Abstract
A lean supply chain (LSC) is a set of organizations directly linked by upstream and downstream value streams between processes that work collaboratively to reduce costs and waste. Currently, supply chains (SCs) have been put to the test as the world has had to face a series of unprecedented disruptions in demand and supply caused by the COVID-19 pandemic. In this paper, a detailed study of constructs and multistructural components was carried out to develop a conceptual reference model that merges Industry 4.0 (I4.0) digital technologies with lean manufacturing tools to reduce waste and minimize costs in the lean supply chain planning (LSCP) context. The main theoretical contribution of this conceptual proposal is to establish a structured relation among the lean, agile, sustainable, resilient and flexible paradigms to improve SC performance by implementing I4.0 enabling technologies. The proposed conceptual model, dubbed as LSCP 4.0, is applied and validated with a case study in a large footwear company. It can help decision-makers and researchers to improve the planning and management of digital SC production processes, even with unexpected disruptions.
1. Introduction
A supply chain (SC) is typically characterized by a forward flow of materials and a backward flow of information (Singh and Sharma Citation2014). Consequently, the objective of supply chain management (SCM) is to create the most value, not simply for the company, but also for the whole SC network, including end customers (Lambert and Cooper Citation2000). One of the key functions affected by implementing different disruptive technologies is SCM (Haddud and Khare Citation2020). (Garay-Rondero et al. Citation2019) indicate that the entire SC structure is changing due to digitalization needs. This means that different conceptual models and a review of Industry 4.0 (I4.0) concepts and elements are necessary to confer greater visibility, as well as information and business flexibility. (Aqlan and Lam Citation2015) describe how SCs are more susceptible to vulnerability and risks due to factors like the globalization of material supply, production, sales competitiveness and natural disasters, among others. Thus in today’s SCs, with considerable offshoring of raw materials and parts suppliers, production planning is a key process along with transportation planning (Díaz-Madroñero, Mula, and Peidro Citation2014; Mula, Díaz-Madroñero, and Peidro Citation2012).
Lean manufacturing (LM) is a way of thinking, where the whole system approach creates a culture in which everyone in the organization continuously improves operations (Panizzolo et al. Citation2012). LM and I4.0 have similar objectives as both approaches seek improvements in productivity and quality, focus on eliminating waste and are customer-oriented (Pagliosa, Tortorella, and Ferreira Citation2019). However, an approach suggested by Moyano-Fuentes, Bruque-Cámara, and Maqueira-Marín (Citation2019) indicates that one of the main challenges that companies face when starting lean implementations is better integration with their key suppliers and customers. Opportunities also arise to improve performance in SCs, such as reducing excess inventories, backlogs, extra supply and transportation costs and the need to cut the cost of supply and transportation (Rossini and Portioli Citation2018). Allaoui, Guo, and Sarkis (Citation2019) refer to supply chain planning (SCP) systems to help organizations to support sustainability.
A lean supply chain (LSC) can be defined as a set of organizations that are directly linked by upstream and downstream flows of products, services, finance and information, which work collaboratively to reduce costs and waste by efficiently and effectively extracting what is required to meet individual customers’ needs Moyano-Fuentes, Bruque-Cámara, and Maqueira-Marín (Citation2019). In line with this, Swenseth and Olson (Citation2016) have contributed to the development of lean supply chain management (LSCM) by providing benefits to improve inventory control and to move towards continuous process improvement by adopting just-in-time manufacturing. In the I4.0 context, one effect is identified in SC that moves towards a more collaborative model by interacting with people, facilitating their work and allowing workers to direct their efforts to solve problems (Ramirez-Peña et al. Citation2020). This suggests improvements in business process development, technology and the need to share best practices and knowledge with companies in the SC network (Wagire et al. Citation2021). Furthermore, process mapping is necessary to define supplier information and material flow to not only implement key control points but also achieve SC improvements (Carmignani Citation2015). Additionally, the operations strategies that adopt lean practices in an I4.0 context as pull production for value stream management can reduce SC inventories and improve both information delivery and management (Takeda Berger, Frazzon, and Carreirao Danielli Citation2019). In 2020, the leagility, resilience and sustainability of SCs were put to the test with a new instigator of SC disruptions caused by COVID-19 and its global pandemic (Ivanov Citation2020b). So despite the considerable progress made in SCs operations management research (Zhang et al. Citation2020), integrative conceptual frameworks of lean technologies and I4.0 are required to help companies and their SCs to guide their decisions to achieve operational efficiency, resilience and sustainability. Several authors underline the link between LM and sustainability (Martínez-Jurado and Moyano-Fuentes Citation2014), and the results to date show LM’s impact on all three sustainability aspects: environmental, economic and social (Ashby, Leat, and Hudson-Smith Citation2012). Accordingly, the risks associated with sourcing in the global SC may derive from environmental or social performance, but also from disruptions in operational processes, as discussed in SC risk management (Seuring and Müller Citation2008). Other LM frameworks and conceptual models identified in the literature (Pereira, Oliveira, and Carravilla Citation2020; Hernandez, Mula, and Ferriols Citation2008; Garay-Rondero et al. Citation2019; Rossini and Portioli Citation2018; Mula, Díaz-Madroñero, and Peidro Citation2012; Mundi et al. Citation2019; Soni and Kodali Citation2016; Tortorella, Miorando, and Marodin Citation2017; Castro and Jaimes Citation2017) have not addressed these disruptions and possible coping strategies (Sharma, Adhikary, and Borah Citation2020). For example, none of the transportation disruption recovery models developed to date has investigated continuous production planning by considering both delivery delay and proportional quantity loss (Paul et al. Citation2019). However, to survive and grow in today’s competitive environment, suppliers should act as a seamless extension of the organization, and it is necessary to extend the lean implementation to their SC partners (Yadav et al. Citation2019). Finally, Ramirez-Peña et al. (Citation2020) provide an overview of the literature that focuses on 11 I4.0 technologies and the lean, agile, resilient, and sustainable paradigms, which connect the necessary social aspects for an I4.0 conceptual model of shipbuilding SC performance. Nevertheless, integration among the enabling I4.0 technologies, LM tools, and SCs forms a wide research gap.
The motivation of our research is to propose a conceptual model of LSC planning (LSCP) with structured relations between I4.0 technologies and current SC paradigms, such as agile, flexible, lean, sustainable and resilient. Thus a systematic literature review was performed according to Denyer and Tranfield (Citation2009) and Seuring and Müller (Citation2008) to answer the following research questions: (i) what is the current state of knowledge about conceptual models encompassing different SC paradigms and I4.0 concepts?; (ii) what are the main structural and technological elements of an LSC for production and operations planning in an I4.0 environment?, (iii) how can an LSCP conceptual model in an I4.0 context contribute to managers and researchers?
Therefore, this paper aims to develop a conceptual model following the methodology proposed by Hernandez, Mula, and Ferriols (Citation2008) from a literature review that defines what LSCP should look like. The purpose of this conceptual proposal is to also provide new knowledge for implementing I4.0 technologies that integrate LM tools into digitized SCM, even with global disruptions.
The remainder of the article is organized as follows. Section 2 provides a literature review on conceptual models and discusses the contribution of several authors to the LSC paradigm. Section 3 discusses the conceptual modelling methodology for SCP processes. Section 4 uses the proposed methodology to develop a conceptual model for LSCP 4.0. Finally, Section 5 presents the conclusions and future research directions.
2. Literature review
Here based on the review methodologies by Denyer and Tranfield (Citation2009) and Seuring and Müller (Citation2008), a brief systematic literature review is presented. It involves three main steps of the research process in the operations and SCM field : (i) establishing the research question; (ii) collecting data; (iii) performing a detailed data analysis. Thus for data collection purposes, we refer to Seuring and Müller (Citation2008), who show that most research is based on keyword searches in relevant scientific databases (Web of Science and Scopus). Hence, combinations of the following keywords were used as search criteria: industry 4.0, conceptual model, supply chain, planning, lean manufacturing, agile, and resilient. A time window between 2011, coinciding with the emergence of the I4.0 initiative, and 2021 was set to identify the main published conceptual models that link SCM, LM, I4.0, and their enabling technologies, in sourcing, production and logistics processes for SCP. Then the main exclusion criterion for both searches was papers not related to the research questions. Finally, 32 references were selected from journals (93.5%) and conferences (6.5%). One group of six journals represented 48.4% of all the reviewed references: International Journal of Production Research, Journal of Manufacturing Technology Management, Production Planning and Control, International Journal of Production Economics, Journal of Cleaner Production and Annals of Operations Research.
A conceptual model is a set of concepts used to represent or describe an event, object or process, and can be based on the integration of different works into the same subject (Mundi et al. Citation2019). Hernandez, Mula, and Ferriols (Citation2008) describe a methodology that identifies and analyses inputs, outputs, processes and subprocesses for the conceptual modelling of production planning processes, which can be applied to other modelling domains. Several SCM models have been defined by other authors in this field. The work of Mula, Díaz-Madroñero, and Peidro (Citation2012) presents a conceptual model that serves as a reference for developing new production technology and integrates material requirements planning, production resource capacities and SC. Other conceptual models of production planning show that this process is one of the most important SC activities in the short and mid-term, and is one of the main inputs for orders (Mundi et al. Citation2019).
