Abstract
Previous studies have reported inconsistent associations between platelet count (PLT) and cancer survival. However, whether there is linear causal effect merits in-depth investigations. We conducted a cohort study using the UK Biobank and a two-sample Mendelian randomization (MR) analysis. PLT levels were measured prior to cancer diagnosis. We adopted overall survival (OS) as the primary outcome. Cox models were utilized to estimate the effects of PLTs on survival outcomes at multiple lag times for cancer diagnosis. We employed 34 genetic variants as PLT proxies for MR analysis. Linear and non-linear effects were modeled. Prognostic effects of gene expression harboring the instrumental variants were also investigated. A total of 65 471 cancer patients were included. We identified a significant association between elevated PLTs (per 100 × 109/L) and inferior OS (HR: 1.07; 95% CI: 1.04–1.10; p < .001). Similar significant associations were observed for several cancer types. We further observed a U-shaped relationship between PLTs and cancer survival (p < .001). Our MR analysis found null evidence to support a causal association between PLTs and overall cancer survival (HR: 1.000; 95% CI: 0.998–1.001; p = .678), although non-linear MR analysis unveiled a potential greater detrimental effect at lower PLT range. Expression of eleven PLT-related genes were associated with cancer survival. Early detection of escalated PLTs indicated possible occult cancer development and inferior subsequent survival outcomes. The observed associations could potentially be non-linear. However, PLT is less likely to be a promising therapeutic target.
Plain Language Summary
What is the context?
Previous studies have reported inconsistent associations between platelet counts (PLTs) and cancer survival. However, it is unclear whether there is a linear causal effect, as most studies measured PLTs at the time of cancer diagnosis, which could be influenced by the cancer itself.
This study aimed to investigate the association and potential causality between pre-diagnostic PLTs and cancer survival outcomes using a large prospective cohort and genetic analysis.
What is new?
The observational cohort study found a significant association between elevated pre-diagnostic PLTs and poorer overall and cancer-specific survival. We also identified a U-shaped relationship between PLTs and cancer survival, suggesting that both high and low PLTs may be detrimental.
The Mendelian randomization analysis did not support a causal effect of PLTs on overall cancer survival, although it hinted at potential non-linear effects at lower PLT ranges.
The study also identified several genes (TPM4, PDIA5, PSMD13, TMCC2, ZFPM2, BAZ2A, CDKN2A, GP1BA, TAOK1, CABLES1, and THPO) related to PLTs that were associated with cancer survival.
What is the impact?
The findings suggest that early detection of elevated PLTs may indicate occult cancer development and poorer subsequent survival outcomes. However, PLTs are less likely to be a promising therapeutic target for improving cancer survival, as the observed associations could be influenced by confounding factors.
The study highlights the need for further research into the complex relationship between PLTs and cancer prognosis, as well as the exploration of other platelet-related traits as potential drug targets.
Introduction
Cancer is one of the leading causes of mortality across 127 countries, resulting in almost 10 million global deaths in 2020.Citation1,Citation2 This highlighted the need to search for promising prognostic biomarker and therapeutic targets to improve outcomes of cancer survivors. Especially, recent research suggests that platelet transcriptomes may serve as biomarkers for cancer diagnoses, offering a noninvasive method for early detection and monitoring of malignancies.Citation3,Citation4
Population-level evidence has reported associations between pretreatment thrombocytosis, namely elevated platelet counts (PLTs), and poor prognosis among cancer patients, including colorectal,Citation5,Citation6 lung,Citation7 and ovarian cancers.Citation8 However, these studies were mostly limited by their retrospective nature and small sample sizes, which might incur biases. Moreover, whether this observed association is linear remains unclear. In addition, previous observational investigations explored the PLT levels recorded at diagnosis,Citation5–11 and thus the observed alterations in PLTs could have occurred after de facto cancer development, given the possible delayed diagnoses. In that case, the PLT level would only serve as biomarker for cancer progression rather than an etiological cause and druggable target.
Previous mechanistic evidence revealed a potential role of platelets in cancer progression and prognosis by interacting with cancer cellsCitation12 and subsequently fostering tumor proliferation, spread, and metastasis.Citation13–15 However, the alteration of inflammatory cytokine environment, exemplified in conditions like carcinogenesis and cancer metastasis,Citation16 can also significantly affect the homeostasis of platelet production. Therefore, the direction of possible causality between PLT and cancer progression, if there is, in the association between PLT and cancer prognosis still remain unknown.
The nature of observational study predisposes it to confounders and thus challenging to prove causality. Mendelian randomization (MR) is an approach employed to infer the causal relationship by utilizing genetic variants as instrumental variables,Citation17 which hinges on the naturally randomized distribution of these variants during gamete formation.Citation18 Herein, we performed a prospective cohort study and two-sample MR analyses to comprehensively evaluate the association and causality between pre-diagnostic PLTs and cancer survival.
