Abstract
Food waste is an alarming issue pertaining to the rising global hunger, huge environmental footprint, and high monetary value. In developing and developed nations, it occurs primarily due to inefficiencies upstream and downstream of the supply chain respectively. A common factor in both developed and developing nations is product flow within the supply chain from farms to retailers. This study aims to identify the root causes of waste generated across the product flow of the beef supply chain from farm to retailer. A workshop involving twenty practitioners of the beef industry was conducted and the collected information was transcribed and coded to generate a current reality tree, which assisted in identifying root causes of waste in the entire beef supply chain. A multi-agent architecture framework spanning the entire beef supply chain from farm to retailer is proposed, which is composed of autonomous agents capable of bringing all segments of the beef industry on a single platform and collaboratively assist them in mitigating root causes of waste. The proposed framework will aid the practitioners in the beef industry to reduce waste, improve their operational efficiency thereby raising food security, economic development whilst curbing their carbon footprint.
1. Introduction
It is estimated that one-third of the aggregate food produced globally (∼1.3 billion tonnes/annum) ends up as waste (FAO Citation2019a). It directly impacts some of the crucial issues across the globe. The foremost is that around 820 million people are suffering from chronic hunger thereby creating a bottleneck in achieving United Nations Sustainable Development Goal no. 2- Zero Hunger (FAO Citation2019b). The food wasted within the supply chain could be utilised to feed them. There are also environmental implications of wasting food as lots of resources (land, water, and energy) are being exploited for producing it. The food waste generated is also being disposed to landfills leading to the generation of methane, which is a very potent greenhouse gas, leading to global warming. According to IPCC, ∼10% of global greenhouse gas emissions are attributed to food waste (IPCC Citation2019). Besides, the food wasted along the supply chain financially affects all the stakeholders of the supply chain including customers, which has been estimated to be around US$1 trillion (FAO Citation2019c). Sustainable consumption and production are one of the most pressing challenges in this sector and is in line with UN SDG number 12- Responsible Consumption and Production. Mitigation of food waste could play a pivotal role in strengthening the fortunes of global food industries and thereby national economies around the world.
Food waste is generally being ignored because the associated expenses are often underrated. Major firms of the food industry usually keep their waste figures confidential because of data sensitivity (Parliament Publications, UK Citation2017). Raising awareness will play a crucial role in drawing the attention of the food industry towards the multi-dimensional consequences of food waste. It will improve the financial return to farmers, who gets the least profit within the supply chain. Simultaneously, it will address the global issues of food security, environmental implications, and financial crisis of food industries and will also help to achieve sustainable consumption and production.
Beef products are deemed as a rich source of protein globally and it contributes to 24% of the entire meat production (Boucher et al. Citation2012). Livestock production generates employment for 1.3 billion people, accounts for 40% of net agricultural GDP (Steinfeld et al. Citation2006). It is estimated that livestock production covers 70% of aggregate agricultural land worldwide (Steinfeld et al. Citation2006). Beef products are also the most resource-intensive in the entire food spectrum (Navarrete-Molina et al. Citation2019). In the UK, ∼14,572 tonnes of waste is created in the product flow of beef from farm to retailer (Whitehead et al. Citation2011). Keeping the same in mind, this study is focused on waste minimisation in the beef supply chain and addresses the following research questions:
RQ1. What are the root causes of waste generated within the product flow of beef products from farm, abattoir, and processor to a retailer?
RQ2. How multi-agent architecture will assist in mitigating root causes of waste generated in the beef supply chain?
The extant studies have focussed on waste minimisation at a given segment (retailer, abattoir, processor, etc.) within the beef supply chain. However, this study has adopted a holistic approach and utilised the current reality tree method to identify the root causes of waste generated in the entire beef supply chain viz. farm, abattoir, processor, and retailer. A multi-agent architecture comprising of five autonomous agents is proposed to improve the vertical coordination across the beef supply chain and thereby mitigate these root causes to improve operational efficiency. It will assist all stakeholders in the beef supply chain viz. farmers, abattoir, processors, logistics, and retailers to make informed decisions to optimise the product flow with minimal losses thereby nurturing production, distribution efficiencies, and resilience towards variable demand by establishing a feedback mechanism. The use of a multi-agent framework will raise the profit margins for all stakeholders and also facilitate better utilisation of resources.
The organisation of this paper is as follows: Section Literature review consists of literature review of the work done in waste minimisation in the food supply chain till date. Section Dynamics of beef supply chain describes dynamics of the beef supply chain. Section Research method describes the research method. Section Results contains results of the study followed by multi-agent architecture for waste minimisation in section Multi-agent framework for waste minimisation. The findings of the study are scrutinised in discussion in section Discussion. Finally, the conclusion of the study is being provided in section Conclusion.
2. Literature review
Food waste is often attributed to overproduction. It was revealed that ∼50% excess of food production is needed to offset the unanticipated losses and to address food security (Raak et al. Citation2017). The root causes of excess food produce originated from the trade demands viz. the probability of deviation from approved food quality standards, punitive contractual sanctions, and return of produce obligations thereby raising the volume of fresh produce (Parfitt et al. Citation2010). The inefficiencies in demand forecasting are driven by the lack of real-time information sharing within the entities of the food chain (Mena et al. Citation2014). Food producers employ historical sales figures in conjunction with their experience to predict the volume of produce needed (Raak et al. Citation2017). Retailers on the other hand have to find an optimum trade-off amongst inflated shelves to boost customer confidence and unsold food produce (Ettouzani et al. Citation2012)
The contribution of beef products to the overarching issue of food waste is well documented in extant literature (Alexander et al. Citation2017). Although fresh vegetables are the hotspots of greenhouse gas emissions pertaining to their volumes rendered to landfills, nevertheless, beef has the highest carbon footprint (amongst all agri-food products) when comparisons are drawn on a per kilogram basis (Beretta et al. Citation2017). The raising of cattle also leads to the highest rates of losses in energy and protein (Alexander et al. Citation2017). The data corresponding to waste generated within the supply chains is not even properly captured in the developing nations, some of them are the highest producer/exporter of beef products (Spang et al. Citation2019).
Like conventional manufacturing and retailing sectors, information sharing is of utmost importance within the beef supply chain (Ding et al. Citation2014). For instance, the flow of information amongst producers and processers with respect to the weight of cattle, its conformation, etc. needs to be precise and timely (Ding et al. Citation2014). The precise and timely information on beef cuts from processors to wholesalers will aid the economic sustainability thereby empowering wholesalers to raise their market share in domestic and overseas markets (Ding et al. Citation2014). Also, efficient exchange of information across the beef supply chain will assist in reducing the carbon footprint (Singh et al. Citation2015). It also aids abattoir and processor in optimum supplier selection of beef cattle (Singh et al. Citation2018). Hornibrook & Fearne (Citation2003) found out that vertical coordination strategy has been successful to manage perceived risk associated with fresh beef for catering customers. Park et al. (Citation2011) concluded that the US beef supply chain has become more coordinated with better information flow.
The concept of waste minimisation in the beef supply chain has drawn the attention of researchers in the last two decades. Simons & Zokaei (Citation2005) have applied the lean approach to the cutting room of the red meat industry. Francis et al. (Citation2008) have drawn attention towards waste elimination opportunities in the UK supply chain at the producer and processor level by comparing with Argentine counterparts. Mishra and Singh (Citation2018) employed Twitter analytics downstream of the beef supply chain to formulate waste minimisation strategies to mitigate root causes of waste upstream of the supply chain. Singh et al. (Citation2018) have followed a social media data analytics approach for the identification of discrepancies/inconsistencies within the food sector to aid decision-makers in the food supply chain regarding problems in product flow and quality of edibles. Mishra et al. (Citation2017) have employed interpretative structural modelling and fuzzy MICMAC approaches for developing a consumer-centric beef supply chain to mitigate avoidable waste.
