Abstract
The combination of lean production and Industry 4.0 exhibits excellent potential in advancing operations management and, especially, production control. This improvement potential is applicable to the semi-process industry (S-PI), as higher product variety and higher demand variability require greater flexibility from production systems. This flexibility is difficult to achieve in S-PI environments, in which it is imperative to cope with the long setup times and stability needed for the high utilization requirements. Heijunka addresses this stability-flexibility paradox but was designed for short setup times and is thus considered not directly applicable. In this article, we use design science research to adapt Heijunka to the S-PI and combine it with cyber-physical systems technologies. The developed production control concept, every product every cycle (EPEC) 4.0, aims to schedule the production system as efficiently as possible, providing the necessary flexibility and minimum schedule perturbation. Furthermore, we present the findings from a validation case study within the automotive supply industry.
1. Introduction
The semi-process industry is facing a trend in demand towards higher product variety and higher demand variability that requires more flexibility from the applied production control approach to react to foreseen and unforeseen demand trends in a timely manner (van Rijn and Schyns Citation1993; Spenhoff, Semini, and Powell Citation2016; King Citation2019; Spenhoff et al. Citation2020). Such flexibility can be difficult to achieve in the semi-process industry when setup times are long and high utilization is required. A practical example of this is the automotive supply industry with the production of high volume serial parts and low volume spare parts production on the same production line. The aim of this study is to develop a production control concept that can enable both the required stability and the needed flexibility simultaneously.
Process industries (PI) are, according to APICS, ‘businesses that add value to materials by mixing, separating, forming, or chemical reactions. Processes may be either continuous or batch and generally require rigid process control and high capital investment’ (Fransoo and Rutten Citation1994, 48). This definition makes it clear that the PI cover a wide production range, from continuous to large- and small-batch production (van Donk and Fransoo Citation2006). Interestingly, many PI are actually hybrids (Billesbach Citation1994; Abdulmalek, Rajgopal, and Needy Citation2006). They produce discrete end items that originate from non-discrete materials. The semi-process industry (S-PI), a subset of the PI, is such a hybrid. An example of this hybridity is the extrusion of electrical wire (Groover Citation2016). In line with the Dutch Society for Production and Inventory Control (van Rijn and Schyns Citation1993), we can understand the S-PI as an industry that processes non-discrete materials in discrete batches and includes non-discrete as well as discrete production.
When it comes to production control in the S-PI, the literature has identified the following relevant industry-specific characteristics for describing the production environment and process (van Rijn and Schyns Citation1993; Spenhoff, Semini, and Powell Citation2016):
Limited production capacity (can usually only be extended by additional machines)
Production stops and restarts are time-intensive and costly
Large and expensive equipment, difficult to relocate
Setup times are product sequence-dependent
Need for high utilization of equipment
Temporary machine configurations
Product changeovers are complex
Output-driven production
Shelf-life constraints
Variable yield.
Our studies of companies in the S-PI reveal a trend in the following challenges for production control: higher product variety and higher demand variability (Cf. Spenhoff, Semini, and Powell Citation2016). These changes in demand trends have also been reported by other scholars for the PI, who also identified a need for new production control concepts as a means to address such challenges (Cf. Packowski and Francas Citation2014; Stevenson and Found Citation2016).
The change in demand within an increasingly cost-focussed and competitive environment in the PI (Stevenson and Found Citation2016) requires more flexibility. In contrast to this, production environment characteristics limit production control flexibility while demanding more stability simultaneously. This stability-flexibility paradox in the S-PI is summarized in .
Table 1. The Stability-flexibility paradox in the S-PI (based on (Spenhoff, Semini, and Powell Citation2016).
In line with our own studies (Cf. Spenhoff et al. Citation2014), other scholars have also reported the limited success of MRP (Dennis and Meredith Citation2000), the inadequacy of MRP in planning capacity (van Donk and Fransoo Citation2006), and the need to identify more appropriate production control concepts for the PI (Schuster, Allen, and D’Itri Citation2000; Stevenson and Found Citation2016). While academic research in this domain has been continuously advancing, implementation success in practice remains challenging for companies (Netland Citation2016). To a significant degree, the implementation success depends on the characteristics of the industry and the company-specific production system (Ashayeri et al. Citation2006; Slomp, Bokhorst, and Germs Citation2009; Panwar et al. Citation2015).
Product variety is a primary driver of higher demand flexibility (Bevilacqua, Ciarapica, and De Sanctis Citation2017) and greatly influences the stability of production systems. The alignment of demand and capacity is at the very core of lean production (Lyons et al. Citation2013). Lean production has previously been identified as a promising approach to addressing the stability-flexibility paradox in the S-PI (Spenhoff, Semini, and Powell Citation2016). Its focus on demand and flow offers excellent potential, especially since it provides features for stability, such as production levelling (Heijunka), just-in-time flexibility, a reduction in long setup times via single minute exchange of die (SMED), and the continuous improvement thinking of the lean philosophy.
Heijunka is one of the core principles of lean production as well as the classic method for scheduling in repetitive environments (Bicheno and Holweg Citation2016). It aims to achieve a levelled schedule combined with the visibility of said schedule and early problem highlighting. Furthermore, the Heijunka schedule acts as the pacemaker for material handling, enabling a regular flow of materials (Bicheno and Holweg Citation2016). With these characteristics, Heijunka establishes not only regularity and stability but also is able to anticipate the need for flexibility, offering a promising approach to addressing the stability-flexibility paradox. However, the application context of many lean production practices, including Heijunka, in discrete industries is fundamentally different from the listed characteristics of the S-PI (Crama, Pochet, and Wera Citation2001; Stevenson and Found Citation2016). This results in certain scepticism regarding the applicability of lean production in S-PI contexts (Bevilacqua, Ciarapica, and De Sanctis Citation2017). Especially challenging for the implementation of Heijunka in the S-PI are its long setup times as well as the need for high utilization (Bertrand, Wortmann, and Wijngaard Citation1990; Spenhoff, Semini, and Powell Citation2016; Stevenson and Found Citation2016). Therefore, the setup time has been utilized most recently as one aspect to differentiate the applicability of the Heijunka in the S-PI based on the takt/setup time ratio (Spenhoff et al. Citation2020).
Due to its success in discrete industries, the literature has reported considerable interest in the adaptation of lean production and Heijunka to the PI and S-PI. Some publications have reported positive results after the successful implementation of Heijunka adaptations for specific production systems in the PI (Glenday and Sather Citation2006; Pool, Wijngaard, and Van der Zee Citation2011; Packowski and Francas Citation2014; King Citation2019). Other publications have investigated this on a more aggregated level (Abdulmalek, Rajgopal, and Needy Citation2006; Lyons et al. Citation2013; Panwar et al. Citation2015; Stevenson and Found Citation2016), reporting that Heijunka could be partly applicable to the PI and help establish smoother production plans.
However, neither specific planning environments within the PI nor industrial applications have been investigated sufficiently, as only one study suggests a differentiated applicability of Kanban, takt time, and Heijunka for the S-PI and based on specific planning environment characteristics (Spenhoff et al. Citation2020). The literature reports existing Heijunka adaptations and implementations as mostly company- and situation-specific (Powell, Alfnes, and Semini Citation2010; Pool, Wijngaard, and Van der Zee Citation2011), making it difficult to generalize from a specific Heijunka implementation to the PI or S-PI (e.g. King (Citation2019)). Also, the reported Heijunka adaptations are rarely transferable and seldom applicable as guidelines for implementation in other production systems in the PI. While the adaptation of Heijunka to the S-PI shows potential, its direct application remains difficult due to long and complex changeovers (Coleman and Vaghefi Citation1994).
The existing literature suggests the adaptation of Heijunka to the PI and S-PI as promising and appropriate as a subject of further research. Reported empirical studies have demonstrated how such an adaption of lean production can improve operational performance (Lyons et al. Citation2013). More specifically, Heijunka seems to be appropriate for the PI and S-PI as it can support the stability and predictability of production (Powell, Alfnes, and Semini Citation2010; Stevenson and Found Citation2016; King Citation2019). Its schedule simplicity and transparency can further support closer coordination between production control and actual schedule execution (Abdulmalek, Rajgopal, and Needy Citation2006; Pool, Wijngaard, and Van der Zee Citation2011). However, the adaptation of Heijunka in the PI is an ongoing but sluggish process in both theory and practice (Melton Citation2005; Abdulmalek, Rajgopal, and Needy Citation2006; Pool, Wijngaard, and Van der Zee Citation2011; Lyons et al. Citation2013; Wilson and Ali Citation2014; Wilson Citation2014; Stevenson and Found Citation2016).
Multiple scholars built on the idea of a production cycle that contains an iterative sequence of all products to be produced. This sequence is known as every product every cycle (EPEC) (Cf. (Pool, Wijngaard, and Van der Zee Citation2011; Glenday and Sather Citation2013; Bicheno and Holweg Citation2016; Stevenson and Found Citation2016). However, previously published EPEC-related lean production control concepts, such as the product wheel (King Citation2019), the rhythm wheels (Packowski Citation2013), EPE (Powell, Alfnes, and Semini Citation2010), or lean rapid flexible supply (Glenday and Sather Citation2013) are not able to address the stability-flexibility paradox sufficiently, as they focus mostly on stability and do not simultaneously address the flexibility challenges.
Lean production and Industry 4.0 both have the potential to provide significant advantages for production control and share the common overall objective of flexibility (Thoben, Wiesner, and Wuest Citation2017; Buer, Strandhagen, and Chan Citation2018). Industry 4.0 has the potential to streamline the flow of information and enable better production control processes (Moeuf et al. Citation2018). Besides the flow of material, the flow of information is increasingly critical and has been previously identified as a ‘building block’ of production control (Dreyer et al. Citation2009). However, few specific studies and concepts combine lean production and Industry 4.0 (Mora et al. Citation2017; Cattaneo et al. Citation2017). Furthermore, recent literature has identified the support of Industry 4.0 technologies for lean production as a critical research area (Buer, Strandhagen, and Chan Citation2018). When considering the adaptation of Heijunka to the S-PI to address the stability-flexibility paradox, the support of Industry 4.0 technologies needs to be considered.
Consequently, the research objective of this article is to design an EPEC-based production control concept for the S-PI that is supported by Industry 4.0 technologies and combines stability with a definable level of flexibility. This objective will be achieved by adapting Heijunka and combining it with the cyber-physical systems (CPS) technologies of Industry 4.0. Thereby, the contribution of this study will go beyond previous results by (1) proposing a lean production control concept that accounts for the combination of both stability and flexibility and (2) integrating the support of Industry 4.0 technologies with lean production control.
