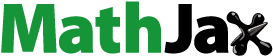
Abstract
In the last century, there was a general perception that scheduling theory was highly irrelevant to scheduling practice. Many recent studies, however, have suggested that the applicability of scheduling approaches is highly intertwined with the manufacturing environment in which the scheduling task is carried out. In this paper we used the constructs of Contingency Theory to suggest specific fits between scheduling approaches and manufacturing environments, after suggesting that the theory-practice gap in production scheduling research has been caused by three issues: (a) simplification of scheduling problems, (b) simplification of the practical scheduling task as a decision process, and (c) lack of relevance of the traditional scheduling approach to all manufacturing environments. Furthermore, we suggest that the dynamism of the state of the system and the complexity of the scheduling problem are the two constituting vectors that define the complexity of the scheduling task. We use both vectors to identify different types of manufacturing environments and propose specific fits with scheduling approaches. Finally, we hypothesize that the fit between scheduling approaches and manufacturing environments is only relevant in environments with high resource utilization where the scheduling task could have a bigger impact on a firm’s performance, and present three case studies to better exemplify the relevance of the conceptual framework.
1. Introduction
Production scheduling has been a very prolific field of study in the last 40 years, as it has covered very different types of scheduling problems while considering a wide variety of constraints and objectives. However, one of the biggest challenges for production scheduling has been to transfer its body of theoretical knowledge to actual practical settings.
Various authors (McKay, Safayeni, and Buzacott Citation1988; Buxey Citation1989; Wiers Citation1997; McKay and Wiers Citation1999; McKay and Buzacott Citation2000) have suggested that the gap between theory and practice in production scheduling is mainly caused by a lack of agreement between the actual scheduling process carried out in shop floors and the tools designed to support the scheduling task. At the same time, several authors only study theoretical problems instead of problems based on real manufacturing environments (Reisman, Kumar, and Motwani Citation1997; Halsall, Muhlemann, and Price Citation1994; Abedinnia et al. Citation2017).
Consequently, the gap between theory and practice could be closed by designing an improved system for supporting decisions related to the scheduling task (Framinan and Ruiz Citation2010; Framinan and Ruiz Citation2012), where all the activities carried out when building a schedule are properly supported.
In 2000, Portougal and Robb suggested that the theory-practice gap could also be caused by the scheduling task’s lack of relevance in certain manufacturing contexts. Portougal and Robb take the view that traditional scheduling theory is not applicable in every context and that some contexts can see better performance by applying different (and simpler) planning practices. This proposal concurs with Contingency Theory (Van de Ven and Drazin Citation1984) which suggests that some particular managerial practices have a better fit with some contextual factors than with others and that even some of the ‘best practices’ in the field could be ill-suited for some particular business environments.
In addition to Portougal and Robb, other authors have undertaken empirical studies on the applicability of production scheduling in different manufacturing contexts and found that the applicability of different approaches to production planning, scheduling and control is context-dependent (see, e.g. Jonsson and Mattsson Citation2003; Romero-Silva, Hurtado, and Santos Citation2016; Tenhiälä Citation2011).
While some evidence from the literature supports the idea that the actual applicability of production scheduling could be directly related to the scheduling environment, no study has synthesized these results into a consolidated framework that explains the main causes of the theory-practice gap in scheduling as well as the reasons for the (lack of) applicability of different scheduling approaches in real production scenarios. Therefore, the objective of this paper is to propose a conceptual framework of the applicability of production scheduling to real production scenarios. Supported by concepts from Contingency Theory, we identify specific fits between manufacturing environments and scheduling approaches. This understanding could help companies to better allocate resources to improve operations, depending on their particular contextual characteristics.
To highlight the relevance of this study and justify the need to propose the conceptual framework, this paper first provides an overview of the current state of the gap between theory and practice in production scheduling research. Supported by the classification of operations management papers using Contingency Theory from Sousa and Voss (Citation2008), we use the findings from previous studies to characterize manufacturing environment types and identify which scheduling approaches (control-theoretic, predictive, reactive and predictive-reactive) are the most suitable for each environment. Three case studies of manufacturing firms are also presented as an illustration of the applicability of the conceptual framework. Finally, we discuss the implications of our propositions and suggest future research opportunities that could help to further close the theory-practice gap in scheduling practice research.
2. The gap between theory and practice in production scheduling research: 20 years later
In 1997, Reisman, Kumar, and Motwani conducted a review of studies concerned with flow-shop scheduling. In that review, they found that <3% of the studies could be classified as studies with a ‘grounding in the real world’. Furthermore, many authors were calling for more research grounded in scheduling realities (King Citation1976; McKay, Safayeni, and Buzacott Citation1988; Buxey Citation1989; Wiers Citation1997; McKay and Wiers Citation1999; McKay and Buzacott Citation2000) to address the needs of schedulers, particularly from the point of view of supporting the scheduling task as a whole and not only as a combinatorial problem.
Those papers suggested that there were two primary reasons that explained why a theory-practice gap existed in the academic literature before 2000: (a) simplification of scheduling problems by developing scheduling solutions focused only on solving either static and deterministic scheduling problems or ‘toy problems’, a term used by Jalali and Van Nieuwenhuyse (Citation2015), where synthetic data is used to represent a scheduling problem, instead of real-world scenarios; and (b) simplification of the practical scheduling task exemplified by the fact that very little research has acknowledged the scheduling task as an iterative and continuous task comprised of more activities than solely building a feasible schedule (e.g. monitoring and control). In this regard, whereas (a) resulted in a lack of applicability of algorithmic and heuristic solutions to solve real-world problems, (b) caused a dearth of effective decision support in real manufacturing environments by ignoring the fundamental characteristics of the scheduling task.
Over the last 20 years, the field of production scheduling has been developing new solutions for complex problems in order to close the gap in terms of issue (a), both in real-world and theoretical instances. In this regard, Fuchigami and Rangel (Citation2018) conducted a very interesting survey of studies related to solving scheduling problems based on real production case studies. Looking at a sample of 46 papers, they found that real applications of scheduling techniques have only been reported since 1992, the most recent years (2011–2016) being the most prolific for this type of study.
In addition, the diversification of theoretical production scheduling research can be clearly seen in Abedinnia et al. (Citation2017) systematic review of 129 reviews of studies concerned with production (machine) scheduling. In that tertiary study, Abedinnia et al. showed that theoretical scheduling research has considered many shop floor environments, a variety of scheduling and resource constraints, and different objective and multi-objective functions. They also show the diversity of solution approaches used to address some of the most complex problems, e.g. exact algorithms, metaheuristics, simulation and artificial intelligence.
On the other hand, a significantly smaller number of studies concerned with addressing (b) have been published, due to the fact that research on (b) needs to be carried out through empirical observations of real scheduling activities, e.g. actual case studies and action research on manufacturing and scheduling operations. The majority of studies addressing issue (b) have investigated the implementation of Advanced Planning and Scheduling systems (APS) in real scenarios (see, e.g. Carvalho, Scavarda, and Lustosa Citation2014; Wiers and de Kok Citation2018a; Wiers Citation2009; Harjunkoski Citation2016). These studies have concluded that dealing with the issue of production plant control and monitoring is equally important as building schedules in order to correctly model the production system and convert it into a tractable scheduling problem.
While recent efforts have certainly helped to close the theory-practice gap that existed 20 years ago in terms of (a) by addressing more realistic scenarios, the industrial market of the 21st century has moved from a mass-production context with stable demands and cyclic production settings towards a customer-responsive market trying to fulfil unstable and variable demands through mass customization (Comstock, Johansen, and Winroth Citation2004; Rebecca Citation2002) and engineer-to-order strategies.
This newer reality has increased the complexity of scheduling problems by adding various constraints and problem characteristics. For instance, the production of different families of products in the same facility has given more relevance to sequence-dependent setup times in current production environments (Tomotani and de Mesquita Citation2018; Romero-Silva, Hurtado, and Santos Citation2016). Furthermore, it is not always easy to predict processing times for customized products accurately, hence the presence of stochastic processing times (Tomotani and de Mesquita Citation2018). Similarly, the change from factory-focus (productivity) to customer-focus (service) has shifted the scheduling objective from minimizing makespan to minimizing the number of tardy jobs (Romero-Silva, Hurtado, and Santos Citation2016). This customer-focused environment has also given issue (b) more relevance regarding the execution of the scheduling task because current dynamic and variable production environments are even more affected by disturbances, such as continuous order arrivals and changes in order specifications, which emphasizes the importance of monitoring and controlling the production plant.
While real scheduling problems that existed more than 20 years ago have been addressed in some cases, as shown by Fuchigami and Rangel (Citation2018) and Abedinnia et al. (Citation2017), the newer realities of a customer-oriented context (Schonberger and Brown Citation2017) have turned static and deterministic schedules into irrelevant solutions for many companies, where a dynamic and stochastic environment exists. Even rescheduling approaches where dynamism and stochasticity are addressed by updating detailed schedules in the presence of new information (see, e.g. Ouelhadj and Petrovic Citation2009; Bidot et al. Citation2009; Vieira, Herrmann, and Lin Citation2003; Framinan, Fernandez-Viagas, and Perez-Gonzalez Citation2019) have been applied seldomly to real industries (Uhlmann and Frazzon Citation2018) or have been found to be inapplicable to certain manufacturing environments because of the high instability and fuzziness of the environment (Abdullah and Abdolrazzagh-Nezhad Citation2014).