It is evident that the existing work in the literature on waste minimisation in the food supply chain is limited to either one of the segments or interfaces of two segments within a food chain. However, this study bridges the gap in the literature by employing the current reality tree method to identify the root causes of waste in the entire beef supply chain from farm to retailer. Furthermore, considering the utmost importance of information sharing within the beef supply chain, a multi-agent architecture is proposed to improve the coordination (information flow in real-time) amongst various stakeholders of the beef supply chain to mitigate the identified root causes of waste. The next section consists of the dynamics of the beef supply chain.
3. Dynamics of beef supply chain
Beef is the most resource-intensive agri-food product. It involves multiple stages to reach from farm to fork, which is described in the following subsection.
3.1. Product flow in beef supply chain
The beef supply chain is complex in nature. It includes all the stakeholders from farmer to retailer. shows an illustrative diagram of the beef supply chain. The beef farms are of different sizes and contain a varying number of cattle. The farmer raises the cattle in beef farms to the finishing age, which could be anywhere between 3 and 30 months. The finishing age depends on the breed of cattle, gender, and demand in the market (local and abroad). The cattle are sent to abattoir and processor, when they reach their finishing age, by deploying logistics. The abattoir slaughters the cattle and slices them into primals. The processor then processes these primals into human consumable products like steak, joint, burger, and meatball, etc. Then, packing and labelling of these fine products are completed and sent to retailers’ warehouses, where they are sorted and dispatched to corresponding retails stores to be procured by customers.
In an ideal scenario, the product flow within the beef supply chain appears to be devoid of any bottlenecks as discussed above. However, in reality, this process is complex given the miscoordination within various stakeholders in the supply chain. For instance, if the diet of cattle is based on grain instead of grass (as required by the retailer), the shelf life derived from the meat will be shorter. Also, carcase imbalance is another major factor in generating product waste. For example, the demand at the retailer end may be higher for hindquarter products (steak, joints, etc.) as compared to forequarter products (mince, burger, etc.). The disproportionate inflated demand for hindquarter products will render the low demand forequarter products as waste. The poor vertical coordination in terms of information flow (regarding demand) from downstream to upstream of the supply chain will generate disparity within supply and demand thereby creating waste. The detailed description of types of waste generated by the interaction of stakeholders within the beef supply chain is discussed in section Results.
3.2. Waste in beef supply chain
OECD/Eurostat (Citation2005) have defined waste as, “Those products which are not the principal product for which the manufacturer has no further utilisation for their own manufacturing, processing or consuming and which they reject or aspire to reject or is needed to reject. Waste could be created while raw material extraction, their processing to end-consumer products, while consumption of these products and while any distinct human occupation.”
Waste is occurring in the beef supply chain at the premises of all stakeholders, which are farm, abattoir, processor, logistics, and retailer. There are various types of waste being generated across the beef supply chain. They can be divided into following categories:
Animal by-products
Product waste
Animal by-products are the non-edible secondary products or carcase obtained from animals. These by-products can be further classified into the following three subcategories:
Category 1 (High-risk by-products) – These have to be disposed of by either incinerating or processing in a government-approved plant. They consist of SRM (Specified Risk Material) like the brain, spinal cord, etc. Approximately, 12.1% of the total live weight of cattle is attributed to category 1 animal by-products (Whitehead et al. Citation2011).
Category 2 (medium risk by-products) – These can be disposed of by either composting or using in biogas production. They consist of deceased animals, digestive tract, and blood. Approximately, 1.9% of the total live weight of cattle is attributed to category 2 animal by-products (Whitehead et al. Citation2011).
Category 3 (Low-risk material) – These can be utilised for the production of pet food, chemical fertiliser, oleo chemicals, etc. Approximately, 19.2% of the total live weight of cattle is attributed to category 3 animal by-products (Whitehead et al. Citation2011).
Product waste- It is defined as the edible meat being lost in the product flow along the beef supply chain due to poor process control and operational issues. It could be due to contamination, over-trimming, beef products going past their shelf life without getting sold (Whitehead et al. Citation2011). Product waste is also rendered as animal by-products mentioned above and is not fit for consumption by humans. This study focuses on the product waste occurring in the beef supply chain.
Animal by-products and product waste are related to each other. Product waste is generated as a result of human error and inefficient management practices across the beef supply chain. The product waste is then treated like animal by-products as per the hazards associated with them. For instance, the meat which is dropped on the floor during butchery and boning operations is a type of product waste and is rendered as category 3 waste. However, if the level of contamination is high then it is disposed of as category 2 waste. In the next section, with the help of the current reality tree method, we have classified different types of waste generated and their corresponding root causes.
4. Research method
This study aims to uncover the root causes of generating waste in the beef supply chain, identify the causal relationship amongst them, and suggest preventive measures to address them. Keeping this in mind, the data collection is conducted via a workshop involving twenty practitioners of the beef industry as discussed below.
4.1. Data collection
The beef supply chain has to be resilient to the challenges posed by temperature abuse, short shelf life, fluctuating demand from farm to fork. Hence, the research method should be overarching to encapsulate these aspects. The scant literature on waste minimisation in the beef supply chain and the causal relationships underpinning it suggests the employment of exploratory research. Keeping this in mind, primary data collection was performed via a workshop.
The waste generated at different segments of a beef supply chain is interconnected to different stakeholders (farm, abattoir, processor, logistics, retailer, etc.). Therefore, in order to establish causality of waste generated pertaining to the interdependence of stakeholders, the workshop provides an ideal setting of in-depth discussion and cross-organisation collaboration to address the waste hotspots. These inter-organisation discussion within the workshop in comparison to interviewing stakeholders individually raises the potency of current reality tree generated later in the study to identify the root cause of waste within the beef supply chain.
The participants of the workshop were twenty practitioners from the beef industry: five representatives each from beef farmers, abattoirs & processors, retailers, and logistics thereby mapping the entire beef supply chain. The farmers invited were having at least fifteen years of work experience in small, medium, and large size beef farms. The participants representing from abattoir and processor were agriculture managers, product technologists, supply chain manager, customer relationship manager, QA (Quality Assurance) supervisor each having experience of five to fifteen years. The representatives of the retailer were supply chain manager, supplier relationship manager, customer, and trading manager, warehouse manager, and store manager each having experience of eight to thirteen years. The representative of logistics was logistics manager, cattle truck driver, transport coordinator, stock controller, and junior operations executive each having experience of seven to fifteen years. The roles, responsibilities, and other characteristics of all the workshop participants have been incorporated in . These twenty practitioners of the beef industry were chosen owing to their vast experience and their close relevance to the aim of this study. Also, their roles and responsibilities within their respective organisations assisted in critically identifying the hotspots of waste within the beef supply chain.
Table 1. Characteristics of the workshop participants.
An intra-group face-to-face brainstorming session was conducted for 2.5 hours in which, the representatives of a particular stakeholder reflected on different kinds of waste generated at their respective premises and tried to identify their root causes. These preliminary findings generated by all stakeholders were amalgamated to capture the various kind of waste generated overall in the beef supply chain and their potential root causes. Based on these, a group discussion of 2 hours was conducted amongst all the industrial practitioners present to further scrutinise these preliminary findings to capture the waste generated at the premises of a stakeholder (for example, retailer) due to the inefficiency of another stakeholder (for example, farmer). Given the segregated nature of the supply chain and inefficient information sharing, the stakeholders were not able to visualise their interdependence on each other with respect to waste minimisation. These intermediate findings were then further investigated with the experiential learnings and discussion among the participants for an hour to map the aggregate waste generated within the beef supply chain with their corresponding root causes. The outcome of the mapping exercise was transcribed and recorded for data analysis as mentioned in the next subsection.