The remainder of this article is structured as follows: Section 2 introduces and examines the existing literature on Heijunka, cyclic planning, relevant concepts for the application of Heijunka in the PI and S-PI, and Industry 4.0-enabled connectivity via CPS. Section 3 describes how the design science research methodology was applied in this research and introduces the case company. Section 4 presents the proposed production control concept design, EPEC 4.0, in detail. Section 5 summarises the results of the industrial case study that validated the appropriateness of EPEC 4.0. Section 6 discusses the application context within the S-PI as well as the benefits and limitations of EPEC 4.0. Section 7 concludes with the contributions to theory and practice, the limitations of the applied research methodology, and further research suggestions.
2. Theoretical background
Production control coordinates and includes the job scheduling of production systems. It considers future developments, evaluates alternatives for future activities, and provides directives for their implementation. Classical planning approaches usually build on the push-based concept of material requirement planning (MRP), which is a successive planning system (Fleischmann, Meyr, and Wagner Citation2008). MRP is used in most enterprise resource planning (ERP) hierarchical planning systems to decompose planning tasks into different levels (Jacobs et al. Citation2011). MRP focuses on production and procurement, while advanced planning systems (APS) integrate the planning of an entire supply chain and use optimization for the evaluation of planning alternatives (Fleischmann, Meyr, and Wagner Citation2008). In contrast, the lean production practices follow the pull-based approach. While both approaches, push-based MRP and pull-based lean production, have often been labelled as opposing concepts, synergies from a combined application (e.g. MRP and Kanban) have been identified and realized in practice (Wortmann and Monhemius Citation1984; Powell et al. Citation2013; Stevenson and Found Citation2016).
The customer order decoupling point (CODP) is a reference point that subdivides forecast-driven material flow upstream of the CODP from the customer order-driven material flow downstream of the CODP ((Olhager Citation2010); (van Donk and van Doorne Citation2016) citing (Sharman Citation1984; Hoekstra and Romme Citation1992)). The CODP is used as a decision support concept to decide where efficiency-related production techniques (e.g. make-to-stock (MTS)) and flexibility-related production techniques (e.g. make-to-order (MTO)) work best. Consequently, lean production- and forecasting-based techniques can serve to make processes as efficient as possible upstream of the CODP and can also support flexibility downstream of the CODP (van Donk and van Doorne Citation2016). In this study, the CODP is used to compare the ability of existing lean production control concepts to address different production techniques and to analyze the production system of the case company.
The discretization point (DP) is defined as a reference point where non-discrete units become discrete during the production process (Abdulmalek, Rajgopal, and Needy Citation2006). The DP has been previously reported as being essential for production control in the PI, as the application of lean production seems especially feasible downstream of the DP in the PI (Abdulmalek, Rajgopal, and Needy Citation2006) and S-PI (Pool, Wijngaard, and Van der Zee Citation2011). Therefore, we use the DP to subdivide the application context of lean production in the S-PI, as it is especially applicable downstream of the DP.
Heijunka, which is also referred to as production smoothing or levelling of the production schedule, is an essential component of lean production (Monden Citation1983; Womack, Jones, and Roos Citation1990; Hüttmeir et al. Citation2009; Bicheno and Holweg Citation2016). Furthermore, Heijunka is critical in creating lean production systems, as it is key for achieving stability (Womack and Jones Citation1996). The levelling aspect of Heijunka describes ‘the effort to balance the workload to be performed to the capacity or capability’ of the machines (Coleman and Vaghefi Citation1994, 31). Therefore, Heijunka has a dual objective:
The reduction of inventories due to mixed and very small batch production.
The associated ability to equate the workload of the production processes to both each other and capacity.
Heijunka aims to avoid production peaks and valleys in production schedules. This goal is either achieved by levelling the schedule with a repeating mixed model sequence or by levelling the production capacity with a pre-determined capacity plan and releasing orders to fill its slots (Cf. Hüttmeir et al. Citation2009; Matzka, Di Mascolo, and Furmans Citation2012; Bicheno and Holweg Citation2016). A repeating mixed model sequence is a specific form of cyclic planning (CP) that involves repetition of the same sequence. Therefore, we will review CP in more detail.
CP uses a fixed cyclic schedule to plan and coordinate production by ‘temporally reserving process steps to products in a preassigned, cyclic way’ (Groenevelt, Johansen, and Lederer 1996, 1). More recent literature identifies CP as being able to provide new ways of production smoothing (Hedenstierna and Disney Citation2018). Groenevelt, Johansen, and Lederer (Citation1996) describe the following potential benefits that can result from applying CP. For instance, CP makes it relatively easy to implement scheduling, improve material flow and material handling, shorten production lead times, reduce planning and control costs, decrease safety stock buffer inventories, and make simple order booking possible.
There is a vast body of literature on cyclic scheduling, which supports repeating short-term schedules that minimize setup and holding costs. A classic example is periodic batch control, a repeating single-cycle planning concept that simplifies production control by using the same throughput time for all products (Burbidge Citation1988; Burbidge Citation1994; Benders and Riezebos Citation2002). In contrast to cyclic scheduling, CP also focuses on the long-term strategic benefits arising from the use of cyclic schedules. As a result, many long-term organizational benefits of CP are not considered by the cyclic scheduling literature. Such long-term benefits could be, for example, those from a known and repeated production sequence or smoothing out variations. Within CP, production capacity is typically reserved before specific product demand is known (Groenevelt, Johansen, and Lederer Citation1996).
CP builds on the notion and relevance of the DP and is especially applicable downstream the DP. The flexibility of the product mix depends on relatively small changeover times (Pool, Wijngaard, and Van der Zee Citation2011). Dedicating production capacity to specific products supports more accurate capacity forecasts compared to heterogeneous job assignments and results in more accurate capacity and resource planning. This dedication allows for reductions in flow time and flow time variability, which means that CP has the potential to simplify production management and increase the efficiency of production resources. Additional potential benefits include a lower work-in-progress (WIP) inventory, decreased safety stock, increased customer responsiveness, and improved delivery reliability.
2.1. Literature analysis
Next, we will analyze the literature we reviewed on lean production control concepts that enable CP in the PI. Only a few publications have reported on the specific adaption of Heijunka to the PI or S-PI. Some scholars have reported company-specific adaptations that have been tested in practice (Groenevelt, Johansen, and Lederer Citation1996; Ashayeri et al. Citation2006; Fauske, Alfnes, and Semini Citation2008; Powell, Alfnes, and Semini Citation2010; Pool, Wijngaard, and Van der Zee Citation2011; Wilson and Ali Citation2014; Wilson Citation2014). Other literature has been published by practitioners or scholars with a very strong applied research focus who have been working with production control in the PI (Packowski Citation1996; Smalley Citation2004; Glenday and Sather Citation2006; Floyd Citation2010; Glenday and Sather Citation2013; King and King Citation2013; Packowski Citation2013; Packowski and Francas Citation2014; King Citation2019).
The reviewed literature that reports on adapting Heijunka to the PI or S-PI has been analyzed regarding the ability to establish stability for the production system and account for a certain level of flexibility simultaneously. In the best-case scenario, the reviewed concepts can additionally account for higher product variety and higher demand variability. First, we will present the analyzed cyclic concepts and their characteristics, which will be followed by a comparison of their ability to address the research objective.
2.1.1. Every product every (EPE)
EPE has been mentioned in recent sources by Bicheno and Holweg (Citation2016); however, Powell, Alfnes, and Semini (Citation2010) defined the application in more detail and presented a case study. EPE is a variant of EPEC that aims to establish stability and predictability for MTS. This goal is achieved through a fixed cyclic schedule that levels production volume and mix. In addition, SMED is utilized to reduce changeover times, which means EPE has the potential of reducing batch sizes and increasing total throughput, as it establishes stability through a repeated production schedule and diminishes the risk of the breakdown of an entire schedule.
2.1.2. Product wheel
The product wheel (King Citation2019) aims to establish a fixed cyclic production schedule for production lines with stable demand. The goal is to achieve a structured, regular production sequence, a so-called ‘wheel cycle’, with the shortest cycle time feasible for the production cycle. The changeover time between products is seen as a significant limiting factor of the production system. The wheel cycle is optimized based on the shortest changeover time between products in order to achieve the shortest possible cycle time.
2.1.3. Classic rhythm wheel
The classic rhythm wheel (Packowski Citation2013) is similar to the product wheel. It establishes a pre-defined repeated production schedule. The wheel cycle is fixed regarding time and product volume; therefore, it is not possible to address demand variations related to product mix and volume, but the wheel ensures high and stable resource utilization. To address long-term demand changes, the classic rhythm wheel needs regular review and re-design. Any short-term demand variations can only be addressed by high safety stock levels.
2.1.4. Breathing rhythm wheel
The breathing rhythm wheel (Packowski Citation2013) is an extension of the classic rhythm wheel. It provides additional cycle flexibility so that the wheel cycle length can vary. Focussing on high volume production lines with stable demand, the added cycle time flexibility allows for addressing demand variations to a certain extent. The choice of the wheel cycle time range determines the utilization rate of the equipment and the flexibility of the production system. Narrow wheel cycle time ranges support a higher utilization, while broader wheel cycle time ranges support more flexibility. Changes to the wheel cycle length require planning adjustment and need to be monitored with great care, as too much cycle time variability can disrupt the entire intention of using a wheel cycle.
2.1.5. High-mix rhythm wheel
The high-mix rhythm wheel (Packowski Citation2013) is another extension of the classic rhythm wheel. It is designed to address larger product portfolios, in which high- and low-volume products are still produced on the same production line but not all low-volume products are produced in every cycle. Instead, the low-volume products are scheduled to be produced only once every pre-specified cycle, with the option to manually account for a limited number of products with uncertain demand. As a result, multiple wheels with different wheel cycles need to be maintained, and higher inventories for low-volume products are required.
2.1.6. Lean rapid flexible supply (lean RfS)
Lean RfS (Glenday and Sather Citation2013) aims for full demand driven one-piece flow. Focussing not only on the level of single production lines but also on the supply chain level, lean RfS uses a five-step process to first achieve stability with a fixed sequence and volume, which reflects the idea of EPE. Each process step reduces the levelled volume until it is based on the actual demand. The goal is to unfix the sequence to achieve fully flexible demand-driven one-piece flow. However, this flexibility applies only to the sequence and volume and not to the product mix, as the products need to be scheduled in advance. A basic level of stability for the scheduled products is maintained using inventory buffers that are usually sized to accommodate half a week of the average demand.