Materials and methods
Prospective cohort study
The UK BiobankCitation19 is a prospectively designed population-based cohort, established between 2006 and 2010, recruited over 500,000 participants ranging in age from 40 to 69 years. The study was approved by the North West-Haydock Research Ethics Committee (21/NW/0157). We retrieved PLT levels at enrollment, cancer diagnoses, death records, genetic variant information, and other covariates (listed in Supplementary Table S1) from the UK Biobank under the Application ID 73 759. The cohort study was reported adhering to the STROBE guidelines.Citation20
Cohort study design
As shown in . Cancer diagnoses after enrollment were documented using the International Classification of Diseases (ICD) codes from both the 9th and 10th editions (ICD codes are detailed in Supplementary Table S2). Individuals were prospectively followed up until 1 July 2021, and their survival status was ascertained by linking to the death registries. The primary outcome was overall survival (OS), and the secondary outcome was cancer-specific survival (CSS). To ensure sufficient statistical power, we limited our study to cancer types with over 1,000 cases.
Statistical analyses
We utilized the Cox proportional hazards model and estimated hazard ratios (HRs) with 95% confidence intervals (CIs) to assess the association between PLTs and cancer survival. Different sets of covariates were adjusted including age, sex, ethnic background, smoking status, alcohol status, body mass index (BMI), and the Townsend deprivation index (TDI). PLTs were modeled in both continuous scale to investigate possible nonlinearity using restricted cubic splines (three knots) and binary scale using cutoff values of 300 × 109/L and 400 × 109/L, respectively. The non-linear associations were tested using a likelihood ratio test by comparing to linear counterparts. To account for the potential influence of the lag time, defined as the time from PLT measurement to cancer diagnosis (), on cancer survival, sensitivity analyses were conducted using multiple lag time intervals, including zero to six months, six months to one year, one to three years, and three or more years. A two-sided p < .05 was adopted as threshold of statistical significance for all cancer analysis, and Bonferroni correction was applied for site-specific analysis (p < .0025 as significant). Analysis was performed using the “survival,”Citation21 “Hmisc”,Citation22 “lmtest”,Citation23 and “smoothHR”Citation24 packages in R (version 4.4.0).Citation25
Mendelian randomization
The design of the two-sample MR study is presented in . To ensure the inclusion of European samples independent of the UK Biobank and to avoid potential overlap and bias, genetic variants along with their effects on PLT levels were obtained from a large meta-analysis of genome-wide association study (GWAS) by Gieger et al., which encompassed 23 studies, excluding the UK Biobank, involving 48 666 individuals of European descent.Citation26 We then estimated the effects of selected variants on survival outcomes using Cox models. The MR study was reported adhering to the STROBE guidelines.Citation27
Genetic instrument selection
Genetic variants were selected based on the three basic assumptions for instrumental variables, namely the relevance, independence, and exclusion restriction assumptionCitation28: Variants robustly associated with PLT levels (p < 5 × 10−8) were retrieved from the GWAS meta-analysis, and those in linkage disequilibrium (LD) were pruned using a 10 million base window and an r2 threshold of 0.001, given that using multiple correlated variants would increase risk of bias in the estimates.Citation29,Citation30 We excluded variants either associated with cancer survival outcomes or linked with other potential confounders (metabolic diseases, inflammatory diseases, infectious diseases, blood pressure, blood lipids, BMI, and smoking) using the PhenoScanner databaseCitation31,Citation32 (www.phenoscanner.medschl.cam.ac.uk.) (p < 5 × 10−8). Variants not presented in the UK Biobank were substituted by proxies in strong LD (r2 >0.8).
Statistical analyses
We created both weighted and unweighted polygenic risk scores (PRS) for all individuals in the UK Biobank, and a univariate linear regression model was employed to assess the associations between these scores and the PLT level, while obtaining the corresponding F-statistic to evaluate the instrument strength (F > 10 showed no weak instrument bias). The effects along with standard errors of variants on PLTs were obtained from the GWAS meta-analysis, and their effects on survival outcomes were estimated from multivariable Cox models. We utilized multiple MR approaches including inverse variance weighted (IVW)Citation33 estimate as the primary estimator, MR-Egger,Citation34 and weighted medianCitation35 to investigate the causal impact of PLTs on cancer survival.
We employed the heterogeneity statistic (Cochran’s Q) to represent potential heterogeneity. The intercept from the MR-Egger was used to evaluate horizontal pleiotropy. Additionally, we utilized the Mendelian Randomization Pleiotropy Residual Sum and Outlier (MR-PRESSO) method to detect and adjust horizontal pleiotropy.Citation36 To determine if our MR estimates were unduly influenced by a particular variant, we conducted leave-one-out analyses. MR analyses were implemented using the “TwoSampleMR” package.Citation37 We also performed non-linear MR analysis using fractional polynomial method.Citation38 Throughout our MR analyses, a two-sided p < .05 indicated statistical significance.
Gene expression analysis
To explore possible biological implications, we retrieved corresponding genes where our instrumental genetic loci are located. Gene expression profiling in primary cancer tissues and clinical follow-up dataCitation39 for various cancers were obtained from The Cancer Genome Atlas (TCGA) database (https://www.cancer.gov/tcga). Individuals were divided into two groups based on the median expression level of each gene with a ten-year follow-up time. Effects of each gene on OS and CSS were estimated using Cox models, adjusting for age and sex. False discovery rate (FDR) was adopted to correct multiple testings. For any genes associated with survival, we identified cis-expression quantitative trait loci (eQTLs; p < 5 × 10−8) from whole blood in the Adult Genotype-Tissue Expression database (GTEx v8; https://www.gtexportal.org), and conducted MR analyses using the Wald ratio approach. It is important to note that participants of these databases are independent from the UK Biobank samples.