Although most studies have focussed on three areas of SC disruptions, namely supply disruption, production disruption and demand fluctuation, several papers have centred on developing models for other SC disruption recovery planning types (Paul et al. Citation2019). To date, many studies have worked on SC network design with disruptions in a variety of domains, such as supplier selection and order allocation (Govindan, Mina, and Alavi Citation2020). Some examples of disruptive risks are earthquakes and tsunamis, man-made catastrophes, legal disputes, strikes and epidemic outbreaks, among others (Ivanov Citation2020a). Recently, Ivanov (Citation2020b) proposed the concept of a viable SC model to help companies to guide their decisions about recovering and rebuilding their SCs after long-term global crises, such as the COVID-19 pandemic. These results are consistent with Ivanov and Dolgui (Citation2020), who analyzed the intertwined supply network model to ensure the provision of societal goods and services, as well as markets in interconnected SCs.
The incorporation of lean thinking into SCM conceptual models is another relevant contribution. There is more than one way of making SC operations management lean. With a system dynamics model, the impact of lean, agile, flexible and responsive structures on logistics performance in SCs has been evaluated (Castro and Jaimes Citation2017). SC risk modelling has also been used to identify appropriate mitigation strategies for risks at minimum cost (Aqlan and Lam Citation2015). According to Swenseth and Olson (Citation2016), leagile SCs models are proposed as a hybrid between lean and agile to incorporate flexibility as a strategic advantage by integrating other business partners to quickly react, but will operationally lead to additional costs in relation to lean operations. Pull production can be implemented into SCs to promote replenishment and production (Takeda Berger, Frazzon, and Carreirao Danielli Citation2019). Finally, Soni and Kodali (Citation2016) provide interpretive structural modelling for LSCs by focussing on structural self-interaction aspects. There are also other structural SC designs for supply-demand allocations (Ivanov Citation2020b; Carmignani Citation2015; Yadav et al. Citation2019; Ramirez-Peña et al. Citation2020). This modelling technique can be applied to several research fields, such as LSCP. These results converge towards models for lean automation, like that proposed by Tortorella et al. (Citation2020) from an intelligent value stream perspective for vertical and horizontal integration that considers both internal processes of manufacturers and external SCs.
Several SCP models have been developed to improve performance (Rossini and Portioli Citation2018; Ramirez-Peña et al. Citation2020; Allaoui, Guo, and Sarkis Citation2019; Mula, Díaz-Madroñero, and Peidro Citation2012; Carmignani Citation2015; Garay-Rondero et al. Citation2019). However, Pereira, Oliveira, and Carravilla (Citation2020) identify that mathematical modelling approaches and solution procedures have been mainly used and do not consider sufficient tools and methodologies for SCP in the midterm given the increasingly complex contexts of modern SCs. Carmignani (Citation2015) highlights the application of the lean total quality management (TQM) tool to align production with suppliers to obtain a better interaction between SC actors, improve product quality and maximize productivity. Rossini and Portioli (Citation2018) analyze information exchange and lean approaches that lead to inventory savings, but require more transportation efforts. Other approaches like Moyano-Fuentes, Bruque-Cámara, and Maqueira-Marín (Citation2019) validate an LSCM measurement tool to improve financial and operational performance in SCP, and to forecast customer demands and use queues and buffers to manage uncertainty. This highlights the use of LM tools like 5S (sort, straighten, shine, systematize, sustain) for workplace cleanliness and standardization, value stream mapping (VSM) to identify environmental impacts or social waste, pull flow, setup time reduction that refers to SMED (single minute exchange of dies) and Kanban systems (Zhu, Zhang, and Jiang Citation2020). A collaborative decision-making framework for sustainable SCP is proposed by Allaoui, Guo, and Sarkis (Citation2019), which contributes to making improvements in the sustainability of delivered products and in a network’s collaborative relationships.
The literature review also indicates models with information and communication technologies with a strong impact. Haddud and Khare (Citation2020) developed a conceptual model to examine the possible impacts of SCs’ digitization on lean operations. Garay-Rondero et al. (Citation2019) present a digital SC model that provides technical guidance for I4.0 technologies and seeks to reduce some of the barriers against implementing all the elements surrounding this fourth transformation in SCM. Raji and Rossi (Citation2019) propose the combination of various lean and agile SC principles with I4.0 technologies as drivers to improve overall performance (Ramirez-Peña et al. Citation2020) put forward a conceptual model that integrates lean, agile, resilient and green paradigms to ensure SCs’ economic sustainability by integrating I4.0-enabling technologies like additive manufacturing (AM), vertical and horizontal integration systems, cybersecurity, big data, machine learning, blockchain, Internet of Things (IoT), cloud computing, simulation, augmented reality, radio frequency identification (RFID) and self-driving vehicles (Ramirez-Peña et al. Citation2020). Maturity models are useful for helping manufacturing organizations track the progress of their I4.0 initiatives and to guide digitization (Caiado et al. Citation2021; Zeller, Hocken, and Stich Citation2018). With these considerations, Carolis et al. (Citation2017) evaluate the digital readiness of manufacturing companies with four structural analysis areas: processes, monitoring and control, technology and organization. More recently, Wagire et al. (Citation2021) put forward a maturity model to assess organizational maturity levels for I4.0 based on seven dimensions: people and culture, I4.0 awareness, organizational strategy, value chain and processes, smart manufacturing technology, product- and services-oriented technology and I4.0 based technology. Based on the above discussion, there is growing concerned about the economic and social impact of the COVID-19 pandemic, and companies around the world are dealing with four major challenges: demand-supply mismatch, technology challenges, sustainable SC challenges, as well as the challenges associated with building a resilient SC (Sharma, Adhikary, and Borah Citation2020). These challenges are related to the adoption of I4.0 technologies. Finally, some articles propose different mathematical programming models for LSCP (Shafiee, Zare Mehrjerdi, and Keshavarz Citation2021; Das Citation2018, Citation2019; Fahimnia, Sarkis, and Eshragh Citation2015; Oh and Jeong Citation2019).
synthetises the literature review on I4.0 technologies and ICT related to SC paradigms. We also identify within the different conceptual frameworks that present the SC paradigms (leanness, agility, resilience, sustainability, flexibility) which reflected the interaction among their technological components, SC structures and SCP processes. Finally, we identify the modelling approach used by the authors: quantitative (T), qualitative (L), or both (Q-L).
Table 1. Literature review synthesis.
We also identified various studies that empirically approximate an LSCP measure in an I4.0 context. Ramirez-Peña et al. (Citation2020) offer a review of conceptual models to connect some key I4.0 technologies with the most important SC paradigms (lean, agile, resilience, green). The term viable SC model is presented by Ivanov (Citation2020b), which discusses the relations between resilience and viability (i.e. agile, lean, sustainable, resilient, and digital SC) with a structural SC design for supply and demand allocations in a changing environment. Despite identifying the paradigms that support the LSCM in their interaction with others, previous works have recognized that it is still necessary to identify the structural relations around these individual frameworks that can conceptually guide their roles and interactions as contemplated in the I4.0 performance model. Consequently, there is a research gap in relating the I4.0 technologies and LM tools that improve SC performance and, more specifically, SCP processes.
3. Conceptual modelling for lean supply chain planning in Industry 4.0
In order to develop a maturity model, the step-by-step approach is followed based on the guidelines suggested by Wagire et al. (Citation2021). Other similar methodologies and approaches have been used in Soni and Kodali (Citation2016) and Garay-Rondero et al. (Citation2019). A conceptual modelling methodology allows a structure to be developed that provides the order and direction of the complex relations in a set of elements. Therefore, the methodology of this research is an adaptation of that proposed by Hernandez, Mula, and Ferriols (Citation2008) to improve the understanding of the I4.0 concept as a system integrated into LSCP. This methodology is based on six main phases to develop a conceptual process model: 1 (visualization), 2 (analysis), 3 (conceptualization), 4 (modelling), 5 (validation) and 6 (proposal), plus phase C for decision making and modelling corrections. presents this scheme applied to LSCP 4.0.
Figure 1. Conceptual methodology for LSCP 4.0 based on (Hernandez, Mula, and Ferriols Citation2008).
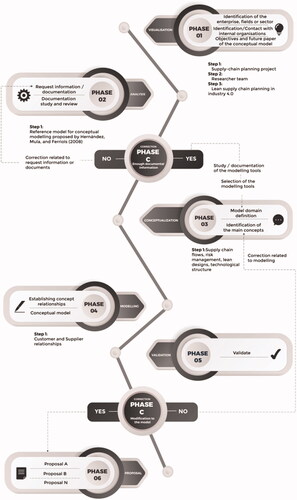
According to , the main phases of the proposed research method follow the steps taken by Hernandez, Mula, and Ferriols (Citation2008) to develop the conceptual model, which are listed below:
Visualization: the first step in the modelling process is about understanding the problem. Here researchers must obtain an understanding of the overall objectives for conceptual modelling
Analysis: it considers the definition of processes and identification of the existing documents in the study area. The techniques and tools for conceptual modelling should also be selected
Conceptualization: in this third step, the scope of conceptual modelling is provided with a literature review of other reference models. Physical resource flows (products and materials) and information flows are also established
Modelling: in this phase, flow chart modelling tools, and conceptual relations like inputs, outputs, environment, users and feedback indicators, are also established
Validation: here the model should be verified according to the parameters established in the analysis and the study objectives
Proposal: the purpose of this step is to document the requirements and improvement proposals generated from the conceptual model for future solutions
Correction: if necessary, this phase should be used when inconsistencies appear in the model validation
Thus firstly as in Mundi et al. (Citation2019), the environment or modelling domain in which the analyzed documents were developed is identified. All these parameters can be used simultaneously for LSCP-related aspects, and the interconnectivity between the actors along the entire chain is graphically visualized to improve its performance. Secondly, the methodology followed to identify and analyze inputs, outputs and processes for the conceptual modelling of SC planning are applied. Finally, the model is validated with the explored SC digitization implications for lean operations.