2.2. Literature analysis summary
summarizes the analysis of the reviewed cyclic concepts and their ability to address the stability-flexibility paradox and account for the research objective of stability combined with a definable level of flexibility. The previously introduced CODP is used as an additional indicator of flexibility, as it shows whether MTS alone or also combined MTS/MTO can be addressed.
Table 2. Comparison of cyclic lean production control concepts.
In summary, the presented literature can be divided into two groups, with most concepts focussing on stability. Those focussing on flexibility seem to be more advanced and take stability as an existing precondition. However, none of the analyzed concepts can address both aspects of stability and flexibility simultaneously, as is needed. The trend in the S-PI from pure MTS to combined MTS/MTO (Cf. Crama, Pochet, and Wera Citation2001; Soman, van Donk, and Gaalman Citation2004; Iravani, Liu, and Simchi‐Levi Citation2012; Stevenson and Found Citation2016; Spenhoff et al. Citation2020) requires a strong focus on maintaining the necessary stability for the production system while supporting a certain level of flexibility, which, in turn, allows for greater responsiveness to short-term customer demand. This CODP shift results in an urgent and increasing need to realize this capability in practice.
2.3. Industry 4.0-enabled connectivity
Based on the Industry 4.0 definition of Moeuf et al. (Citation2018, 1118) citing Kohler and Weisz (Citation2016), we refer to Industry 4.0-enabled connectivity for production control as ‘a new approach for controlling production processes by providing real-time synchronization of flows’. A key element of Industry 4.0 is a deep change in the connectivity of production systems (Kagermann et al. Citation2013; Schwab Citation2017). Multiple authors report on Internet-of-Things (IoT) and CPS as main enabling technologies, which integrate production and network connectivity (Hofmann and Rüsch Citation2017; Buer, Strandhagen, and Chan Citation2018; Xu, Xu, and Li Citation2018).
IoT offers advanced connectivity of physical objects, enables object-to-object communication, and data sharing with the production system over the internet (Zhong et al. Citation2017; Fatorachian and Kazemi Citation2018). It enables real-time data collection and its further processing (Sanders, Elangeswaran, and Wulfsberg Citation2016; Babiceanu and Seker Citation2016). IoT relies on technologies to manage the wireless tracking of all objects, their origin, current status, and their destination via integrated devices for communication.
CPS integrate physical processes and digital technologies (Thoben, Wiesner, and Wuest Citation2017; Buer, Strandhagen, and Chan Citation2018). They manage the interconnected systems of physical objects and available computational capabilities (Lee, Bagheri, and Kao Citation2015). CPS are seen as the central element of Industry 4.0 (Thoben, Wiesner, and Wuest Citation2017) and as the ‘system of systems’, which can manage the interconnectivity of production assets and products realized via IoT.
CPS control two parallel networks. On the physical level, the network of interconnected objects on the shop floor, and on the cyber level, the digital network (Parvin et al. Citation2013). Real-time data from production assets, products, and other connected objects on the shop floor can be collected and transferred to the digital network. Advanced information analytics can process this data into relevant information about physical assets that can become available in real-time (Lee, Bagheri, and Kao Citation2015). As a result, the flow of information improves significantly from the perspectives of quality, automation and temporal availability, and granularity (Buer, Strandhagen, and Chan Citation2018). Real-time systems connected to transactional systems, such as ERP, provide a major opportunity to keep the transactional backbone of a company up-to-date. In turn, this allows production control to be based on timely and accurate information. Moreover, analytical information on production problems and workflows can be collected and used to improve quality control and maintenance and to improve the parameters used for production control. Such automated information analytics capabilities are a significant enabler in make production systems more efficient, collaborative, and resilient (Lee, Bagheri, and Kao Citation2015).
2.4. Industry 4.0-supported lean production control
Industry 4.0 has been reported for its potential to increase the flexibility of lean production (Rüttimann and Stöckli Citation2016) and the maturity of lean implementations (Roy, Mittag, and Baumeister Citation2015). The importance of accurate and up-to-date information is not mentioned often in the literature, but it is a prerequisite for a lean production control concept that can provide consistent advantages (Bevilacqua, Ciarapica, and Paciarotti Citation2015). The flow of information becomes the foundation for establishing a proper flow of material.
3. Research methodology
Design science research (DSR) is a domain-independent research strategy focussing on developing knowledge on generic actions, processes, and systems to address field problems or exploit promising opportunities. DSR aims for creating improvements based on a thorough understanding of such problems or opportunities (van Aken, Chandrasekaran, and Halman Citation2016). It is a solution-oriented methodology, building on description-oriented research and supporting explanatory disciplines that aim to create generic knowledge that is motivated by specific field problems (van Aken Citation2014).
The DSR methodology is a valid and valuable research methodology, especially in engineering sciences. The design of an explicitly applicable solution to a problem is a well-accepted engineering research paradigm in disciplines such as information systems research (Peffers et al. Citation2007) and operations management (van Aken, Chandrasekaran, and Halman Citation2016).
3.1. Design science research strategy and research method
DSR projects are typically motivated by a field problem and contain two main components: A descriptive/explanatory component, analyzing the chosen problem, its causes and context, and a design component, developing a generic design for the problem (van Aken, Chandrasekaran, and Halman Citation2016). The descriptive/explanatory component of this DSR project has been reported previously in Spenhoff et al. (Citation2014) and Spenhoff, Semini, and Powell (Citation2016). This study focuses on the design component. To develop a generic design for a problem, the DSR strategy can alternatively address a class of problems building a meta-artefact as a general solution or address a client-specific problem that creates a unique artefact from which a general solution emerges to address a class of problems (Iivari Citation2015). This research follows the latter strategy and addresses a general solution distilled from a specific problem encountered in an industrial case.
A crucial element in DSR is the design proposition, which links the artefact and its intended outcome to the field of application (Holmström and Romme Citation2012). depicts the design proposition using the Context, Intervention, Mechanism, Outcome (CIMO) framework (Pawson and Tilley Citation1997; Denyer, Tranfield, and Van Aken Citation2008).
Table 3. Design proposition.
The industrial case was used for both main components of the DSR project of investigating the specific problem and designing the artefact. The case company has been involved in several revision cycles of the designed artefact and these iterations have served to validate the appropriateness of the generic artefact design. This approach had the advantage of validating the artefact in the same company in which the problem was investigated and discussing it with the same individuals who had been encountering the problem for many years. Artefact design validation is an important aspect of DSR. It evaluates the future use and effectiveness of the artefact in its context (van Aken, Chandrasekaran, and Halman Citation2016). The artefact design validation case study was conducted via focus groups and semi-structured interviews. Similar to panel studies, focus groups can be used to make predictions, like the future use and effectiveness of a design artefact (Cf. Flynn et al. Citation1990; Stewart and Shamdasani Citation2014; van Aken, Chandrasekaran, and Halman Citation2016), and represent an appropriate means to validate the artefact design.
Research rigour has been addressed by appropriate data collection and analysis techniques during the problem investigation, construction, and evaluation phase of the artefact design (Cf. Von Alan et al. Citation2004). It was essential for the research to place a major emphasis on the pragmatic validity and practical relevance of the study.
3.2. Case study selection and specific problem description
The case company is an automotive metal parts producer supplying automotive original equipment manufacturers (OEM) with aluminium-made bumper beams. Their production system covers the entire value creation process through a multi-stage production process, starting with raw material and ending with the finished product, which is made in large batches. The case company can be classified as part of the PI and, more specifically, as part of the S-PI. In the following, their production system’s characteristics are detailed, the applied production control approach is described, and the specific production control-related challenges of their production system are summarized.
3.2.1. Production system detailed description
The detailed description of the production system outlines the characteristics that are critical for production control in this case and are related to the dimensions of the production process, product, and demand.
The production process contains three major production stages: casting, extrusion, and forming. It starts with the casting of non-discrete materials in intermittent batches. These materials are extruded and then formed on different production lines into discrete end items. The production system exhibits an early DP in the first production stage of casting. Significant constraints are observable in a capacity bottleneck during extrusion, which exhibits long setup times. The plan is to use the available capacity as close to 100% utilization as possible. In case the demand exceeds the total available capacity, the equipment is the limiting factor of the production system capacity. As the regular production schedule utilizes a two-shift system, it is possible to increase the equipment utilization by modifying the working shifts (i.e. switching to a three-shift system and make use of an additional night shift and the weekend). Additional shifts imply significant increasing labour costs, and represent a major change of the working conditions for employees that affects their productivity. Shift changes should be done rarely and only after giving sufficiently advanced notice.
The production involves heavy machinery and product-item specific tools such that switching the product always requires changing the set of tools on the production lines. This tool change is a complex task requiring significant time, labour, and material, which results in long lead and changeover times. The setup times consist of not only the internal setup times of the lines, which are often already long (i.e. more than 10 minutes), but also external setup time, which includes the preparation of tools and usually takes several hours. This results in a labour-intensive production process, as the setup, the feeding of the production lines, and the transfer of WIP products between the production stages requires manual work. While the application of SMED helped to reduce the setup times substantially, they remain a crucial challenge that motivates the strong interest of the company towards further implementation of lean production to improve the flow of material. Other motivations stem from the low level of automation and desire to improve the flow of information through Industry 4.0 application. summarizes the characteristics critical for production control in this case related to product and demand.
Table 4. Critical production control characteristics of the case company.
As it can be seen, the contracted delivery period has a major influence on the demand. Over time, bumper beams transform from serial parts for new vehicles into spare parts for aging vehicle models. Automotive parts typically have high demand during the initial four- to seven-year period. After this serial production phase, the company is contracted to deliver the product as a spare part for at least another 15 years. While the main product variants of the product group ‘spare parts I’ have a similar demand pattern as serial parts but with lower volumes, the additional product variants of product group ‘spare parts II’ appear as high-variety and very low-volume rush-orders that need to be produced within two to three weeks once an order is received.
3.2.2. Applied production control approach
The applied production control approach is a two-step and MRP-based process that can be described as a utilization of the long-term and short-term planning horizon (Cf. Berry and Hill Citation1992; Jacobs et al. Citation2011). At the master production scheduling level, the company performs a high-level material requirement planning approach:
The ERP system performs the related offsetting and netting after the receipt of a customer order. Later, this is manually controlled by the production planners in terms of capacity restrictions and is manually adjusted. For each production stage, a four-week production schedule is created based on the data in the ERP system. This schedule is seen as a 1 + 3 weeks planning schedule. The first week describes the firm production schedule that is planned to be executed. Beyond that, the plan for weeks two to four is more of a forecast, as it is very likely to be updated before execution.
