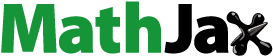
Abstract
The main objective of this paper is to revisit the Euro method in a critical and constructive way. We have analysed some arguments against the Euro method published recently in the literature as well as some other relevant aspects of the SUT-Euro and SUT-RAS methods not covered before. Although not being the Euro method perfect, we believe that there is still space for the use of the Euro method in updating/regionalizing Supply and Use tables.
1. Background
The article entitled ‘On the Euro method’ (Temursho, Citation2020) would not exist had we (Valderas-Jaramillo et al., Citation2019) not published ‘Projecting Supply and Use tables: new variants and fair comparisons’ in this journal. In our original piece, we empirical compare variants of the SUT-RAS and the SUT-Euro methods for projecting Supply and Use tables (SUTs). Moreover, unlike in Temurshoev and Timmer (Citation2011), we used the same exogenous information in both cases. In our piece, we show that the SUT-RAS method performs better than the SUT-Euro when industry outputs are known, while the SUT-Euro method performs better than SUT-RAS when industry outputs are unknown.
Notwithstanding the insights and developments revealed by Temursho about the details of the SUT-Euro method in its different variants (most of them already familiar to Euro users to some extent) and his excellent mathematical formalizations, we believe that none of the arguments that we (Valderas-Jaramillo et al., Citation2019) presented are questioned. Actually, we find that some of Temursho’s thoughts on related aspects require further clarification and yet others are simply misleading. In particular, Temursho incorrectly considers GRAS-1 comparable to the endo-SUT-RAS method; rather, it is equivalent to the exo-SUT-RAS method, as we elaborate upon later.
2. Discussion points
2.1. Endogenous and exogenous estimates
On page 14, Temursho (Citation2020) states that the output by product and the Supply matrix ‘are given exogenously (i.e. outside the Euro method’s algorithm) … obtained from … [equation] (9)’. This is done, despite that the constant market-share hypothesis has always been part of the core of all the existing Euro versions (Beutel, Citation2002, Citation2008; Valderas-Jaramillo et al., Citation2019), and commodity output (q) is estimated in the same way as in the standard Euro method (see equations 1.14 and 1.8 in Temursho, Citation2020). Indeed, as a result, the Supply and Use matrices are jointly derived as an integrating part of the updating process even though Temursho states that the Euro method fails to fulfil this as one of its advantages listed in Eurostat (Citation2008).Footnote1 This statement contrasts with Equation 15 in which he estimates industry output and, subsequently, he, henceforth, calls this approach the new GRAS variant or ‘GRAS-1’. Nowhere does he use the term ‘exogenous’ for this estimate of industry output in GRAS-1, although it may be perhaps implicitly assumed. In any case, if the industry outputs are endogenously determined in the GRAS method, then it is evidently beyond the GRAS-1 method, as GRAS-1/exo-SUT-RAS algorithm accepts output as exogenous only.
We show in section 2.2 that Temursho provides a good example of a reliable estimation using available data. As he mentions, it is always possible to find any other method that provides us with a good estimation of output by industry. Hence, one way or the other, GRAS-1 takes this output as given, and it is exogenous to the SUT-RAS setup. In fact, this is precisely what national statistical offices do in making their official macroeconomic forecasts. In our view, it makes no difference who estimates the industry outputs or how they are produced (simple or complex methods) as long as they are reliable. In the end, if we manage to get a reliable estimate of industry output, Temursho advocates something like the exo-SUT-RAS method. In summary, Temursho’s GRAS-1 method is something akin to our (Valderas-Jaramillo et al., Citation2019) exo-SUT-RAS method, in which the official macroeconomic forecast of industry outputs are replaced by alternative estimates.
2.2. Indirect estimation of industry output
Temursho (Citation2020, p. 20) estimates industry output via a simple method based on pre-existing industry output data and the corresponding GVA/output ratio of the base year (his equation 15). But this output is not endogenous to the SUT-RAS process. Interestingly enough, we wind up showing that his simple output generation method can yield rather good estimates. Despite this, it is not so clear that GRAS-1 outperforms SUT-Euro.