4. Lean supply chain planning model design
SCP involves several important decisions that range from the strategic opening of a facility to the operational scheduling of a task on a particular machine. In general, three decision-making levels are considered: strategical, tactical and operational (Allaoui, Guo, and Sarkis Citation2019). To achieve this, the focus lies in information systems, customer orientation and suppliers. Six processes that constantly interact are considered: strategical, production, marketing, logistics, supplier, collaboration management (Siddh et al. Citation2017; Soni and Kodali Citation2016). According to these identified criteria, it is necessary to define and understand all the constructs that are incorporated as important LSCM elements, regardless of them being digital and physical. Several authors suggest that epidemic outbreaks like COVID-19 require operations managers to think and act in new and unfamiliar ways by revising the conceptual models used to understand SC disruptions (Ivanov and Dolgui Citation2020; Craighead, Ketchen and Darby Citation2020). Therefore, formal operational planning is needed to minimize the impact of sudden disruptions, such as transportation disruptions (Paul et al. Citation2019).
shows the elements that LSCP 4.0 can present for current SC planning environments from the multi structural perspective of fulfilling business objectives. As a result, a basic view of the elements needed to improve information flows is proposed (Garay-Rondero et al. Citation2019), along with the resources among six internal processes that receive digital inputs from the planning process (Allaoui, Guo, and Sarkis Citation2019). The presented analysis showed the potential of four external perspectives to reduce SC waste, and to improve collaborative management, among customers, suppliers and producers through inbound and outbound logistics. In an innovative way, LM practices are now integrated as support tools for risk management (Seuring and Müller Citation2008; Martínez-Jurado and Moyano-Fuentes Citation2014). This is important for reducing global SC disruptions and developing customer value. On the marketing side, proactive innovation becomes a driver to manage this process in an efficient supply chain environment, and customer satisfaction is an indicator of the degree to which this synergy is achieved (Soni and Kodali Citation2016).
4.1. Supply chain flows
The SCM framework consists of three closely interrelated elements: the SC network structure, SC business processes and SCM (Lambert and Cooper Citation2000). According to Garay-Rondero et al. (Citation2019), the most important outcome in any digital SC is virtual value creation. Several authors refer to flows in an SC, such as materials and information flowing through the main components of the physical distribution channel, as suppliers, factories and distribution warehouses, and even end customers (Haddud and Khare Citation2020; Garay-Rondero et al. Citation2019; Ivanov Citation2020b). The literature also refers to knowledge flow with lean initiatives for integrations with suppliers, and to obtain benefits from reducing commercial risks (Soni and Kodali Citation2016). In economic flow terms, both the methods and concepts that jointly optimize the economic, environmental and social costs of SC operations are a challenge (Allaoui, Guo, and Sarkis Citation2019). For example, Choi (Citation2020) shows how government subsidies can support service operations with health crises by responding to the need for greater SC resilience (Remko Citation2020). Moreover, as I4.0 technologies support information flows in all SC processes, the value flow in a company also provides benefits by favouring both vertical and horizontal integration (Tortorella et al. Citation2020). Consequently, LSCM aims to provide a waste-free flow of goods, services and technology from suppliers to customers (Khorasani, Cross, and Maghazei Citation2020).
Supplier and customer integrations in the I4.0 configuration require a hub firm to integrate the flow of information, materials and finance with its customers and suppliers (Ghobakhloo and Fathi Citation2019). The inputs and outputs of SCs’ main processes are proposed in , which shows information feedbacks mainly from SCs’ performance indicators. This customer value flow through social network analyses facilitate, for example, demand forecasting in companies by accelerating the reaction to emergency events like natural disasters, improving marketing programmes and reducing waste (Choi, Guo, and Luo Citation2020). In short, this knowledge framework integrates LM into business technology implementations and offers a model of strategic interactions between SC links and their ecosystems to provide sustainable survivability.
4.2. Risk management
A disaster management cycle is an attempt made to plan the strategies and measures that come into play from the time a disaster starts until it ends (Govindan, Mina, and Alavi Citation2020). In addition, pandemics require academics to once again discuss what lenses offer an understanding of SCs’ phenomena to help managers to better prepare for the next pandemic and to foster resilience (Craighead, Ketchen, and Darby Citation2020). Current SC dimensions must be integrated into lean thinking to reduce waste. By being lean, responsive and globalized in structural designs, SCs must not only become sustainable during the disruptions triggered by severe natural or human problems but must also use the advantages of digital technologies for this management (Araz et al. Citation2020; Ivanov Citation2020b). With the COVID-19 outbreak, a radical change in the global logistics system has come about, and the proposed planning process should stimulate new strategies. Such SC risks are distinctively characterized by simultaneous long-term disruptions in supply, demand and logistics infrastructures (Ivanov Citation2020a). However, as companies face challenges in supply and demand mismatch terms, applying technologies like artificial intelligence and blockchain can help SCs to become more resilient (Sharma, Adhikary, and Borah Citation2020). Governments have heavily relied on not only the use of simulation to determine how best to reduce the impact of natural disasters and health systems but also the risks of SCs’ disruptions due to unprecedented demands (Currie et al. Citation2020). During pandemics, there are legal risks for companies that boost their logistics operations without disruption. For example, a court ruling led to the closure of Amazon’s distribution centres in France because of government restrictions imposed by the health crisis. For the company, this meant that the risk of accidentally shipping non-essential items was too high (Craighead, Ketchen, and Darby Citation2020).
In performance terms, one of the objectives is to analyze the impact of SCP on several service levels. SC performance indicators have been mainly classified as: (i) supply lead time; (ii) costs with supply and raw material; (iii) inventory level; (iv) delivery service level; (v) quality (Tortorella, Miorando, and Marodin Citation2017). Currently, there are constraints that inhibit I4.0 adoption: inadequate understanding of I4.0, lack of strategic vision, lack of competent personnel, worker training needs and no access to capital, which comprise the main constraints (Ivanov et al. Citation2021). Yet value-oriented, long-term SCM and value-oriented management approaches increase companies’ resilience to cope with extremely volatile and extreme events like COVID-19 (Trautrims et al. Citation2020). Accordingly, the key factors for eliminating SC risks include the need to balance global sourcing with local sourcing, adopting multiple sourcing, and broader utilization of available information technology (Remko Citation2020). The advent of I4.0 has created opportunities to improve operations in different fields. Rodríguez-Espíndola et al. (Citation2020) indicate that, in order to improve information flow, products, and financial resources in humanitarian SCs, three emerging disruptive technologies must be integrated: artificial intelligence, blockchain, and 3D printing. However, this poses a major challenge for production and operations planners. According to Usuga Cadavid et al. (Citation2020), machine-learning models provide manufacturing systems with the ability to adapt to unexpected events and to forecast production problems. For instance, building analytical models to explore whether traditional service operations, such as static operations in a fixed location supported by technologies, can become mobile service operations that bring services close to customers and help companies to survive disasters like pandemics (Choi Citation2020). In short, the relation of disruptions like the COVID-19 pandemic with lean practices has revealed weaknesses in operations planning and JIT (just-in-time) deliveries.
4.3. Lean design
Some recent studies reveal that organizations should view LSCM as an opportunity to improve their system flow by eliminating non-added value (Garcia-Buendia, Moyano-Fuentes, and Maqueira-Marín Citation2021; Khorasani, Cross, and Maghazei Citation2020). Authors like Khorasani, Cross, and Maghazei (Citation2020) identify unique LSCM elements/practices, of which eight practices are defined as pillars of efficient SCM implementation: information technology management, supplier management, waste elimination, JIT production, customer relationship management, logistics management, top management commitment and continuous improvement. So the real value for customers can be specified by waste minimization during processes. In this context, to date seven 7 waste types are known in LM: overproduction, inventory, extra processing, motion, waiting for time, defects and transportation (Amrina and Lubis Citation2017). More recently, digital operations in the I4.0 context have generated new waste types: non-utilized talent, poor information management, poor supplier quality. Thus according to Reyes et al. (Citation2018), the application of LM has become one of the most widely applied methodologies to reduce costs and to improve seamless flows between processes.