This reality suggests that the scheduling approach needed to solve different scheduling problems depends on the manufacturing environment where the scheduling task is executed and that the traditional approach studied in scheduling theory (full, detailed resource assignments for all the jobs), represented in seminal scheduling studies (e.g. Pinedo Citation2016; Morton and Pentico Citation1993; Graham et al. Citation1979), is not a ‘one-size-fits-all’ approach.
Therefore, a third reason for the theory-practice gap becomes apparent, because many practitioners will find that using the traditional scheduling paradigm will not help them carry out their scheduling task: (c) lack of relevance of the traditional scheduling approach to all manufacturing environments. In this regard, several studies have identified the (lack of) applicability of traditional scheduling theory to different manufacturing contexts. For instance, Wiers and Van der Schaaf (Citation1997) argued that traditional scheduling, i.e. proactive scheduling (Vieira, Herrmann, and Lin Citation2003), is highly applicable to environments with no need for shop floor autonomy due to a lack of environmental uncertainty (Smooth shops), whereas it is ill-suited to very uncertain environments that require a high level of human recovery (Socio-technical shops). On the other hand, in environments with high uncertainty and complexity (Stress shops), where a reactive scheduling approach (Vieira, Herrmann, and Lin Citation2003) could be more applicable, human recovery will be handicapped to have good scheduling performance.
Portougal and Robb (Citation2000) suggested that the applicability of traditional scheduling theory depends on the relationship between the mean production cycle time and the planning period. They also suggested that scheduling could be more applicable in job-shop environments than in flow-shop environments with batch production where, they contend, short cycles are more common.
Wiers (Citation2009) and Kjellsdotter (Citation2012) studied the applicability of Advanced Planning and Scheduling (APS) systems depending on shop floor characteristics, based on the classification proposed by Wiers and Van der Schaaf (Citation1997). They both found that APS systems are in fact more applicable to Smooth shops than to Socio-technical shops, where environmental uncertainty hinders the ability of the APS to produce feasible schedules because of the constant changes in the manufacturing system.
De Snoo, Van Wezel, and Jorna (Citation2011) found that uncertainty, measured both by the stability of information and the frequency of informational feedback, is a significant contextual factor in the scheduling performance criteria used by companies, since firms with higher scheduling task uncertainty were more concerned with quick and feasible schedule generation than with schedule optimization, whereas companies with lower uncertainty were more focused on schedule optimization.
Finally, Tenhiälä (Citation2011) suggested that the relationship between the complexity of a manufacturing environment, as defined by Hayes and Wheelwright’s (Citation1979) product-process matrix, and the level of detail in capacity planning is not a direct one. Thus, contrary to what Portougal and Robb (Citation2000) suggested regarding scheduling being more applicable in job-shop environments, Tenhiälä contended that job-shops could have a better fit with the simplest capacity planning method of rough-cut planning, whereas the less complex environment of production lines and batch processes with bottleneck control have a good fit with the more detailed level of finite-loading capacity planning, i.e. detailed scheduling.
Thus, 20 years after Crawford and Wiers (Citation2001) questioned whether the scheduling task was a context-dependent or domain-free task—a dichotomy between scheduling being a task that can be described in a general way or specifically to the context in which it is carried out—there is a consensus on the fact that the scheduling task is a task that it is inherently associated with the environment in which it is carried out, since different scheduling approaches are suitable for particular scheduling contexts. This suggests that a deliberate decision should be made to identify the correct fit between the scheduling task at hand and the scheduling approach used to support that task.
Summarizing, the gap between theory and practice in the academic literature on scheduling can be characterized by three issues that are still relevant to this day. synthesizes the three main causes (issues) of the theory-practice gap in production scheduling, as well as the consequences of these issues, the efforts needed to overcome them, and the current state of the gap in terms of each issue.
Table 1. Summary of causes (issues) for the theory-practice gap in production scheduling academic literature.
The common simplification of scheduling problems (issue (a)) by academia (Abedinnia et al. Citation2017) to study more tractable problems (static-deterministic) has resulted in a lack of focus on developing scheduling approaches that could be more suitable for a variety of practical manufacturing environments. This phenomenon has also permeated to industry, where the majority of APS are focused on solving a static-deterministic scheduling problem (Wiers and de Kok Citation2018b; Hvolby and Steger-Jensen Citation2010), producing a lack of both awareness and interest in developing and applying other scheduling approaches that differ from the traditional approach. This reality, in combination with the fact that the traditional scheduling approach is not applicable to all environments (issue (c)) has resulted in the continued existence of the theory-practice gap in scheduling. The fact that the scheduling field still regards the scheduling task as a static decision process and not as an iterative task (issue (b)) has also resulted in a myopic view regarding the lack of applicability of many algorithmic approaches (issue (c)) [by far the most commonly studied scheduling approach in the literature (Romero-Silva and Marsillac Citation2019; Laengle et al. Citation2017)], hindering the opportunity to study scheduling approaches that acknowledge the realities of practical scheduling.
Overcoming these three issues to carry out an applicable scheduling task is a holistic undertaking because an accurate characterization of the scheduling problem (overcoming issue (a)) does not only entail a better description of the resources, jobs, constraints and objectives of the problem, but also of the stability, dynamism and stochasticity of the problem, which are key factors in deciding which level of monitoring support will be needed to execute the practical scheduling task, thus overcoming issue (b). Furthermore, by overcoming issue (b), a better understanding of the needs of the scheduling task in terms of monitoring and control can be obtained in order to find the appropriate level of control support (see, e.g. Romero-Silva and Hernández-López Citation2020; Rossit, Tohmé, and Frutos Citation2019) required for carrying out the scheduling task. However, even if issues (a) and (b) have been addressed, the scheduling task performance will suffer if issues (a) and (b) have not been concurrently considered in selecting a relevant scheduling approach that fits the characteristics of the scheduling task at hand, overcoming issue (c).
Therefore, a study that helps align scheduling approaches with real scheduling environments will not only help improve the applicability of production scheduling theory proposals, it will also highlight the fact that the traditional scheduling approach (static-deterministic) is not relevant for all environments and that more effort is needed to support current, very complex scheduling environments.
This paper is focused on overcoming issue (c) to help close the theory-practice gap in scheduling, as proposals for overcoming this issue have generally been overlooked by researchers. While many studies have highlighted the existence of issue (c), to the best of our knowledge, only the studies by Jonsson and Mattsson (Citation2003), Wiers and Van der Schaaf (Citation1997) and Tenhiälä (Citation2011) have tried to support the efforts in overcoming issue (c) by understanding how planning approaches and environments relate. However, Jonsson and Mattsson (Citation2003) and Tenhiälä (Citation2011) only considered an aggregate level of planning, whereas Wiers and Van der Schaaf (Citation1997) only took into account the level of autonomy on the shop floor (and not specific scheduling approaches) when trying to find a fit with scheduling environments. Additionally, none of these studies considered the different scheduling approaches available in the literature or characterized the manufacturing environments to explicitly find a correspondence between scheduling approaches and manufacturing environments. By suggesting the fit between manufacturing environments and scheduling approaches, this paper will provide practitioners with a framework to select a scheduling methodology applicable to the needs of their specific production units as well as suitable monitoring support.
3. Methodological approach
According to Wacker’s (Citation1998) classification of theory-building research, our research can be classified as an analytical conceptual study, as it tries to build a relational model between the factors that constitute the problem, i.e. the scheduling approaches and the manufacturing environments, and to postulate the effect that applicable and non-applicable scheduling approaches have on scheduling performance.
Regarding the process of good theory-building, Wacker (Citation1998) suggests carrying out four incremental steps: defining variables, defining the domain, building relationships (among the variables) and generating theory predictions. Christensen (Citation2006), on the other hand, proposes that three steps are needed for the process of descriptive theory-building: first, observe, describe and measure the phenomena; second, categorize the phenomena according to their attributes; and, third, make statements of correlation.
We believe that conclusions stemming from the literature can be used to define the domain and variables of our conceptual framework. Furthermore, as Christensen (Citation2006) has suggested that Contingency Theory (CT) can help in the step of categorizing the attributes of the phenomena, we will also use previous studies that take a CT approach regarding planning methods as a basis for identifying the most significant factors regarding the applicability of production scheduling as well as to define some of the relationships between the variables.
The next two subsections present two of the foundational concepts for this research. The first concept, the notion of the practical production scheduling task (PPST), will support the process of defining the domain, whereas the tenets of CT will help us structure the conceptual framework, as we identify its relevant variables and describe the relationships among the variables. Section 4 will develop what Wacker (Citation1998) described as the steps for building relationships and generating theory predictions by postulating propositions that explain the applicability of production scheduling.