A brief inspection of the Spanish SUTs that Temursho uses suggests that his simple estimates are quite accurate – close to the official macroeconomic forecast growth rates.Footnote2 Indeed, as shown in the supplementary documentation (section A1), growth in gross value added is highly correlated with output growth and stable for Spain between 2000–2007 and 2008–2015. As such, the better the industry output estimate (closer to the official macroeconomic forecast), ‘the closer the results will be to those of GRAS with available industry output … ’ (Temursho, Citation2020, p. 19). Thus, in the end, this good estimate of industry output leads to great similarity in algorithm performance, Temursho eventually compares (cf. Temurshoev & Timmer, Citation2011) updating methods using different exogenous information, i.e. the endo-SUT-Euro method and the GRAS-1/exo-SUT-RAS method. In Valderas-Jaramillo et al. (Citation2019), we achieved parallel findings. Thus, when reliable estimates of output by industry exist, the exo-SUT-RAS or GRAS-1 methods perform better used than do SUT-Euro setups. If such a reliable estimates of output by industry do not exist, it is clear that the endo-SUT-Euro approach performs better than all others considered by Valderas-Jaramillo et al. (Citation2019). Hence, Temursho supports and even reinforces our findings.
2.3. National or domestic concept?
An additional issue concerns the practical applicability of both the SUT-Euro and the SUT-RAS methods. This heavily depends on how their input data requirements match up against ‘actual data’ in official macroeconomic forecasts.
The United Nations System of National Accounts (SNA) establishes that final demand macroeconomic forecasts in SUTs should be valued in national terms.Footnote3,Footnote4 On the contrary, in SUT tables the product breakdown typically applied is in domestic terms.Footnote5,Footnote6 Hence, SUTs can be projectedFootnote7 under both national and domestic concepts. To align them with the domestic concept of SUT tables, macroeconomic forecasts require some adjustment to convert import totals and final demand categories from national to domestic terms in SUT-Euro and SUT-RAS methods. If no additional expert informationFootnote8 is available beyond ordinary macroeconomic forecasts, conversion can be achieved by assuming constant national/domestic ratios:
(1)
(1)
This issue is typically not discussed in the literature; so, it is usually unclear whether the national or domestic concept is used to define final demand in purchasers’ prices and/or whether any other transformations are applied.Footnote9 This is important, since there can be major differences in the evolution can emerge across national and domestic consumption and exports figures, especially in countries that depend heavily on tourism.
In this sense, it is possible to perform the SUT-RAS projection method in domestic terms using nationally based targets without need for further transformations. With such purpose, we revisit Temursho’s (Citation2020) Equation 14 for unknown target totals of CIF-FOB adjustments, direct purchases abroad and non-residents’ purchases in domestic territory.
(2)
(2)
Three additional rows were added to Temursho’s (Citation2020) framework. Two of them to account for the DPA and NRPT adjustments made in the import, household consumption and export vectors; and an additional row to account for the CIF-FOB adjustments that affect the import and export vectors. These transformations are row-wise neutral for the total economy. Hence, the
target vector is completed with a vector of zeros for these three new rows. Unlike the SUT-Euro method, Equation 2 is more flexible when updating SUTs in practical applications since it does not require any additional assumption, e.g. Equation 1. This flexibility of SUT-RAS was present in variants developed in Valderas-Jaramillo et al. (Citation2019).
2.4. Common features of the SUT-Euro, GRAS-1 and other bi-proportional methods
Temursho (Citation2020) calls the Euro method ‘ad hoc’, because of its use of industry gross value added (GVA) growth rates to estimate imports, among other reasons. Linking import growth rates to industry GVA growth rates may well be a weak point of the Euro method. In our opinion, calling such linking ad hoc is unwarranted.