Some major challenges for applying lean practices are the two-way feedback evaluation, the value chain management team and the intervention strategy (Tortorella, Miorando, and Marodin Citation2017). However, by being lean and globalized in structures, the SCs of many companies are especially vulnerable to SC disruptions, simultaneous disruption propagation (i.e. the ripple effect), and epidemic outbreak propagation (Ivanov Citation2020a). In fact, minimizing waste and reducing disruption while providing a flow of goods, services, and technologies from suppliers to customers maximize the value added to all SC agents (Moyano-Fuentes, Bruque-Cámara, and Maqueira-Marín Citation2019). For example, Reyes et al. (Citation2018) mention that total productive maintenance (TPM) enables high-quality standards to be achieved by compliance with planned shutdowns, and by reducing non-productive times and, consequently, optimal costs. More importantly, LM represents a prerequisite for unlocking the I4.0 potential and preventing waste automation (Chiarini, Belvedere, and Grando Citation2020).
Essentially, the SCP model based on lean management can increase the number of delivery trips, which can lead to higher transportation costs (Rossini and Portioli Citation2018). This important aspect refers to lead time delivery and forecasted capacity, which leads to the better management of the entire planning system, production flow and staff involvement (Carmignani Citation2015). In addition, some findings show that Heijunka in production levelling (Khorasani, Cross, and Maghazei Citation2020) and JIT production by the Kanban concept through integrated information systems helps to maintain a continuous value flow in SCs (Tortorella et al. Citation2020). Given the abrupt changes in consumer behaviour and an evident increase in demand for various products (e.g. from the pharmaceutical and medical industry) when the COVID-19 pandemic started, (Trautrims et al. Citation2020) identify how some SCs faced insufficient supply availability, which meant having to rapidly incorporate new suppliers, in contrast to previously used LSC practices like single sourcing cutting, buffer inventory and slack capacity.
Process and product standardization is basically an LM tool that offers the best way to preserve an organization’s knowledge base (Soni and Kodali Citation2016). This means that lean practices like Poka-Yoke and Kaizen use a wide variety of automatic devices in the event of errors to reduce defective products and to foster a continuous improvement culture (Haddud and Khare Citation2020). Remko (Citation2020) states that during SC disruptions caused by COVID-19, inventory cost issues have arisen, and risk has shifted to the suppliers along the chain insofar as while lean tools may have been widely applied to reduce inventories, SC agility has been reduced by factors beyond the organization, such as logistical bottlenecks. This has resulted in insufficient preparation for supply disruptions, and in not applying existing contingency plans.
I4.0 technologies that effectively digitize lean tools can be deployed to address production and operations challenges (Chiarini, Belvedere, and Grando Citation2020). This shift redefines the SC landscape towards the implementation of new strategies for agile and flexible manufacturing. I4.0 can be the solution for implementing a completely flexible manufacturing system (Kumar, Suhaib, and Asjad Citation2020). Indeed artificial intelligence is suitable for generating knowledge from production planning and control data, which is crucial in I4.0, where data abound, and it can provide useful guidelines to improve a company’s knowledge (Usuga Cadavid et al. Citation2020). Shahin et al. (Citation2020) show a fail-safe system like intelligent Jidoka, enabled for cyberphysical systems (CPS), which integrates service-oriented architecture, cloud computing, and IoT to provide a flexible configuration. Pagliosa, Tortorella, and Ferreira (Citation2019) indicate that lean tools like Poka-Yoke and Andon can help to share data in real-time. Recent studies like that by Ghobakhloo and Fathi (Citation2019) demonstrate the successful application of lean practices to statistical quality control operations and real-time TQM through information technology.
4.4. Technological structure
I4.0 is a generic term for highly complex and automated manufacturing systems, services, and business processes, where devices are self-aware, communicate with one another and with humans and can be remotely accessed, using information available in the network and in the cloud (Kumar, Suhaib, and Asjad Citation2020). However, it is necessary to protect information flows with cyber security technologies to avoid the risk of data, information and knowledge being stolen and misused (Chiarini, Belvedere, and Grando Citation2020). In the I4.0 context, some research works have focussed mainly on manufacturing applications of technologies, such as AM, IoT, blockchain, advanced robotics, and artificial intelligence (Ivanov et al. Citation2021). The approaches to artificial intelligence based on machine learning are classified into three groups: supervised learning, unsupervised learning and reinforcement learning (Coronato et al. Citation2020). The advantages of this technology enable the design of decision support systems to perform supplier selection, introduce agility into SCs, big data mining patterns for risk identification purposes and manage information from multiple sources (Rodríguez-Espíndola et al. Citation2020). Although many machine-learning applications exist, smart planning and scheduling are the most widely addressed problems in the recent scientific literature (Usuga Cadavid et al. Citation2020). According to Raji and Rossi (Citation2019), the main advantages of 3D printing or AM for SCM in different stages along SCs are to increase manufacturing flexibility, offer shorter lead times, reduce inventory and increase product customization.
According to Garay-Rondero et al. (Citation2019), a digital SC model in I4.0 consists of six continuously interconnected dimensions: (i) physical and digital SCM components and processes; (ii) an interconnected physical and digital SC network structure; (iii) I4.0 technology implementation and digitization; (iv) SC material, product, service, knowledge and digital information flows; (v) virtual value chain; (vi) a globally connected agile and collaborative approach. These dimensions are aligned with other reference production planning models that relate product, information and decision flow (Hernandez, Mula, and Ferriols Citation2008). One novelty that forms part of emerging I4.0 technologies is the use of social networks in combination with blockchain and big data analyses to minimize waste through consumer insights, as presented by Mishra and Singh (Citation2018), Choi, Guo, and Luo (Citation2020), Gupta, Modgil, and Gunasekaran (Citation2020), Garay-Rondero et al. (Citation2019), Peralta et al. (Citation2020) and Zangiacomi et al. (Citation2020).
The development of lean-digitized manufacturing systems is a viable business strategy for enterprises’ survival in the I4.0 environment (Ghobakhloo and Fathi Citation2019). Currently, there is evidence for positive interaction between lean practices and I4.0 technologies towards achieving higher operational performance. However, Pagliosa, Tortorella, and Ferreira (Citation2019) mention that those manufacturers undergoing LM implementation and I4.0 technologies should preferably invest their efforts in IoT and CPS technologies.
As organizations’ analytics capabilities rapidly develop, technological innovations like data analytics are being used in the industry to improve their operational risk management (Araz et al. Citation2020). Tracking and tracing systems aim to identify and analyze SC deviations, alert about disruptions that have occurred or may occur, and performing control actions to restore SC operability (Ivanov et al. Citation2019). To protect against disruption risks, simulation is another technology used to quantitatively analyze process performance in SCs (Currie et al. Citation2020; Rossini and Portioli Citation2018). This analysis identifies successful and unsuccessful elements of risk mitigation or preparedness policies and outbreak recovery (Ivanov Citation2020a). A combination of simulation, optimization, and data analytics constitutes a digital twin: a new data-driven vision of managing disruption risks in SC (Ivanov et al. Citation2019). A digital SC twin is a model that can represent the network state for any given moment in time and allows for complete end-to-end SC visibility to improve resilience and test contingency plans (Ivanov et al. Citation2019). Regarding the relation between LM and I4.0, technologies like RFID and blockchain can accelerate information exchange and improve the visibility of inventory positions and logistic flows (Haddud and Khare Citation2020; Remko Citation2020). As blockchain technology is computational in nature, the most recent studies have focussed on analyzing social network data as a critical factor in SC operations management (Choi, Guo, and Luo Citation2020). Finally, as a machine-learning subfield, reinforcement learning can enhance the efficiency and continuous improvement of processes by promoting behavioural decision-making capabilities by employing world interaction experience and evaluative feedback in, for example, healthcare domains for automated medical diagnostics (Coronato et al. Citation2020).
4.5. The conceptual model
, based on Ivanov (Citation2020a), illustrates the main scheme of the material and information flows in today’s SCs. The proposed conceptual model provides a framework to adopt the various SC paradigms by the literature review and I4.0 concepts with current management approaches, for example, lean, which have been integrated to provide higher performance and disruption support levels. This approach is shown within a multidimensional and interconnected framework with various technological and managerial implications.
The LSCP 4.0 multi-structural model consists of a set of processes interconnected by digital SC material and information flows, in combination with physical SCs. The ecosystem framework shown in is based on the SC itself, performance indicators and production and/or operations planning. Their interaction results from feedback about the risk of SC disruptions, LM practices to reduce waste and the technology framework for value stream creation purposes. The relations of interest are those associated with chain logistics. These interactions provide information for important performance indicators of this process, such as delivery lead times, supply costs, inventory level, service level and quality. Correlations are used to determine the relations between input parameters and outputs to generate value for customers. For example, Choi, Guo, and Luo (Citation2020) employed blockchain, and indicate that consumer confidence in online reviews on social networks would significantly affect customer satisfaction.
From the methodological point of view, the conceptual model presents four perspectives: structural view, dynamic state view, performance view and control view (). The needs assessment was performed for the digitization of SCs to provide companies with resilience, agility, sustainability and flexibility in employing the technological capabilities that enhance their ability to run their SCs without disruption (Haddud and Khare Citation2020). In addition, new applications for optimization models for tactical production planning are identified (Díaz-Madroñero, Mula, and Peidro Citation2014) in the SC viability area due to feedback considerations and waste management dynamics in an I4.0 environment. Another aspect regards the essential elements for the successful implementation of digital I4.0 technologies driven by data analytics to uncover the decision support potential (Coronato et al. Citation2020), even in SC flow disruption environments (Ivanov and Dolgui Citation2020). SC network integration through technologies like big data and blockchain (Choi, Guo, and Luo Citation2020), and the generation of contingency plans for disruption risks by simulation and operations planning systems based on machine learning (Usuga Cadavid et al. Citation2020), support the basis among SC efficiency, agility and sustainability.