3.1. Practical production scheduling task
Various studies have stated that the actual task of production scheduling is not only concerned with solving the combinatorial scheduling problem, but it is also a process that is concerned with the activities of continuously monitoring and controlling the shop floor, among other activities (Berglund and Karltun Citation2007; McKay and Wiers Citation2003; Jackson, Wilson, and MacCarthy Citation2004; Wezel, Jorna, and Meystel Citation2006).
An extended definition of the PPST that considers all the activities of the actual scheduling task was proposed by Romero-Silva, Santos, and Hurtado (Citation2015). This proposed definition of the PPST conceives of scheduling as a continuous feedback loop where the schedule construction/update task and the monitoring task of the manufacturing environment are both critical activities for attaining the desired production objectives; therefore, both activities are intertwined in the PPST. In this conceptualization, the monitoring task is regarded as an activity that is as important as the actual task of building a schedule because it aids in the capacity to continuously identify changes in the state of the system, e.g. variable job arrivals or machine breakdowns, to further adapt the schedule to the actual state of the manufacturing system.
Furthermore, as the PPST is a dynamic task that needs to cope with job arrivals and disturbances in some manufacturing environments, building static and complete schedules is not feasible for all contexts. For this reason, the schedule construction/update activity is an activity in which scheduling agents use different scheduling approaches to cope with different levels of environmental uncertainty, which could lead them to disregard the practice of building complete schedules and use simpler techniques instead.
Based on this definition of the PPST, the scheduling task that is actually executed is highly influenced by organizational and human factors, which transcends the issue of combinatorial optimization and makes it a suitable topic of study for CT.
3.2. Research structure based on contingency theory
Contingency Theory proposes that some managerial practices are more suited to specific contexts since practices are not universally applicable to all contexts. CT suggests that practices are adopted with the aim of improving performance (Donaldson Citation2001), depending on the characteristics of the environment, i.e. contextual factors. Thus, a good fit is achieved when the selected practices produce a good performance.
Since this notion of fit could explain why some scheduling approaches are not suitable for organizing the production of all manufacturing environments, we use it as a theoretical basis for our conceptual framework. We use the classification of operations management (OM) literature using CT proposed by Sousa and Voss (Citation2008) to structure our research design. Sousa and Voss categorize OM studies using CT based on three axes: research variables and measurement of performance, research design, and the approach to studying contingency fits.
In line with previous OM studies using CT (Sousa and Voss Citation2008), we consider operating contexts (manufacturing environment) and organizational practices (scheduling approaches) as research variables while our research design is what Sousa and Voss call an inferential detailed design, meaning that we aim to find specific fits between the research variables (Sousa and Voss suggest that ‘both conceptual and empirical studies may be considered inferential’).
In terms of the approach to studying contingency fits, we consider the systems approach. The systems approach suggests that a good fit can be attained by different combinations of practices and contextual factors rather than linear one-to-one fits due to the complexity of the cross-interactions among the different contextual factors. A good fit will exist as long as the behavior of the organizational system (Romero-Silva, Santos, and Hurtado Citation2018) remains consistent, regardless of the particular changes in the organizational environment and organizational structure, which are the constituent parts of the organizational system.
Thus, companies that have different specific contextual factors and structures but the same global behavior will be considered to be similar in the present study. Following the suggestion in Romero-Silva, Santos, and Hurtado (Citation2018), we will use the concept of organizational systems to identify the types of manufacturing firms instead of considering individual contextual and structural factors.
The outline of the steps that were taken to propose the conceptual framework of the applicability of production scheduling is shown in . First, we define the research variables of our framework by characterizing the manufacturing environments and the scheduling approaches. Based on previous research, we then identify which scheduling approaches could be most suitable to address the PPST of each manufacturing environment. This will provide us with the elements to formulate propositions on the fits between manufacturing environments and scheduling approaches and present our conceptual framework of the applicability of production scheduling. Finally, as a didactical exercise, we describe three case studies exemplifying four different manufacturing environments to illustrate the applicability of the conceptual framework.
4. Conceptual framework of the applicability of production scheduling
From the studies covered in previous sections it can be concluded that the PPST is a highly context-dependent activity. For this reason, the tasks of variable and domain definition in this conceptual framework are activities that are tightly intertwined with the categorization of the phenomenology of the PPST. Therefore, in Sections 4.1 and 4.2 we develop a categorization of manufacturing environments and describe the different scheduling approaches to arrive at a categorization of the phenomena according to their attributes (Christensen Citation2006) and describe the research variables. In Section 4.3 we use the findings of previous studies reporting on the use of different scheduling approaches to identify the fits between manufacturing environments and scheduling approaches and formulate propositions on the fits, where we build relationships among the variables and propose theory predictions (Wacker Citation1998).
4.1. Manufacturing environments characterization
The most common typology used to classify manufacturing environments is the product-process matrix by Hayes and Wheelwright (Citation1979) as it captures a very logical strategic alignment between the demand of a manufacturing firm and the production process carried out to satisfy that demand. Even though it has proven to be a useful classification for modeling the strategic degree of integration between market demands and the configuration of manufacturing processes (Safizadeh et al. Citation1996; Ahmad and Schroeder Citation2002; Tenhiälä Citation2011), some empirical studies have found that the product-process matrix is not able to describe the strategic fits currently existing in the industry (Helkiö and Tenhiälä Citation2013; Kemppainen, Vepsäläinen, and Tinnilä Citation2008). Because of this, we base our categorization of manufacturing environments on a generalization of the product-process matrix proposed by Helkiö and Tenhiälä (Citation2013) who characterize the strategic context of a firm using three dimensions: specificity, complexity of the production task, and dynamism of the production environment.
Process specificity in Helkiö and Tenhiälä’s model accounts for the complexity of the production process flows (layout and flexibility) while the complexity of the production task models product variety as well as product modularity and the complexity of the bill of materials. The dynamism vector considers the rate of change in the operating environment.
Since the scope of this study is focused on the operational level and not the strategic level considered in Helkiö and Tenhiälä (Citation2013), we adapt Helkiö and Tenhiälä’s concepts to agree with the operational level scope considered in the current study. In this regard, we consider the concept of scheduling problem complexity, based on complexity theory (Lenstra, Rinnooy Kan, and Brucker Citation1977), instead of the complexity of the production task. The complexity of a scheduling problem considers variables such as the number of jobs, the production flows, constraints, and scheduling objectives, incorporating notions included in Helkiö and Tenhiälä’s dimensions of specificity (e.g. production flows) and complexity of the production task (e.g. number of jobs, release date constraints). In addition, dynamism was considered by Helkiö and Tenhiälä (Citation2013) on a strategic level. However, in our study, this vector is concerned with the operational level and considers how deterministic (or stochastic) the actual manufacturing process is, e.g. variable processing times, machine breakdowns, random job routings, unplanned order arrivals. Thus, in this study, we consider scheduling problem complexity and dynamism of the system as the two vectors that characterize the manufacturing environments at the operational level.
4.1.1. Dynamism of the system
System dynamism describes the amount of change that a manufacturing system state has over a time period, i.e. the predictability of a system. For example, manufacturing environments that have very few products and carry out fixed production cycles (Tomotani and de Mesquita Citation2018) with very high volumes and little product variety will have a very low degree of dynamism since the state of their production system will be highly predictable. Because of this predictability, it is expected that a shop floor with low dynamism will work under a make-to-stock policy.
On the other hand, highly dynamic systems are unpredictable because their demands have a high degree of variation due to continuous order arrivals or very different product types. In these scenarios, the manufacturing process is also highly unpredictable because the processing and setup times are stochastic and machine breakdowns may occur frequently.
It should be noted that the predictability of the environments could be a direct result of market needs, such as product variety and modularity, but these demand characteristics are not a direct measurement of the dynamism of the manufacturing system. Therefore, system dynamism (Dyn) could be parameterized as a function of some of the following characteristics, taken from Halsall, Muhlemann, and Price (Citation1994) and Tomotani and de Mesquita (Citation2018):
Predictability of demand (Dmd), which can be revealed by the stability of production runs. For instance, fixed production sequences with a make-to-stock strategy result in predictable demand.
Predictability of job routing (Rou), which can be the result of clearly known job/order specifications or fuzzy specifications because of engineer-to-order production.
Randomness of processing and setup times (Stch).
Probability of machine breakdowns (Brk): overall machine efficiency (Hopp and Spearman Citation2000).
Stability of due dates and other constraints (Scnstr), i.e. probability of change for existing constraints.
Job arrivals dynamism (Arr), which can be measured by the effective degree of dynamism proposed in the domain of the dynamic vehicle routing problem (Pillac et al. Citation2013; Larsen, Madsen, and Solomon Citation2002).
