Abstract
Maintenance is evolving due to the double-sided influence of the Asset Management paradigm and digitalization. In this evolution, assessing the maintenance management process status in terms of process completeness, information and data completeness and integration is paramount to boost reliable data-driven decision-making. Grounding on Design Science Research, a methodology is realized to favour the comparison of two data models, a reference one and a company-specific one, used as a means to evaluate the process status. In particular, the methodology embeds a reference data model for the maintenance management process. Both methodology and data model are artefacts tested and refined during action research in an automotive company willing to improve the maintenance management process. The application of both artefacts demonstrates that the company is facilitated in planning improvement actions for various time horizons to foster a modern maintenance practice whose decision-making is more data-driven.
Disclosure statement
No potential conflict of interest was reported by the author(s).
Correction Statement
This article has been republished with minor changes. These changes do not impact the academic content of the article.
Additional information
Notes on contributors
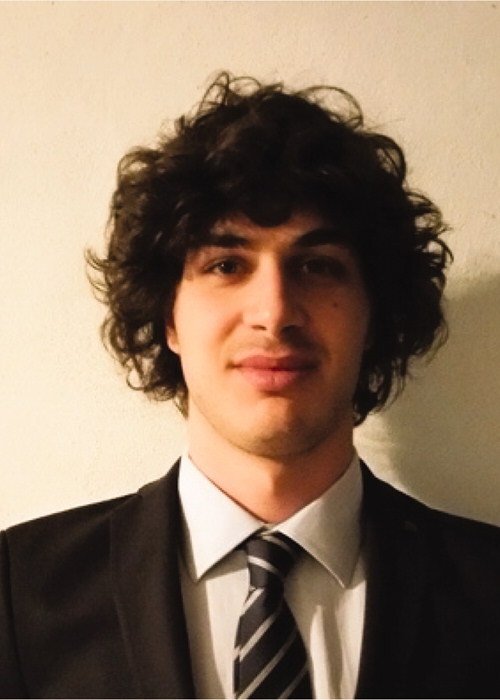
Adalberto Polenghi
Adalberto Polenghi is a Post-doctoral researcher at the Department of Management, Economics and Industrial Engineering at the Politecnico di Milano. He obtained his Ph.D. in Industrial Engineering, specializing in ontological modelling for smart maintenance. His research interests relate to operations management, with attention towards industrial asset management and maintenance, in the manufacturing and process industry. He is the coordinator of research activities at the Industry 4.0 Laboratory (https://www.industry40lab.org) of Politecnico di Milano. Also, he is a researcher of the Observatory on Technologies and Services for Maintenance of the School of Management of the Politecnico di Milano. He authored and co-authored several scientific works published in international and national journals.
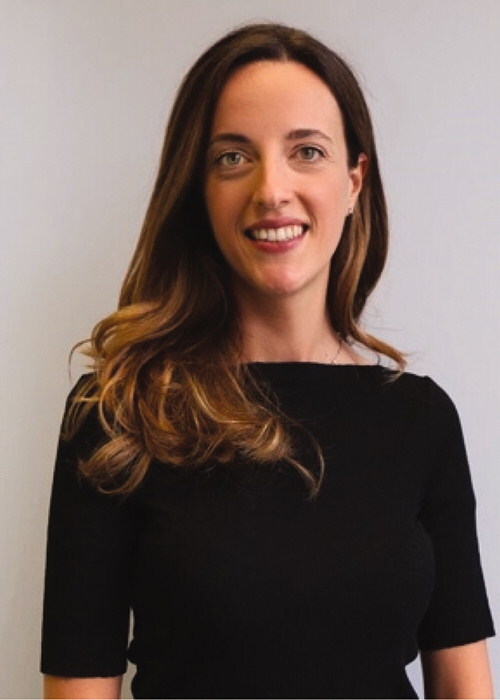
Irene Roda
Irene Roda is Assistant Professor at the Department of Management, Economics and Industrial Engineering at the Politecnico di Milano and she holds a Ph.D. in Industrial Engineering. Her main research and teaching areas are related to industrial asset lifecycle management, operations and maintenance management, and smart manufacturing. She is Director of the Observatory on Technologies and Services for Maintenance of the School of Management of the Politecnico di Milano. She is a member of the IFIP TC5 WG 5.7 Advances in Production Management Systems and co-leader of the Special Interest Group on ‘Product and Asset Lifecycle Management’ at the IFIP TC5 WG 5.7. She is Book Reviews Editor of the International Journal Production Planning & Control: The Management of Operations, Taylor & Francis. She is the author of several scientific publications in national and international journals.