Alternatively, Ramirez-Peña et al. (Citation2020) have focussed on establishing a conceptual framework for defining a specific industrial sector, the shipbuilding SC, according to the model established in I4.0 performance considering the paradigms lean, agile resilient and green. Ivanov (Citation2020b) offers a conceptual framework in SC ecosystems that exhibits the features of leagility and resilience against disruptions like pandemic resistance. Here we propose a novel multi structural integrative approach to these paradigms by placing an emphasis on LM tools to improve SC performance and, more specifically, SCP processes. The results show a strong impact between SCP and its performance, especially in environments of crisis and disruption, such as pandemics. Thus using LM practices like JIT in conjunction with I4.0 initiatives to guide the digitization of collaborative relations in the SC structure and risk management is a new scenario for recovering SCs after natural disasters, and for fostering flexibility to processes and sustainability to operations.
4.6. Model validation
In order to validate the model, we interviewed several decision-makers, who are LM and ICT engineering experts from a large footwear manufacturing company. This methodology allowed us to diagnose the possibility of applying the conceptual proposal to improve SCP processes from lean, agile, sustainable, resilient, and flexible paradigms. Based on the literature analysis (Ivanov et al. Citation2021; Chiarini, Belvedere, and Grando Citation2020; Haddud and Khare Citation2020; Tortorella et al. Citation2020), we constructed the questionnaire to help to understand the research participants’ opinions, experiences and values.
Thus, we consider diagnosing the SC of this footwear industry with the proposed conceptual model. From the lean position for waste reduction, manufactured shoes must follow a production-to-order plan. However, disruptions like the COVID-19 pandemic have led demand to unexpectedly drop. Consequently, across the entire SC (e.g. leather, glue, textile and packaging suppliers), decision-makers were forced to reduce production and to limit their inventory. As customer requirements constantly change in design and material quality terms, the SC must be agile enough to respond as quickly as possible to actual demand, especially during high-demand seasons, so that demand planners use I4.0 technologies (e.g. IoT, big data and simulation) and ICT to establish forecasted and centralized safety inventories to meet customer demand in time. Therefore, processes are evaluated using performance indicators (e.g. delivery service level, supplier quality, among others). However, the company has no risk management for SC resilience decisions to measure how quickly it reaches the state prior to disruption. Therefore from the flexibility position, the company does not apply its full technological potential and must strengthen its I4.0 technologies to provide collaborative systems (e.g. tracking and tracing systems for shoes, big data for production and logistics decision making, among others) with real-time information across the SC network to react in time to changing environments and its executives’ effective decision making. This application of the conceptual model is shown in , which provides the company understudy with a diagnosis of its current situation of implementing LM practices and I4.0 technologies, but also generates some practical implications for improvement.
Table 2. Diagnosis of the company using the conceptual model.
shows the improvement proposals and practices to be implemented by decision-makers along the footwear company’s SC structure, which are defined according to the SC paradigms that affect the performance indicators based on the four conceptual model dimensions (). These practices are described below:
Table 3. Improvement SCP proposals in the footwear industry.
LP1: use VSM to identify and eliminate waste throughout the SC
AP1: ICT integration with critical suppliers to improve performance in flexibility and responsiveness terms to demand variations
AP2: Employ big data and business process management systems to manage demand uncertainty
AP3: Short-term forecasting of customer demands to reduce standard inventory deviation and to improve service levels
SP1: Implement machine learning into production processes and cloud computing to share knowledge throughout the SC
SP2: Forecast the propagation of disruptions and their economic impact throughout the SC to draw up contingency plans
SP3: Install a photovoltaic power plant
RP1: Adjust production and inventory plans with JIT philosophy using cloud computing, and tracking and tracing systems.
RP2: Frequent feedback and teamwork to share solutions using the IoT among SC members
RP3: Utilising big data for the early detection of SC disruptions
FP1: Apply operational and contingency policies using simulation for deployment in the different scenarios in a disruption
FP2: Leverage Andon and cloud computing systems in production plants and warehouses to confer changes in process performance responsiveness
FP3: Implement sewing cells with artificial intelligence
5. Conclusions
The main objective of this study is to develop a conceptual planning model for managing SCs in I4.0 environments. Mainly, the lean philosophy to reduce waste was incorporated. Additionally, other current SC paradigms, such as agility, flexibility, and resilience, by considering some emerging risks of global disruptions between suppliers and manufacturers, are contemplated. Next, the answers to the specific research questions and the conclusions of this paper are presented.
What is the current state of knowledge on conceptual models encompassing different SC paradigms and I4.0 concepts? The analysis based on a systematic literature review allowed us to identify the most significant conceptual models in the LSCM area, where the influence on the planning processes of different SC paradigms and technological components was revealed. Thus, the effects of lean, agile, flexible and resilient structures on SCs’ performance were addressed. This allowed us to deduce that there is very little theoretical knowledge to date that integrates I4.0 technologies, LM tools and other paradigms as fundamental concepts that strongly influence the planning, organization, and control of SC processes. I4.0 digital technologies were also contemplated for the transformation of SC operations into a digital environment. Here, digital machine-learning technologies for the design of decision support systems were highlighted, along with big data and blockchain, as enabling technologies for the most important lean practices that reduce waste in companies. At the same time, the output of this study revealed several theoretical and practical implications in LSCP. In this way, lean, resilient, and agile paradigms reinforce SC through the implementation of the 11 remaining enabling technologies. From a practical perspective, to successfully implement lean into enterprises, proper management and owner commitment is recommended, and training should be provided to managers to improve their leadership (Yadav et al. Citation2019). This finding is consistent with other studies (Raghu Kumar, Agarwal, and Sharma Citation2016; Ramirez-Peña et al. Citation2020; Ivanov Citation2020b; Garay-Rondero et al. Citation2019).
What are the main structural and technological elements of an LSC for production and operations planning in an I4.0 environment? In order to move towards a planning approach, LSCP elements in the I4.0 context were identified from a multi-structural perspective. Strategies like pull production for customer value stream management, which can reduce waste and improve performance in organizations, were contemplated. This article considered the methodology proposed by Hernandez, Mula, and Ferriols (Citation2008) for developing conceptual models. Thus a 6-phase sequential process was followed with two differentiated correction stages for obtaining documentary information and defining the model’s final criteria. This modelling methodology was applied to define SC planning by employing lean tools and I4.0 technologies, and in a disruptive risk environment. This means that an innovative approach of lean practices integrated into the SC planning model was proposed. As mentioned by Garcia-Buendia, Moyano-Fuentes, and Maqueira-Marín (Citation2021), to realize the potential benefits of LM, it is vital that lean principles and practices extend throughout the SC. An understanding of collaboration and communication aspects in a lean-digitized manufacturing system context was, therefore, provided. This revealed that lean tools, such as Kanban, JIT and VSM, impact SC operations management in an I4.0 context. Simulation tools led to more collaborative management between suppliers and producers which can help to determine the best way to reduce the impact of disasters. Moreover, risk management aspects were considered based on Ivanov (Citation2020b), but with benefits and future directions that focus on lean planning to address shortage risks in SCs in the I4.0 environment, and even in a crisis environment during a pandemic like COVID-19. As a result, this confirmed the importance of technologies like social network data analytics for SC operations management.
How can an LSCP conceptual model in an I4.0 context contribute to managers and researchers? Here we tested the proposed conceptual model in a large footwear company by making a global diagnosis of the application of LM practices and I4.0 enabling technologies to provide improvement proposals. After presenting the diagnosis and SCP improvement proposals to the company, the managers involved positively validated the usefulness of them within the conceptual model framework. Thus, managerial implications are oriented to provide a tool that evaluates the current state of an organization and to support them to streamline improvement actions for SCP by reducing waste in a globalized environment of I4.0 technologies. The obtained results are a contribution that can help managers to solve the challenges of risks of interruptions in SCs, such as the implications of COVID-19 in planning, sourcing, and inventory acquisitions. The model can be used by researchers and practitioners as a tool to identify common characteristics with other conducted research. For example, practitioners can apply structural conceptual models to address core SC dimensions as a path plan to implement quality, such as perishable foods to improve the organization’s sustainable yield (Siddh et al. Citation2018). In this way, for the SC flows observed in the examined models, ICT development has had a huge impact on information management and its integrated control, finances, risks and merchandise flows, which enables a new range of production systems and distributions (Garay-Rondero et al. Citation2019). Besides, researchers can use it as a framework to identify gaps to direct future research.