Thus, similar to other authors (Ahmad and Schroeder Citation2002; Safizadeh et al. Citation1996; Kemppainen, Vepsäläinen, and Tinnilä Citation2008; Helkiö and Tenhiälä Citation2013), we suggest all these parameters be measured in a linear manner. For example, a linear measure can be implemented to assess the degree of randomness of processing times by measuring the percentage of jobs that have random processing times. If each parameter is operationalized with values between 0 and 1 by setting a range between the minimum and maximum values of the firms in the sample and all the values of the sample are interpolated, then a linear combination of all the weighted parameters can be used to calculate Dyn. For example:
(1)
(1)
where wi for i = 1, 2, 3, 4, 5, 6 is the weight that each parameter has for Dyn, and
(2)
(2)
4.1.2. Scheduling problem complexity
The complexity of the scheduling problem (SCpx) is a vector that is also critical in understanding the applicability of different scheduling approaches because the method selected for executing the PPST of a specific firm needs to correspond with the complexity of the combinatorial problem at hand. This vector has been commonly studied by Complexity Theory in Computer Science by identifying the complexity of scheduling problems according to a complexity hierarchy (see Appendices D and E in Pinedo Citation2016) defined by the type of machine environment, the objective function, and the number of additional constraints found in the problem.
However, since a simple parametrization of SCpx would be very difficult to build from this hierarchy because the characterization is not linear, one way to operationalize this vector would be to consider the notions from Complexity Theory in a linear manner. The following parameters and their corresponding values of complexity (in brackets) could be used to measure SCpx:
Number of individual jobs/orders/batches to be scheduled (Jobs)
Machine environment (ME) in order of complexity:
○Single machine [1]
○Parallel machines, flow-shop, open-shop [2]
○Flexible flow-shop, job-shop [3]
○Flexible job-shop [4]
Number of additional constraints (Cons), e.g. sequence-dependent setup times or due date constraints
Scheduling objective (Obj) in order of complexity:
○Total completion time [1]
○Maximum lateness, sum (average) of cycle times [2]
○Weighted sum (average) of cycle times, sum (average) of tardiness, sum of number of tardy jobs [3]
○Weighted sum (average) of tardiness, weighted sum of number of tardy jobs [4]
○Multi-objective: sum of the scheduling objectives
Similar to what we proposed with Dyn parameters, SCpx parameters could be operationalized with values ranging between 0 and 1 by linearly interpolating the values between the minimum and maximum range, depending on the sample. For instance:
(3)
(3)
where vi for i = 1, 2, 3, 4 is the weight that each parameter has for SCpx, and
(4)
(4)
It is worth noting that there are many different alternatives for the parameterization of Dyn and SCpx, even if we consider the same parameters shown in (1) and (3). For instance, the value of Cons could also depend on values associated with the difficulty of handling the constraints that are part of the environment as tight due dates and sequence-dependent setup times create significantly more challenging scheduling problems than shop floors with loose due dates and permutation constraints.
4.1.3. Manufacturing environments classification
The classification of shop floors proposed by Wiers and Van der Schaaf (Citation1997) acknowledges the interaction between the dynamism of the environment (uncertainty) and to some extent incorporates the complexity of the environment by taking into account the need to adapt to the dynamism and complexity of the environment by giving autonomy to the shop floor. Thus, their classification of shop floor types can be used to capture the different combinations of dynamism and complexity that are relevant for identifying applicable scheduling approaches. However, instead of considering shop floor autonomy, we consider scheduling complexity as one of the two main vectors that characterize a shop floor system, in addition to the dynamism (or uncertainty) of the system, in order to classify manufacturing environments types only by their characteristics and not by the scheduling approaches needed to operate those systems.
describes the four manufacturing environment types and some of their representative characteristics, including the expected general magnitudes for Dyn and SCpx.
Table 2. Manufacturing environment types and characteristics.
This classification of manufacturing environments and its parameterization into two vectors constitute the variable and domain definition that will help describe the phenomenon of production scheduling applicability.
4.2. Scheduling approaches
In this study, we consider a scheduling approach as any method that helps to organize the production at the shop floor level, even if no specific schedule is built. Next, we give an overview of scheduling approaches based on the general classifications proposed by Vieira, Herrmann, and Lin (Citation2003) and Ouelhadj and Petrovic’s (Citation2009), and on the study of computer-based manufacturing scheduling tools by Dios and Framinan (Citation2016).
4.2.1. Control-theoretic approaches
The basis of this approach is to control the flow entering or moving through the shop floor by imposing a production rhythm, irrespective of which job is arriving or being processed. Examples of control-theoretic techniques are Kanban, CONWIP (CONstant Work in Process) and DBR (Drum-Buffer-Rope) (see Jodlbauer and Huber Citation2008 for an overview). Due to the limited informational needs and straightforward mechanisms of these methods, control-theoretic techniques can be more easily implemented in the daily operations of shop floors and directly improve performance, as demonstrated by several case studies (Mukhopadhyay and Shanker Citation2005; Pegels and Watrous Citation2005; Leonardo et al. Citation2017).
4.2.2. Predictive approaches
Predictive scheduling is the subject of traditional scheduling theory (Conway, Maxwell, and Miller Citation1967) as its focus is on building schedules by assigning jobs to capacity-constrained resources and allocating resource time-slots to each job, solving a static-deterministic problem. Predictive scheduling assumes that disturbances or incoming jobs will not change the predicted execution of the schedule (see, e.g. Framinan and Perez-Gonzalez Citation2015; Shen, Dauzère-Pérès, and Neufeld Citation2018); consequently, no constant monitoring of the shop floor status is needed for this approach. Algorithmic techniques using exact (Blazewicz, Dror, and Weglarz Citation1991) and heuristic (Ruiz Citation2016) methods are used to build these detailed schedules.
4.2.3. Reactive approaches
Reactive scheduling tries to cope with high levels of environmental uncertainty by refraining to build complete schedules. Instead, with reactive scheduling one generates job processing sequences on each resource and/or controls which jobs are released to the shop floor. Complete, detailed schedules are not built with this approach but sequencing lists are dynamically created/updated for each resource every time a job arrives on the shop floor or a resource queue. Dispatching/priority rules (see, e.g. Romero-Silva et al. Citation2018; Xiong et al. Citation2017) and workload control techniques (see, e.g. Gómez Paredes et al. Citation2022; Thürer, Stevenson, and Silva Citation2011) are reactive methods addressing dynamic scheduling problems, either deterministic or stochastic. While some of these techniques are easy to implement due to the limited amount of data needed to operate them [e.g. the SPT rule (Qi, Bard, and Yu Citation2006)], some others require continuous monitoring of the shop floor status (see, e.g. Branke, Hildebrandt, and Scholz-Reiter Citation2015), which could limit their usability.
4.2.4. Predictive-reactive approaches
Predictive-reactive scheduling generates complete schedules that are constantly subject to revision by different rescheduling policies, e.g. periodic or event-driven rescheduling, to cope with disturbances in the shop floor status and with job arrivals (see, e.g. Framinan, Fernandez-Viagas, and Perez-Gonzalez Citation2019; Dong and Maravelias Citation2021). Predictive-reactive scheduling needs a feedback loop between the shop floor monitoring system and the scheduling module to be able to update the schedules in the face of disturbances or job arrivals, limiting their applicability in certain manufacturing environments with low monitoring capabilities. Furthermore, because of the dynamic and possibly stochastic nature of the problems faced by predictive-reactive approaches, simulation might be used to test the feasibility of the schedule instances (Lin and Chen Citation2015) or as a platform to test complex operating rules (Jeong and Kim Citation1998).
4.2.5. Scheduling performance
A scheduling approach that has a good fit with a specific manufacturing environment will produce the best performance for the manufacturing firm. Scheduling theory has considered many objectives related to either the optimal utilization of resources (e.g. minimize idle times, minimize the makespan, minimize costs) or the optimal delivery performance (e.g. minimize tardiness, lateness or number of tardy jobs). On top of aiming to optimize their delivery and resource performance (Romero-Silva, Hurtado, and Santos Citation2016), manufacturing firms are also concerned with keeping quality and inventory levels in check, reaching their service agreements, and even having a smooth and stable production (Tomotani and de Mesquita Citation2018).
Thus, performance in the case of this conceptual framework is concerned with the performance of the shop floor over the long run, including any performance goal(s) that are relevant for the manufacturing firm.
4.3. The fit between manufacturing environments and scheduling approaches
Previous studies have already proposed fits between manufacturing environment types and some planning methods (Jonsson and Mattsson Citation2003; Tenhiälä Citation2011; Wiers and Van der Schaaf Citation1997)—but not for scheduling approaches—where it has been shown that the ‘one-size-fits-all’ approach is not applicable in the case of planning activities. In this section, we extend those previous conclusions into the domain of the PPST by proposing fits between manufacturing environments and scheduling approaches that will result in a better performance of the PPST.
Previous studies that focused on the implementation of control-theoretic techniques, such as Kanban (Mukhopadhyay and Shanker Citation2005) and DBR (Benavides and Van Landeghem Citation2015), in companies with low product variety and simple flow shops with no additional constraints (low Dyn and SCpx values) have found that the implementation of control-theoretic methods has significantly improved the performance of their selected case studies. So very simple manufacturing environments only need simple control techniques to manage their production operations. From this, we can formulate that:
Proposition 1:
Control-theoretic approaches fit the scheduling requirements of Social shops.