The Euro method was originally conceived by Beutel (Citation2002) to assess the economic impacts of European regional policies and by then, there was no information at all about regional imports by product so, understandably, the author assumed the same growth rates as those of gross value added (GVA). For better or worse, this feature was bypassed during revisions to the Euro method. It can be readily solved by assuming something different about the trajectory of imports; this would not imply the use of other external sources ‘more than “only official sources”’,Footnote10 nor would it change the essence of the Euro method itself. For instance, we could link import growth by product to the growth of the total product output.Footnote11
Temursho mentions several times that the Euro method shares many similarities with other bi-proportional methods such as GRAS. This is both a complement and an insult. The GRAS/SUT-RAS methods solve optimisation problems and find solutions as long as the problem is feasibly defined. For instance, a ‘bad’ prior matrix or at least gross structural difference between the actual and prior can yield major distortions that make attaining solutions infeasible.
Changes in the prior to remove infeasibilities is another issue that we should address. Such changes are common when applying Euro and GRAS methods, as is relaxing certain constraints (Lenzen et al., Citation2009; Tarancón & Del Río, Citation2005). Nonetheless, GRAS/SUT-RAS methods are usually quite stable (Valderas-Jaramillo et al., Citation2019, p. 437) and, thus, tend to converge more easily than do SUT-Euro methods under identical conditions (same prior and same targets). Our experience suggests that convergence in Euro methods tends not to be achieved when large structural differences arise between the prior and the target values. This occurs more often when the Supply matrix is far from being diagonal and when industry mix changes substantially between the prior and target year.
The problem of sign switches is not exclusive to the Euro method. It is common to all sign-preserving methods, including GRAS. For instance, in GRAS, a problem arises when all the elements in a vector are zero or positive (resp. zero or negative) and the total to match is negative (resp. positive). In such situations, GRAS breaks down and a solution is unfeasible, unless the analyst changes parts of the prior or some non-sign preserving alternatives are invoked (cf. Lenzen et al., Citation2014; Temurshoev et al., Citation2013). Lenzen et al.’s (Citation2014) solution is equivalent to flipping the sign of the elements in the prior so that they conform to the signs of constraints. This solution, not derived from an optimisation problem, can also be applied as needed under the Euro method.
Moreover, if any element in a row (or column) of the prior matrix is zero, it cannot be altered to achieve a non-zero target value. To enable such a change, the analyst must alter zero values in the prior to some positive value and run GRAS or for that matter any other bi-proportional algorithm. Similarly, GRAS and other bi-proportional methods have trouble changing positive and negative elements in a row (or column) to achieve a zero target row or column sum. This issue is particularly problematic when both the prior and target matrices are sparse.Footnote12 Instead, they adjust the corresponding positive and negative elements to achieve a zero sum. Unlike GRAS, RAS and the Euro method (with all elements positive or all elements negative) set all array elements to zero whenever the targeted total industry/product output is zero. This is desirable in some situations.
Another negative aspect raised by Temursho concerning the Euro method relates to Proposition 2.1Footnote13 in which he concludes that GVA multipliers, , are apparently different from genuine GVA multipliers,
, since
. But it is straightforward that due to convergence
, i.e.
, where
is the preliminary gross output vector (cfr. Temursho, Citation2020, Equation 1.6), and
the gross output estimate coming from the Leontief model with fixed product sales structure (cf. Temursho, Citation2020, Equation 1.8), both in iteration t. Since when convergence is achieved, then
, it follows that
. That is, GVA multipliers are equal to genuine GVA official macroeconomic forecasts multipliers,
, when the method converges.
To make a long story short, all non-survey methods are to some degree ‘ad hoc’ depending upon the circumstances. Needless to say, changing model assumptions is a typical way to improve models; they do not make the models arbitrary in any real sense if the essence of the model remains unchanged. Thus, we continue to disagree with Temursho (Citation2020) and opine that the SUT-Euro method is not ‘ad hoc’.
2.5. Eurostat references
Temursho (Citation2020) asserts that the Euro method does not have the same footing as other more or less data-demanding updating techniques. By extension of Temursho’s reasoning (p. 22), application of the SUT-Euro method affects Eurostat ability to produce high-quality statistics for Europe. We find this undermined by Temursho’s own calculations. Moreover, the Euro method was suitable when Eurostat started to estimate missing countries and time series of national Supply and Use tables. Since then, industry output data have not been collected for a number of countries and years.