On the one hand, specific limitations of this work need to be addressed. Thanks to the employed conceptual modelling method, although it was possible to propose a framework, currently not many papers on the management of disruptions in LSCs have been published. On the other hand, the COVID-19 pandemic is a new phenomenon, which means that complementary ideas on the subject could be addressed in relation to business experiences and post-pandemic research into the application of lean tools to streamline operations between suppliers and manufacturers for customer deliveries. Finally, we propose the following future research agenda: (i) although lean practices have been widely used, in the digital technologies I4.0 environment they can now help managers to generate a greater value stream for customers, and a digital LSC that faces risks is a line for future experimental research using simulation and optimization; (ii) extend the literature review about the impact of I4.0 technologies on multidisciplinary decision-making processes of SC members for sourcing planning, production and distribution of goods; (iii) study the role of digitalization, specifically artificial intelligence and big data, to implement performance indicators into SCP by a disruptive event like the COVID-19 pandemic; (iv) develop optimization and simulation models to support the conceptual proposal by also taking multicriteria decision-making approaches (Yadav and Sharma Citation2015; Singh and Sharma Citation2014); (v) model the different LSCM aspects, specifically the social and economic aspects required to support flexibility and a strong corporate culture provided by the resilient SC from an I4.0 perspective.
Disclosure statement
No potential conflict of interest was reported by the author(s).
Additional information
Funding
Notes on contributors
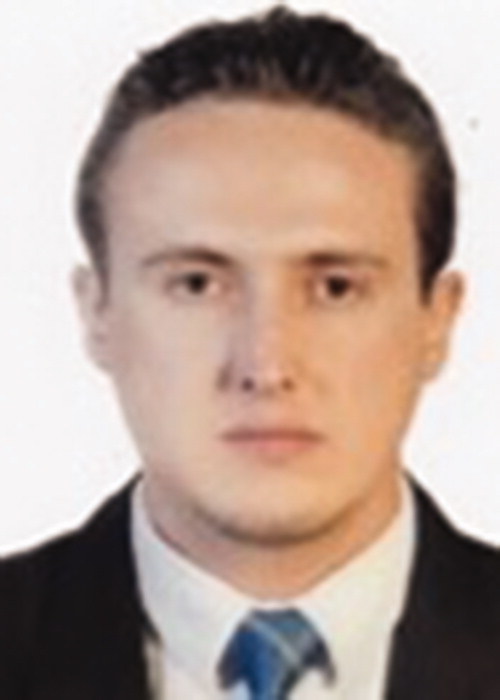
John Reyes
John Reyes is Aggregate Professor in the Technical University of Ambato (UTA), Ecuador. He has worked as an Industrial Engineer in the area of consulting for mining companies and the footwear sector, where he has been involved in logistical, operational and management processes, as well as in the area of risk analysis. He is currently pursuing a PhD program in engineering and industrial production at the Universitat Politècnica de València (UPV), Spain. In addition, he has served as Director of Research and Development at UTA. His research interests include information technologies, industrial engineering, supply chain management, operations planning, lean manufacturing tools, business process management, and modelling and simulation.
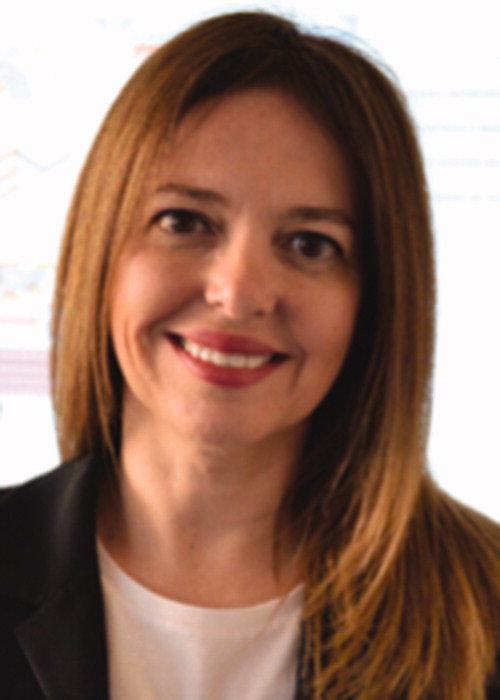
Josefa Mula
Josefa Mula is Professor in the Department of Business Management of the Universitat Politècnica de València (UPV), Spain. She is a member of the Research Centre on Production Management and Engineering (CIGIP) of the UPV. Her teaching and principal research interests concern production engineering and management, operations research and supply chain simulation. She is editor in chief of the International Journal of Production Management and Engineering. She is author of more than 100 papers mostly published in international books and high-quality journals, among which International Journal of Production Research, Fuzzy sets and Systems, International Journal of Production Economics, European Journal of Operational Research, Computers and Industrial Engineering, and Production Planning and Control.
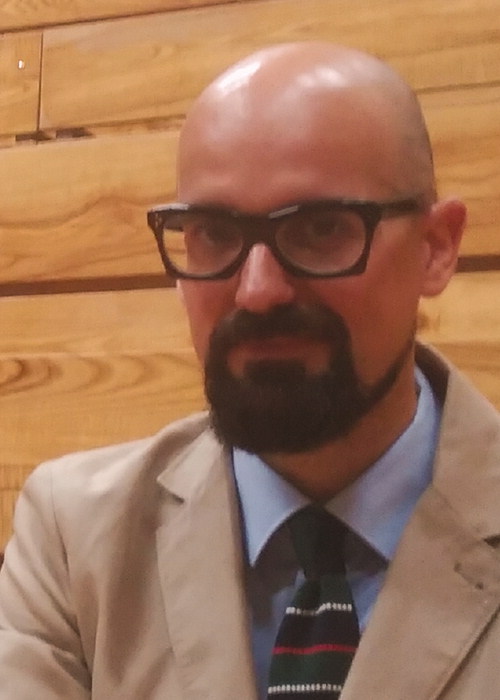
Manuel Díaz-Madroñero
Manuel Díaz-Madroñero is Associate Professor in the Department of Business Management of the Universitat Politècnica de València (UPV), Spain. He teaches subjects related to Information Systems, Operational Research and Operations Management and Logistics. He is member of the Research Centre on Production Management and Engineering (CIGIP) of the UPV. He has participated in different research projects funded by the European Commission, the Spanish Government, the Valencian Regional Goverment and the UPV. As a result, he has published (in collaboration) more than forty articles in different indexed journals and international conferences. He is co-author of the book Operations Research Problems: Statements and Solutions (Springer, 2014). His research areas include production planning and transportation, fuzzy mathematical programming and robust optimisation, multicriteria decision making and sustainable operations management.
References
- Allaoui, H., Y. Guo, and J. Sarkis. 2019. “Decision Support for Collaboration Planning in Sustainable Supply Chains.” Journal of Cleaner Production 229: 761–774. doi:10.1016/j.jclepro.2019.04.367.
- Amrina, E., and A. A. Lubis. 2017. “Minimizing Waste Using Lean Manufacturing: In Cement Production.” Paper presented at the 4th International Conference on Industrial Engineering and Applications ICIEA, Nagoya, Japan, April 21–23.
- Aqlan, F., and S. S. Lam. 2015. “Supply Chain Risk Modelling and Mitigation.” International Journal of Production Research 53 (18): 5640–5656. doi:10.1080/00207543.2015.1047975.
- Araz, O. M., T.-M. Choi, D. L Olson, and F. S. Salman. 2020. “Data Analytics for Operational Risk Management.” Decision Sciences 51 (6): 1316–1319. doi:10.1111/deci.12443.
- Ashby, A., M. Leat, and M. Hudson-Smith. 2012. “Making Connections: A Review of Supply Chain Management and Sustainability Literature.” Supply Chain Management 17 (5): 497–516. doi:10.1108/13598541211258573.
- Caiado, R. G. G., L. F. Scavarda, L. O. Gavião, P. Ivson, D. L. D. M. Nascimento, and J. A. Garza-Reyes. 2021. “A Fuzzy Rule-Based Industry 4.0 Maturity Model for Operations and Supply Chain Management.” International Journal of Production Economics 231: 107883. doi:10.1016/j.ijpe.2020.107883.
- Carmignani, G. 2015. “Lean Supply Chain Model and Application in an Italian Fashion Luxury Company.” In Understanding the Lean Enterprise: Strategies, Methodologies, and Principles for a More Responsive Organization, edited by A. Chiarini, P. Found, and N. Rich, 203–220. Cham, Switzerland: Springer. doi:10.1007/978-3-319-19995-5_9.
- Carolis, A. D., M. Macchi, E. Negri, and S. Terzi. 2017. “A Maturity Model for Assessing the Digital Readiness of Manufacturing Companies.” IFIP Advances in Information and Communication Technology 513: 13–20. doi:10.1007/978-3-319-66923-6_2..
- Castro, J. A. O., and W. A. Jaimes. 2017. “Dynamic Impact of the Structure of the Supply Chain of Perishable Foods on Logistics Performance and Food Security.” Journal of Industrial Engineering and Management 10 (4): 687–710. doi:10.3926/jiem.2147..
- Chiarini, A., V. Belvedere, and A. Grando. 2020. “Industry 4.0 Strategies and Technological Developments. An Exploratory Research from Italian Manufacturing Companies.” Production Planning & Control 31 (16): 1385–1398. doi:10.1080/09537287.2019.1710304.
- Choi, T.-M. 2020. “Innovative ‘Bring-Service-Near-Your-Home’ operations under Corona-Virus (COVID-19/SARS-CoV-2) outbreak: Can logistics become the Messiah?” Transportation Research. Part E, Logistics and Transportation Review 140: 101961. doi:10.1016/j.tre.2020.101961.