As the Social shop turns into a Socio-technical shop owing to greater dynamism and low scheduling problem complexity, it would also need a more dynamic scheduling approach to deal with the constant changes in the state of the shop floor. In this regard, Hendry, Huang, and Stevenson (Citation2013) suggest that Work Load Control (WLC) techniques are suitable techniques to use in Socio-technical systems. Furthermore, dispatching rules (Rajendran and Holthaus Citation1999) have also been found to be very useful for scheduling very dynamic environments, such as semi-conductor wafer fabrication factories (Johri Citation1993; Münch, Fowler, and Mason Citation2013; Cigolini Citation1999). Since some studies (Lu, Huang, and Yang Citation2011; Slotnick Citation2011) have found that shop floor performance depends on a correlation between the use of different WLC techniques and dispatching rules, the use of simple WLC policies and simple dispatching rules are suitable approaches for addressing the PPST in Socio-technical shops. Therefore, we state that:
Proposition 2:
Reactive approaches fit the scheduling requirements of Socio-technical shops.
Transitioning from a stable and simple shop to a shop floor that has a more complex scheduling problem but a non-dynamic environment will create the need for a more accurate approach that can deal with the complexities of the problem. In this case, exact algorithmic methods, such as Mixed-Integer Linear Programming (Pinedo Citation2016), can be used to schedule and optimize production as the demand is highly predictable and jobs/orders could be planned in a static manner (see, e.g. Kaçmaz, Alakaş, and Eren Citation2019; Zanda, Zuddas, and Seatzu Citation2018). However, if the scheduling problem becomes more complex because of a more complex machine environment or the presence of additional constraints, heuristic methods (Ruiz and Stützle Citation2007) may be needed to build feasible and well-performing schedules, even though they are not optimal.
On the other hand, when SCpx is high and Dyn starts to increase to an intermediate level, a more flexible scheduling method is needed to cope with the constant rescheduling and schedule repairs. Thus, for this type of system, constraint-based programming could be more applicable (Hvolby and Steger-Jensen Citation2010; Salido, Garrido, and Barták Citation2008; Novas and Henning Citation2010) in building or repairing complete, feasible schedules in the presence of continuous changes in the state of the system. Based on this, we suggest that:
Proposition 3:
Predictive approaches fit the scheduling requirements of Smooth shops.
Very dynamic systems with intermediate levels of SCpx need more complex scheduling techniques than simple WLC and dispatching rules because of the presence of particular constraints. For instance, shop floors with dynamic environments and sequence-dependent setup times require specialized and specifically designed dispatching rules to cope with this particular constraint (see, e.g. Sharma and Jain Citation2014; Pickardt and Branke Citation2012; Lee, Bhaskaran, and Pinedo Citation1997). Moreover, the hyper-heuristic development of dispatching rules (Pickardt et al. Citation2013; Branke et al. Citation2016; Korytkowski, Wiśniewski, and Rymaszewski Citation2013), which are specifically designed for particular manufacturing plants, is also an applicable scheduling approach for this type of complex and dynamic system.
Finally, the most complex system to schedule, i.e. highly dynamic and complex Stress shops, needs the most sophisticated approaches to carry out the PPST. For these systems, expert systems with either knowledge-based rules (López-Santana and Ramiro Citation2016) or machine learning (Priore et al. Citation2014; Aytug et al. Citation1994) and simulation-based scheduling (see, e.g. Parthanadee and Buddhakulsomsiri Citation2010; Yang, Kuo, and Chang Citation2004) can be a good fit since coping with these realities requires a thorough understanding of the interactions among the various possible system states (due to the high dynamism and complexity) and scheduling methodologies. Thus:
Proposition 4:
Predictive-reactive approaches fit the scheduling requirements of Stress shops.
summarizes the proposed fits by plotting the scheduling techniques that fit different manufacturing environments according to their Dyn and SCpx values. It is worth noting that in we illustrated specific scheduling techniques instead of general scheduling approaches because some scheduling techniques can be categorized as two different scheduling approaches depending on the monitoring capabilities used by the shop floor. For instance, constraint-based programming can be regarded as a predictive approach to solve a static and deterministic problem or as a predictive-reactive approach to solve a dynamic and deterministic problem. In addition, since the practical scheduling task is not only concerned with building feasible schedules but also with monitoring and control, different scheduling approaches need different levels of monitoring support in order to be executed. For instance, control-theoretic methodologies only need information about the general amount of work-in-process (WIP) and the global output of the system; whereas simple dispatching rules and static-deterministic schedules (exact and heuristic algorithms) need information about the input (arriving jobs), WIP (jobs already scheduled and in-process) and output (completed jobs) to be able to build schedules.
More complex environments, on the other hand, need a higher level of monitoring because the high Dyn and SCpx require a continuous update of information regarding job arrivals and characteristics, machine status (availability in terms of breakdowns and queues), and job traceability (needed because of alternative routings, stochasticity of processing and setup times, and queueing).
The monitoring needs of each of the seven scheduling methodologies identified in are summarized in , where we suggest the level of monitoring needed to support the specific scheduling approach required for a particular manufacturing environment.
These proposed fits define the relationships among the variables of the phenomenon of production scheduling applicability and describe a part of what can be regarded as theory predictions, as we are suggesting that the performance of scheduling tasks will depend on a correct fit. However, we think that another layer of variables and interactions is needed to completely predict the phenomenon of the applicability of practical production scheduling. We develop such a layer in the next section to further generate the theoretical predictions of the conceptual framework.
4.4. Complexity of the PPST
Even though we characterized the scheduling problem complexity (SCpx) that schedulers face in their daily operations, that variable only accounts for the complexity of the combinatorial problem at hand. However, the dynamism of the environment creates an additional layer of complexity to the PPST as the scheduler faces continuous changes in the state of the shop floor. To account for this overall complexity, we consider an additional research variable called complexity of the PPST (PPSTCpx). From the characteristics described in , it can be seen that Stress shops are the most complex manufacturing environments to schedule because of their particular combination of high Dyn and high SCpx, while Social shops have a very simple PPST. Thus, the vectors Dyn and SCpx can be used to assess PPSTCpx in the following manner:
(5)
(5)
The two vectors constituting PPSTCpx have a quadratic term because we wanted to model how higher values of Dyn and SCpx have a much greater impact on PPSTCpx than lower values in order to represent the greater effort needed to schedule those environments. Furthermore, since both Dyn and SCpx have a range of values between 0 and 1 for any given sample of manufacturing firms, PPSTCpx = 2 represents a straightforward way of identifying a manufacturing environment with the highest PPST complexity, while PPSTCpx = 0 represents a manufacturing environment with no complexity; for this reason, the PPSTCpx term in Equationequation (5)(5)
(5) is not modelled as a quadratic term.
A final consideration regarding PPSTCpx is that it is not linearly related to the manufacturing environment types previously described, as this match depends on the combination of values for Dyn and SCpx, i.e. four quadrants with high-low values for Dyn and SCpx. However, PPSTCpx is directly related to the complexity of the scheduling approach that is needed to solve the PPST because firms with higher PPSTCpx will need more sophisticated methods for scheduling, as the next section will explain.
4.5. Compounding effects of capacity utilization and fit on performance
Sousa and Voss (Citation2008), basing their work on Strategic-Choice Theory (Child Citation1972), suggested that some companies do not need to use fitting practices to reach good levels of performance because they use slack capacity, which creates a margin of operational execution for managers. We pick up this idea and propose that the effect of resource capacity utilization, influenced by the fit of scheduling approaches, is highly significant in the performance of manufacturing systems. We consider utilization as an additional relevant research variable because utilization is a factor that characterizes the operating conditions of a shop floor in a straightforward manner by reflecting various contextual factors in the shop floor, such as the capacity, the demand rate, and even the effect of some constraints, in one single value. Utilization is normally measured as the percentage capacity utilization of the bottleneck(s) (Chakravorty and Atwater Citation2006; Roser, Nakano, and Tanaka Citation2002), or in the case of very dynamic shop floors with no clear bottleneck, the average capacity utilization of the shop floor (Bertrand and Van Ooijen Citation2002; Bertrand Citation1983).
In this regard, Queueing Theory (Gross et al. Citation2008) shows that the performance of stochastic systems exponentially deteriorates as resource capacity utilization (ρ) approaches 100%, a behavior that is very clearly represented by Throughput-Cycle Time curves (Yang, Ankenman, and Nelson Citation2007). We argue that the effect of the fit on performance also depends on the values of ρ, as it has been shown that sequencing methods have a bigger impact on performance when utilization is high (Romero-Silva et al. Citation2018; Romero-Silva and Hernández-López Citation2020). For instance, a bad fit will be irrelevant when resources are under-utilized but it will have a high impact on performance when the resources have very little slack, resulting in additional exponential deterioration of performance. This compounding effect that resource capacity utilization and fit have on performance could be greatly intensified by higher PPSTCpx values since a bad fit in highly complex scheduling tasks will produce much worse results than in moderately complex PPSTs.