4.2. Data analysis
This study is focussed on tracing the root causes of waste generated in the beef supply chain, their causal relationships, and corresponding preventive measures to address them. Hence, the qualitative approach is followed given the context of the study. Firstly, data collected was analysed, coded, and transferred to a specific template. Then, significant information of stakeholder’s firms was transformed to tables to aid in comparing and contrasting the information provided by different firms.
The identification of root causes of waste generated in beef supply chain was done via causal maps (Jenkins and Johnson Citation1997; Fiol and Huff Citation1992). In the literature, casual maps have been used for various purposes like in developing cause and effect diagrams (Ishikawa Citation1990) and interrelation diagrams (Doggett Citation2005) for quality management. Kaplan and Norton (Citation2004) have used strategy maps to demonstrate the long-term strategy of a firm.
The causal relationship between components of a framework are represented by graphs, where nodes denote problems, concepts or ideas and the unidirectional arcs connecting these nodes denote the causal relationship between them (Scavarda et al. Citation2006). There are various kinds of causal maps available for root cause identification (Doggett Citation2005). However, the current reality tree (CRT) has been used in this article, considering its clear logical flow and capability to identify distinct and logical root causes (Walker and Cox Citation2006; Doggett Citation2005). CRT has been actively employed in the domain of operations and supply chain management. Mena et al. (Citation2011) have employed CRT to identify the root causes of waste generated at the supplier-retailer interface in the UK and Spain. Chou et al. (Citation2012) have raised the inventory management of an aerospace firm by eradicating the contradictory inventory management practices of various departments. Rahman (Citation2002) have utilised CRT to unravel crucial factors to raise the performance of a supply chain and also investigated causal linkages amongst these factors. The creation of CRT begins with finding out the surface issues or unwanted consequences (Walker and Cox Citation2006). It utilises three unique symbols: nodes represent unwanted consequences, arcs represent causal relationship and oval denotes the ‘AND’ logical function, which means that two or more causes are needed to generate an effect. The unwanted consequences are connected via an if-then logic. The process creates a graph or tree having the final issues or problems at the top and the root causes can be found out at the bottom.
In this article, CRT for the beef supply chain is being created. The top of the tree denotes the generation of waste across the beef supply chain, which is the major focus of this research. The central part of the tree denotes the intermediate causes of waste generation. The root causes of waste generated in the entire beef supply chain are located at the bottom of the tree, which will be discussed in detail in the following section.
5. Results
The results of the study are discussed in this section. The major root causes of waste in the entire beef supply chain (from farm to retailer) have been identified using the Current Reality Tree as shown in . Some of the root causes are interconnected and could be broadly clustered into the following categories:
Poor vertical co-ordination (PVC)- The waste generated within the beef supply chain due to lack of information sharing creates a bottleneck for the product flow from upstream to downstream of the supply chain. For instance, delivery of an incorrect quantity of products by abattoir and processor to retailers.
Operational inefficiency (OI)- This category of waste is generated pertaining to poor process controls by stakeholders of the beef supply chain. For instance, failure of cold chain management within retailer store.
Different stakeholders are generating different kinds of waste at their premises (as identified by the current reality tree). These wastes have been linked to the aforementioned clustered categories and mentioned as following:
Farm – The main root cause of waste occurring at the farm end is the absence of fresh grass in the diet of cattle (OI). So, they are deficient in vitamin E. Hence, the meat derived from them have a shorter shelf life. Also, lack of cattle management leads to cattle not adhering to the weight and conformation specifications of a retailer, when they reach the finishing age (OI). Lack of animal welfare at beef farms might lead to cattle getting an infection or being physically injured, which might result in rejection by the abattoir.
Abattoir and Processor – The inefficiency in butchery and boning operations generates waste at the abattoir and processor. A significant amount of edible beef is lost because of over-trimming by the unskilled workforce (OI). Lack of maintenance of machines can lead to stoppage of line and thereby loss of product stuck within it (OI). Edible beef products fall over the floor because of incompetency and inefficiency in butchery and boning operations (OI). The lack of compliance of butchery and boning operations with takt time grounded on the forecasted demand of retailer leads to misalignment of supply and demand (OI & PVC). Butchery and boning operations get slowed down due to a violation of line balancing principles (OI). Over maturing of carcase in maturation park shortens the shelf life of beef products (OI). The absence of periodic changeover of a set of knives leads to slow operations (OI). Butchery and boning operations being performed against gravity results in lower productivity and exhaustion of abattoir staff (OI). Excess of contact with metallic blades leads to beef products being discarded in metal detection test (OI). Beef products get contaminated due to non-adherence to cleaning, packing regimes, and exposure to temperature abuse (OI).
Retailer - The main root cause of waste occurring at retailer’s warehouses and stores is due to lack of coordination (product, information, and finance) between abattoir, processor, and retailer leading to loss of beef (PVC). Inefficient cold chain management leads to temperature abuse of beef products (OI). Inflated orders placed by retailer store pertaining to higher availability of products overlooks the consequent potential waste (OI). Inappropriate stacking and shelving procedures at retailer store result in beef products going past their shelf life without getting sold (OI). Short-sighted promotion management by retailers leads to cannibalisation of products thereby generating waste (OI). Negligence of dedicated waste management human resources to frame the efficient waste management policy and their implementation leads to avoidable waste occurring at the retailer’s distribution centre and retail store (OI). Adoption of incorrect packaging results in shorter shelf life (OI). For example, employing modified atmosphere packaging (MAP) instead of vacuum skin packs (VSP) shortens the shelf life.
Logistics- Logistics assists in the shipment of cattle and edible beef products between various stakeholders of the supply chain. They also contribute in generating waste like their counterparts. The major root cause of waste generated by logistics is inefficient cold chain management in logistics vehicles leading to temperature abuse of beef products (OI). Delayed delivery of beef products to retailers shortens the shelf life of beef products available for sale (OI). Improper stacking of beef products raises the probability of damage (OI). Employing cheaper transport channels taking full truckload pertaining to cost savings makes beef products more vulnerable to damage (OI). Moreover, they also follow longer routes (to avoid tolls, etc.) thereby shortening the shelf life of beef products available for sale. Cattle get injured or stressed during transportation from farm to abattoir due to non-compliance with legal space allowance requirements.
6. Multi-agent framework for waste minimisation
Supply chain management incorporates numerous components/stakeholders interacting amongst each other with varying or potentially contradictory requirements. Therefore, it provides an ideal scenario for agent-based formalisations, where these components in the supply chain are modelled in the form of autonomous agents to mitigate complex issues (Baryannis et al. Citation2019). Multi-agent architecture, which is a key branch of artificial intelligence has been widely employed in operations and supply chain management. Chan and Chan (Citation2006) have utilised multi-agent architecture for inventory management to lower the expenditure and raise fill rate. Kwon, Im, and Lee (Citation2007) have employed multi-agent systems (MAS) to address coordination issues within supply chain partners pertaining to uncertainty in demand and supply. Zarandi, Pourakbar, and Turksen (Citation2008) have applied MAS to mitigate the bullwhip effect and its financial consequences within a multi-stage supply chain. Mishra et al. (Citation2016) have proposed a self-reactive cloud-based multi-agent framework for mitigating internal and external issues within the distributed manufacturing environment. Kumari et al. (Citation2015) have incorporated MAS to facilitate outsourcing within SMEs manufacturing supply chain. Mishra et al. (Citation2014) have employed MAS to raise the efficiency of the lamb supply chain and to make it consumer-centric. All of these studies have selected multi-agent architecture over mathematical optimisation given its innate ability to facilitate collaboration and conciliation amongst various stakeholders of the supply chain with comparatively minimal computational power needed (Baryannis et al. Citation2019).