Later, as mentioned by Temursho (Citation2020), SUT-RAS was further developed within the WIOD Project. Due to its success and the increasing amount of information received from EU Member States in succeeding years, Eurostat opted to explore SUT-RAS as a replacement for the SUT-Euro method. The results and conclusions of Valderas-Jaramillo et al. (Citation2019) were used by Eurostat in arriving at this decision.Footnote14,Footnote15 This, of course, requires that industry output again will become more widely available. Remond-Tiedrez and Rueda-Cantuche (Citation2019) discuss how Eurostat shifted to SUT-RAS in work related to the construction of the European inter-country Supply, Use and input-output tables – the so-called FIGARO Project.Footnote16 Thus, any concerns about Eurostat not providing high quality statistics should be assuaged.
2.5. Constant market shares
In our opinion, Temursho’s (Citation2020, p. 14) concern about the validity of the constant market-share assumption is somewhat inconsistent. Initially, he views this assumption as ‘already restrictive, as in reality industries’ commodity output proportions are not constant’ and favouring more flexibility, as exemplified in the variants of Harthoorn and van Dalen method (p. 9, fn. 9 &10). But elsewhere he advocates for the opposite, i.e.: constant market-shares are desirable and flexibility is a negative feature in the GRAS-0 method (p. 18–19).Footnote17,Footnote18 Ultimately, it is unclear why Temursho (Citation2020) opts to favour more flexibility in the GRAS-1/exo-SUT-RAS but not in the endo-SUT-RAS/GRAS-0.
With respect to the empirical validity of the constant market-share hypothesis we produced Table to get some insight into the stability of the market-shares across Spanish Supply tables from 2000 to 2007 and 2008 to 2015, using WAPEs as a measure. From these results, we conclude that a constant market shares in not unrealistic at all is the short-term for Spain, this despite Temursho’s (p. 20) contrary claims.
TABLE 1. Distance (WAPEs) between average market-share for Spain 2000–2015Table Footnotea.
2.6. Is really the evidence so much against the Euro method?
Temursho (Citation2020, Table 4) notes there is enough evidence that militates against the use of the SUT-Euro method when industry output is missing. But he uses the average of WAPE indicators covering an increasing time span to come to this conclusion. Interestingly, just a change in the focus of the empirical analysis leads to a very different conclusion. His evidence is insufficiently robust.
In Table , the WAPEs for the annual projections (short-term) for the same Spanish data show that in four out of seven of the annual projections reported, the two variants of the endo-SUT-Euro perform better than GRAS-1, so conclusions from Valderas-Jaramillo et al. (Citation2019) still hold. These results are quite in line with Temursho's (Citation2020, Table 4) results.Footnote19 As expected, endo-SUT-Euro performs better, on average, compared to GRAS-1 in all blocks except for imports, TLS and the imported Use table. As a whole, global estimated SUTs appear better using the endo-SUT-Euro method. That is, in the short term, the endo-SUT-Euro performs more poorly in TLS blocks, imports and sometimes in imported use and final demand blocks (as expected). But it performs as good, if not better, in domestic intermediate and domestic final uses. These results are not so overwhelmingly negative so that one might be able to claim that enough evidence exists to dispense with use of the endo-SUT-Euro method. Moreover, in five out of seven short-term projections, the endo-SUT-Euro appears to produce better estimates of industry output than one can achieve assuming a constant the GVA/output ratio.Footnote20 Interestingly, despite the good industry output estimates yielded via the GVA/output ratio, the Euro method performs better than the exo-SUT-RAS approach.
TABLE 2. Performance of GRAS-1 vis-á-vis the Euro variants.
Temursho’s (Citation2020, section 3) counter-example seems designed to justify avoiding the use of the SUT-Euro method in the absence of industry output. But it is easy to show cases where the SUT-Euro outperforms GRAS-1, as illustrated in Table , or with a numerical example using the Temursho’s simple approach, see section A2 of our online supplementary documentation for more details.