Therefore, there is a triple interaction among (a) a good fit between the manufacturing environment (located using the ‘coordinates’ of Dyn and SCpx) and the scheduling approach; (b) the scheduling task complexity, described by the sum of squares of Dyn and SCpx; and (c) resource capacity utilization percentage (ρ). We contend that this complex interaction describes the performance of the scheduling task in practical terms. This completes the last step in the conceptual model building (generate theory predictions). Therefore, we propose the following:
Proposition 5:
The scheduling performance of a manufacturing firm is influenced by a compounding relationship among (a) the fit of scheduling approaches and manufacturing environments, (b) the complexity of the scheduling task, and (c) capacity utilization.
This proposition suggests that the relevance of Scheduling Theory on scheduling practice can only be assessed through the lens of Contingency Theory since it is only through identifying a fit between scheduling approaches and manufacturing environments that one could assess the applicability of scheduling proposals. Moreover, the proposition represents a good starting point for predicting the impact of the applicability of production scheduling on the relative performance of manufacturing firms.
It is worth noting that the utilization of resources is not constant at all times, particularly in markets where the mean demand rate is variable (Cheevaprawatdomrong and Smith Citation2004; Boysen, Bock, and Fliedner Citation2013). Thus, the relevance of a fitting scheduling approach will vary over time, depending on the utilization levels. On the other hand, the constitutional vectors of the manufacturing environment (Dyn and SCpx) are factors that seldom vary over time, as they are constituent parts of the structure of the system, which may be more difficult to change (Romero-Silva, Santos, and Hurtado Citation2018).
5. Case studies illustrating the relevance of the conceptual framework
To exemplify how certain scheduling approaches might be more effective in addressing the PPST of different manufacturing environments, we present three representative companies with different manufacturing environments: a Smooth shop (case A, presented in Section 5.1); a company with two very different production units, namely, a Social shop (case B1) and a Stress shop (case B2), both presented in Section 5.2; and a Socio-technical shop (case C, presented in Section 5.3). Along with the description of the characteristics of the manufacturing environment for each case study, we also use our conceptual framework to propose which scheduling approach could have the best fit to address the scheduling task of each case study.
These three companies were considering installing a commercial APS in order to support their scheduling task and they were part of a larger study where the scheduling needs and manufacturing environments of 50 companies (see the Appendix for an overview of the manufacturing environments characterization of the shop floors in that sample) were investigated (see Romero-Silva, Hurtado, and Santos Citation2016 for more details on the data collection approach of the case studies). These companies were selected because they embody different manufacturing environments and scheduling needs, and because of their willingness to be subject to a more detailed analysis, as they were assessing the applicability of using an APS.
5.1. A make-to-stock/make-to-order company producing bricks
The first example (case A) is a case of a firm producing bricks for furnace walls at steelworks. The manufacturing process consisted of five stages, but the most critical step was pressing the powder material into bricks. There were five unrelated parallel (Jungwattanakit et al. Citation2008) presses. The scheduler assigned to each press a sequence of batches, depending on the materials and the dimensions of each batch. No specific assignments were made for any other resources, as the sequence used in the presses was carried over the other resources.
The most important feature of this scheduling problem was that, if the dimensions or materials deviated from a previous batch, a setup/changeover time was needed. The bigger the deviation was, the longer setup time was needed. This is commonly described in scheduling theory as sequence-dependent setup times (Allahverdi, Gupta, and Aldowaisan Citation1999). In addition, the company worked under a combination of make-to-order and make-to-stock strategies. Some of their demand was stable enough to forecast with some certainty but a portion of the orders arrived without notice and was too specific in terms of dimensions to be predictable. Taking these characteristics into account, the scheduler organized the weekly production by combining make-to-stock and make-to-order batches of similar materials and dimensions into the same press and time slots. The scheduler tried to find a balance between maximizing the service level in the make-to-stock market and minimizing the number of tardy jobs in the make-to-order market. Unplanned events, such as rush orders or machine breakdowns were rescheduled on a daily basis.
Thus, in this production line, Dyn was low and SCpx was very high, i.e. a Smooth shop. These values would place this company in the bottom-right corner of , suggesting that a scheduling approach based on heuristics or metaheuristics will be fitting for this environment. Since this manufacturing environment is very heavily dependent on sequence-dependent setup times, a heuristic procedure that takes into account this constraint (see, e.g. Lee, Bhaskaran, and Pinedo Citation1997; Lee and Pinedo Citation1997) is an applicable methodology for this company.
Regarding its monitoring needs, this company needs information about the incoming jobs, the overall WIP, and the production output because the applicable scheduling approach in this production line does not need a full online monitoring system to track every single step of the process or the full updated status of the shop.
5.2. Production of refrigeration industrial equipment
This firm produced industrial equipment for refrigeration tasks in two different lines, one for standard equipment and the other for completely customized, engineered-to-order (Gosling and Naim Citation2009) equipment. Since the first line (case B1) produced four standard products, production was organized in an assembly line. The four standard products were produced in constant cyclic production runs where no scheduling needed to be carried out. Case B1 exemplifies a Social shop. Only a basic production control was needed for this line. Thus, only the daily production output was measured because Dyn and SCpx were very low (bottom-left corner in ). For case B1, a good fit was irrelevant for the performance of the production unit as both Dyn and PPSTCpx were zero.
The other production line (case B2) was a little more complex as it produced engineered-to-order equipment where each order was unique. Order sizes were commonly 1, but some orders included up to 4 pieces of equipment. Some orders required two days to be completed, while other orders required four weeks, so there was a large disparity in the manufactured products. There were 7 possible stages for this production line, but each order required different routings, depending on the characteristics of the equipment. In addition, each stage could be completed by multiple operators/machines, so the flow pattern was characterized as a hybrid job shop. Processing times for some operations, e.g. welding, cutting, and fabrication, were long and stochastic, because of the intricacies of building completely unique components.
Despite this, job arrival dynamism was not very high, as the potential market was small and the total flow time of orders was long. Furthermore, capacity constraints were difficult to predict since the bottleneck of the system depended on the mix of orders and some assembly operations could be carried out concurrently by two workers, which is a characteristic not normally taken into account by traditional scheduling approaches. Thus, there was a complex interconnection between worker and task assignments, which complicated the scheduling task.
For this production line, the scheduler was concerned with minimizing the number of tardy jobs, while taking into account the complexity of worker and task assignments, multiple precedence constraints and concurrent operations. In this case, Dyn was moderate and SCpx was relatively high, resulting in a moderate value of PPSTCpx, which places this company in the middle-right position in . Case B2 is a representative example of a Stress shop.
Mainly due to the complexity of the constraints, but also due to the constant rescheduling efforts needed to adjust for stochastic processing times, we argue that this production line has a good fit with constraint-based programming scheduling. Moreover, since most commercial APSs generate schedules with constraint-based programming (Hvolby and Steger-Jensen Citation2010), this production line is one of the companies that could actually take advantage of using a commercial APS. From we can see that this company needs an online system that is able to acquire data about the progress of the jobs at each station in order to schedule incoming jobs or reassign workers, mainly because of unexpected lengths of processing times (stochasticity).
The impact of using a fitting scheduling approach in this production line is important because both capacity utilization and PPSTCpx are high.
5.3. Production of pumps for different markets
The third example (case C) is a company that produced make-to-order (from a standard catalogue of products) and engineered-to-order pumps in small to medium batches. Due to the high variety of pump dimensions, materials and applications, this firm had very different, alternative machines for each stage of the manufacturing process, which consisted of five stages (all pumps completed the same flow pattern). Thus, this plant had excess capacity to be able to deal with the high mix of products and volumes. Since this company worked under a make-to-order strategy, its main objective was to fulfil the orders on time (minimize the number of tardy jobs). The plant was equipped with a Manufacturing Execution System (MES—Meyer, Fuchs, and Thiel Citation2009), which allowed the workers to register all the starting and finishing times per job and allowed management to have complete traceability of jobs and worker productivity.
This company’s management team wanted to install an APS to have better worker assignments (to increase productivity), as this task was assigned manually by the scheduler in a spreadsheet. However, since the Dyn of this company was very high and the SCpx was low (top-left corner in ), a full, detailed schedule was not needed to successfully carry out production in this firm. This is even more evident when taking into account that both machine and worker capacity utilizations were not high, limiting the need to implement tight schedules. Therefore, there was enough capacity slack (designed to have increased flexibility) to be able to organize production with simple dispatching rules, e.g. SPT (Shortest Processing Time) or AVPRO (Average Processing Time) (Jayamohan and Rajendran Citation2000).
Interestingly, this company did not require the level of shop floor monitoring that was already installed in the MES to carry out an effective scheduling approach, since implementing simple dispatching rules only requires input, WIP and output information (see ) and not an online system. Moreover, using a fitting scheduling approach for this environment would not be as critical to the production performance of this company as it would be for other more highly utilized companies because of its slack capacity. Scheduling performance will increase if they use SPT or AVPRO instead of a control-theoretic approach, e.g. CONWIP, but we contend that it will not be critical to reach a good production performance because of the low capacity utilization of the shop floor.