- Choi, T. M., S. Guo, and S. Luo. 2020. “When Blockchain Meets Social-Media: Will the Result Benefit Social Media Analytics for Supply Chain Operations Management?” Transportation Research Part E: Logistics and Transportation Review 135: 101860. doi:10.1016/j.tre.2020.101860.
- Coronato, A., M. Naeem, G. De Pietro, and G. Paragliola. 2020. “Reinforcement Learning for Intelligent Healthcare Applications: A Survey.” Artificial Intelligence in Medicine 109: 101964. doi:10.1016/j.artmed.2020.101964.
- Craighead, C. W., Jr., D. J. Ketchen, and J. L. Darby. 2020. “Pandemics and Supply Chain Management Research: Toward a Theoretical Toolbox.” Decision Sciences 51 (4): 838–866. doi:10.1111/deci.12468.
- Currie, C. S. M., J. W. Fowler, K. Kotiadis, T. Monks, B. S. Onggo, D. A. Robertson, and A. A. Tako. 2020. “How Simulation Modelling Can Help Reduce the Impact of COVID-19.” Journal of Simulation 14 (2): 83–97. doi:10.1080/17477778.2020.1751570.
- Das, K. 2018. “Integrating Lean Systems in the Design of a Sustainable Supply Chain Model.” International Journal of Production Economics 198: 177–190. doi:10.1016/j.ijpe.2018.01.003.
- Das, K. 2019. “Integrating Lean, Green, and Resilience Criteria in a Sustainable Food Supply Chain Planning Model.” International Journal of Mathematical, Engineering and Management Sciences 4 (2): 259–275. doi:10.33889/IJMEMS.2019.4.2-022.
- Denyer, D., and D. Tranfield. 2009. “Producing a Systematic Review.” In The Sage Handbook of Organizational Research Methods, 671–689. Thousand Oaks, CA: Sage Publications Ltd.
- Díaz-Madroñero, M., J. Mula, and D. Peidro. 2014. “A Review of Discrete-Time Optimization Models for Tactical Production Planning.” International Journal of Production Research 52 (17): 5171–5205. doi:10.1080/00207543.2014.899721.
- Fahimnia, B., J. Sarkis, and A. Eshragh. 2015. “A Tradeoff Model for Green Supply Chain Planning: A Leanness-versus-Greenness Analysis.” Omega 54: 173–190. doi:10.1016/j.omega.2015.01.014.
- Garay-Rondero, C. L., J. L. Martinez-Flores, N. R. Smith, S. O. C. Morales, and A. Aldrette-Malacara. 2019. “Digital Supply Chain Model in Industry 4.0.” Journal of Manufacturing Technology Management 31 (5): 887–933. doi:10.1108/JMTM-08-2018-0280.
- Garcia-Buendia, N., J. Moyano-Fuentes, and J. M. Maqueira-Marín. 2021. “Lean Supply Chain Management and Performance Relationships: What Has Been Done and What is Left to Do.” CIRP Journal of Manufacturing Science and Technology 32: 405–423. doi:10.1016/j.cirpj.2021.01.016.
- Ghobakhloo, M., and M. Fathi. 2019. “Corporate Survival in Industry 4.0 Era: The Enabling Role of Lean-Digitized Manufacturing.” Journal of Manufacturing Technology Management 31 (1): 1–30. doi:10.1108/JMTM-11-2018-0417.
- Govindan, K., H. Mina, and B. Alavi. 2020. “A Decision Support System for Demand Management in Healthcare Supply Chains considering the Epidemic Outbreaks: A Case Study of Coronavirus Disease 2019 (COVID-19).” Transportation Research. Part E Logistics and Transportation Review 138: 101967. doi:10.1016/j.tre.2020.101967.
- Gupta, S., S. Modgil, and A. Gunasekaran. 2020. “Big Data in Lean Six Sigma: A Review and Further Research Directions.” International Journal of Production Research 58 (3): 947–969. doi:10.1080/00207543.2019.1598599.
- Haddud, A., and A. Khare. 2020. “Digitalizing Supply Chains Potential Benefits and Impact on Lean Operations.” International Journal of Lean Six Sigma 11 (4): 731–765. doi:10.1108/IJLSS-03-2019-0026.
- Hernandez, J., J. Mula, and F. Ferriols. 2008. “A Reference Model for Conceptual Modelling of Production Planning Processes.” Production Planning & Control 19 (8): 725–734. doi:10.1080/09537280802476128.
- Ivanov, D. 2020a. “Predicting the Impacts of Epidemic Outbreaks on Global Supply Chains: A Simulation-Based Analysis on the Coronavirus Outbreak (COVID-19/SARS-CoV-2) Case.” Transportation Research. Part E Logistics and Transportation Review 136: 101922. doi:10.1016/j.tre.2020.101922.
- Ivanov, D. 2020b. “Viable Supply Chain Model: Integrating Agility, Resilience and Sustainability Perspectives—Lessons from and Thinking beyond the COVID-19 Pandemic.” Annals of Operations Research . doi:10.1007/s10479-020-03640-6.
- Ivanov, D., and A. Dolgui. 2020. “Viability of Intertwined Supply Networks: Extending the Supply Chain Resilience Angles towards Survivability. A Position Paper Motivated by COVID-19 Outbreak.” International Journal of Production Research 58 (10): 2904–2915. doi:10.1080/00207543.2020.1750727.
- Ivanov, D., A. Dolgui, A. Das, and B. Sokolov. 2019. “Digital Supply Chain Twins: Managing the Ripple Effect, Resilience, and Disruption Risks by Data-Driven Optimization, Simulation, and Visibility.” International Series in Operations Research and Management Science 276: 309–332. doi:10.1007/978-3-030-14302-2_15..
- Ivanov, D., C. S. Tang, A. Dolgui, D. Battini, and A. Das. 2021. “Researchers’ Perspectives on Industry 4.0: Multi-Disciplinary Analysis and Opportunities for Operations Management.” International Journal of Production Research 59 (7): 2055–2078. doi:10.1080/00207543.2020.1798035.
- Khorasani, S. T., J. Cross, and O. Maghazei. 2020. “Lean Supply Chain Management in Healthcare: A Systematic Review and Meta-Study.” International Journal of Lean Six Sigma 11 (1): 1–34. doi:10.1108/IJLSS-07-2018-0069.
- Kumar, S., M. Suhaib, and M. Asjad. 2020. “Industry 4.0: Complex, Disruptive, but Inevitable.” Management and Production Engineering Review 11 (1): 43–51. doi:10.24425/mper.2020.132942..
- Lambert, D. M., and M. C. Cooper. 2000. “Issues in Supply Chain Management.” Industrial Marketing Management 29 (1): 65–83. doi:10.1016/S0019-8501(99)00113-3.
- Martínez-Jurado, P. J., and J. Moyano-Fuentes. 2014. “Lean Management, Supply Chain Management and Sustainability: A Literature Review.” Journal of Cleaner Production 85: 134–150. doi:10.1016/j.jclepro.2013.09.042.
- Mishra, N., and A. Singh. 2018. “Use of Twitter Data for Waste Minimisation in Beef Supply Chain.” Annals of Operations Research 270 (1–2): 337–359. doi:10.1007/s10479-016-2303-4.
- Moyano-Fuentes, J., S. Bruque-Cámara, and J. M. Maqueira-Marín. 2019. “Development and Validation of a Lean Supply Chain Management Measurement Instrument.” Production Planning & Control 30 (1): 20–32. doi:10.1080/09537287.2018.1519731.
- Mula, J., M. Díaz-Madroñero, and D. Peidro. 2012. “A Conceptual Model for Integrating Transport Planning: MRP IV.” In IFIP Advances in Information and Communication Technology, edited by J. Frick and B. T. Laugen, 54–65. Berlin, Germany: Springer.
- Mundi, I., M. M. E. Alemany, R. Poler, V. S. Fuertes-Miquel, I. Mundi, M. M. E. Alemany, R. Poler, and V. S. Fuertes-Miquel. 2019. “Review of Mathematical Models for Production Planning under Uncertainty Due to Lack of Homogeneity: Proposal of a Conceptual Model.” International Journal of Production Research 57 (15–16): 5239–5283. doi:10.1080/00207543.2019.1566665.
- Oh, J., and B. Jeong. 2019. “Tactical Supply Planning in Smart Manufacturing Supply Chain.” Robotics and Computer-Integrated Manufacturing 55: 217–233. doi:10.1016/j.rcim.2018.04.003.
- Pagliosa, M., G. Tortorella, and J. C. E. Ferreira. 2019. “Industry 4.0 and Lean Manufacturing: A Systematic Literature Review and Future Research Directions.” Journal of Manufacturing Technology Management 32 (3): 543–569. doi:10.1108/JMTM-12-2018-0446.
- Panizzolo, R., P. Garengo, M. K. Sharma, and A. Gore. 2012. “Lean Manufacturing in Developing Countries: Evidence from Indian SMEs.” Production Planning & Control 23 (10–11): 769–788. doi:10.1080/09537287.2011.642155.