Results
Prospective cohort study
Of the 502,461 individuals enlisted in the UK biobank 94 533 (representing 18.8% of the total) were diagnosed with cancer. Among them 65 471 cancer patients were eligible for the cohort study (Diagram for patient selection in Supplementary Figure S1). The median time span from enrollment to cancer diagnosis was 6.66 years (), and during a median of 4.14 years follow-up 15 856 deaths were documented. provides a summary of characteristics for eligible cancer patients.
Figure 2. (A) distribution of lag time. (B) Examination of the non-linear association between platelet counts and overall cancer survival. (C) Mendelian randomization analyses for platelet counts on overall cancer survival. (D) Survival analyses of TPM4 gene expression (primary tumor tissue) in overall cancer survival. Gene expression data were obtained from The Cancer Genome Atlas (TCGA) database.
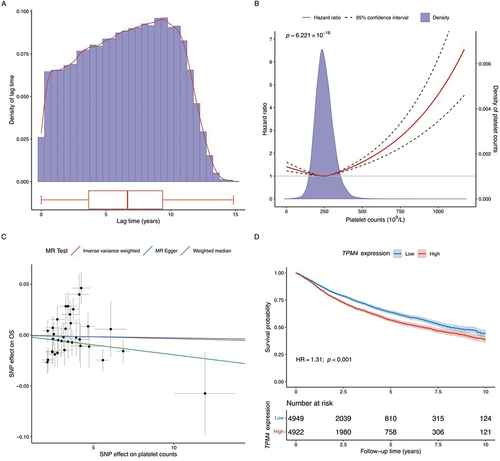
Table I. Characteristics of eligible cancer patients in the UK biobank.
In the fully adjusted linear model (covariates listed in Supplementary methods), we found a significant association between elevated pre-diagnostic PLTs (every 100 × 109/L increase) and inferior OS (HR: 1.07; 95% CI: 1.04–1.10; p < .001). A similar association was observed for CSS (HR: 1.06; 95% CI: 1.03–1.09; p < .001). These associations remained consistent in the age- and sex-adjusted baseline Cox model (Supplementary Figure S4). We then dichotomized PLT levels based on cutoff values in common use (≥300 × 109/L and ≥ 400 × 109/L), and observed 12% (≥300 × 109/L) and 35% (≥400 × 109/L) higher hazards for cancer patients with escalated PLTs compared with the reference group (OS: ≥300 × 109/L vs. <300 × 109/L: HR: 1.12; 95% CI: 1.08–1.17; p < .001; ≥400 × 109/L vs. <400 × 109/L: HR: 1.35; 95% CI: 1.21–1.51; p < .001; Supplementary Figure S2-S3).
Similar associations were identified between escalated PLTs and poor survival for patients with solid tumors and secondary cancer. As for site-specific analysis, we found substantial amount of heterogeneities across various cancer types, and no significant effects were detected for most cancer types, except marginal evidence for skin cancer (Forest plots in and Supplementary Figure S2-S6). Sensitivity analyses yielded an overall decreased trend in cancer survival with increased lag time intervals, though this trend could be non-significant and varied across different cancer types (Supplementary Figure S7-S18).
Figure 3. Association between platelet counts (increments of 100 × 109/L) and cancer survival in the UK biobank cohort. Hazard ratios were derived from cox proportional hazards regression, with adjustments made for factors including age, sex, ethnic background, body mass index, Townsend deprivation index, smoking status, and alcohol status.
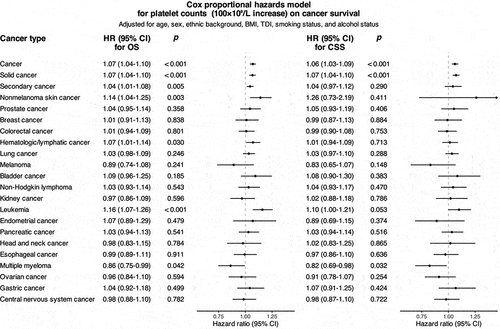
We identified a U-shaped non-linear relationship between PLT and cancer survival (p < .001). Compared to the median level (~250 × 109/L), patients with higher or lower PLTs were both linked with poor survival outcomes (). Similar trends were found among subtypes of solid cancer, hematologic/lymphatic cancer, secondary cancer, skin cancer, melanoma, and leukemia (p < .05), although an inverse U-shaped association with multiple myeloma was found (p < .05) (Supplementary Figure S19-S20).
Mendelian randomization
A total of 34 variants were selected as genetic instruments (Diagram presented in Supplementary Figure S21). Both the weighted and unweighted PRS showed a strong association with PLT levels in the linear regression model (p < .001, details in Supplementary Figures S22-S23), with an F-statistic of 18 060.810 for the weighted and 14 796.503 for unweighted PRS. No variant showed potential impact on cancer survival outcomes (Supplementary Table S3-S4).