6. Research implications
We believe that the two principal vectors—dynamism and scheduling problem complexity—proposed in this paper for the characterization of manufacturing environments can be very useful to identify the scheduling approaches needed to better support the scheduling task of different manufacturing environment types and to provide a good measure, through their combined effect, for estimating the complexity (and effort) of carrying out the practical production scheduling task. In this regard, this paper provides a framework to help increase the applicability of scheduling research by formulating propositions on the best fits between scheduling approaches and manufacturing environment types, and provides three case studies exemplifying such fits.
As other authors have suggested, traditional scheduling theory, i.e. deterministic and static scheduling, is not applicable in every context, but it is relevant in some specific scheduling scenarios where dynamism is low and scheduling complexity is high. Such a scenario was presented in Section 5.1.
While traditional scheduling theory has been closing the gap with practice in the last 20 years by incorporating more relevant features into its proposals, as the studies by Fuchigami and Rangel (Citation2018) and Harjunkoski et al. (Citation2014) show, more research is needed in the field of production scheduling that considers more realistic complex environments, such as the ones found in Stress shops. A representative example of a Stress shop was described in Section 5.2 (case B2), where we suggested that a good fit for this manufacturing environment, which had high scheduling problem complexity and moderate dynamism, was constraint-based programming scheduling, which is the approach normally implemented in APS. Furthermore, we highlighted the fact that, to be able to carry out this scheduling approach, this company would need to be supported by an online monitoring and control activity since it will need frequent updates on the status of the manufacturing environment. Thus, the conceptual framework presented in this study also stresses the importance of considering the monitoring capabilities of production lines—issue (b), the simplification of the practical scheduling task—in practical scheduling studies, particularly since it has been suggested (Romero-Silva, Hurtado, and Santos Citation2016) that very few companies possess the monitoring capabilities needed to support complex scheduling approaches.
In addition, some approaches directly related to the practice of scheduling, such as control-theoretic techniques and WLC and dispatching rules, can perform the task of supporting the execution of the PPST in other system types that are ill-suited to be supported by the traditional scheduling approach. For instance, the Socio-technical shop described in Section 5.3 (case C), with high dynamism but low scheduling problem complexity, had a manufacturing environment that is suitable for using simple WLC and dispatching rules; whereas a very simple control-theoretic technique can be used for case B1 (presented in Section 5.2) due to its very low complexity and dynamism. Case C also highlights the fact that some companies might not need to have an online monitoring system to carry out their scheduling task, as a lower level of monitoring is sufficient to support the suitable scheduling approach. Thus, at least in terms of scheduling needs, some companies might save some resources in terms of monitoring capacity investment (Romero-Silva and Hernández-López Citation2020) by matching scheduling and monitoring needs.
We also think that the implications of Proposition 5, which describes the compounding effect that resource capacity utilization and fit have on scheduling performance, could be very relevant in the current market context because many firms could either elect to have a higher capacity slack in order to focus on a customer-responsive strategy (Schonberger and Brown Citation2017) or follow a cost-oriented strategy through focused factories (Ketokivi and Jokinen Citation2006) with high workloads to maximize resource capacity utilization and reduce operating costs.
According to this proposition, the performance of shop floors electing the cost-oriented strategy will greatly depend on selecting a fitting scheduling approach, whereas shop floors that have adopted a customer-responsive approach will be less dependent on having a good fit because of an increased slack capacity. The influence of a good fit on the performance of (focused) factories with high capacity utilization percentages could be even more significant if the company elects to have operational excellence as a competitive advantage since the scheduling task has a highly significant effect on the resulting performance of a firm’s operations.
Thus, it is only through the lens of Contingency Theory that we can understand the applicability of production scheduling because the scheduling performance of shop floors does not depend on using the most complex scheduling technique but the most fitting. This study follows the tradition of OM studies using an inferential detailed design in Contingency Theory by identifying the operational contexts in which certain practices (scheduling approaches) will work best in terms of scheduling performance through a systems-based approach to fits.
Paradoxically, as we present this conceptual model, there is evidence that the industry is trending towards more under-utilized scenarios on a global scale, as the utilization percentage for the manufacturing sector capacity in the US has steadily declined to a level of around 75% in recent years (Board of Governors of the Federal Reserve System Citation2022a)—probably due to an increase in flexibility, as the total production capacity has also significantly increased (Board of Governors of the Federal Reserve System Citation2022b). However, companies with variable and seasonal mean demand rates could still find that a good fit is relevant to their operations, despite having a non-critical average annual capacity utilization percentage, because surges in demand will produce higher utilization scenarios where the scheduling task could greatly influence performance.
Additionally, companies electing a customer-responsive strategy are faced with higher dynamism because of the unpredictability of demand. To accommodate that demand, they need more complex manufacturing processes. This combination of two realities results in greater complexity in the practical production scheduling task, which we contend also has a very significant influence on the relevance of having a good fit to have a good performance.
Therefore, manufacturing firms could use this conceptual framework (summarized in and ) to support the decision-making process regarding whether to invest in a fitting scheduling approach and monitoring system, depending on the relevance and perceived improvement in performance that would come from implementing such an approach.
Finally, even though the objective of this paper was to help to close the theory-practice gap by overcoming issue (c), i.e. lack of relevance of the traditional scheduling approach to all manufacturing environments (see ), some researchers could be motivated by the current study to further investigate issues (a) and (b) due to the need to empirically test which parameters in the scheduling environment characterization are actually relevant for different scheduling approaches. The conceptual framework proposed in this study sheds some light on the need to develop more methodologies that are fitting for a variety of scheduling environments, particularly very dynamic and constrained environments, since it explicitly acknowledges that traditional scheduling theory is only applicable to a small portion of companies. This highlights the need to further close the gap in terms of issue (a).
Overall, we believe that the propositions presented here could constitute a point of departure for a future research agenda to help to close the theory-practice gap in practical production scheduling research.
7. Conclusions
The objective of this study was to propose a conceptual framework of the applicability of production scheduling. Since previous studies have suggested that the practical production scheduling task is closely associated with the manufacturing context, we applied the constructs of Contingency Theory to explain that scheduling is only applicable when particular manufacturing environment types are matched with fitting scheduling approaches. Based on previous literature, we provide an overview of the theory-practice gap in scheduling and suggest that this gap has been caused by three issues: (a) a simplification of scheduling problems, (b) a simplification of the practical scheduling task as a decision process, and (c) the lack of relevance of the traditional scheduling approach to all manufacturing environments.
Furthermore, we argue that manufacturing environments can be characterized by the dynamism of the state of the system and the complexity of the scheduling problem when considering the applicability of production scheduling, and that the combination of both factors, in turn, results in a measurement of scheduling task complexity. This characterization of a manufacturing environment is then used to identify which scheduling approaches are most suitable for different manufacturing environments and what level of monitoring support will be needed to execute the fitting scheduling approach. To exemplify this proposal, we presented three cases studies of manufacturing firms with different characteristics and scheduling needs.
Finally, we suggest that the relevance of a fitting scheduling approach on the performance of a firm depends on the overall capacity utilization of the resources in the firm, as companies with under-utilized resources would not necessarily require a fitting scheduling approach because of available capacity slack. On the other hand, the performance of companies with high levels of resource utilization will be more dependent on using a fitting scheduling approach, as highly utilized factories are more sensitive to the use of scheduling methodologies and could have a smaller margin in which to conduct sub-optimal operations.
7.1. Limitations of the study
The most significant limitation of this study is the lack of empirical support. As both Wacker (Citation1998) and Christensen (Citation2006) suggest, producing evidence of any conceptual model is a necessary step. While we based our results on the parameterization of real companies using the cases of three manufacturing firms in terms of Dyn, SCpx and PPSTCpx, the information about their range of operating utilization values was only a rough estimate. Moreover, no information was obtained regarding the actual selection of scheduling approaches by each company and the performance of such companies was not measured. Thus, only suggestions regarding the fitting scheduling approaches and a hypothesized model of the relative performance were provided.
Even though more empirical research is needed to support our propositions, the logical structure of our proposals, which are based on a synthesis of previous studies, is thorough and the proposals can be used as a starting point for studying the issue of production scheduling applicability and further proposing a normative theory. Furthermore, the case studies presented here provide interesting examples of the relevance of our conceptual model and serve as a motivation for other researchers to study this phenomenon further.
In addition, despite the fact that we have presented a broad picture of how to model the two vectors that characterize the manufacturing environment types, the parameterization of these vectors (illustrated in the Appendix), was only an exercise conducted for exemplification purposes, since the sample was not designed to specifically collect all the data needed to parameterize Dyn and SCpx. Moreover, we did not explicitly define the actual Dyn and SCpx values that a firm should have in order to classify it as a certain type of manufacturing environment.
It is worth noting that we only considered the combined effect of resource capacity utilization and fit on the scheduling performance, while firm performance considerations were left out. It is possible that in some environments the profits generated by an increase in performance due to increased revenue caused by better service and due to reduced costs do not make up for the costs of implementing a fitting scheduling approach (see, e.g. Romero-Silva and Hernández-López Citation2020; Kjellsdotter and Jonsson Citation2010).