The planning is taken directly to the shop floor level by following the push principle. Based on the four-week rolling production schedule, a final daily production schedule is confirmed every morning. A second and final offsetting, netting, and capacity balancing is done based on the detailed shop floor data. This second step incorporates rescheduled delivery dates, specific setup times, physical inventory, and the actual availability of operators and machines. This detailed level of planning is within the responsibility of the department manager on the shop floor.
3.2.3. Production system challenges
The case company was purposively selected based on the fact that it had faced many of the typical challenges of MRP-based production control in the S-PI (Cf. Spenhoff, Semini, and Powell Citation2016). In addition, the case company was highly receptive to supporting this research motivated by their need for stability and flexibility, and the following production control challenges:
Increasing product variety, product demand variability, and volume variability.
Focus on high resource utilization and large batch sizes results in overproduction and subsequent high inventories of WIP and finished goods.
The planned utilization is often too high for the actual schedule execution when considering delays and the actual shop floor situation. The production schedule requires repeated updating. The optimal sequence of changeovers cannot be followed, and production time is often lost due to increased starting and stopping time losses.
Time-consuming planning process on the shop floor every morning.
Changes in demand cause additional frequent plan changes and costly rush orders to ensure on-time delivery.
As a result of these challenges, the flow of information and the flow of material are not synchronized, and the production costs are consistently higher than anticipated.
4. The EPEC 4.0 production control concept
EPEC 4.0 is the problem-specific artefact that emerged to address the situation of the case company. It is a lean production control concept supported by Industry 4.0 technologies for the S-PI that addresses the flow of material and the flow of information. These are the two main pillars of EPEC 4.0: Pillar I, the flow of material, is addressed with the adaptation of Heijunka and pillar II, the flow of information, is addressed by integrating the advanced connectivity of Industry 4.0 managed via CPS technologies. Guided by the overall idea of demand-driven flow, EPEC 4.0 incorporates a hybrid push-pull strategy that combines push-based stability with a certain level of pull-based flexibility. The EPEC 4.0 production control concept includes four main elements: (1) product categorization, (2) cyclic master schedule, (3) detailed cyclic schedule, 4) CPS.
Product categorization uses the lean concept of runners, repeaters, and strangers to subdivide the product portfolio into product groups. The schedule generation follows a hierarchical control approach (Cf. Müller, Tolujew, and Kienzle Citation2014; Stadtler, Kilger, and Meyr Citation2015), with two planning levels, a cyclic master schedule and a detailed cyclic schedule.
The cyclic master schedule represents the schedule on an aggregated and capacity-oriented level. It balances the overall demand and available capacity for a planning horizon of several cycles. Runners and repeaters are scheduled in a fixed sequence with a levelled production volume as MTS. EPEC 4.0 operates with a finished goods inventory for runners, which is used as a ‘capacity stock’ buffer to compensate for possible deviations between the levelled production volume and actual demand volume. Strangers are scheduled on an aggregated level as one entity by reserving so-called ‘free slots’.
The detailed cyclic schedule is confined to the next cycle and assigns specific strangers as MTO to the free slots. The detailed cyclic schedule is executed on the shop floor level.
The CPS support the flow of information by managing physical assets and computational capabilities. CPS embody a prognostics and health management system for automated shop floor data collection and processing and act as a decision support system for the production planner. Furthermore, they can provide the foundation for a resilient control system and pave the way towards automated decision-making and schedule execution control.
EPEC 4.0 aims to run the production system as efficiently as possible with as much flexibility as needed and the stability of as few ad hoc schedule changes as possible. While it is desired to maintain an unchanged cyclic master schedule for as many cycles as possible to achieve maximum stability, the detailed cyclic schedule is intended to be fixed as late as possible to allow for maximum flexibility. is a schematic of the EPEC 4.0 production control concept, illustrating the two planning levels above shop floor execution and the support of CPS.
4.1. Pillar I: the flow of material
Pillar I addresses the flow of material and features the capacity-oriented and item-oriented production control. Both aspects will now be explained in detail.
4.1.1. Capacity-oriented production control
Initially, the product portfolio is classified based on its demand frequency and demand volume into runners, repeaters, and strangers. Runners are fast moving products with stable high-volume demand. Repeaters are slower moving products. Their demand is still stable, but with a lower frequency and lower volume. Strangers are volatile low-volume products that have a high product variety, high demand variability, and more uncertain demand predictability. Strangers are often ordered on short notice (e.g. rush orders). The categorization into runners, repeaters, and strangers provides the input for the cyclic master schedule (Cf. ).
Cyclic master schedule
The cyclic master schedule aligns available production capacity with overall product demand. Furthermore, it addresses the optimal product sequence for an overall minimum changeover time between all products of the cycle.
Stability and predictability for runners and repeaters are achieved by levelling the production volume over several cycles. Based on the projected demand for an extended period of time, the levelled production volume and production frequency for each runner and repeater are determined. This determination is supported by historical data, available forecasts, and call-off data. By levelling the production volume, the produced volume equals the average demand and results in a controlled finished goods inventory. This alignment enables the compensation for demand peaks without adjusting the production volume level. Unlike runners and repeaters, the demand for strangers is variable and uncertain. This demand pattern results in a need for greater flexibility, which means that, in the cyclic master schedule, strangers are addressed through free slots.
To identify the optimal product sequence, the levelled production volumes and often sequence-dependent changeover and setup times between products are considered in designing the cyclic master schedule. This design step first involves runners and repeaters. Free slots are integrated into this sequence at segments for which the total minimum changeover time of the cycle is least affected. Strangers are assigned to free slots in the detailed cyclic schedule, which supports a flexible accommodation for actual demand. shows an example of a cyclic master schedule. In this example, one cycle covers one week, with the weekend as additional, unassigned capacity.
Representing the capacity-oriented production control level, the cyclic master schedule needs to be periodically checked to ensure that overall demand is in balance with the available capacity. Adjustments could be necessary due to seasonal or other changes in demand. The periodicity of this formal capacity control depends, in general, on the demand stability of runners and repeaters. For some periods, the available capacity might not be able to fulfil the overall demand (i.e. due to high demand seasons, factory closure times, overhaul, or more extensive and planned maintenance activities). In such cases, our concept of stocked capacity (Bertrand, Wortmann, and Wijngaard Citation1990) recommends storing capacity in the form of finished inventory. Stocked capacity differs in its intention from regular finished goods inventory control. The intention is not to build up buffers on the item level but to create a dedicated inventory to meet the overall demand during periods in which it is anticipated that demand volume exceeds the overall capacity volume.
Stocked capacity is an additional part of the cyclic master schedule and is planned and controlled separately from the finished goods inventory. Compared to regular inventory control, the in-/decrease of stocked capacity needs to be considered during planning as it is built up based upon the available capacity. Depletion of the stocked capacity is controlled based on the actual demand and production capacity situation.
This capacity-oriented control element is used to ensure that the dedicated inventory surpluses are used to mitigate foreseeable future shortages. As production capacity can only be stored in finished products, runners, as fast-moving products with stable demand, are stored in the stocked capacity inventory. Stocked capacity is especially useful for exceptional cases, such as factory closure periods, when demand continues during the shutdown. Moreover, substantial capacity will be needed for the backlog of stranger demand after such a period.
4.1.2. Item-oriented production control
Detailed cyclic schedule
The detailed cyclic schedule builds on the cyclic master schedule and assigns the free slots to specific strangers. If there are more free slots than demand for strangers, they can be used for supportive activities like total productive maintenance (TPM) or SMED.
Beyond this, there might be situations in which supporting activities do not fill all remaining free slots. In such a case, there are two options: the first focusses strictly on lean thinking and demand-based production. If a free slot is not needed to supply a specific demand, the slot would not be used. The multi-skilled workforce would be assigned to different tasks during this free slot. The second option is to account for the need for high utilization in the S-PI and the potential need to increase the stocked capacity. As a result, the free slot would provide additional capacity if a runner precedes the free slot, thereby extending the levelled volume. Such an extension can also be used to smooth out deviations caused by variable yield, machine breakdowns, or other output limiting events (Arica, Haskins, and Strandhagen Citation2016).
illustrates an example of a detailed cyclic schedule. The figure shows how the free slots from the cyclic master schedule (Cf. ) have been assigned to specific strangers. As their production volume is low, two strangers share one slot in this detailed cyclic schedule.
Another aspect that needs to be considered for the flow of material is the material supply. Runners and repeaters have a more stable demand. Therefore, the material supply can be forecast and made available. However, this is not the case for strangers, as their demand is variable, difficult to predict, and often occurs on short notice. This means that material inventory for strangers needs to be built up to meet the maximum reasonable demand while accounting for the replenishment time.
The replenishment lead time of materials is used to ensure that sufficient material is available to produce the demanded products and that information regarding material availability and current volumes is always accurate. The latter is addressed by the flow of information within EPEC 4.0.
4.2. Pillar II: the flow of information
Pillar II addresses the flow of information and includes the CPS architecture and its advanced information analytics capabilities. We describe this in detail and explain the implications from both production assets and product perspectives.
CPS architecture
The scheduled flow of the material of EPEC 4.0 and its control is largely dependent on the availability of up-to-date and accurate information from two perspectives: the demand perspective and the shop floor production perspective. From the shop floor production perspective, this dependency applies specially to demand-related situations that require more flexibility (e.g. short-term orders or order changes) as well as production-related situations (e.g. machine breakdowns), and is valid for any deviations that need to be addressed to ensure the on-time execution of the detailed cyclic schedule and the satisfaction of customer orders. CPS improve the availability of this information and support the efficient flow of material while enabling the necessary level of flexibility of the EPEC 4.0 schedule during any given period.
The use of CPS addresses the flow of information through a five-level process that realises three supportive systems. summarizes the EPEC 4.0 CPS architecture as well as the specific functions that are utilized.
Figure 4. The EPEC 4.0 cyber-physical systems architecture (based on Lee, Bagheri, and Kao (Citation2015).
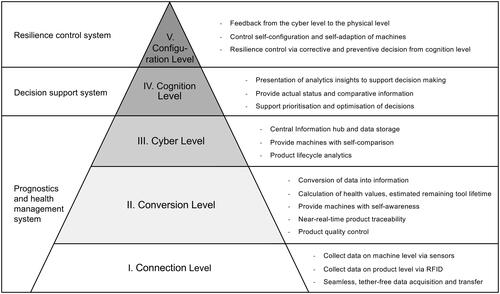
4.2.1. Production assets perspective
CPS enable networked machines to utilize advanced information analytics. The three realized supportive systems itemized in the left hand of are described here in detail, based on Lee, Bagheri, and Kao (Citation2015).
