution. Economic Systems Research, 25, 20–49. doi: 10.1080/09535314.2013.769938
- Lenzen, M., A. Geschke, T. Wiedmann, J. Lane, N. Anderson, T. Baynes, J. Boland, P. Daniels, C. Dey, J. Fry, M. Hadjikakou, S. Kenway, A. Malik, D. Moran, J. Murray, S. Nettleton, L. Poruschi, C. Reynolds, H. Rowley, J. Ugon, D. Webb and J. West (2014) Compiling and Using Input–Output Frameworks Through Collaborative Virtual Laboratories. Science of the Total Environment, 485-486, 241–251. doi: 10.1016/j.scitotenv.2014.03.062
- Lenzen M, A. Geschke, M.D. Abd Rahman, M. Daaniyall, Y. Xiao, J. Fry, R. Reyes, E. Dietzenbacher, S. Inomata, K. Kanemoto, B. Los, D. Moran, H. Schulte in den Bäumen, A. Tukker, T. Walmsley, T. Wiedmann, R. Wood and N. Yamano (2017a) The Global MRIO Lab – Charting the World Economy. Economic Systems Research, 29, 158–186.
- Lenzen M., A. Geschke, A. Malik, J. Fry, J. Lane, T. Wiedmann, S. Kenway, K. Hoang and A. Cadogan-Cowper (2017b) New Multi-Regional Input–Output Databases for Australia – Enabling Timely and Flexible Regional Analysis. Economic Systems Research, 29, 275–295.
- Los, B. and A.E. Steenge (2010) Tourism Studies and Input–Output Analysis: Introduction to a Special Issue. Economic Systems Research, 22, 305–311. doi: 10.1080/09535314.2010.525741
- Los, B., M.P. Timmer and G.J. de Vries (2015) How Global are Global Value Chains? A New Approach to Measure International Fragmentation. Journal of Regional Science, 55, 66–92. doi: 10.1111/jors.12121
- Meng, B., Y. Zhang and S. Inomata (2013) Compilation and Applications of IDE-JETRO’s International Input–Output Tables. Economic Systems Research, 25, 122–142. doi: 10.1080/09535314.2012.761597
- Miller, R.E. and P.D. Blair (2009) Input–Output Analysis: Foundations and Extensions. Cambridge, Cambridge University Press.
- Moran, D. and R. Wood (2014) Convergence Between the EORA, WIOD, EXIOBASE, and OPENEU’s Consumption-Based Carbon Accounts. Economic Systems Research, 26, 245–261. doi: 10.1080/09535314.2014.935298
- Narayanan, B., A. Aguiar and R. McDougall (2012) Global Trade, Assistance, and Production: The GTAP 8 Data Base. West Lafayette, IN, Center for Global Trade Analysis, Purdue University.
- OECD (2015) Inter-Country Input–Output (ICIO) Tables, edition 2015. Inter-Country Input–Output (ICIO) Tables, edition 2015.
- Okuyama, Y. and J.R. Santos (2014) Disaster Impact and Input–Output Analysis. Economic Systems Research, 26, 1–12. doi: 10.1080/09535314.2013.871505
- Owen, A. (2017) Techniques for Evaluating the Differences in Multiregional Input–Output Databases: A Comparative Evaluation of CO2 Consumption-Based Accounts Calculated Using Eora, GTAP and WIOD. Cham, Switzerland, Springer.
- Owen, A., K. Steen-Olsen, J. Barrett, T. Wiedmann and M. Lenzen (2014) A Structural Decomposition Approach to Comparing MRIO Databases. Economic Systems Research, 26, 262–283. doi: 10.1080/09535314.2014.935299
- Reyes R.C., A. Geschke, A. de Koning, R. Wood, T. Bulavskaya, K. Stadler, H. Schulte in den Bäumen and A. Tukker (2017) The Virtual IELab – An Exercise in Replicating Part of the EXIOBASE V.2 Production Pipeline in a Virtual Laboratory. Economic Systems Research, 29, 209–233.