Keeping above in mind, in this study, a multi-agent framework spanning the entire beef supply chain is proposed. The proposed multi-agent framework consists of five autonomous agents, which will communicate among themselves and aid in waste minimisation in the beef supply chain. The schematic diagram of this scheme is depicted in . These agents will capture information of product flow at different stages of the supply chain, which will be centrally stored in a knowledge base agent. These agents along with their responsibilities have been described below:
6.1. Beef husbandry agent
This agent focuses on the upstream (beef farms) of the supply chain. Its function is to do the record-keeping of cattle feed (grass, silage, dry feed), method of raising of cattle (in house, free grazing or barn reared). The information regarding weight, fat, and conformation specifications (based on EUROP classification) of cattle is also captured. Moreover, beef husbandry agent regularly monitors the wellbeing of cattle, for instance, hygiene, disease prevention, traceability issues, and farm assurance. The captured information is shared with all other agents and is stored in a knowledge base agent.
6.2. Abattoir optimisation agent
The abattoir optimisation agent is active at the abattoir and processor end of the supply chain. It keeps the track of floor waste generated in butchering & boning operations at each workstation. It captures the detailed information of beef products (with respect to SKU), which are discarded at quality cheques pertaining to over contact with metallic blades, contamination, temperature abuse, poor packaging & labelling. It also measures the performance of butchery & boning operations against the takt time (calculated based on the forecasted demand of the retailer). The discrepancies in the performance achieved on an hourly basis are recorded. It also records the beef products becoming inedible due to machine failures occurring due to maintenance issues. The recorded information is stored in a knowledge base agent.
6.3. Efficient retailing agent
This agent is based downstream of the supply chain (retail warehouses and retail stores). It monitors the coordination (information, product, and monetary flow) between abattoir, processor, and retailer. It records the waste generated due to over delivery and under-delivery of beef products by abattoir and processor to a retailer. An efficient retailing agent also captures the data corresponding to beef products becoming inedible due to temperature abuse or failure of cold chains at their warehouse and retail stores. It also stores the information of beef products, which goes past their shelf life prior to getting sold. The information of consumer complaints regarding beef products is recorded and this information is fed back to the abattoir optimising agent to identify its root causes. This agent also observes the impact of promotion on sales of corresponding products & similar products in the segment to identify the cannibalisation phenomenon. The information corresponding to the packaging utilised (MAP, VSP) for different beef products is also captured. An efficient retailing agent sends all information to the Knowledge base agent.
6.4. Logistics agent
Logistics agent spans the entire supply chain. This agent does the record-keeping of the cattle getting injured or stressed during transportation from farm to abattoir. It also captures the information on beef products going to waste due to temperature abuse (failure in the cold chain in lorries). The beef products damaged due to mishandling (with reasons- improper stacking, overloading, etc.) whilst transit is also recorded. It also keeps track of all the late deliveries made (and the navigation route followed) within the supply chain with corresponding reasons. All the captured information is shared with the knowledge base agent.
6.5. Knowledge base agent
Knowledge base agent is like the nervous system of this multiagent framework. It stores all the information and data sent from other agents and monitors their operations. The information received will be vast in scale and varying in formats. There are multitudes of tools employed for processing this gigantic data such as association rule mining, genetic algorithms, and machine learning. Literature suggests that there is not a particular tool, which could be employed universally pertaining to varying scale and format of datasets managed by knowledge base agent. Therefore, it employs an algorithm portfolio to follow a case-by-case approach to choose the best tool to manage a given dataset depending on its characteristics. The algorithm portfolio is capable to learn from its past experiences and is able to update itself both online and offline (Kumari et al. Citation2015). The track record of all successful and unsuccessful decisions made in the past is also maintained by knowledge base agent. This information is used to nurture other agents to prevent them from committing similar mistakes again.
6.6. Communication channel
The efficacy of a multi-agent framework is dependent on the rapid and accurate interaction amongst agents. The agents employ signals for communicating among themselves. The receiving agent computes the necessary action that needs to be taken by decoding these signals. Numerous languages have been developed by developers for reliable communication amongst agents such as knowledge query manipulation language (KQML), agent communication language, etc. (Mishra et al. Citation2014). Nevertheless, one of the most sophisticated and widely recognised languages is a multi-agent logic language for encoding teamwork (MALLET) (Mishra et al. Citation2016). It was developed with the objective to strengthen team-oriented programming. MALLET assists in streamlining the information flow within the framework and precise encoding of data, which could be declarative or procedural in nature (Kumari et al. Citation2015). MALLET permits the execution of various levels of intelligence to mimic the characteristics of human teamwork (Fan et al. Citation2005). Also, teamwork information (team process and the structure) should be recorded in a manner, which reduces its complexity to interpret at the team level. This phenomenon could only be achieved via an advanced language like MALLET instead of conventional agent communication language such as JACK Teams (Winikoff Citation2005). MALLET (by using sequential and iterative processes) will be employed in the proposed beef supply chain to boost communication within the agents. The interpreter for MALLET is a collaborative agent for simulating teamwork (CAST) as shown in Figure 5.
The salient features of the communication channel are summarised as following:
Interpretability – The communication language should be simple and less resource-intensive in terms of decoding by agents.
Precision – It should be accurate, clear and highly expressive.
Multiple usability- It should have the attribute of multiple usability so that the stored data could be accessed numerous times thereby achieving savings in resources.
Prior to initiating communication, agents define their abilities, tasks and workplan. Thereafter, they assimilate their tasks with agents as per their norms. The receiving agent sends its pre-requisites such as information and knowledge required to following agent. This interaction could be classed as parallel (PAR), iterative (WHILE, FOR), choice specific (CHOICE), sequential (SEQ) or conditional (IF). The agent is able to conclude its workplan only when its pre-requisites are accomplished. However, if an agent could not achieve their pre-requisites, then other agents assists it by active cooperation amongst themselves.
7. Discussion
The analysis of the root causes map shown in suggested that the root causes of waste in the beef supply chain can be broadly classified into two groups: poor vertical co-ordination (PVC) and operational issues inefficiency (OI). The former is driven by poor information sharing within stakeholders of the beef supply chain thereby generating disparity amongst supply and demand. The latter reflects the inconsistencies and inefficiencies within the management practices across the stakeholders of the beef supply chain. These waste categories are mitigated by novel multi-agent architecture as discussed below by various stakeholders:
Farm- During the workshop, it was identified that beef farmers lack awareness in terms of modern practices of raising cattle. They should be given appropriate training in terms of proper diet of cattle, animal welfare, and overall animal husbandry. The beef husbandry agent (BFA) will inform the farmers that cattle should be fed on fresh grass, which is rich in Vitamin E. It will help to improve the shelf life of beef derived from them (Warren et al. Citation2008). It was revealed that animals get rejected because of health reasons. A regular health check-up facilitated by BFA will prevent the rejection of cattle owing to infection. If a health issue is diagnosed, it can be timely cured to curb its unfavourable consequences. The beef farmers will be informed by BFA that cattle who are on medication should not be sent to abattoir as they will get rejected Kühl et al. (Citation2020). There should be ample time given to the sick cattle to recover and then sent to abattoir and processor. Similarly, their weight and conformation specifications will be observed regularly by BFA so that appropriate alterations in their diet could be suggested to farmers. It will help to meet the weight and conformation specifications of abattoir and processor when the cattle reach their finishing age.