Using the IVW approach, we found limited evidence supporting a causal relationship between higher PLTs and survival outcomes in all cancer and most cancer types, with an exception of central nervous system cancer, where a nominal significant causal effect was found (IVW estimate for OS: 1.006; 95% CI: 1.000–1.012; p = .037). MR-Egger and median-based estimates yielded similar non-significant results for all cancer and multiple cancer types (details in , , and Supplementary Figure S24). The intercepts of MR-Egger estimates were not significant (Supplementary Table S5), suggesting no evidence of horizontal pleiotropy for any cancer types. We observed certain amount of heterogeneity across the effects of the 34 genetic instruments (Cochran’s Q-tests in Supplementary Table S6). MR-PRESSO analysis identified no significant outliers or distortions in the causal estimates (Supplementary Table S7). Leave-one-out sensitivity analyses showed no single variant had major influence on the overall causal effect (shown in Supplementary Figure S25). Non-linear MR analysis suggested a potential L-shape effect where larger detrimental effects were observed for lower PLT levels (<400 × 109/L), although statistical significance for the non-linearity was not obtained (Supplementary Figure S26).
Table II. The causal estimates of platelet counts on different types of cancer survival.
Gene expression analysis
As shown in Supplementary Table S8, 26 unique genes harboring the included instrumental variants were mapped. Among the 9,871 cancer patients from TCGA, expression of eleven genes (TPM4, PDIA5, PSMD13, TMCC2, ZFPM2, BAZ2A, CDKN2A, GP1BA, TAOK1, CABLES1, and THPO) were significantly associated with cancer survival ( and Supplementary Figure S27, pFDR <0.05). An instrumental variant rs8109288 was identified as an eQTL for the TPM4 gene. However, the MR analyses did not find significant causal effect of the TPM4 gene expression on overall cancer survival (HR: 0.891; 95%CI: 0.760–1.046; p = .158) (Supplementary Table S9).
Discussion
Principal findings and interpretation
This study identified associations between early escalation of PLTs and poor cancer survival outcomes, and revealed possible non-linearity underlying the observed associations. Compared to previous reports, our large prospective cohort observed smaller effect estimates of associations and MR analysis supported null causal effects of PLTs on cancer prognosis, although possible non-linear effects could not be excluded.
Abundant previous studies have investigated the association between elevated PLTs, or thrombocytosis, and survival outcomes of a wide spectrum of cancers, including colorectal,Citation5,Citation6 lung,Citation7 and ovarian malignancies.Citation8 A recent cohort study also found that PLTs were associated with survival of patients with all cancer types combined.Citation11 Note that these prior studies typically measured PLTs at cancer diagnosis, and the absence of temporality impeded subsequent causal investigation. In contrast, our cohort measured the PLT level prior to cancer diagnosis, and confirmed associations even adjusting for a lag time interval of three or more years. Also, our findings revealed an overall decreasing effect sizes in cancer survival with increased lag time intervals, which implied that early detected thrombocytosis indicated occult malignancies, resonating with previous evidence that elevated PLTs were linked with increased cancer riskCitation9,Citation10,Citation40 and the fact that prolonged diagnostic intervals were associated with worse cancer survival outcomes.Citation41 A recent study also suggested that platelet RNA merited further exploration for early cancer detection across various tumor types. RNA signatures in platelets have shown potential as a pan-cancer biomarker for cancer screening and prognostication.Citation3
Our analysis on various cancer sites identified mostly non-significant associations between survival of specific cancer types and PLT level. Although we only included cancer types over 1,000 incident cases, yielding greater sample sizes than most previous studies,Citation5–8 smaller effect sizes were observed by our study. This might be attributed to the early PLT measurement for our study, or potential over estimation on the effects for previous reports that originated from their retrospective nature.Citation10 In-depth evaluation revealed a U-shaped trend underlying the observed associations for all cancer and certain sites. This suggested detrimental effects of decreased PLTs, a signal for essential physiological dysfunctions that impaired patients’ outcomes.Citation42 Although the pooled analysis of all cancers reflected general incidence of different cancer types in the UK Biobank cohort, it should be noted that the U-shaped association observed in all cancers may be confounded due to the unbalanced distribution of incident cancer types within the cohort, and therefore should be interpreted with caution. Our findings hinted at the limitations of dichotomy (≥300 or 400 × 109/L) in current clinical use for thrombocytosis that might have caused important information loss in predicting cancer survival. We also reported an interesting inverse U-shaped non-linear association for multiple myeloma, which underscored the unique biology in the disease and warranted future investigations.
While the association between PLT and cancer survival outcomes is significant, it is essential to account for various competing risk factors such as death from coronary heart disease (CHD) as well as other cardiovascular disease (CVD). In our analysis, the HRs differ between OS and CSS, indicating the presence of non-cancer mortality. Numerous studies have reported on the relationship between PLT and non-cancer mortality.Citation43 For instance, individuals in the general population with either higher or lower PLT have increased CHD mortality.Citation44,Citation45 High PLT is associated with increased CVD mortality, and both high and low PLT are linked to higher mortality from other causes.Citation44,Citation46 Future studies should incorporate competing risks models to provide a more nuanced understanding of these relationships.