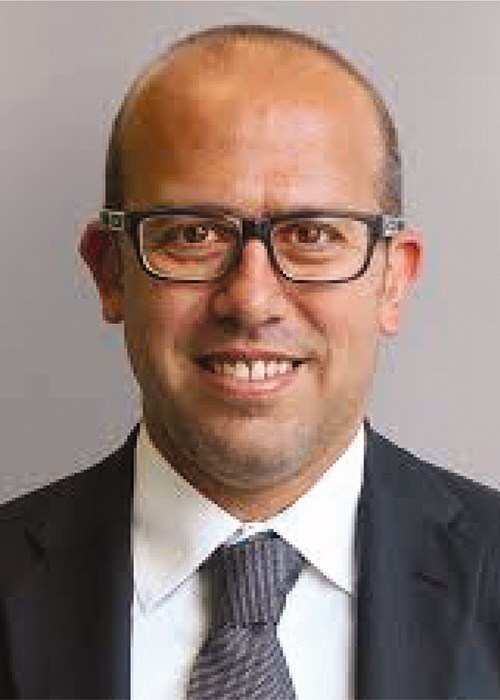
Marco Macchi
Marco Macchi is a Full Professor at the Department of Management, Economics and Industrial Engineering at the Politecnico di Milano. His teaching and research activities regard industrial technologies, asset lifecycle management, operations and maintenance management, smart manufacturing. Serving the scientific community, he is currently Chair of the technical committee IFAC TC 5.1 Manufacturing Plant Control; he is a Member of the IFIP WG 5.7 Advances in Production Management Systems and the IFAC TC 5.3 Integration and Interoperability of Enterprise Systems (I2ES); he is co-leader of the Special Interest Group (SIG) on ‘Product and Asset Lifecycle Management’ at the IFIP TC5 WG 5.7 Advances in Production Management Systems. Concerning his role in scientific journals, he is a Member of the International Editorial Board of Production Planning & Control: the Management of Operations, and he is an Associate Editor of the Journal of Intelligent Manufacturing.
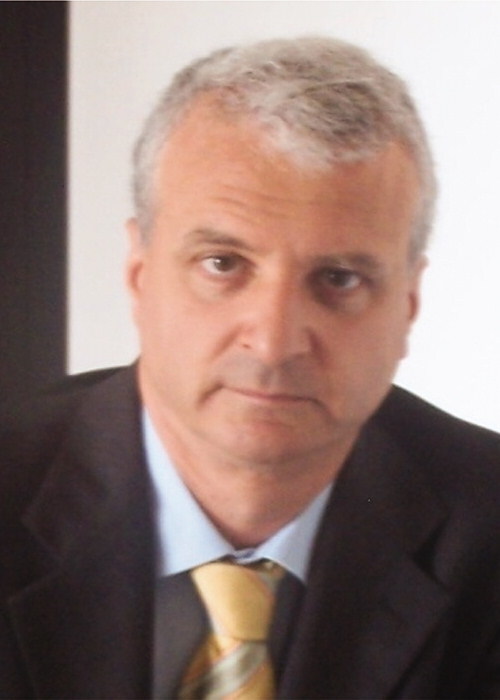
Alessandro Pozzetti
Alessandro Pozzetti is Full Professor of Logistics and Production Management at the Politecnico di Milano, Department of Management, Economics and Industrial Engineering. He is also a Professor of Operations and Quality Management at the MIP Politecnico di Milano Graduate School of Business. He is currently a member of the Evaluation Committee at the Politecnico di Milano where he was previously the Dean of the School of Systems Engineering, the Head of the Department of Management, Economics and Industrial Engineering, and a member of the Academic Senate. His main research interests are in the areas of the design of production systems and operations management. His research publications have appeared in international journals, such as International Journal of Production Research, International Journal of Operations & Production Management, International Journal of Quality & Reliability Management, Production Planning & Control, International Journal of Computer Integrated Manufacturing, International Journal of Production Economics, Journal of Manufacturing Systems.