Models are simplifications of reality. The simplifications are implemented via the hypotheses and assumptions, each of which is critical. Naturally, the closer hypotheses and assumptions are to real world conditions, the better the model’s estimates will be. The essence of a model lies in capturing the mechanisms that rule the relationships among the variables involved. Temursho’s (Citation2020) ‘counter-example’ is a good instance. If we provide the SUT-RAS method with reliable industry output estimates, SUT-RAS performs better than the SUT-Euro method. But other ‘weak’ hypotheses and assumptions are also involved, such as the constant market-shares assumption. We show that even with a good exogenous estimate of industry output endo-SUT-Euro outperforms SUT-RAS/GRAS-1.
2.7. Euro applications and rectangular SUTs
Unlike Temursho (Citation2020), we believe that the SUT-Euro method has numerous viable applications when industry output cannot be meaningfully estimated. While adapting a version of the Euro method, Avelino (Citation2017) breaks down annual SUTs and IOTs into intra-year (e.g. quarterly) tables with specific technical structures using commonly available quarterly GDP data. Another important field of applications is regionalisation or estimation of SUT frameworks for small areasFootnote21 where only basic information such as the one the endo-SUT-Euro method requires may be available (see e.g. Mainar-Causapé et al., Citation2017).
Finally, a key drawback of the canonical variants of the Euro method developed by Beutel (Citation2002, Citation2008) and Valderas-Jaramillo et al. (Citation2019) is that they cannot work upon non-square SUTs. This is a general weakness of any model that implements a Leontief inverse. But one can modify SUT-Euro to build a variant that can work on rectangular SUTs. This is possible via a linear programme and leaving Leontief’s quantity model behind (Duchin & Levine, Citation2011). This variant enables the Euro method to handle large rectangular SUTs and mitigates this traditional criticism attributed to the Euro method (Temursho, Citation2020, pp. 13–17).
3. Conclusions
In this paper, we attempt to clarify the picture of our prior work as presented by Temursho (Citation2020). We conclude by summarising via the following remarks:
We contend that the basic conclusions of Valderas-Jaramillo et al. (Citation2019) still hold. In our view, the GRAS-1 method is equivalent to the exo-SUT-RAS method since both actually use exogenous industry outputs. In the example provided by Temursho (Citation2020), the only difference is that he replaces official industry outputs with simply calculated equivalents. When these simple estimates are accurate (as in the real example shown by Temursho), his findings support those of Valderas-Jaramillo et al. (Citation2019). So, it is not critical who provides industry output estimates or how they are produced.
Good estimates of industry output alone are an insufficient reason to cast dispersions upon the SUT-Euro method. Using a numerical counterfactual with ‘good’ estimates of industry output using Temursho’s (Citation2020) approach, we show that the SUT-Euro method outperforms the exo-SUT-RAS/GRAS-1 method due to some of its other assumptions (e.g. constant market shares in short-term projections). This is at least the case, where and when a constant market-share hypothesis is realistic (in the short-term) and when a reliable estimate of industry output cannot be meaningfully estimated. Thus, we continue to believe that the SUT-Euro method has numerous viable applications. We concur that replacing the Leontief quantity model in a linear programme (Duchin & Levine, Citation2011) could enable the Euro method to handle Supply and Use tables with different number of products and industries (rectangular SUTs).
SUT-Euro and GRAS methods share the same need to deal with necessary transformations when addressing the problem of infeasibilities and/or sign-flips situations. This is also the case when transforming ordinary macroeconomic forecasts from national to domestic terms.Footnote22 Improving assumptions in models and methods via such transformations is not an ad hoc venture.
Eurostat continues to produce only the highest quality statistics in the form of EU national SUTs, including consolidated EU SUTs/IOTs. The shift from SUT-Euro to the SUT-RAS method moved swiftly over recent years. Nowadays, SUT-RAS is the norm rather than the exception in the Eurostat work that updates SUTs. This change was partly made by compelling evidence displayed in Valderas-Jaramillo et al. (Citation2019).
Supplemental Material
Download PDF (1.3 MB)Acknowledgements
We thank three anonymous referees for their valuable suggestions to improve this paper. Special thanks go to Mike Lahr for his dedicated efforts to streamline the paper.
Disclosure statement
The views expressed in this paper are those of the authors and do not necessarily reflect an official position of the European Commission.