- Rose, A. and W. Miernyk (1989) Input–Output Analysis: The First Fifty Years. Economic Systems Research, 1, 229–272. doi: 10.1080/09535318900000016
- Steen-Olsen, K., A. Owen, E.G. Hertwich and M. Lenzen (2014) Effects of Sector Aggregation on CO2 Multipliers in Multiregional Input–Output Analyses. Economic Systems Research, 26, 284–302. doi: 10.1080/09535314.2014.934325
- Suh, S. and S. Kagawa (2005) Industrial Ecology and Input–Output Economics: An Introduction. Economic Systems Research, 17, 349–364. doi: 10.1080/09535310500283476
- Timmer, M.P. and P. Aulin-Ahmavaara (2007) New Developments in Productivity Analysis Within an Input–Output Framework: An Introduction. Economic Systems Research, 19, 225–227. doi: 10.1080/09535310701571828
- Timmer, M.P., A.A. Erumban, B. Los, R. Stehrer and G.J. de Vries (2014) Slicing Up Global Value Chains. Journal of Economic Perspectives, 28, 99–118. doi: 10.1257/jep.28.2.99
- Tukker, A. and E. Dietzenbacher (2013) Global Multiregional Input–Output Frameworks: An Introduction and Outlook. Economic Systems Research, 25, 1–19. doi: 10.1080/09535314.2012.761179
- Tukker, A., E. Poliakov, R. Heijungs, T. Hawkins, F. Neuwahl, J.M. Rueda-Cantuche, S. Giljum, S. Moll, J. Oosterhaven and M. Bouwmeester (2009) Towards a Global Multi-Regional Environmentally Extended Input–Output Database. Ecological Economics, 68, 1928–1937. doi: 10.1016/j.ecolecon.2008.11.010
- Tukker, A., A. de Koning, R. Wood, T. Hawkins, S. Lutter, J. Acosta, J.M. Rueda Cantuche, M. Bouwmeester, J. Oosterhaven, T. Drosdowski and J. Kuenen (2013) EXIOPOL – Development and Illustrative Analyses of a Detailed Global MR EE SUT/IOT. Economic Systems Research, 25, 50–70. doi: 10.1080/09535314.2012.761952
- United Nations (2017) System of Environmental-Economic Accounting 2012 – Applications and Extensions. New York, United Nations.
- Wang (2017) An Industrial Ecology Virtual Framework for Policy Making in China. Economic Systems Research, 29, 252–274.
- Wiebe, K.S., M. Bruckner, S. Giljum and C. Lutz (2012) Calculating Energy-Related CO2 Emissions Embodied in International Trade Using a Global Input–Output Model. Economic Systems Research, 24, 113–139. doi: 10.1080/09535314.2011.643293
- Wiedmann, T. (2009) Editorial: Carbon Footprint and Input–Output Analysis – An Introduction. Economic Systems Research, 21, 175–186. doi: 10.1080/09535310903541256
- Wiedmann, T. (2017) An Input–Output Virtual Laboratory in Practice – Survey of Uptake, Usage and Applications of the First Operational IELab. Economic Systems Research, 29, 296–312.
- Wiedmann, T., H.C. Wilting, M. Lenzen, S. Lutter and V. Palm (2011) Quo vadis MRIO? Methodological, Data and Institutional Requirements for Multi-Region Input–Output Analysis. Ecological Economics, 70, 1937–1945. doi: 10.1016/j.ecolecon.2011.06.014
- Wiedmann, T.O., G. Chen and J. Barrett (2016) The Concept of City Carbon Maps: A Case Study of Melbourne, Australia. Journal of Industrial Ecology, 20, 676–691. doi: 10.1111/jiec.12346
- Wittwer, G. and M. Horridge (2010) Bringing Regional Detail to a CGE Model Using Census Data. Spatial Economic Analysis, 5, 229–255. doi: 10.1080/17421771003730695
- Yamano, N. and N. Ahmad (2006) The OECD Input–Output Database. 2006/08, Paris, OECD Publishing.