Abattoir and Processor end – The representatives of abattoirs and processors suggested that the lack of coordination between them and retailers is the major root cause of waste at their premises. It should be improved by employing abattoir optimisation agent (AOA) and thereby information sharing between these two stakeholders will increase. It will help abattoir and processor to forecast their demand more precisely, thereby curbing overproduction and loss of revenue in the event of underproduction (Ding et al. Citation2014). Moreover, inefficiencies in the butchery and boning operations were identified. Certain good practices with the assistance of AOA should be adopted in butchery and boning operations like adhering to takt time principle, line balancing, etc. It will improve their efficiency and reduce waste (Francis et al. Citation2008). The working staff should be given appropriate training arranged by AOA so that there is no loss of beef because of over-trimming and meat is handled carefully so that it doesn’t fall on the floor (Mena et al. Citation2014). They should be made aware of the hygiene and temperature requirements of beef products. A provision should be made for a reliable auxiliary power supply in the event of power failure so that the cold chain is maintained and there is no temperature abuse of beef products (Singh et al. Citation2018). The knives used by the working staff should be changed periodically to avoid the slowing down of operations. Unnecessary contact of beef products with metallic blades or knives should be avoided to prevent rejection in metal detector tests. Finally, regular maintenance of machines should be performed by AOA to lower the frequency of machine breakdowns (Mishra and Singh Citation2018). These practices will collectively help to mitigate the root causes of waste occurring at abattoir and processor end.
Retailer –CRT revealed that majority of waste is occurring at retailer end because of a lack of coordination between abattoir, processor, and retailer. Retailer will be able to share their real-time sales information with the abattoir and processor via an efficient retailing agent (ERA) so that they can do accurate forecasting at their end. It will reduce the phenomenon of over and under-delivery of beef products to them (Singh et al. Citation2015). The retailer will be guided by ERA to employ the latest forecasting techniques and updated data mining framework to lower the error in forecasting at their premises. It was observed that retailer was still using the conventional modified atmosphere ackaging (MAP) instead of the latest Vacuum Skin Packaging (VSP). Hence, the retailers should adopt VSP (for premium beef cuts) to avoid waste and improve their revenue (Mishra et al. Citation2017). They should make an appropriate trade-off between the availability of products and waste generated with the assistance of ERA. The beef products in a retail store should only be ordered based on demand or sales of previous stock (Kaipia et al. Citation2013). It will help to reduce the unnecessary overstocking of beef products on retails shelves, which are left unsold. The managers of retailer told that stacking and shelving procedures are not being followed properly. The staff in a retail stores should be given proper training to do so and must be regularly supervised by the store manager or their supervisor (Mckinnon et al. Citation2007). There should be efficient cold chain management both in-retailer warehouses and stores so that there is no loss of beef products because of temperature abuse (Aung and Chang Citation2014). It was observed that promotions of a certain product were leading to waste of anther beef product. The retailer must closely study the behaviour of customers via ERA and employ a clear strategy for promotions so that it does not lead to the generation of waste. The CRT analysis pointed out that the waste occurring in the whole supply chain is not being properly quantified and there is no workforce to address it. Recruitment of a dedicated team for waste minimisation can help in quantifying waste, which helps in identifying the hotspots of waste in the retailer supply chain (Mena et al. Citation2014). These hotspots can then be mitigated to avoid waste.
Logistics- Logistics plays a crucial role throughout the supply chain. It was revealed that the majority of losses were occurring because of delayed delivery of beef products from abattoir and processor to the retailer. The retailer was receiving some products below their threshold shelf life. Hence, the retailer was rejecting them (Milgate Citation2001). The retailer must employ a logistics agent (LA) to hire an efficient logistics firm, which will have a reliable lead time. The underperforming logistics company must be penalised for the delays so their performance keeps up to the mark. LA should assist in utilisation of reliable technology for refrigeration in logistics vehicles to avoid temperature abuse of beef products (Wu and Hsiao Citation2021). It was observed that they were taking full truckload and following the longer route (to avoid toll tax, etc.) to save expenses. These practises should be avoided and an optimum load optimisation procedure must be followed (Singh et al. Citation2015). A safe and quick transport route prescribed by LA should be followed to avoid unnecessary delays in the delivery of products. The practitioners noticed during their site visits to logistics firms that the beef products were not stacked properly, which was causing damage to products. The logistics personnel should be trained regarding the appropriate stacking procedures so that product damage is avoided (Singh et al. Citation2018). It was also revealed that cattle were found to be stressed and injured when transported from beef farms to abattoir and processors. The logistics vehicle must follow the guidelines of the government and should not overcrowd their vehicle with cattle. There should be enough space allowance given to each individual cattle and extra care should be taken in loading and unloading of cattle in the logistic vehicle (González et al. Citation2012).
During the analysis, it was found that some root causes of waste were associated with a particular stakeholder of the beef supply chain. For each stakeholder, the root causes and preventive measures were suggested above in detail and they are also summarised in . It was revealed in the workshop that some beef farms were generating more waste as compared to others. Therefore, the employment of a multi-agent framework spanning the entire beef supply chain provides an opportunity for high waste generating farms to learn the good practices form low waste generating beef farms. Also, some root causes of waste were dependent on more than one stakeholder. There is a need of strong vertical coordination in the beef supply chain to address them. In order to achieve this, a holistic approach is proposed (multi-agent architecture) to bring all stakeholders on one platform and exchange information thereby minimising the waste in the beef supply chain.
Table 2. Root causes of waste in beef supply chain and corresponding preventive measures.
8. Conclusion
This paper is focussed on the exploration of waste occurring in the beef supply chain, predominantly highlighting their root causes to improve their operational efficiency and reduce carbon footprint. A workshop involving twenty practitioners of the beef industry was conducted for primary data collection. The collected data were transcribed, coded and a current reality tree (CRT) was developed identifying the root causes of waste in the beef supply chain generated by all stakeholders. These root causes of waste have been broadly clustered into two categories- poor vertical co-ordination (PVC) and operational inefficiency (OI). PVC was generated by lack of information sharing whereas OI was generated by poor process controls adopted by the stakeholders. A multi-agent architecture comprising of five autonomous agents was proposed to mitigate these waste categories. The artificial intelligence embedded in them facilitates strong vertical coordination and improves the information exchanged amongst the stakeholders of the supply chain. Hence, demand forecasting, waste mitigation, and promotions management will improve. The proposed multi-agent architecture within the beef supply chain will support all the stakeholders in optimal decision-making to mitigate all the management root causes generated by operational inefficiencies. The qualitative research methodology has been followed in this article. It helped to unravel the root causes of waste in the beef supply chain. A suggestive framework involving multi-agent architecture was proposed to mitigate the root causes of waste. Although this study was qualitative in nature, it could play a crucial role in diverting the attention of academic researchers and industrial practitioners to waste occurring in the beef supply chain.
8.1. Research implications
The existing studies in the literature are limited to waste minimisation at a given segment or interface of two segments in the food (beef, red meat supply chain, etc.). However, this study bridges the gap by adopting a holistic approach via spanning the entire beef supply chain to mitigate root causes throughout the supply chain from farm to retailer. The proposed framework assists in identifying the waste hotspots and the linkages amongst them. Further, this is a pioneering study to employ multi-agent architecture within the domain of the food supply chain to address the waste generated in the product flow from upstream to downstream.
8.2. Practice implications
The proposed framework is developed by the inputs of twenty practitioners from different segments of the beef industry. Given the waste generated in the supply chain is interrelated with different stakeholders, the proposed framework improves the data visibility of product flow from farm to retailer, monitoring of waste generated and the underpinning root causes in real-time thereby assisting various segments in the beef industry to adopt the corresponding suggested measures whenever a given hotspot generating waste is identified by the multi-agents. Apart from raising vertical coordination within the beef supply chain, it also improves the horizontal co-ordination in the beef supply chain. For instance, small size farmers could be benefitted from good practices adopted by bigger farms for waste minimisation. This study is insightful for the government bodies and policymakers for waste minimisation within the food chain thereby raising food security, cost efficiency, and environmental stewardship.