Notes
aIn the year 2008, the change of the NACE classification breaks the comparability of the time series.
Source: own elaboration.
Note: Relative WAPE of method EURO-X to methos GRAS-1 is (WAPEEURO-X/WAPEGRAS-1 – 1).
1 Similarly, his Proposition 2.3 loses its meaning since by definition commodity outputs are not given exogenously.
2 We have verified the high correlations over time for all countries available in the Eurostat database. See supplementary documentation, section A1. Our findings suggest that Spain is a country in which the value added/output ratio is most stable over time. Except for Slovakia, Latvia and Denmark, all EU countries have correlation coefficients larger than 0.82, with Spain, Germany, UK and Portugal having the largest (0.97–0.98), given a one-year lag. As one might expect, these coefficients decrease as the lag lengthens, although less so for countries such as Sweden, Germany, France and Portugal. As a result, the Spanish data and sample period used by Temursho (Citation2020) as an example might not be so representative. Despite these high correlations, we also find that the value added/output ratio might not be a good proxy for industry output. For example, Austria in 2015, Romania in 2010 and 2012, Denmark in 2010 and Latvia in 2011, among others, deviate by more than 20% using weighted average percentage error (WAPE) as a measure.
3 Final demand values in national concept must be adjusted for CIF-FOB adjustments, direct purchases abroad made by residents (DPA) and purchases on the domestic territory by non-residents (NRPT). The total imports of the economy in national concept includes the CIF-FOB adjustments and DPA.
4 For European standards, see Eurostat (Citation2013, ESA-2010, par. 3.173) for a definition of these concepts.
5 Final consumption expenditure by households does not include DPA and includes NRPT. Imports by product do not include DPA and CIF-FOB adjustments. Moreover, exports (fob) by products do not include NRPT.
6 See Eurostat (Citation2013) ESA-2010 Transmission Programme, footnote 3 on p. 101 and footnote 5 on p. 103.
7 In the online supplementary document (section A3) we assess both updated SUTs (domestic and national concepts) using GRAS-1.
8 According to the ESA-2010 Transmission Programme (p. 64), it is possible that countries provide total of household consumption in both domestic and national terms. For the time being, however, this is not the case of Spain. Exports and imports total are provided only in national terms according to ESA-2010 Transmission Programme.
9 In the projection exercises in Valderas-Jaramillo et al. (Citation2019) and Temursho (Citation2020), it is possible to calculate targets under the domestic concept since all the elements of the target tables are fully known. This is done in Valderas-Jaramillo et al., although not explicitly stated. Still, it is not entirely clear what concept was applied in Temursho’s expressions (13) and (14). In any case, this situation is not realistic since direct purchases abroad, non-residents’ purchases in domestic territory and CIF-FOB adjustments are not regularly provided in official macroeconomic forecasts, so not all the elements of in target tables are fully known.
10 Temursho (Citation2020, p. 6).
11 We do not suggest that this is the best option (according to empirical assessments). Rather we use it to illustrate that other possibilities exist given ‘limited data requirements’ of the SUT-Euro method and without employing ‘new’ external sources.
12 This issue has an arguable economic meaning except in very specific situations such as the allocation of trade and transports margins to trade and transport sectors whenever a conversion from purchaser’s prices to basic prices is required. This also holds, as shown in the first row of Equation 2, when the difference between the total use of intermediate and final products and their total supply must be zero.
13 Temursho (Citation2020, p. 8).
14 See Temursho (Citation2020).
15 It is important to remark that the calculations and conclusions reported in Valderas-Jaramillo et al. (Citation2019) were known by Eurostat as early as 2015 since the calculations therein were carried out and drawn during autumn and winter 2014 and spring 2015 as part of the first author’s Ph. D. Thesis, defended in January 2016.
17 ‘ … the particular high relative WAPEs of the Supply matrix estimates implies that the assumption of constant market shares matrix … is largely inconsistent with data and is best avoided in the practice of SUT updating’
18 ‘ … the Euro method uses the base-year market share matrix that imposes certain structural constraints on the domestic supply update. The general poor performance of the GRAS-0 method … ’ is due to this assumption.