The prognostics and health management system builds on data collected at the machine level (level I) via sensors from the production resources. This automated data collection encompasses not only the actual production lines and their machines but also inventory storage areas and transportation vehicles. Via advanced information analytics this data can be converted (level II) into information concerning machine health, the prediction of degradation and performance, the status and availability of material and products, and stocked capacity. Both the automated flow of information and the high availability of information support production control with very accurate tracking of each machine’s status and the status of the detailed cyclic schedule execution. This interconnectedness can provide the machine level with self-awareness of its status. As a central information hub, the cyber level (level III) can internally compare current information to historical records and the actual status of previous situations. This comparison is able to identify trends and patterns and is especially relevant for tracking the performance of each machine and the prediction of potential productivity limitations that require maintenance, for example. This information builds the basis for the decision support system.
The decision support system aggregates the acquired insights from the analytics and supports the decision-making process (level IV) of the logistics manager while creating the EPEC 4.0 detailed schedule by providing relevant information to assign specific strangers to free slots or making recommendations to adjust the detailed cyclic schedule. In case of larger disruptions, changes to the cyclic master schedule can be proposed. This support is especially useful for the handling of order changes, machine breakdowns, or when productivity limitations are predicted. If, for example, a maintenance need is predicted, it can be scheduled as a TPM activity for a free slot for one of the next detailed cyclic schedules based on the predicted urgency and availability of the free capacity, as illustrated in .
The resilience control system can automate the decision-making process (level V) and provide feedback from the cyber level back to the physical level. Based on the collected information from the shop floor, the cyber-based decisions for schedule updates and adjustments can be communicated to the production lines and their machines. The resilience control system can maintain a self-configuration and self-adaption of the schedule on the machine level and oversee the execution of manual decisions taken by the production planner by collecting new data from the shop floor.
4.2.2. Product perspective
EPEC 4.0 initially uses CPS to improve the production control-related flow of information and addresses the perspective of production assets. In addition, there are also benefits from the product perspective.
The measurement of data on the product level (level I) via sensors (e.g. radio-frequency identification (RFID) or similar technology) enables the CPS to register and track materials and products on the batch or individual product level. Both materials and product volumes can be tracked, and individual items can be located. The availability of this information enables not only near-real-time traceability but also is relevant for the production quality of products and the quality assurance process. As a central information hub, the cyber level (level II) makes information available while serving as long-term data storage for all information. Information on product-specific level can be found at a later point in time, and the product history can be traced back to its used materials and their sources.
Beyond internal traceability during the production phase (level II), the product-related data can also be used for the further distribution path of the customers, enabling advanced supply chain traceability and new product lifecycle management opportunities. In summary, the CPS-managed flow of information provides improvements from a product perspective both during the production phase and later in supporting the product lifecycle analytics of customers.
4.3. Handling of demand changes
As yield is variable in the S-PI, so is customer demand, which exhibits variations in volume. EPEC 4.0 reacts to such yield fluctuations and changes in demand through a two-level approach:
Compensation at the aggregate level via finished goods inventory or stocked capacity. The levelled production volume is based on the average demand and expected fluctuations in demand. As a result, a controlled finished goods inventory builds up for individual products and is used to cover their small demand fluctuations. In addition, however, higher demand fluctuations may arise that require average levelled capacity in a more significant way (e.g. high season or factory closing times). Such situations are accounted for with stocked capacity: additional stocks in runners allow for surplus capacity to be used, anticipating periods of capacity storage during which these stocks are depleted. Using these two inventory mechanisms, EPEC 4.0 is expected to be able to account for fluctuations in yield and demand.
Schedule adjustments of individual products. In some cases, it might not be possible to compensate for the greater demand of an individual product than planned via the stocked capacity and finished goods inventory. Addressing such demand changes requires an exceptional adjustment of the detailed cyclic schedule when it is possible to cope with a demand change by using free slots or adjusting runners and repeaters within one specific cycle. If a change is affecting more than one cycle, an adjustment of the cyclic master schedule is required.
5. Validation at the case company
The results of the case study provided indications as to how EPEC 4.0 can support production control at the case company. The case study was conducted via two focus group discussions with the production planners and line managers and two semi-structured interviews with the lean manager and former logistics manager. Altogether, the eight participants and seven hours of discussions and interviews provided abundant data on the applicability of the artefact design in practice. This included detailed feedback on the expected efficacy and benefits of the implementation related to the flow of material and the flow of information. The validation case study results below are structured according to the main elements of EPEC 4.0 and first list the related question(s) used in the focus groups and semi-structured interviews. The subsequent summarized results are reinforced by selected participant remarks.
5.1. Overall goal of EPEC 4.0
Do the two aspects of stability and flexibility address the core challenges of production control at your company?
According to the former logistics manager, production planners, and production line managers, the combination of stability and flexibility addresses the core challenges of production control for the case company. There was consensus that stability was more important than flexibility, while both are required, and that it is essential for the company to address the flow of material and the flow of information through an integrated approach.
According to the lean manager, an Industry 4.0-supported lean production control concept has been confirmed as ‘a suitable means’ to address the production control challenges the company is facing. Lean production and Industry 4.0 both play a crucial strategic role in the current and future endeavours of the company in improving its production system.
5.2. Cyclic master schedule and detailed cyclic schedule
How important is the alignment of demand changes with the available capacity in the cyclic master schedule?
Does the free slots concept accommodate short-term orders in a useful way?
Compared to today, will the detailed cyclic schedule () be an improvement for the production lines?
According to the production planners, the alignment of demand and overall available capacity ‘is of critical importance’. Together with the former logistics manager, the production planners rated the free slots concept as ‘very useful’ in accounting for short-term orders and order changes. The production line managers view the free slots as ‘very important’, while they ‘prefer a maximum of stability’. They evaluated the flexibility of EPEC 4.0 as an ‘enabler of the stability of the schedule’. Furthermore, all participants, and, especially, the production line managers, rated the concept of stocked capacity as ‘highly needed’. All participants expect the finished goods inventory to cover the expected short-term fluctuations of demand. The production planners stated that this buffer is intended to be sized ‘between 10 and 15% of the annualized output’ for the case company. According to the production line managers, the detailed cyclic schedule would be a ‘significant improvement’ for the production lines.
5.3. Cyber-physical systems
Would it be useful to connect the manufacturing execution system (MES) software used on the shop floor with the ERP software in a central information hub via CPS technologies to make all data for planning available in near-real-time?
Especially for the currently planned and future automated production lines, the production planners mentioned the use of CPS technologies as ‘essential and of strategic importance to automate production’. They stated that automated decision-making will be ‘required in the future for all production lines’, and automated and near-real-time information would improve the stability of material and tool availability for the production lines. Furthermore, the production planners stated that this would support production control with stability in material supply and enhance responsiveness to demand changes.
5.4. Schedule adjustments
Do the options for schedule adjustment cover the situations occurring in your daily business?
The production line managers and production planners stated that the options for schedule adjustments to handle changes cover all situations occurring in the daily business of the case company.
5.5. Potential benefits
Do you see an advantage of EPEC 4.0 over the current approach for production control?
As an overall conclusion, the production line managers and production planners stated that EPEC 4.0 would present an advantage over today’s MRP-based production control approach. The production line managers stated that they specifically see an advantage from ‘the gained stability and availability of current information’. According to the production line managers, this advantage is expected due to the stability of the production lines, the predictability of the schedule, the stability and predictability of the material supply upstream, and the stability and predictability of the maintenance of tools. The production planners stated that they see a ‘clear advantage in the lean material flow, the automation of the information flow, and the advanced information analytics capabilities’. Furthermore, they highlighted EPEC 4.0 as a ‘must have’ for their company ‘to stay competitive in the future’. The production planners quantified the expected benefits of EPEC 4.0 for the company based on two of their key inventory performance indicators. For WIP, they expected a decrease of 50% or more, while for finished goods inventory they expected it to nearly double, which is in line with the expected effect of levelled production and the use of stocked capacity.
6. Discussion
EPEC 4.0 improves production control by addressing the shortcomings of MRP in the ‘correct order’, as the validation case company stated: combining the required push-based stability with the needed level of pull-based flexibility. The resulting hybrid push-pull strategy shows excellent potential in addressing the stability-flexibility paradox in the S-PI with its flexibility-requiring and flexibility-limiting production environment characteristics. Regarding the application context of EPEC 4.0, two aspects need to be considered.
The product portfolio needs more than a few products. There is no need to create an EPEC 4.0 schedule for a production system producing only two or three products.
The S-PI shows different characteristics upstream the DP than downstream. EPEC 4.0 is designed to be applied downstream the DP.
The benefits that can be expected from EPEC 4.0 are listed here and grouped related to aspects of stability and flexibility, and additional expected efficiency improvements are stated, before existing limitations are discussed.
6.1. Stability
The combined push-pull system of EPEC 4.0 can address regular and volatile demand simultaneously. The regularity of EPEC 4.0 addresses the regular demand (i.e. its fixed, repeated production schedule maintains a predefined production cycle). Then, the flow of material becomes balanced.
This solution results in higher predictability for the fixed production interval with stable and levelled capacity utilization. Furthermore, output volumes and delivery dates are more predictable, and forecasts for suppliers of material consumption and their deliveries become more predictable as well. The buffering of demand variations and stocked capacity account for periods with higher demand than the available capacity.
Reliability is achieved from a more robust schedule and a higher product quality. This higher reliability allows for a reduction in safety stocks. In addition, production planners can more accurately plan with EPEC 4.0, which is more straightforward and transparent compared to the described MRP-based production control approach. Furthermore, customers can better tune their internal processes with product deliveries by building upon the shared knowledge of the cyclic EPEC 4.0 pattern. A reduced production system nervousness is expected, not only for the production system itself but also for the entire supply chain. Finally, less ‘firefighting’ and ‘administrative coordination’ is required for production control and the schedule execution.
6.2. Flexibility
EPEC 4.0 can manage higher product variety and higher demand variability to a certain extent, as it addresses combined MTS/MTO. The free slots concept enables higher responsiveness to short-term demand and short-term demand changes.
The Industry 4.0-enabled connectivity and its central element CPS enable the improved and automated flow of information by connecting physical assets and computational capabilities to realize a near-real-time availability of information. Materials, WIP, and finished products become traceable at any time. WIP and the finished goods inventory contain only controlled items. The support of these technologies can realize three different support systems for: (1) prognosis and health management, through automated shop floor data collection and processing; (2) decision support for production control; and (3) resilience control for automated decision-making for the schedule execution control.