8.3. Limitations
This research does not incorporate the waste generated at consumer households. Also, the waste generated at the warehouse of the retailer was not critically examined. In this study, twenty practitioners of the beef industry participated in the workshop for data collection and analysis, which could be extended in the future by wider participation to gain deeper insights into the root causes of waste generated at each segment of the beef supply chain. The proposed recommendations are suggestive in nature and may not apply to certain firms pertaining to their inter-organizational relationships, process control, work ethic, etc.
8.4. Future research
In the future, inter-disciplinary research can be carried out with veterinary sciences to uncover the factors affecting the shelf life of beef products such as the breed of cattle, method of raising cattle (inhouse or open grasslands). A deeper investigation could be performed to explore the best packaging to maximise the shelf life of beef products (steak, joint, etc.). In the future, real-world data could be collected at all segments of the beef supply chain to investigate the impact of the proposed multi-agent framework. There is scope to deduce the empirical relation amongst various waste categories identified in this study and thereby uncover additional driving factors generating waste within the beef supply chain. The efficacy of the proposed framework could also be investigated for other major issues within the food chain- optimising the supplier selection process, reducing the carbon footprint, and developing a consumer-centric supply chain. Finally, future studies could be concentrated on other products of the red meat industry like lamb, pork, and to other agri-food products such as fresh vegetables, dairy, poultry.
Disclosure statement
No potential conflict of interest was reported by the author(s).
Additional information
Notes on contributors
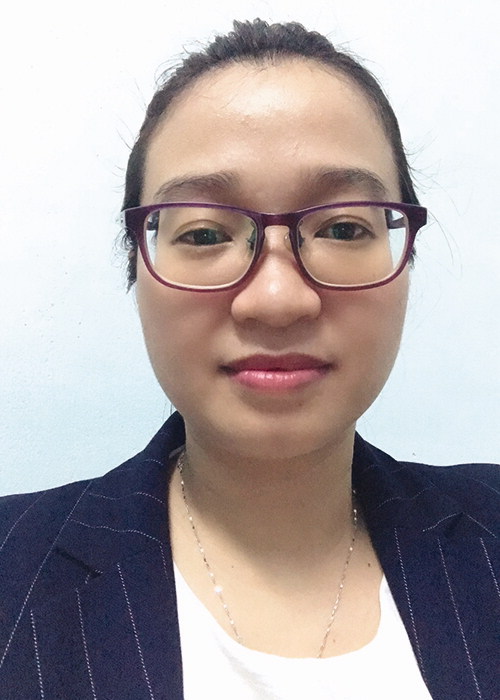
Ai Ha Thi Nguyen
Ai Ha Thi Nguyen is a Lecturer in Agronomy Faculty at Nong Lam University Ho Chi Minh city, Vietnam. She did M.Sc. and Bachelor’s degree of agriculture science from Nong Lam University. Her research is close to implementation side and she is also involved in consultancy work. She is involved in European commission funded project titled “Integration of Global and Local Agri-Food Supply Chains Towards Sustainable Food Security.”
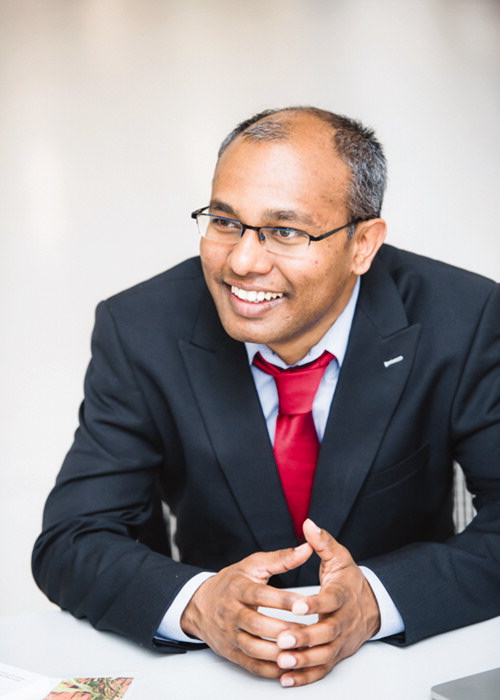
Akshit Singh
Akshit Singh is a Lecturer in Operations Management at University of Liverpool Management School. Prior to this, he was Lecturer in Management Sciences at Alliance Manchester Business School, The University of Manchester. At Manchester, he also worked as a Theme 2 (Resilient Supply Chains) Lead pertinent to N8AgriFood Project, which involves eight Russell Group Universities of Northern England for improving sustainability of food supply chains from farm to fork. He obtained his PhD from University of East Anglia, Norwich, which was funded by an Innovate UK project worth £2.7m. In his PhD, he successfully developed a decision support system for waste minimisation of Sainsbury’s beef supply chain. His research articles have been published in renowned journals such as Transportation Research Part E: Logistics and Transportation Review, International Journal of Production Economics, Annals of Operation Research and Robotics and Computer-Integrated Manufacturing Production Planning & Control.
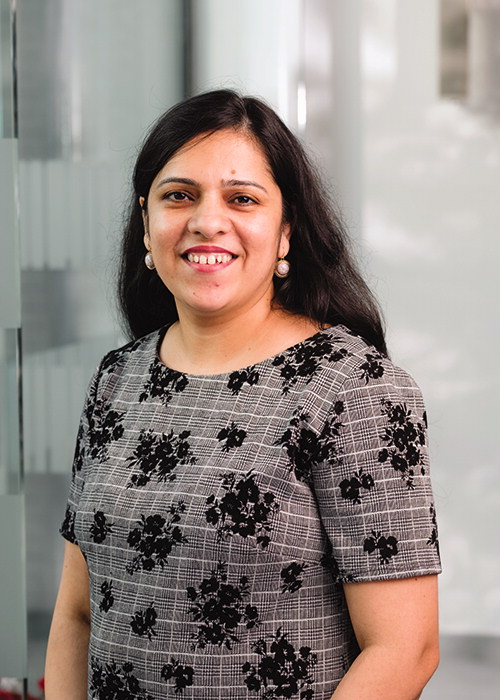
Sushma Kumari
Sushma Kumari is afull-time Lecturer at the University of Hull, UK. Prior to that, she worked in different international firms as HR executive. She has worked as PI in projects funded by AURA and Co-PI in EPSRC funded project. She has also worked as a research assistant on numerous projects funded by Biotechnology and Biological Sciences Research Council (BBSRC), British Academy (BA), Horizon 20-20, Innovate UK, Higher Education Academy (HEA). Her research articles have been published in various renowned journals of Operations Research and Operations & Supply Chain Management. Her research interest includes SMEs' supply chain, knowledge management, food supply chain and general supply chain area. She is supervising PhD candidates in the areas of sustainability, Logistics, and Supply Chain Management.