There has been an abundance of mechanistic evidence illuminating the key role of platelets in cancer development. In particular, platelets represent the largest repository of vascular endothelial growth factor that promotes angiogenesis in cancer progression.Citation47 Moreover, platelets could shield circulating tumor cells from immunological detection,Citation14,Citation15,Citation48 facilitating tumor cell extravasation and migration.Citation14 Our MR analyses, however, found a null causal effect of PLTs on cancer survival, pointing to possible influence of unobserved confounders that might have driven the observed associations. However, our study could not rule out possible small to modest causal effects given the limited sample size. It is worth mentioning that our non-linear MR analysis also indicated possible detrimental effects of lower PLT levels. Due to the limited statistical power after multiple testings, we did not conduct non-linear MR analysis for each specific cancer type, which should be investigated by future research.
Note that PLT might not be the optimal trait that measures platelet bioactivities. For instance, aspirin exerts antiplatelet effects by inhibiting cyclooxygenase (COX) and preventing platelet aggregation, but shows minimum influence on PLT level.Citation49 A pooled study of multiple randomized trials found that aspirin could reduce cancer metastasis and improve patient outcomes.Citation50 However, the ASPREE randomized controlled trial indicated that apparently healthy older adults who received daily aspirin had higher all-cause mortality compared to those who received a placebo, primarily due to an increase in cancer-related deaths.Citation51 Other regulators of platelet production, such as thrombopoietin,Citation52 have also been linked to cancer survival outcomes.Citation53 Our study identified TPM4 gene associated with platelet function as a potential prognostic marker, but whether it was promising drug target needs further investigation. Tropomyosin 4 (TPM4) is a member of the tropomyosin family of actin-binding proteins, which are involved in the cytoskeleton of non-muscle cells.Citation54 Abnormal TPM4 expression has been observed in many cancers, including ovarian, breast cancersCitation55,Citation56 and non-small cell lung carcinoma.Citation57 Therefore, future efforts are still needed to explore novel platelet-related traits with therapeutic potentials.
Clinical implication
Our findings indicated that early escalated PLTs may suggest occult cancer development, and these individuals need proper surveillance to improve early diagnosis. PLT is also predictive for cancer prognosis and should be actively monitored during follow-up. However, the optimal cutoff value for clinical use merits further investigation, especially for different cancer types. Current evidence does not support antiplatelet therapies targeting PLTs to improved survival outcomes for cancer patients with thrombocytosis.
Strengths and limitations
Our cohort study presented a comprehensive analysis exploring the role of early detection of aberrant PLTs in cancer prognostification and the potential as future drug target. However, certain inherent limitations warrant notation. Firstly, our PLTs were gauged at enrollment, and could not reflect the dynamic effects of exposure levels throughout the follow-up. Secondly, our study exclusively utilized data from European populations, and hence, our findings might not apply to other ethnic or geographical groups. Last but not the least, the relatively small sample size for certain cancer types limited the statistical power, and the site-specific effects of PLT on cancer survival warranted future investigation.
Conclusion
Early detection of escalated PLTs is indicative for occult carcinogenesis and long-term survival of cancer patients, although the observed effects were smaller in size than previous reports and even non-significant for certain cancer subtypes. However, pre-diagnostic PLT may not serve as a therapeutic target due to hidden confounders, although possible non-linear effects could not be excluded. Impact of other markers for platelet bioactivity on cancer prognosis should be explored in the future.
Author contributions
CL, JC, XC, YZ, and YH are the guarantors of integrity of the entire study. CL, JC, YZ, and YZ were responsible for study concepts and design. CL carried out the literature research. CL, JC, DH, CS, JH, LW, HL, and QW were responsible for the statistical analysis. CL prepared the manuscript. CL, JC, DH, CS, JH, LW, HL, QW, XC, YH, and YZ edited the manuscript. All authors drafted or revised the manuscript and approved the final version.
Ethics approval statement
The UK Biobank was approved by the North West-Haydock Research Ethics Committee (21/NW/0157).
Supplementary_Tables.xlsx
Download MS Excel (75.1 KB)Supplementary_Methods.pdf
Download PDF (116.2 KB)Supplementary_Figures.pdf
Download PDF (2.4 MB)Acknowledgments
We acknowledge the UK Biobank, The Cancer Genome Atlas (TCGA), and the Genotype-Tissue Expression (GTEx) project for their efforts on human health and the sharing of scientific data.
Disclosure statement
No potential conflict of interest was reported by the author(s).
Data availability statement
Individual data from the UK Biobank could be accessed via application. Access to public datasets is described in article. All R source codes for analysis are available at https://github.com/lict99/PLT_Cancer_Survival.
Supplementary material
Supplemental data for this article can be accessed online at https://doi.org/10.1080/09537104.2024.2379815
Additional information
Funding
References
- Bray F, Laversanne M, Weiderpass E, Soerjomataram I. The ever-increasing importance of cancer as a leading cause of premature death worldwide. Cancer. 2021;127(16):3029–12. doi:10.1002/cncr.33587.