- Paul, S. K., S. Asian, M. Goh, and S. A. Torabi. 2019. “Managing Sudden Transportation Disruptions in Supply Chains under Delivery Delay and Quantity Loss.” Annals of Operations Research 273 (1–2): 783–814. doi:10.1007/s10479-017-2684-z.
- Peralta, C. B. L., M. E Echeveste, F. H. Lermen, A. Marcon, and Guilherme Tortorella. 2020. “A Framework Proposition to Identify Customer Value through Lean Practices.” Journal of Manufacturing Technology Management 31 (4): 725–747. doi:10.1108/JMTM-06-2019-0209.
- Pereira, D. F., J. F. Oliveira, and M. A. Carravilla. 2020. “Tactical Sales and Operations Planning: A Holistic Framework and a Literature Review of Decision-Making Models.” International Journal of Production Economics 228: 107695. doi:10.1016/j.ijpe.2020.107695.
- Raghu Kumar, B. R., A. Agarwal, and M. K. Sharma. 2016. “Lean Management – A Step towards Sustainable Green Supply Chain.” Competitiveness Review 26 (3): 311–331. doi:10.1108/CR-05-2015-0040.
- Raji, I. O., and T. Rossi. 2019. “Exploring Industry 4.0 Technologies as Drivers of Lean and Agile Supply Chain Strategies.” Paper presented at the International Conference on Industrial Engineering and Operations Management, Bangkok, Thailand, March 5–7.
- Ramirez-Peña, M., A. J. Sánchez Sotano, V. Pérez-Fernandez, F. J. Abad, and M. Batista. 2020. “Achieving a Sustainable Shipbuilding Supply Chain under I4.0 Perspective.” Journal of Cleaner Production 244: 118789. doi:10.1016/j.jclepro.2019.118789.
- Remko, V. H. 2020. “Research Opportunities for a More Resilient Post-COVID-19 Supply Chain – Closing the Gap between Research Findings and Industry Practice.” International Journal of Operations & Production Management 40 (4): 341–355. doi:10.1108/IJOPM-03-2020-0165.
- Reyes, J., K. Alvarez, A. Martínez, and J. Guamán. 2018. “Total Productive Maintenance for the Sewing Process in Footwear.” Journal of Industrial Engineering and Management 11 (4): 814–822. doi:10.3926/jiem.2644.
- Rodríguez-Espíndola, O., S. Chowdhury, A. Beltagui, and P. Albores. 2020. “The Potential of Emergent Disruptive Technologies for Humanitarian Supply Chains: The Integration of Blockchain, Artificial Intelligence and 3D Printing.” International Journal of Production Research 58 (15): 4610–4630. doi:10.1080/00207543.2020.1761565.
- Rossini, M., and A. Portioli. 2018. “Supply Chain Planning: A Quantitative Comparison between Lean and Info-Sharing Models.” Production & Manufacturing Research 6 (1): 264–283. doi:10.1080/21693277.2018.1509744.
- Seuring, S., and M. Müller. 2008. “From a Literature Review to a Conceptual Framework for Sustainable Supply Chain Management.” Journal of Cleaner Production 16 (15): 1699–1710. doi:10.1016/j.jclepro.2008.04.020.
- Shafiee, M., Y. Zare Mehrjerdi, and M. Keshavarz. 2021. “Integrating Lean, Resilient, and Sustainable Practices in Supply Chain Network: Mathematical Modelling and the AUGMECON2 Approach.” International Journal of Systems Science: Operations and Logistics. Advance online publication. doi:10.1080/23302674.2021.1921878.
- Shahin, M., F. F. Chen, H. Bouzary, and K. Krishnaiyer. 2020. “Integration of Lean Practices and Industry 4.0 Technologies: Smart Manufacturing for next-Generation Enterprises.” The International Journal of Advanced Manufacturing Technology 107 (5–6): 2927–2936. doi:10.1007/s00170-020-05124-0.
- Sharma, A., A. Adhikary, and S. B. Borah. 2020. “Covid-19's impact on supply chain decisions: Strategic insights from NASDAQ 100 firms using Twitter data.” Journal of Business Research 117: 443–449. doi:10.1016/j.jbusres.2020.05.035.
- Siddh, M. M., G. Soni, R. Jain, and M. K Sharma. 2018. “Structural Model of Perishable Food Supply Chain Quality (PFSCQ) to Improve Sustainable Organizational Performance.” Benchmarking 25 (7): 2272–2317. doi:10.1108/BIJ-01-2017-0003.
- Siddh, M. M., G. Soni, R. Jain, M. K. Sharma, and V. Yadav. 2017. “Agri-Fresh Food Supply Chain Quality (AFSCQ): A Literature Review.” Industrial Management & Data Systems 117 (9): 2015–2044. doi:10.1108/IMDS-10-2016-0427.
- Singh, R. K., and M. K. Sharma. 2014. “Selecting Competitive Supply Chain Using Fuzzy AHP and Extent Analysis.” Journal of Industrial and Production Engineering 31 (8): 524–538. doi:10.1080/21681015.2014.999723.
- Singh, R. K., and M. K. Sharma. 2014. “Prioritising the Alternatives for Flexibility in Supply Chains.” Production Planning & Control 25 (2): 176–192. doi:10.1080/09537287.2013.782951.
- Soni, G., and R. Kodali. 2016. “Interpretive Structural Modeling and Path Analysis for Proposed Framework of Lean Supply Chain in Indian Manufacturing Industry.” Journal of Industrial and Production Engineering 33 (8): 501–515. doi:10.1080/21681015.2016.1174959.
- Swenseth, S. R., and D. L. Olson. 2016. “Trade-Offs in Lean vs. Outsourced Supply Chains.” International Journal of Production Research 54 (13): 4065–4080. doi:10.1080/00207543.2016.1173251.
- Takeda Berger, S. L., E. M. Frazzon, and A. M. Carreirao Danielli. 2019. “Pull-Production System in a Lean Supply Chain: A Performance Analysis Utilizing the Simulation-Based Optimization.” Paper presented at the 2018 13th IEEE International Conference on Industry Applications, Sao Paulo, Brazil, November 11–14.
- Tortorella, G. L., R. Miorando, and G. Marodin. 2017. “Lean Supply Chain Management: Empirical Research on Practices, Contexts and Performance.” International Journal of Production Economics 193: 98–112. doi:10.1016/j.ijpe.2017.07.006.
- Tortorella, G., R. Sawhney, D. Jurburg, I. Carisio de Paula, D. Tlapa, and M. Thurer. 2020. “Towards the Proposition of a Lean Automation Framework: Integrating Industry 4.0 into Lean Production.” Journal of Manufacturing Technology Management 32 (3): 593–620. doi:10.1108/JMTM-01-2019-0032.
- Trautrims, A., M. C. Schleper, M. Selim Cakir, and S. Gold. 2020. “Survival at the Expense of the Weakest? Managing Modern Slavery Risks in Supply Chains during COVID-19.” Journal of Risk Research 23 (7–8): 1067–1072. doi:10.1080/13669877.2020.1772347.
- Usuga Cadavid, J. P., S. Lamouri, B. Grabot, R. Pellerin, and A. Fortin. 2020. “Machine Learning Applied in Production Planning and Control: A State-of-the-Art in the Era of Industry 4.0.” Journal of Intelligent Manufacturing 31 (6): 1531–1558. doi:10.1007/s10845-019-01531-7.
- Wagire, A. A., R. Joshi, A. P. S. Rathore, and R. Jain. 2021. “Development of Maturity Model for Assessing the Implementation of Industry 4.0: Learning from Theory and Practice.” Production Planning and Control 32 (8): 603–622. doi:10.1080/09537287.2020.1744763.
- Yadav, V., R. Jain, M. L. Mittal, A. Panwar, and M. K. Sharma. 2019. “An Appraisal on Barriers to Implement Lean in SMEs.” Journal of Manufacturing Technology Management 30 (1): 195–212. doi:10.1108/JMTM-12-2017-0262.
- Yadav, V., and M. K. Sharma. 2015. “Multi-Criteria Decision Making for Supplier Selection Using Fuzzy AHP Approach.” Benchmarking 22 (6): 1158–1174. doi:10.1108/BIJ-04-2014-0036.
- Zangiacomi, A., E. Pessot, R. Fornasiero, M. Bertetti, and M. Sacco. 2020. “Moving towards Digitalization: A Multiple Case Study in Manufacturing.” Production Planning & Control 31 (2–3): 143–157. doi:10.1080/09537287.2019.1631468.
- Zeller, V., C. Hocken, and V. Stich. 2018. “Acatech Industrie 4.0 Maturity Index – A Multidimensional Maturity Model.” IFIP Advances in Information and Communication Technology 536: 105–113. doi:10.1007/978-3-319-99707-0_14.
- Zhang, F., X. Wu, C. S. Tang, T. Feng, and Y. Dai. 2020. “Evolution of Operations Management Research: From Managing Flows to Building Capabilities.” Production and Operations Management 29 (10): 2219–2229. doi:10.1111/poms.13231.
- Zhu, X.-Y., H. Zhang, and Z.-G. Jiang. 2020. “Application of Green-Modified Value Stream Mapping to Integrate and Implement Lean and Green Practices: A Case Study.” International Journal of Computer Integrated Manufacturing 33 (7): 716–731. doi:10.1080/0951192X.2019.1667028.