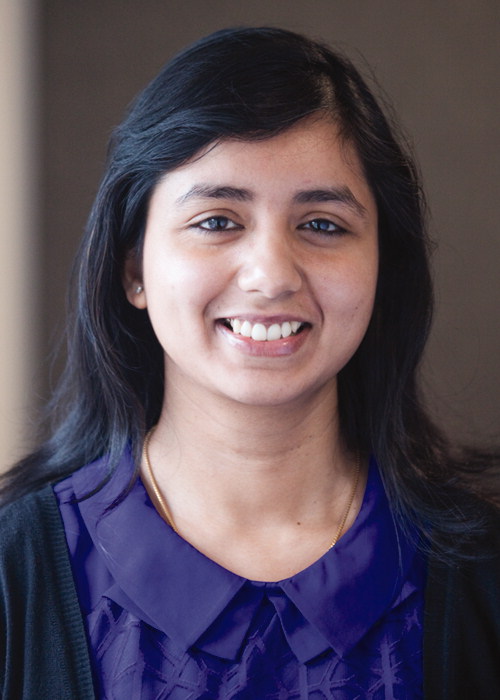
Sonal Choudhary
Sonal Choudhary is the Head of Operations Management and Decision Sciences Research Centre and a Senior Lecturer in Sustainable Management at the management school, University of Sheffield, UK. She specialises in sustainable operations and supply chain management research along with technological adoptions for enhancing the resilience and sustainability of food systems. She investigates how different types of interventions from multidiscipline and multi-stakeholders can help in designing a sustainable and circular agri-food value chain. She holds a PhD in Environmental Sustainability from the University of Sheffield and has double masters with Gold medal in Environmental Science. She has created a network of national and international stakeholders in food, energy and water industry that includes some of the big players, service providers, retailers, processors, SMEs, farmer organisations, NGOs, certification bodies and policy makers in the UK and beyond. She has published in leading management journals such as Production, Planning & Control, Annals of Operation Research, Technology Forecasting and Social Change, Journal of Environmental Management, Ecological economics, among others. She has led, worked and reviewed numerous projects funded by Science and Technology Facilities Council (STFC), Biotechnology & Biological Sciences Research Council (BBSRC), Economic and Social Research Council (ESRC), European Commission (FP7 & H2020), Marie Curie (ITN), British Academy, Newton Fund and Higher Education Funding Council for England (HEFCE).
References
- Alexander, P., C. Brown, A. Arneth, J. Finnigan, D. Moran, and M. D. A. Rounsevell. 2017. “Losses, Inefficiencies and Waste in the Global Food System.” Agricultural Systems 153: 190–200.
- Aung, M. M., and Y. S. Chang. 2014. “Temperature Management for the Quality Assurance of a Perishable Food Supply Chain.” Food Control 40: 198–207. doi:10.1016/j.foodcont.2013.11.016.
- Baryannis, G., S. Validi, S. Dani, and G. Antoniou. 2019. “Supply Chain Risk Management and Artificial Intelligence: State of the Art and Future Research Directions.” International Journal of Production Research 57 (7): 2179–2202. doi:10.1080/00207543.2018.1530476.
- Beretta, C., M. Stucki, and S. Hellweg. 2017. “Environmental Impacts and Hotspots of Food Losses: Value Chain Analysis of Swiss Food Consumption.” Environmental Science & Technology 51 (19): 11165–11173.
- Boucher, D., P. Elias, L. Goodmen, C. May- Tobin, K. Mulik, and S. Roquemore. 2012. Grade A choice? Solutions for Deforestation Free Meat. Accessed March 27, 2014. http://www.ucsusa.org/global_warming/solutions/stop-deforestation/solutions-for-deforestation-free-meat.html
- Chan, F. T., and H. K. Chan. 2006. “A Simulation Study with Quantity Flexibility in a Supply Chain Subjected to Uncertainties.” International Journal of Computer Integrated Manufacturing 19 (2): 148–160. doi:10.1080/09511920500324381.
- Chou, Y. C., C. H. Lu, and Y. Y. Tang. 2012. “Identifying Inventory Problems in the Aerospace Industry Using the Theory of Constraints.” International Journal of Production Research 50 (16): 4686–4698. doi:10.1080/00207543.2011.631598.
- Ding, M. J., F. Jie, K. A. Parton, and M. J. Matanda. 2014. “Relationships between Quality of Information Sharing and Supply Chain Food Quality in the Australian Beef Processing Industry.” The International Journal of Logistics Management. doi:10.1108/IJLM-07-2012-0057.
- Doggett, A. M. 2005. “Root Cause Analysis: A Framework for Tool Selection.” Quality Management Journal 12 (4): 34–45. doi:10.1080/10686967.2005.11919269.
- Ettouzani, Y., N. Yates, and C. Mena. 2012. “Examining Retail on Shelf Availability: Promotional Impact and a Call for Research.” International Journal of Physical Distribution & Logistics Management 42 (3): 213–243. doi:10.1108/09600031211225945.
- Fan, X., J. Yen, M. Miller, T. R. Ioerger, and R. Volz. 2005. “MALLET-a Multi-Agent Logic Language for Encoding Teamwork.” IEEE Transactions on Knowledge and Data Engineering 18 (1): 123–138.
- FAO. 2019a. Food Loss and Food Waste. Accessed 22 June 2020. http://www.fao.org/food-loss-and-food-waste/en/
- FAO. 2019b. Hunger and Food insecurity. Accessed 22 June 2020. http://www.fao.org/hunger/en/
- FAO. 2019c., Policy Support and Governance. Accessed 24 June 2020. http://www.fao.org/policy-support/policy-themes/food-loss-food-waste/en/
- Fiol, C. M., and A. S. Huff. 1992. “Maps for Managers: Where are we? Where do we Go from Here?” Journal of Management Studies 29 (3): 267–285. doi:10.1111/j.1467-6486.1992.tb00665.x.
- Francis, M., D. Simons, and M. Bourlakis. 2008. “Value Chain Analysis in the UK Beef Foodservice Sector.” Supply Chain Management: An International Journal 13 (1): 83–91. doi:10.1108/13598540810850346.
- González, L. A., K. S. Schwartzkopf-Genswein, M. Bryan, R. Silasi, and F. Brown. 2012. “Space Allowance during Commercial Long Distance Transport of Cattle in North America.” Journal of Animal Science 90 (10): 3618–3629. doi:10.2527/jas.2011-4771.
- Hornibrook, S. A., and A. Fearne. 2003. “Managing Perceived Risk as a Marketing Strategy for Beef in the UK Foodservice Industry”. International Food and Agribusiness Management Review 6 (3): 70–93.
- IPCC. 2019. IPCC Special Report on Climate Change, Desertification, Land Degradation, Sustainable Land Management, Food Security, and Greenhouse gas fluxes in Terrestrial Ecosystems. Accessed 24 June 2020. https://www.ipcc.ch/site/assets/uploads/2019/08/4.-SPM_Approved_Microsite_FINAL.pdf
- Ishikawa, K. 1990. Introduction to Quality Control. 3rd ed. California: 3A Corporation.
- Jenkins, Mark, and Gerry Johnson. 1997. “Entrepreneurial Intentions and Outcomes: A Comparative Causal Mapping Study.” Journal of Management Studies 34 (6): 895–920. doi:10.1111/1467-6486.00077.
- Kaipia, R., I. Dukovska‐Popovska, and L. Loikkanen. 2013. “Creating Sustainable Fresh Food Supply Chains through Waste Reduction.” International Journal of Physical Distribution & Logistics Management 43 (3): 262–276. doi:10.1108/IJPDLM-11-2011-0200.
- Kaplan, R. S., and D. P. Norton. 2004. “How Strategy Maps Frame an Organization's Objectives.” Financial Executive 20 (2): 40–45.
- Kühl, S., G. Busch, and M. Gauly. 2021. “How Should Beef Be Produced? Consumer Expectations and Views on Local Beef Production in South Tyrol (Italy).” British Food Journal 123 (4): 1578–1595. doi:10.1108/BFJ-07-2020-0571.
- Kumari, S., A. Singh, N. Mishra, and J. A. Garza-Reyes. 2015. “A Multi-Agent Architecture for Outsourcing SMEs Manufacturing Supply Chain.” Robotics and Computer-Integrated Manufacturing 36: 36–44. doi:10.1016/j.rcim.2014.12.009.
- Kwon, O., G. P. Im, and K. C. Lee. 2007. “MACE-SCM: A Multi-Agent and Case-Based Reasoning Collaboration Mechanism for Supply Chain Management under Supply and Demand Uncertainties.” Expert Systems with Applications 33 (3): 690–705. doi:10.1016/j.eswa.2006.06.015.
- McKinnon, A. C., D. Mendes, and M. Nababteh. 2007. “In-Store Logistics: An Analysis of on-Shelf Availability and Stockout Responses for Three Product Groups.” International Journal of Logistics Research and Applications 10 (3): 251–268. doi:10.1080/13675560701478075.