- Sung H, Ferlay J, Siegel RL, Laversanne M, Soerjomataram I, Jemal A, Bray F. Global cancer statistics 2020: GLOBOCAN estimates of incidence and mortality worldwide for 36 cancers in 185 countries. CA A Cancer J Clin. 2021 May;71(3):209–49. doi:10.3322/caac.21660.
- Wurdinger T, In ‘t Veld SGJG, Best MG. Platelet RNA as pan-tumor biomarker for cancer detection. Cancer Res. 2020 Apr 2;80(7):1371–3. doi:10.1158/0008-5472.CAN-19-3684.
- Nilsson RJA, Balaj L, Hulleman E, Van Rijn S, Pegtel DM, Walraven M, Widmark A, Gerritsen WR, Verheul HM, Vandertop WP. et al. Blood platelets contain tumor-derived RNA biomarkers. Blood. 2011 Sep 29;118(13):3680–3. doi:10.1182/blood-2011-03-344408.
- Ishizuka M, Nagata H, Takagi K, Iwasaki Y, Kubota K. Preoperative thrombocytosis is associated with survival after surgery for colorectal cancer. J Surg Oncol. 2012 Dec;106(7):887–91. doi:10.1002/jso.23163.
- Lee YS, Suh KW, Oh SY. Preoperative thrombocytosis predicts prognosis in stage II colorectal cancer patients. Ann Surg Treat Res. 2016 June;90(6):322–7. doi:10.4174/astr.2016.90.6.322.
- Kim M, Chang H, Yang HC, Kim YJ, Lee CT, Lee JH, Jheon S, Kim K, Chung J-H, Lee JS, et al. Preoperative thrombocytosis is a significant unfavorable prognostic factor for patients with resectable non-small cell lung cancer. World J Surg Oncol. 2014 Feb 12;12(1):37. doi:10.1186/1477-7819-12-37.
- Stone RL, Nick AM, McNeish IA, Balkwill F, Han HD, Bottsford-Miller J, Rupaimoole R, Armaiz-Pena GN, Pecot CV, Coward J, et al. Paraneoplastic thrombocytosis in ovarian cancer. N Engl J Med. 2012 Feb 16;366(7):610–8. doi:10.1056/NEJMoa1110352.
- Giannakeas V, Narod SA. Incidence of cancer among adults with thrombocytosis in Ontario, Canada. JAMA Network Open. 2021 Aug 12;4(8):e2120633. doi:10.1001/jamanetworkopen.2021.20633.
- Giannakeas V, Kotsopoulos J, Cheung MC, Rosella L, Brooks JD, Lipscombe L, Akbari MR, Austin PC, Narod SA. Analysis of platelet count and new cancer diagnosis over a 10-year period. JAMA Network Open. 2022 Jan 11;5(1):e2141633. doi:10.1001/jamanetworkopen.2021.41633.
- Giannakeas V, Kotsopoulos J, Brooks JD, Cheung MC, Rosella L, Lipscombe L, Akbari MR, Austin PC, Narod SA. Platelet count and survival after cancer. Cancers. 2022 Jan;14(3):549. doi:10.3390/cancers14030549.
- Plantureux L, Mège D, Crescence L, Dignat-George F, Dubois C, Panicot-Dubois L. Impacts of cancer on platelet production, activation and education and mechanisms of cancer-associated thrombosis. Cancers (Basel). 2018 Nov 14;10(11):441. doi:10.3390/cancers10110441.
- Menter DG, Tucker SC, Kopetz S, Sood AK, Crissman JD, Honn KV. Platelets and cancer: a casual or causal relationship: revisited. Cancer Metastasis Rev. 2014 Mar;33(1):231–69. doi:10.1007/s10555-014-9498-0.
- Gay LJ, Felding-Habermann B. Contribution of platelets to tumour metastasis. Nat Rev Cancer. 2011 Feb;11(2):123–34. doi:10.1038/nrc3004.
- Franco AT, Corken A, Ware J. Platelets at the interface of thrombosis, inflammation, and cancer. Blood. 2015 Jul 30;126(5):582–8. doi:10.1182/blood-2014-08-531582.
- George JN. Systemic malignancies as a cause of unexpected microangiopathic hemolytic anemia and thrombocytopenia. Oncology (Williston Park); 2011 Sep;25(10):908–14.
- Didelez V, Sheehan N. Mendelian randomization as an instrumental variable approach to causal inference. Stat Methods Med Res. 2007 Aug;16(4):309–30. doi:10.1177/0962280206077743.
- Gupta V, Walia GK, Sachdeva MP. “Mendelian randomization”: an approach for exploring causal relations in epidemiology. Public Health. 2017 Apr;145:113–19. doi:10.1016/j.puhe.2016.12.033.
- Sudlow C, Gallacher J, Allen N, Beral V, Burton P, Danesh J, Downey P, Elliott P, Green J, Landray M, et al. UK biobank: an open access resource for identifying the causes of a wide range of complex diseases of middle and old age. PLOS Med. 2015 Mar;12(3):e1001779. doi:10.1371/journal.pmed.1001779.
- von Elm E, Altman DG, Egger M, Pocock SJ, Gøtzsche PC, Vandenbroucke JP, STROBE Initiative. The strengthening the reporting of observational studies in epidemiology (STROBE) statement: guidelines for reporting observational studies. Int J Surg. 2014 Dec;12(12):1495–9. doi:10.1016/j.ijsu.2014.07.013.