19 Temursho (Citation2020, p. 21), p. 21. In particular, GRAS-1 performance (Euro-G to GRAS-1), for the period 2000–2001.
20 It may be a good idea (not empirically tested) to perform the endo-SUT-Euro to estimate industry outputs and then use them as exogenous information for exo-SUT-RAS.
21 As two anonymous referees have pointed to us, and we agree, the gains of non-survey methods are likely to be larger if used for the regionalisation of national SUTs rather than for time projections of national SUTs. After all, impact studies can still be done using a bit outdated tables as evidence strongly suggests that underlying structures are rather stable in the short term.
22 However, the flexibility of the SUT-RAS framework enables an alternative to deal with this issue without the need of estimating target totals in domestic concept.
References
- Avelino, A. F. T. (2017). Disaggregating Input–Output tables in time: The temporal Input–Output framework. Economic Systems Research, 29(3), 313–334. https://doi.org/10.1080/09535314.2017.1290587
- Beutel, J. (2002). The economic impact of objective 1 interventions for the period 2000–2006. Report to the Directorate-General for Regional Policies.
- Beutel, J. (2008). An Input–Output system of economic accounts for the EU Member States. Interim Report for Service Contract Number 150830-2007 FISC-D to European Commission, Directorate-General Joint Research Centre, Institute for Prospective Technological Studies.
- Duchin, F., & Levine, S. H. (2011). Sectors may use multiple technologies simultaneously: The rectangular choice-of-technology model with binding factor constraints. Economic Systems Research, 23(3), 281–302. https://doi.org/10.1080/09535314.2011.571238
- Eurostat. (2008). Manual of Supply, Use and Input–Output tables. Official Publications of the European Communities. KS-RA-07-013.
- Eurostat. (2013). European System of National Account ESA 2010. Publications Office of the European Union, KS-02-13-269.
- Lenzen, M., Gallego, B., & Wood, R. (2009). Matrix balancing under conflicting information. Economic Systems Research, 21(1), 23–44. https://doi.org/10.1080/09535310802688661
- Lenzen, M., Moran, D. D., Geschke, A., & Kanemoto, K. (2014). A non-sign-preserving RAS variant. Economic Systems Research, 26(2), 197–208. https://doi.org/10.1080/09535314.2014.897933
- Mainar-Causapé, A., Rueda-Cantuche, J. M., Cardenete, M. A., Fuentes-Saguar, P., Delgado, M. C., Santini, F., & Gómez y Paloma, S. (2017). Estimating regional social accounting matrices to analyse rural development. SORT, 41(2), 319–346. https://www.idescat.cat/serveis/biblioteca/docs/bib/publicacions/r00262017v412.pdf
- Remond-Tiedrez, I., & Rueda-Cantuche, J. M. (Eds.). (2019). EU inter-country Supply, Use and Input–Output tables — Full international and global accounts for research in input-output analysis (FIGARO). European Union, Publications Office of the European Union in Luxembourg. https://doi.org/10.2785/008780
- Tarancón, M. A., & Del Río, P. (2005). Projection of input–output tables by means of mathematical programming based on the hypothesis of stable structural evolution. Economic Systems Research, 17(1), 1–23. https://doi.org/10.1080/09535310500034119
- Temursho, U. (2020). On the Euro method. Economic Systems Research, 1–26. https://doi.org/10.1080/09535314.2020.1772727
- Temurshoev, U., Miller, R. E., & Bouwmeester, M. C. (2013). A note on the GRAS method. Economic Systems Research, 25(3), 361–367. https://doi.org/10.1080/09535314.2012.746645
- Temurshoev, U., & Timmer, M. P. (2011). Joint estimation of supply and use tables. Papers in Regional Science, 90(4), 863–882. https://doi.org/10.1111/j.1435-5957.2010.00345.x
- Valderas-Jaramillo, J. M., Rueda-Cantuche, J. M., Olmedo, E., & Beutel, J. (2019). Projecting supply and use tables: New variants and fair comparisons. Economic Systems Research, 31(3), 423–444. https://doi.org/10.1080/09535314.2018.1545221