- Mena, C., B. Adenso-Diaz, and O. Yurt. 2011. “The Causes of Food Waste in the Supplier–Retailer Interface: Evidences from the UK and Spain.” Resources, Conservation and Recycling 55 (6): 648–658. doi:10.1016/j.resconrec.2010.09.006.
- Mena, C., L. A. Terry, A. Williams, and L. Ellram. 2014. “Causes of Waste across Multi-Tier Supply Networks: Cases in the UK Food Sector.” International Journal of Production Economics. 152: 144–158. doi:10.1016/j.ijpe.2014.03.012.
- Milgate, M. 2001. “Supply Chain Complexity and Delivery Performance: An International Exploratory Study.” Supply Chain Management: An International Journal 6 (3): 106–118. doi:10.1108/13598540110399110.
- Mishra, N., and A. Singh. 2018. “Use of Twitter Data for Waste Minimisation in Beef Supply Chain.” Annals of Operations Research 270 (1–2): 337–359. doi:10.1007/s10479-016-2303-4.
- Mishra, N., W. Morris, A. Singh, and S. McGuire. 2014. “A Multi-Agent Architecture for Lamb Supply Chain.” International Journal of Intelligent Engineering Informatics 2 (2/3): 195–214. doi:10.1504/IJIEI.2014.066213.
- Mishra, N., A. Singh, S. Kumari, K. Govindan, and S. I. Ali. 2016. “Cloud-Based Multi-Agent Architecture for Effective Planning and Scheduling of Distributed Manufacturing.” International Journal of Production Research 54 (23): 7115–7128. doi:10.1080/00207543.2016.1165359.
- Mishra, N., A. Singh, N. P. Rana, and Y. K. Dwivedi. 2017. “Interpretive Structural Modelling and Fuzzy MICMAC Approaches for Customer Centric Beef Supply Chain: Application of a Big Data Technique.” Production Planning & Control 28 (11–12): 945–963. doi:10.1080/09537287.2017.1336789.
- Navarrete-Molina, C., C. A. Meza-Herrera, M. A. Herrera-Machuca, N. Lopez-Villalobos, A. Lopez-Santos, and F. G. Veliz-Deras. 2019. “To Beef or Not to Beef: Unveiling the Economic Environmental Impact Generated by the Intensive Beef Cattle Industry in an Arid Region.” Journal of Cleaner Production 231: 1027–1035. doi:10.1016/j.jclepro.2019.05.267.
- OECD/Eurostat. 2005. Environmental protection expenditure and revenue joint questionnaire/SERIEE environmental protection expenditure account: conversion guidelines. Luxemburg office for official publications of the European communities. http://epp.eurostat.ec.europa.eu/cache/ITYP_OFFPUB/KS-EC-05-001/EN/KS-EC-05-001-EN.PDF.
- Parfitt, J., M. Barthel, and S. Macnaughton. 2010. “Food Waste within Food Supply Chains: Quantification and Potential for Change to 2050.” Philosophical Transactions of the Royal Society of London. Series B, Biological Sciences 365 (1554): 3065–3081.
- Park, M., Y. Jin, and A. H. Love. 2011. “Dynamic and Contemporaneous Causality in a Supply Chain: An Application of the US Beef Industry.” Applied Economics 43 (30): 4785–4801. doi:10.1080/00036846.2010.498357.
- Parliament Publications, UK. 2017. Food waste in England-Eighth Report of Session 2016–17. Accessed on 24 June 2020. https://publications.parliament.uk/pa/cm201617/cmselect/cmenvfru/429/429.pdf
- Raak, N., C. Symmank, S. Zahn, J. Aschemann-Witzel, and H. Rohm. 2017. “Processing-and Product-Related Causes for Food Waste and Implications for the Food Supply Chain.” Waste Management 61: 461–472.
- Rahman, S. U. 2002. “The Theory of Constraints’ Thinking Process Approach to Developing Strategies in Supply Chains.” International Journal of Physical Distribution & Logistics Management 32 (10): 809–828. doi:10.1108/09600030210455429.
- Scavarda, A. J., T. Bouzdine‐Chameeva, S. M. Goldstein, J. M. Hays, and A. V. Hill. 2006. “A Methodology for Constructing Collective Causal Maps*.” Decision Sciences 37 (2): 263–283. doi:10.1111/j.1540-5915.2006.00124.x.
- Simons, D., and K. Zokaei. 2005. “Application of Lean Paradigm in Red Meat Processing.” British Food Journal 107 (4): 192–211. doi:10.1108/00070700510589495.
- Singh, A., S. Kumari, H. Malekpoor, and N. Mishra. 2018. “Big Data Cloud Computing Framework for Low Carbon Supplier Selection in the Beef Supply Chain.” Journal of Cleaner Production 202: 139–149. doi:10.1016/j.jclepro.2018.07.236.
- Singh, A., N. Mishra, S. I. Ali, N. Shukla, and R. Shankar. 2015. “Cloud Computing Technology: Reducing Carbon Footprint in Beef Supply Chain.” International Journal of Production Economics 164: 462–471. doi:10.1016/j.ijpe.2014.09.019.
- Singh, A., N. Shukla, and N. Mishra. 2018. “Social Media Data Analytics to Improve Supply Chain Management in Food Industries.” Transportation Research Part E: Logistics and Transportation Review 114: 398–415. doi:10.1016/j.tre.2017.05.008.
- Spang, E. S., Y. Achmon, I. Donis-Gonzalez, W. A. Gosliner, M. P. Jablonski-Sheffield, M. A. Momin, L. C. Moreno, et al. 2019. “Food Loss and Waste: measurement, Drivers, and Solutions.” Annual Review of Environment and Resources 44 (1): 117–113.40. doi:10.1146/annurev-environ-101718-033228.
- Steinfeld, H., P. Gerber, T. Wassenaar, V. Castel, M. Rosales, and C. Haan. 2006. Livestock Long shadow: Environmental Issues and Options. Food and Agriculture Organisation of United Nations. http://www.europarl.europa.eu/climatechange/doc/FAO%20report%20executive%20summary.pdf
- Walker, E. D., and J. F. Cox. III. 2006. “Addressing Ill-Structured Problems Using Goldratt's Thinking Processes: A White Collar Example.” Management Decision 44 (1): 137–154. doi:10.1108/00251740610641517.
- Warren, H. E., N. D. Scollan, G. R. Nute, S. I. Hughes, J. D. Wood, and R. I. Richardson. 2008. “Effects of Breed and a Concentrate or Grass Silage Diet on Beef Quality in Cattle of 3 Ages. II: Meat Stability and Flavour.” Meat Science 78 (3): 270–278. doi:10.1016/j.meatsci.2007.06.007.
- Whitehead, P., M. Palmer, C. Mena, A. Williams, and C. Walsh. 2011. Resource Maps for fresh meat across retail and wholesale supply chains. http://www.wrap.org.uk/sites/files/wrap/RSC009-002_-_Meat_Resource_Map.pdf
- Winikoff, M. 2005. “JACK™ Intelligent Agents: An Industrial Strength Platform.” In Multi-Agent Programming. Boston, MA: Springer, 175–193.
- Wu, J. Y., and H. I. Hsiao. 2021. “Food Quality and Safety Risk Diagnosis in the Food Cold Chain through Failure Mode and Effect Analysis.” Food Control. 120: 107501. doi:10.1016/j.foodcont.2020.107501.
- Zarandi, M. F., M. Pourakbar, and I. B. Turksen. 2008. “A Fuzzy Agent-Based Model for Reduction of Bullwhip Effect in Supply Chain Systems.” Expert Systems with Applications 34 (3): 1680–1691. doi:10.1016/j.eswa.2007.01.031.