- Therneau TM, Grambsch PM. Modeling survival data: extending the cox model. (NY): Springer; 2000. ( Statistics for biology and health).
- Frank EH. Hmisc: Harrell Miscellaneous [Internet]; 2024. https://CRAN.R-project.org/package=Hmisc.
- Achim Z, Torsten H. Diagnostic checking in regression relationships. R News. 2002;2(3):7–10.
- Meira-Machado L, Cadarso-Suárez C, Gude F, Araújo A. smoothHR: an R package for pointwise nonparametric estimation of hazard ratio curves of continuous predictors. Comput Math Methods Med. 2013;2013:1–11. doi:10.1155/2013/745742.
- R Core Team. R: a language and environment for statistical computing [Internet]; 2024. https://www.R-project.org/.
- Gieger C, Radhakrishnan A, Cvejic A, Tang W, Porcu E, Pistis G, Serbanovic-Canic J, Elling U, Goodall AH, Labrune Y, et al. New gene functions in megakaryopoiesis and platelet formation. Nature. 2011 Nov 30;480(7376):201–8. doi:10.1038/nature10659.
- Skrivankova VW, Richmond RC, Woolf BAR, Yarmolinsky J, Davies NM, Swanson SA, VanderWeele TJ, Higgins JPT, Timpson NJ, Dimou N. et al. Strengthening the reporting of observational studies in epidemiology using Mendelian randomization: the STROBE-MR statement. JAMA. 2021 Oct 26;326(16):1614–21. doi:10.1001/jama.2021.18236.
- Zheng J, Baird D, Borges MC, Bowden J, Hemani G, Haycock P, Evans DM, Smith GD. Recent developments in Mendelian randomization studies. Curr Epidemiol Rep. 2017;4(4):330–45. doi:10.1007/s40471-017-0128-6.
- Swerdlow DI, Kuchenbaecker KB, Shah S, Sofat R, Holmes MV, White J, Mindell JS, Kivimaki M, Brunner EJ, Whittaker JC. et al. Selecting instruments for Mendelian randomization in the wake of genome-wide association studies. Int J Epidemiol. 2016 Oct;45(5):1600–16. doi:10.1093/ije/dyw088.
- Burgess S, Dudbridge F, Thompson SG. Combining information on multiple instrumental variables in Mendelian randomization: comparison of allele score and summarized data methods. Statist Med. 2016 May 20;35(11):1880–906. doi:10.1002/sim.6835.
- Staley JR, Blackshaw J, Kamat MA, Ellis S, Surendran P, Sun BB, Paul DS, Freitag D, Burgess S, Danesh J. et al. PhenoScanner: a database of human genotype–phenotype associations. Bioinformatics. 2016 Oct 15;32(20):3207–9. doi:10.1093/bioinformatics/btw373.
- Kamat MA, Blackshaw JA, Young R, Surendran P, Burgess S, Danesh J, Butterworth AS, Staley JR. PhenoScanner V2: an expanded tool for searching human genotype–phenotype associations. Bioinformatics. 2019 Nov 1;35(22):4851–3. doi:10.1093/bioinformatics/btz469.
- Burgess S, Butterworth A, Thompson SG. Mendelian randomization analysis with multiple genetic variants using summarized data. Genet Epidemiol. 2013 Nov;37(7):658–65. doi:10.1002/gepi.21758.
- Bowden J, Davey Smith G, Burgess S. Mendelian randomization with invalid instruments: effect estimation and bias detection through Egger regression. Int J Epidemiol. 2015 Apr;44(2):512–25. doi:10.1093/ije/dyv080.
- Bowden J, Davey Smith G, Haycock PC, Burgess S. Consistent estimation in mendelian randomization with some invalid instruments using a weighted median estimator. Genet Epidemiol. 2016 May;40(4):304–14. doi:10.1002/gepi.21965.
- Verbanck M, Chen CY, Neale B, Do R. Detection of widespread horizontal pleiotropy in causal relationships inferred from mendelian randomization between complex traits and diseases. Nat Genet. 2018 May;50(5):693–8. doi:10.1038/s41588-018-0099-7.
- Hemani G, Zheng J, Elsworth B, Wade KH, Haberland V, Baird D, Laurin C, Burgess S, Bowden J, Langdon R. et al. The MR-Base platform supports systematic causal inference across the human phenome. eLife. 2018 May 30;7:e34408. doi:10.7554/eLife.34408.
- Staley JR, Burgess S. Semiparametric methods for estimation of a nonlinear exposure-outcome relationship using instrumental variables with application to mendelian randomization. Genet Epidemiol. 2017 May;41(4):341–52. doi:10.1002/gepi.22041.
- Liu J, Lichtenberg T, Hoadley KA, Poisson LM, Lazar AJ, Cherniack AD, Kovatich AJ, Benz CC, Levine DA, Lee AV. et al. An integrated TCGA pan-cancer clinical data resource to drive high-quality survival outcome analytics. Cell. 2018 Apr 5;173(2):400–16.e11. doi:10.1016/j.cell.2018.02.052.