6.3. Efficiency improvements
EPEC 4.0 realizes an optimized production sequence that considers the shortest possible setup times. This reduction in total setup time improves both the average capacity utilization and planning efficiency (i.e. instead of building plans from scratch each week, planning becomes an exercise of filling in the free slots). In addition, SMED targets the continuous reduction of setup times to include more products more frequently in shorter production cycles.
6.4. EPEC 4.0 limitations
Besides these expected benefits, EPEC 4.0 has certain limitations. First, the demand pattern requires that a majority of products exhibit stable demand. If more than 30% of the demand is unknown, other production control concepts might be a better option, as the flexibility of EPEC 4.0 is limited and reflected in the schedule via free slots. Second, the trade-off between stability and flexibility needs to be balanced by the targeted capacity utilization, which influences the size of the free slots. Furthermore, it should be said that EPEC 4.0 does not define an optimal batch size. It is meant as a conceptual design for production control. This focus is also the reason why no simulation was applied in this study. Simulation is used to consider dynamic effects like the sequence-dependent setup and waiting times, which are situated on a very detailed level, below the conceptual design. As other scholars have stated for similarly sized production systems such as the one used in this research, modelling would be ambitious and perhaps an impossible task (De Smet and Gelders Citation1998). This gauge also applies to EPEC 4.0, mainly as the human factor will, at least for some years, continue to play an essential role for production control in the S-PI. This human aspect only recently has begun to be considered in mathematical modelling (Grosse et al. Citation2017; De Sanctis, Ordieres Meré, and Ciarapica Citation2018).
7. Conclusions
The S-PI is facing a trend of higher product variety and higher demand variability, which requires production systems to not only be stable in their output but also more flexible in reacting to new demand patterns. Traditionally applied production control approaches face limitations in addressing this stability-flexibility paradox adequately. Lean production practices, in general, as well as the specific adaptation of Heijunka, show great potential for the S-PI. Obstacles to implementation, such as complex changeovers with long setup times, have prevented the direct implementation of Heijunka thus far. Existing concepts for the PI or S-PI that adapted Heijunka focussed either on establishing stability for the production system or on targeting flexibility without putting emphasis on maintaining the necessary stability. However, current challenges in the S-PI require a combined approach of stability and certain flexibility. In addition, meeting these challenges will require improvements in the flow of information (e.g. with the use of Industry 4.0 technologies and their advanced information analytics capabilities).
With EPEC 4.0, we present the design of a production control concept that adapts Heijunka to the S-PI. EPEC 4.0 not only addresses the flow of material but also the flow of information. It aims to run the production system as efficiently as possible, with as much flexibility as needed, and with as few ad hoc schedule changes as possible. The concept of free slots introduces flexibility for reacting responsively to demand changes without interfering with the stable execution of the pre-defined cycle. The hybrid push-pull strategy combines push-based stability for runners and repeaters with a certain level of pull-based flexibility for strangers. With the support of the Industry 4.0-enabled connectivity and its central element CPS, the flow of information is automated, and routine decisions can also be automated.
7.1. Contribution to theory
This research contributes to theory in multiple ways. First, it contributes to the theory of production planning and control by proposing a production control concept that enables combined stability and flexibility in the S-PI. Second, it contributes to the further adaptation of lean production practices in the PI. Third, it advances knowledge on the adaptation of lean production practices to the S-PI using the specific adaptation of Heijunka and considers the combined need for stability and flexibility. Fourth, it integrates Industry 4.0 and lean production for a digitized and automated flow of information that becomes the foundation for the flow of material.
7.2. Contribution to practice
In addition, this research makes several contributions to practice. First, it provides a conceptual design for the implementation of Heijunka for the S-PI that can be used for specific production systems. Second, it outlines the importance of the flow of information for the flow of material as well as ways in which to improve both in a combined approach. Third, it shows how lean production and Industry 4.0 can be combined in practice.
7.3. Limitations and further research
There are a few limitations of the applied DSR methodology that should be mentioned. First, the designed artefact has not been tested by its implementation in practice. The case company clearly stated that this would be a priority project for them, but, first, a new version of their ERP system and the installation of new, automated production lines need to be put in place. These actions are currently underway at the case company, but these prerequisites to testing EPEC 4.0 in practice could not be met during the validation case study. Nevertheless, qualified personnel reached a consensus regarding the perceived feasibility, efficacy, and usefulness of the designed artefact. Second, even if the design artefact was validated at the case company, EPEC 4.0 cannot be claimed to be a generally applicable solution in the S-PI domain. However, it is a context-specific and promising design artefact rated as having much potential.
Additional research should test EPEC 4.0 through its implementation in specific production environments in the S-PI. It would also be interesting to adapt EPEC 4.0 for an application upstream of the DP. Synchronizing the flow of material for multiple production stages to achieve true flow for a batch through the entire production system would be another interesting aspect of further research. Such a ‘one-batch-flow’ is especially interesting, as batch production is the primary production mode in the S-PI, and different production stages within the same production system might have differing batch size requirements.
Finally, the use of CPS and the transition to Industry 4.0 will require significant investment in relevant physical sensors and hardware, and the associated information technology infrastructure that needs to be aligned with the operations and business strategy.
Acknowledgements
The authors would like to express their gratitude to the representatives of the case company for the excellent and long-term cooperation. We would like to thank Cecilia Haskins for her careful review and thoughtful comments. Furthermore, we would like to thank the anonymous reviewers of the peer-review process for their very valuable comments to improve the paper.
Disclosure statement
No potential conflict of interest was reported by the author(s).
Additional information
Funding
Notes on contributors
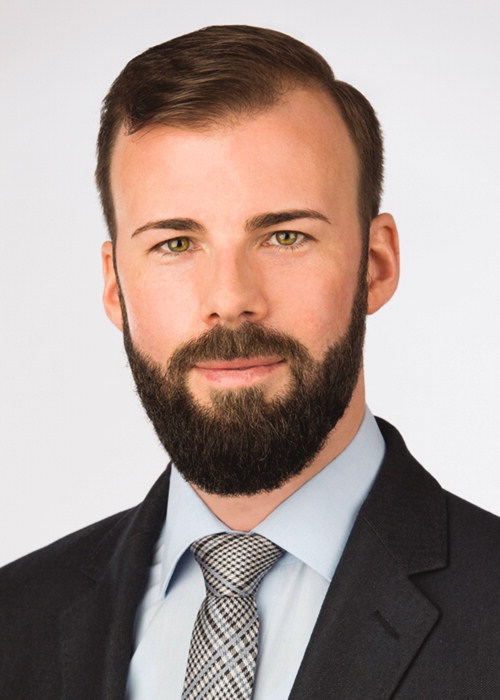
Philipp Spenhoff
Philipp Spenhoff is a PhD Candidate and Lecturer at the Department of Mechanical and Industrial Engineering at the Norwegian University of Science and Technology (NTNU) in Trondheim, Norway. He is an IT Expert in Systems Integration, holds a B.Sc. in Information Systems and Management, and a European M.A. in International Project Management. His research interests cover both the theory and practical application of lean production and Industry 4.0. His research builds on a strong background in the industry with 15 years of experience at Siemens and in various research and consulting projects. His pro bono work includes three years as vice president and board member in DION NTNU.
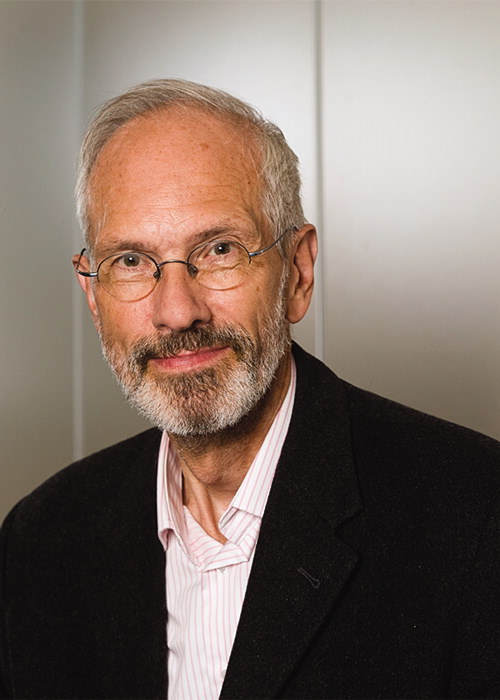
Johan C. (Hans) Wortmann
Johan C. (Hans) Wortmann is a Full Professor of information management at the Faculty of Economics and Business within the University of Groningen (RuG) in the Netherlands. His particular field of interest is in enterprise information systems. For 24 years he was the Editor-in-Chief of ‘Computers in Industry’. Before joining RuG, he was Vice-President of R&D at Baan, a vendor of standard enterprise software. Before joining Baan, he was a Full Professor in industrial information systems at Eindhoven University of Technology (TUE) where he gained much experience with innovation in science-based industries. He advised many companies in various industrial branches on the selection and implementation of information systems.
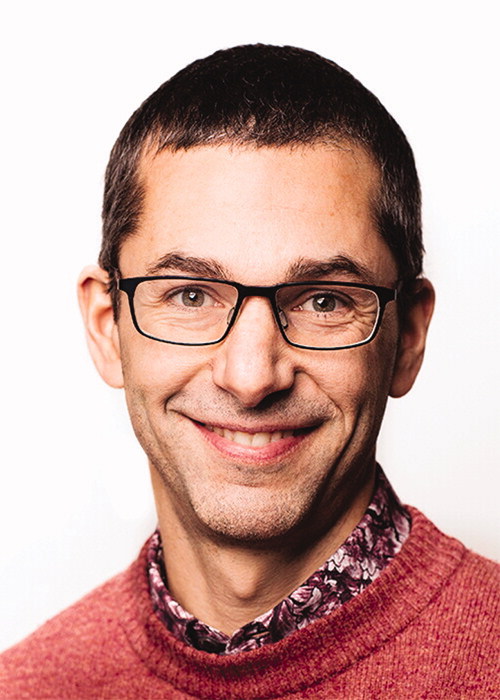
Marco Semini
Marco Semini is an Associate Professor in Manufacturing Logistics at the Department of Mechanical and Industrial Engineering at the Norwegian University of Science and Technology (NTNU) in Trondheim, Norway. He holds a master degree in mathematics from the University of Lausanne, Switzerland and a PhD in Manufacturing Logistics from NTNU, Norway. His research interests cover the areas of operations management, production planning and control, manufacturing strategy, and simulation and modelling. In parallel to his involvement in academia, he has been for many years a multi-project manager at the industrial research consultancy organization SINTEF in the areas of production, logistics, and supply chain management.
References
- Abdulmalek, Fawaz A., Jayant Rajgopal, and Kim LaScola Needy. 2006. “A Classification Scheme for the Process Industry to Guide the Implementation of Lean.” Engineering Management Journal 18 (2): 15–25. doi:10.1080/10429247.2006.11431690.
- Arica, Emrah, Cecilia Haskins, and Jan O. Strandhagen. 2016. “A Framework for Production Rescheduling in Sociotechnical Manufacturing Environments.” Production Planning & Control 27 (14): 1–205. doi:10.1080/09537287.2016.1193910.
- Ashayeri, J., R. J. M. Heuts, H. G. L. Lansdaal, and L. W. G. Strijbosch. 2006. “Cyclic Production-Inventory Planning and Control in the Pre-Deco Industry: A Case Study.” International Journal of Production Economics 103 (2): 715–725. doi:10.1016/j.ijpe.2006.02.001.
- Babiceanu, R. F., and R. Seker. 2016. “Big Data and Virtualization for Manufacturing Cyber-Physical Systems: A Survey of the Current Status and Future Outlook.” Computers in Industry 81: 128–137. doi:10.1016/j.compind.2016.02.004.
- Benders, J., and J. Riezebos. 2002. “Period Batch Control: Classic, Not Outdated.” Production Planning & Control 13 (6): 497–506. doi:10.1080/09537280210162941.
- Berry, W. L., and T. Hill. 1992. “Linking Systems to Strategy.” International Journal of Operations & Production Management 12 (10): 3–15. doi:10.1108/01443579210017204.
- Bertrand, J. W. M., J. C. Wortmann, and J. Wijngaard. 1990. Production Control: A Structural and Design Oriented Approach. Amsterdam: Elsevier.
- Bevilacqua, M., F. E. Ciarapica, and C. Paciarotti. 2015. “Implementing Lean Information Management: The Case Study of an Automotive Company.” Production Planning & Control 26 (10): 753–768. doi:10.1080/09537287.2014.975167.
- Bevilacqua, M., F. E. Ciarapica, and I. De Sanctis. 2017. “Lean Practices Implementation and Their Relationships with Operational Responsiveness and Company Performance: An Italian Study.” International Journal of Production Research 55 (3): 726–769. doi:10.1080/00207543.2016.1211346.
- Bicheno, J., and M. Holweg. 2016. The Lean Toolbox: A Handbook for Lean Transformation. 5th ed. Buckingham: PICSIE Books.
- Billesbach, T. J. 1994. “Applying Lean Production Principles to a Process Facility.” Production and Inventory Management Journal 35 (3): 40–44.
- Buer, S.-V., J. O. Strandhagen, and F. T. S. Chan. 2018. “The Link between Industry 4.0 and Lean Manufacturing: Mapping Current Research and Establishing a Research Agenda.” International Journal of Production Research 56 (8): 2924–2940. doi:10.1080/00207543.2018.1442945.
- Burbidge, J. 1988. “Operation Scheduling with GT and PBC.” International Journal of Production Research 26 (3): 429–442. doi:10.1080/00207548808947874.
- Burbidge, J. 1994. “The Use of Period Batch Control (PBC) in the Implosive Industries.” Production Planning & Control 5 (1): 97–102. doi:10.1080/09537289408919475.
- Cattaneo, L., M. Rossi, E. Negri, D. Powell, and S. Terzi. 2017. “Lean Thinking in the Digital Era.” Paper Presented at the IFIP International Conference on Product Lifecycle Management. Cham.
- Coleman, B. J., and M. R. Vaghefi. 1994. “Heijunka: A Key to the Toyota Production System.” Production and Inventory Management Journal 35: 31–35.
- Crama, Y., Y. Pochet, and Y. Wera. 2001. A Discussion of Production Planning Approaches in the Process Industry." In CORE Discussion Paper: 2001/42. Ottignies-Louvain-la-Neuve: Center for Operations Research and Econometrics (CORE). http://hdl.handle.net/2078.1/4196.
- De Sanctis, I., J. Ordieres Meré, and F. E. Ciarapica. 2018. “Resilience for Lean Organisational Network.” International Journal of Production Research 56 (21): 6917–6936. doi:10.1080/00207543.2018.1457810.
- De Smet, R., and L. Gelders. 1998. “Using Simulation to Evaluate the Introduction of a Kanban Subsystem within an MRP-Controlled Manufacturing Environment.” International Journal of Production Economics 56–57: 111–122. doi:10.1016/S0925-5273.
- Dennis, D. R., and J. R. Meredith. 2000. “An Analysis of Process Industry Production and Inventory Management Systems.” Journal of Operations Management 18 (6): 683–699. doi:10.1016/S0272-6963(00)00039-5.
- Denyer, D., D. Tranfield, and J. E. van Aken. 2008. “Developing Design Propositions through Research Synthesis.” Organization Studies 29 (3): 393–413. doi:10.1177/0170840607088020.
- Dreyer, H. C., E. Alfnes, J. O. Strandhagen, and M. Kolberg Thomassen. 2009. “Global Supply Chain Control Systems: A Conceptual Framework for the Global Control Centre.” Production Planning & Control 20 (2): 147–157.
- Fatorachian, H., and H. Kazemi. 2018. “A Critical Investigation of Industry 4.0 in Manufacturing: Theoretical Operationalisation Framework.” Production Planning & Control 29 (8): 633–644. doi:10.1080/09537287.2018.1424960.
- Fauske, H., E. Alfnes, and M. Semini. 2008. “Lean Supply Chain Control in Hydro Automotive Structures.” Paper Presented at the IFIP International Conference on Advances in Production Management Systems (APMS), Espoo.
- Fleischmann, B., H. Meyr, and M. Wagner. 2008. “Advanced Planning.” In Supply Chain Management and Advanced Planning, edited by Stadtler H., Kilger C., 81–106. Berlin, Heidelberg: Springer. https://doi.org/10.1007/978-3-540-74512-9_5
- Floyd, R. C. 2010. Liquid Lean: Developing Lean Culture in the Process Industries. New York: CRC Press.
- Flynn, B., S. Sakakibara, R. Schroeder, K. Bates, and J. Flynn. 1990. “Empirical Research Methods in Operations Management.” Journal of Operations Management 9 (2): 250–284. doi:10.1016/0272-6963(90)90098-X.
- Fransoo, J. C., and W. Rutten. 1994. “A Typology of Production Control Situations in Process Industries.” International Journal of Operations & Production Management 14 (12): 47–57. doi:10.1108/01443579410072382.
- Glenday, I., and R. Sather. 2006. Breaking through to Flow. Ross-on-Wye: Lean Enterprise Academy.
- Glenday, I., and R. Sather. 2013. Lean RFS (Repetitive Flexible Supply): Putting the Pieces Together. Boca Raton: CRC Press.
- Groenevelt, H., J. Johansen, and P. J. Lederer. 1996. Cyclic Planning. Rochester: University of Rochester.
- Groover, M. P. 2016. Principles of Modern Manufacturing: Materials, Processes, and Systems, SI version. 6th global ed. New York: Wiley.
- Grosse, E. H., M. Calzavara, C. H. Glock, and F. Sgarbossa. 2017. “Incorporating Human Factors into Decision Support Models for Production and Logistics: Current State of Research.” IFAC-PapersOnLine 50 (1): 6900–6905. doi:10.1016/j.ifacol.2017.08.1214.
- Hedenstierna, C. P. T., and S. M. Disney. 2018. “Avoiding the Capacity Cost Trap: Three Means of Smoothing under Cyclical Production Planning.” International Journal of Production Economics 201: 149–162. doi:10.1016/j.ijpe.2018.04.008.
- Hoekstra, S., and J. Romme. 1992. Integral Logistic Structures: Developing Customer-Oriented Goods Flow. London: McGraw-Hill
- Hofmann, E., and M. Rüsch. 2017. “Industry 4.0 and the Current Status as Well as Future Prospects on Logistics.” Computers in Industry 89: 23–34. doi:10.1016/j.compind.2017.04.002.
- Holmström, J., and A. G. L. Romme. 2012. “Guest Editorial: Five Steps towards Exploring the Future of Operations Management.” Operations Management Research 5 (1–2): 37–42. doi:10.1007/s12063-011-0060-8.
- Hüttmeir, A., S. de Treville, A. van Ackere, L. Monnier, and J. Prenninger. 2009. “Trading off between Heijunka and Just-in-Sequence.” International Journal of Production Economics 118 (2): 501–507. doi:10.1016/j.ijpe.2008.12.014.
- Iivari, J. 2015. “Distinguishing and Contrasting Two Strategies for Design Science Research.” European Journal of Information Systems 24 (1): 107–115. doi:10.1057/ejis.2013.35.
- Iravani, S. M. R., T. Liu, and D. Simchi‐Levi. 2012. “Optimal Production and Admission Policies in Make‐to‐Stock/Make‐to‐Order Manufacturing Systems.” Production and Operations Management 21 (2): 224–235. doi:10.1111/j.1937-5956.2011.01260.x.
- Jacobs, F. R., W. L. Berry, T. E. Vollmann, and D. C. Whybark. 2011. Manufacturing Planning and Control for Supply Chain Management. 6th ed. New York: McGrawHill/Irwin.
- Kagermann, H., J. Helbig, A. Hellinger, and W. Wahlster. 2013. Recommendations for Implementing the Strategic Initiative INDUSTRIE 4.0: Securing the Future of German Manufacturing Industry; Final Report of the Industrie 4.0 Working Group. Frankfurt: Forschungsunion.
- King, P. L. 2019. Lean for the Process Industries: Dealing with Complexity. Second Edition. New York: Productivity Press, T&F.
- King, P. L., and J. S. King. 2013. The Product Wheel Handbook: Creating Balanced Flow in High-Mix Process Operations. Boca Raton: CRC Press.
- Kohler, D., and J.-D. Weisz. 2016. Industry 4.0: Les Défis de la Transformation Numérique du Modèle Industriel Allemand. [Industry 4.0: The Challenges of the Digital Transformation of the German Industrial Model]. Paris: La Documentation française.
- Lee, J., B. Bagheri, and H.-A. Kao. 2015. “A Cyber-Physical Systems Architecture for Industry 4.0-Based Manufacturing Systems.” Manufacturing Letters 3: 18–23. doi:10.1016/j.mfglet.2014.12.001.
- Lyons, A. C., K. Vidamour, R. Jain, and M. Sutherland. 2013. “Developing an Understanding of Lean Thinking in Process Industries.” Production Planning & Control 24 (6): 475–494. doi:10.1080/09537287.2011.633576.