- Moullet M, Funston G, Mounce LT, Abel GA, De Wit N, Walter FM, Zhou Y. Pre-diagnostic clinical features and blood tests in patients with colorectal cancer: a retrospective linked-data study. Br J Gen Pract. 2022 Aug;72(721):e556–63. doi:10.3399/BJGP.2021.0563.
- Tørring ML, Frydenberg M, Hamilton W, Hansen RP, Lautrup MD, Vedsted P. Diagnostic interval and mortality in colorectal cancer: U-shaped association demonstrated for three different datasets. J Clin Epidemiol. 2012 June;65(6):669–78. doi:10.1016/j.jclinepi.2011.12.006.
- Smock KJ, Perkins SL. Thrombocytopenia: an update. Int J Lab Hematol. 2014 June;36(3):269–78. doi:10.1111/ijlh.12214.
- Izzi B, Bonaccio M, De Gaetano G, Cerletti C. Learning by counting blood platelets in population studies: survey and perspective a long way after Bizzozero. J Thromb Haemostasis. 2018 Sep;16(9):1711–21. doi:10.1111/jth.14202.
- Kabat GC, Kim MY, Verma AK, Manson JE, Lin J, Lessin L. et al. Platelet count and total and cause-specific mortality in the women’s health initiative. Ann Epidemiol. 2017 Apr;27(4):274–80. doi:10.1016/j.annepidem.2017.02.001.
- Thaulow E, Erikssen J, Sandvik L, Stormorken H, Cohn PF. Blood platelet count and function are related to total and cardiovascular death in apparently healthy men. Circulation. 1991 Aug;84(2):613–7. doi:10.1161/01.CIR.84.2.613.
- Tsai MT, Chen YT, Lin CH, Huang TP, Tarng DC. U-shaped mortality curve associated with platelet count among older people: a community-based cohort study. Blood. 2015 Sep 24;126(13):1633–5. doi:10.1182/blood-2015-06-654764.
- Möhle R, Green D, Moore MAS, Nachman RL, Rafii S. Constitutive production and thrombin-induced release of vascular endothelial growth factor by human megakaryocytes and platelets. Proc Natl Acad Sci USA. 1997 Jan 21;94(2):663–8. doi:10.1073/pnas.94.2.663.
- Palumbo JS, Talmage KE, Massari JV, La Jeunesse CM, Flick MJ, Kombrinck KW, Jirousková M, Degen JL. Platelets and fibrin(ogen) increase metastatic potential by impeding natural killer cell–mediated elimination of tumor cells. Blood. 2005 Jan 1;105(1):178–85. doi:10.1182/blood-2004-06-2272.
- Erhart S, Beer JH, Reinhart WH. Influence of aspirin on platelet count and volume in humans. Acta Haematol. 1999;101(3):140–4. doi:10.1159/000040940.
- Rothwell PM, Wilson M, Price JF, Belch JF, Meade TW, Mehta Z. Effect of daily aspirin on risk of cancer metastasis: a study of incident cancers during randomised controlled trials. The Lancet. 2012 Apr 28;379(9826):1591–601. doi:10.1016/S0140-6736(12)60209-8.
- McNeil JJ, Nelson MR, Woods RL, Lockery JE, Wolfe R, Reid CM, Kirpach B, Shah RC, Ives DG, Storey E. et al. Effect of aspirin on all-cause mortality in the healthy elderly. N Engl J Med. 2018 Oct 18;379(16):1519–28. doi:10.1056/NEJMoa1803955.
- Schafer AI. Thrombocytosis. N Engl J Med. 2004 Mar 18;350(12):1211–19. doi:10.1056/NEJMra035363.
- Zhou CL, Su HL, Dai HW. Thrombopoietin is associated with a prognosis of gastric adenocarcinoma. Rev Assoc Med Bras (1992). 2020 May;66(5):590–5. doi:10.1590/1806-9282.66.5.590.
- Lin JJC, Eppinga RD, Warren KS, McCrae KR. Human tropomyosin isoforms in the regulation of cytoskeleton functions. Adv Exp Med Biol. 2008;644:201–22.
- Bailey MJ, Shield-Artin KL, Oliva K, Ayhan M, Reisman S, Rice GE. Stage-specific analysis of plasma protein profiles in ovarian cancer: difference in-gel electrophoresis analysis of pooled clinical samples. J Carcinog. 2013;12(1):10. doi:10.4103/1477-3163.114216.
- Li DQ, Wang L, Fei F, Hou YF, Luo JM, Wei-Chen. Identification of breast cancer metastasis-associated proteins in an isogenic tumor metastasis model using two-dimensional gel electrophoresis and liquid chromatography-ion trap-mass spectrometry. Proteomics. 2006 June;6(11):3352–68. doi:10.1002/pmic.200500617.
- Kopantzev EP, Monastyrskaya GS, Vinogradova TV, Zinovyeva MV, Kostina MB, Filyukova OB, Tonevitsky AG, Sukhikh GT, Sverdlov ED. Differences in gene expression levels between early and later stages of human lung development are opposite to those between normal lung tissue and non-small lung cell carcinoma. Lung Cancer. 2008 Oct;62(1):23–34. doi:10.1016/j.lungcan.2008.02.011.