- Matzka, J., M. Di Mascolo, and K. Furmans. 2012. “Buffer Sizing of a Heijunka Kanban System.” Journal of Intelligent Manufacturing 23 (1): 49–60. doi:10.1007/s10845-009-0317-3.
- Melton, T.. 2005. “The Benefits of Lean Manufacturing: What Lean Thinking Has to Offer the Process Industries.” Chemical Engineering Research and Design 83 (6): 662–673. doi:10.1205/cherd.04351.
- Moeuf, A., R. Pellerin, S. Lamouri, S. Tamayo-Giraldo, and R. Barbaray. 2018. “The Industrial Management of SMEs in the Era of Industry 4.0.” International Journal of Production Research 56 (3): 1118–1136. doi:10.1080/00207543.2017.1372647.
- Monden, Y. 1983. Toyota Production System. Norcross: Industrial Engineering and Management Press.
- Mora, E., P. Gaiardelli, B. Resta, and D. Powell. 2017. “Exploiting Lean Benefits through Smart Manufacturing: A Comprehensive Perspective.” Paper Presented at the IFIP International Conference on Advances in Production Management Systems (APMS), Hamburg.
- Müller, E., J. Tolujew, and F. Kienzle. 2014. “Push-Kanban – a Kanban-Based Production Control Concept for Job Shops.” Production Planning & Control 25 (5): 401–413. doi:10.1080/09537287.2012.701021.
- Netland, T. H. 2016. “Critical Success Factors for Implementing Lean Production: The Effect of Contingencies.” International Journal of Production Research 54 (8): 2433–2448. doi:10.1080/00207543.2015.1096976.
- Olhager, J. 2010. “The Role of the Customer Order Decoupling Point in Production and Supply Chain Management.” Computers in Industry 61 (9): 863–868. doi:10.1016/j.compind.2010.07.011.
- Packowski, J. 2013. LEAN Supply Chain Planning: The New Supply Chain Management Paradigm for Process Industries to Master Today's VUCA World. Boca Raton: CRC Press.
- Packowski, J., and D. Francas. 2014. “Lean SCM.” Die Pharmazeutische Industrie 76 (1): 69–73.
- Packowski, J. 1996. Betriebsführungssysteme in der Chemischen Industrie. Wiesbaden: Gabler.
- Panwar, A., B. P. Nepal, R. Jain, and A. P. S. Rathore. 2015. “On the Adoption of Lean Manufacturing Principles in Process Industries.” Production Planning & Control 26 (7): 564–587. doi:10.1080/09537287.2014.936532.
- Parvin, S., F. K. Hussain, O. K. Hussain, T. Thein, and J. S. Park. 2013. “Multi-Cyber Framework for Availability Enhancement of Cyber Physical Systems.” Computing 95 (10–11): 927–948. doi:10.1007/s00607-012-0227-7.
- Pawson, R., and N. Tilley. 1997. Realistic Evaluatio. London: Sage.
- Peffers, K., T. Tuunanen, M. A. Rothenberger, and S. Chatterjee. 2007. “A Design Science Research Methodology for Information Systems Research.” Journal of Management Information Systems 24 (3): 45–77. doi:10.2753/MIS0742-1222240302.
- Pool, A., J. Wijngaard, and D.-J. Van der Zee. 2011. “Lean Planning in the Semi-Process Industry, a Case Study.” International Journal of Production Economics 131 (1): 194–203. doi:10.1016/j.ijpe.2010.04.040.
- Powell, D., E. Alfnes, and M. Semini. 2010. “The Application of Lean Production Control Methods within a Process-Type Industry: The Case of Hydro Automotive Structures.” Paper presented at the IFIP International Conference on Advances in Production Management Systems (APMS), Cernobbio, Italy.
- Powell, D., E. Alfnes, J. O. Strandhagen, and H. Dreyer. 2013. “The Concurrent Application of Lean Production and ERP: Towards an ERP-Based Lean Implementation Process.” Computers in Industry 64 (3): 324–335. doi:10.1016/j.compind.2012.12.002.
- Roy, D., P. Mittag, and M. Baumeister. 2015. “Industrie 4.0 - Einfluss der Digitalisierung auf die fünf Lean-Prinzipien - Schlank vs. Intelligent.” Productivity Management 20 (2): 27–30.
- Rüttimann, B. G., and M. T. Stöckli. 2016. “Lean and Industry 4.0—Twins, Partners, or Contenders? A Due Clarification regarding the Supposed Clash of Two Production Systems.” Journal of Service Science and Management 09 (06): 485–500. doi:10.4236/jssm.2016.96051.
- Sanders, A., C. Elangeswaran, and J. Wulfsberg. 2016. “Industry 4.0 Implies Lean Manufacturing: Research Activities in Industry 4.0 Function as Enablers for Lean Manufacturing.” Journal of Industrial Engineering and Management 9 (3): 811–833. doi:10.3926/jiem.1940.
- Schuster, E. W., S. J. Allen, and M. P. D’Itri. 2000. “Capacitated Materials Requirements Planning and Its Application in the Process Industries.” Journal of Business Logistics 21 (1): 169–189.
- Schwab, K. 2017. The Fourth Industrial Revolution. New York: Crown Business.
- Sharman, G. 1984. “The Rediscovery of Logistics.” Harvard Business Review 62 (5): 71–79.
- Slomp, J., J. A. C. Bokhorst, and R. Germs. 2009. “A Lean Production Control System for High-Variety/Low-Volume Environments: A Case Study Implementation.” Production Planning & Control 20 (7): 586–595. doi:10.1080/09537280903086164.
- Smalley, A. 2004. Creating Level Pull: A Lean Production-System Improvement Guide for Production-Control, Operations, and Engineering Professionals. Cambridge: Lean Enterprise Institute.
- Soman, C. A., D. P. van Donk, and G. Gaalman. 2004. “Combined Make-to-Order and Make-to-Stock in a Food Production System.” International Journal of Production Economics 90 (2): 223–235. doi:10.1016/S0925-5273(02)00376-6.
- Spenhoff, P., J. C. Wortmann, M. Semini, D. J. Powell, J. O. Strandhagen, and E. Alfnes. 2021. “Differentiating the Application of Lean Production Practices in the Semi-Process Industry: A Typology.” Production Planning & Control. Working paper.
- Spenhoff, P., M. Semini, and D. J. Powell. 2016. “Investigating Production Planning and Control Challenges in the Semi-Process Industry, the Case of a Metal Parts Producer.” Paper presented at the IEEE International Conference on Industrial Engineering and Engineering Management (IEEM), Bali, Indonesia.
- Spenhoff, P., M. Semini, E. Alfnes, and J. O. Strandhagen. 2014. “The Fit of Planning Methods for the Part Manufacturing Industry, A Case Study.” Advances in Manufacturing 2 (2): 165–172. doi:10.1007/s40436-014-0075-0.
- Stadtler, H., C. Kilger, and H. Meyr. 2015. Supply Chain Management and Advanced Planning. Berlin: Springer.
- Stevenson, S., and P. Found. 2016. “Completely Taktless! What is Pull in the Context of the Process Industries?” In Understanding the Lean Enterprise: Strategies, Methodologies, and Principles for a More Responsive Organization, edited by Andrea Chiarini, Pauline Found and Nicholas Rich, 153–184. Cham: Springer.
- Stewart, D. W., and P. N. Shamdasani. 2014. Focus Groups: Theory and Practice. Vol. 20. Los Angeles: Sage publications.
- Thoben, K.-D., S. Wiesner, and T. Wuest, BIBA – Bremer Institut für Produktion und Logistik GmbH, the University of Bremen 2017. “Industrie 4.0" and Smart Manufacturing – a Review of Research Issues and Application Examples.” International Journal of Automation Technology 11 (1): 4–16. doi:10.20965/ijat.2017.p0004.
- van Aken, J., A. Chandrasekaran, and J. Halman. 2016. “Conducting and Publishing Design Science Research: Inaugural Essay of the Design Science Department of the Journal of Operations Management.” Journal of Operations Management 47–48 (1): 1–8. doi:10.1016/j.jom.2016.06.004.
- van Aken, J. 2014. “Two Hurdles to Take for Maximum Impact of Design Science Research in the is-Field.” Design Science 447: 28–40.
- van Donk, D. P., and J. C. Fransoo. 2006. “Operations Management Research in Process Industries.” Journal of Operations Management 24 (3): 211–214. doi:10.1016/j.jom.2005.01.001.
- van Donk, D. P., and R. van Doorne. 2016. “The Impact of the Customer Order Decoupling Point on Type and Level of Supply Chain Integration.” International Journal of Production Research 54 (9): 2572–2584. doi:10.1080/00207543.2015.1101176.
- van Rijn, M. J., and B. V. P. Schyns. 1993. MRP in Process: The Applicability of MRP-II in the Semi-Process Industry. Assen: Van-Gorcum.
- Von, A., R. Hevner, S. T. March, J. Park, and S. Ram. 2004. “Design Science in Information Systems Research.” MIS Quarterly 28 (1): 75–105. doi:10.2307/25148625.
- Wilson, S., and N. Ali. 2014. “Product Wheels to Achieve Mix Flexibility in Process Industries.” Journal of Manufacturing Technology Management 25 (3): 371–392. doi:10.1108/JMTM-03-2012-0026.
- Wilson, S. 2014. “Sequencing in Process Manufacturing – the Product Wheel Approach.” Paper Presented at the Proceeding of International Production and Operations Management Society (POMS) Conference, Singapore.
- Womack, J. P., and D. T. Jones. 1996. “From Lean Production to the Lean Enterprise.” IEEE Engineering Management Review 24 (4): 38–46.
- Womack, J. P., D. T. Jones, and D. Roos. 1990. The Machine That Changed the World: The Story of Lean Production. New York: Simon & Schuster.
- Wortmann, J. C., and W. Monhemius. 1984. “Kanban – Its Use as a Final Assembly Scheduling Tool within MRP.” Paper presented at the International Conference on Operational Research (IFORS), Washington, DC, USA, August 6–10.
- Xu, L. D., E. L. Xu, and L. Li. 2018. “Industry 4.0: State of the Art and Future Trends.” International Journal of Production Research 56 (8): 2941–2962. doi:10.1080/00207543.2018.1444806.
- Zhong, R. Y., X. Xu, E. Klotz, and S. Newman. 2017. “Intelligent Manufacturing in the Context of Industry 4.0: A Review.” Engineering 3 (5): 616–630. doi:10.1016/J.ENG.2017.05.015.