7.2. Future research opportunities
More empirical research is needed to validate Propositions 1–4, which are summarized in and , and Proposition 5. Thus, a cross-sectional multiple-case-study/survey is needed to assess the relevance of each proposed parameter in terms of the characterization of the manufacturing environment types, i.e. how important each parameter is for describing Dyn or SCpx. A selection of companies with different scheduling environments and different scheduling approaches will be needed to assess the performance of different environment-approach combinations, as this study only presented examples of four production units and did not empirically test the propositions. While the four production unit examples presented here provide the reader with clear examples of how to identify a good fit between a manufacturing environment and a scheduling approach (and monitoring capabilities), they only illustrate a limited number of scheduling approaches, so future research should also focus on including all the scheduling approaches presented here.
It is worth noting that even though we proposed expert systems to solve the most complex manufacturing environment type of Stress shops, expert systems have proven to be very difficult to implement on real shop floors (Kerr Citation1992) and have fallen into disuse in recent years in this field (Romero-Silva and de Leeuw Citation2021). However, the technological developments over the last years in terms of the supervision and control of manufacturing systems, e.g. Manufacturing Execution Systems (Meyer, Fuchs, and Thiel Citation2009) and Smart Factories (Kusiak Citation2018; Mittal et al. Citation2018), along with recent advances in Machine Learning (Jordan and Mitchell Citation2015), could be very helpful in providing expert systems with a better technical framework for supporting the PPST. Thus, future research could focus on the feasibility that expert systems could have for supporting the scheduling task in the current technological and organizational context.
To empirically test the validity of this conceptual framework, future research will need to design a questionnaire specifically designed to capture data regarding the parameters of Dyn and SCpx. The complexity of this type of data requires that the questionnaire be administered on-site, at the plant facilities, so the researcher has first-hand knowledge about the manufacturing process and the scheduling task (see, e.g. Kemppainen, Vepsäläinen, and Tinnilä Citation2008; Romero-Silva, Hurtado, and Santos Citation2016). Results from the questionnaire will provide insights into the relevance of each parameter (see Sections 4.1.1 and 4.1.2) in defining manufacturing environment types (see Section 4.1.3) as well as providing information about the weight values (wi and vi) associated with each parameter. With this information, then the manufacturing environment type of each company can be identified to measure and assess its performance (e.g. lead times, due date fulfilment, or service levels) resulting from the scheduling approach used by the company. Furthermore, information about the shop floor capacity utilization needs to be included in future studies to investigate whether capacity utilization in fact plays a role in the relevance of the fit between scheduling environments and scheduling approaches.
The empirical validation of the conceptual framework can also be carried out through longitudinal case studies using action research, where researchers can first study the performance of a company under a scheduling approach previously selected by the firm to subsequently implement a more suitable scheduling approach (based on ) and measure its performance for the same manufacturing environment. Both cross-sectional and longitudinal case studies can be carried out within the same research, which could result in a more insightful and interesting investigation (see, e.g. Koufteros, Verghese, and Lucianetti Citation2014).
Future research will also need to investigate what thresholds of Dyn and SCpx define the manufacturing environment types, e.g. Stress shops could be defined by Dyn > 0.4 and SCpx > 0.4, and whether those thresholds are independent of each other or have an interaction, e.g. the higher the SCpx of a production line is, the lower Dyn will need to be to define a Stress shop.
Whereas the effect of fitting practices on the performance of certain company environments has been previously studied (Sousa and Voss Citation2008; Tenhiälä and Helkiö Citation2015), more research is needed to develop an analysis of the trade-offs between implementing fitting practices/technologies and the impact that implementation may have on the financial health of the company (see, e.g. Ketzenberg and Metters Citation2019).
Finally, a comprehensive theory of the effects of capacity utilization on the overall strategic position of companies is needed, beyond its effect on the relevance of the fits between scheduling environment and approach, as there have been very few studies in operations management regarding the role of capacity utilization (or slack) on the performance and competitive advantage of manufacturing firms (see, e.g. Abdi and Labib Citation2017).
Disclosure statement
No potential conflict of interest was reported by the author(s).
Additional information
Notes on contributors
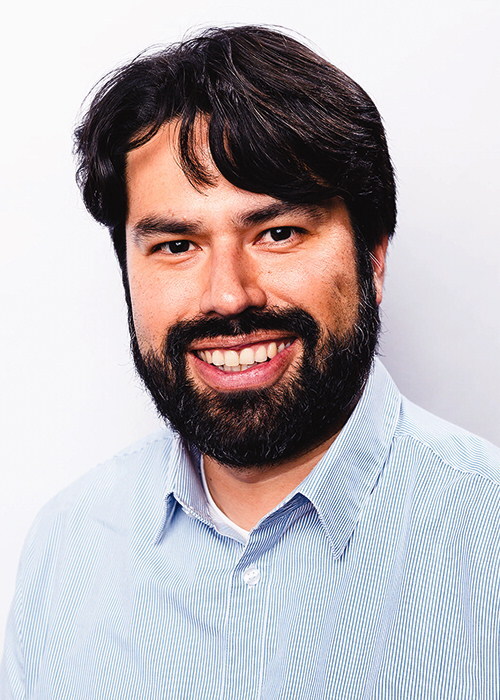
Rodrigo Romero-Silva
Rodrigo Romero-Silva is an Assistant Professor at the Operations Research and Logistics Group of Wageningen University & Research (The Netherlands) and an Assistant Professor at the Faculty of Engineering at Universidad Panamericana (Mexico). He received his Ph.D. in Industrial Engineering from the University of Navarra (Spain) in 2012. Rodrigo’s research is focused on understanding the behavior of stochastic and dynamic production and logistics systems while creating applicable planning approaches for their operation. Before joining academia, Rodrigo worked as a data analyst in the retail sector.

Javier Santos
Javier Santos is Professor of Business Organization at the Engineering School of University of Navarra in Spain. He has a PhD in Industrial Engineering and is a Lean Manufacturing expert, area in which he has been teaching for 25 years. As a researcher, he has supervised nine Ph.D. theses and is the author of 50 indexed scientific publications. He has also participated in 23 research projects, being the main researcher in seven of them, highlighting the European project LIFE MCUBO (2016–2020). He is the main inventor of a patent. He has been Deputy Director of the Doctoral School of the University of Navarra (2012–2017). In 2006 he published the book ‘Improving Production with Lean Thinking’ which has been translated into four languages.
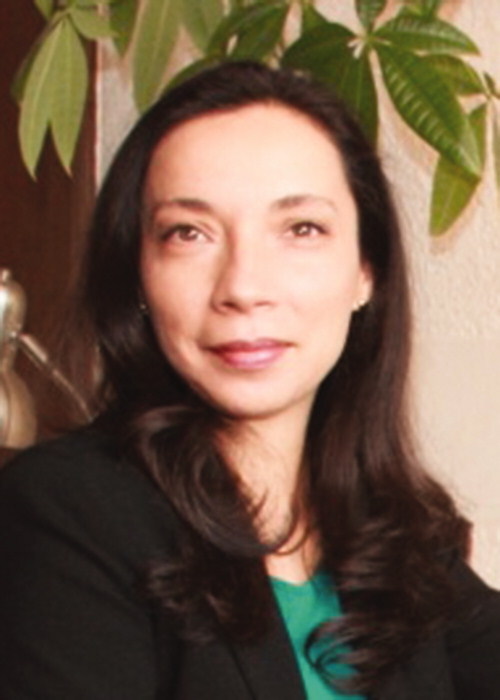
Margarita Hurtado-Hernández
Margarita Hurtado-Hernández is an academician oriented towards the application of knowledge obtaining positive results in organizations, through business training and the higher education of young professionals. She is the Director of graduate programs at the Faculty of Engineering of Universidad Panamericana (Mexico) and a teacher—researcher at the Operations Management Department. She has a Ph.D. in Operations Research and Management Science and has been the leader on a number of research projects on how to improve the performance of organizations through the systems thinking approach. She has published three books on how to address real problems using systems thinking.
References
- Abdi, M. Reza, and Ashraf Labib. 2017. “RMS Capacity Utilisation: Product Family and Supply Chain.” International Journal of Production Research 55 (7): 1930–1956. doi:10.1080/00207543.2016.1229066.
- Abdullah, Salwani, and Majid Abdolrazzagh-Nezhad. 2014. “Fuzzy Job-Shop Scheduling Problems: A Review.” Information Sciences 278: 380–407. doi:10.1016/j.ins.2014.03.060.
- Abedinnia, Hamid, Christoph H. Glock, Eric H. Grosse, and Michael. Schneider. 2017. “Machine Scheduling Problems in Production: A Tertiary Study.” Computers & Industrial Engineering 111 (Supplement C): 403–416. doi:10.1016/j.cie.2017.06.026.
- Ahmad, Sohel, and Roger G. Schroeder. 2002. “Refining the Product-Process Matrix.” International Journal of Operations & Production Management 22 (1): 103–124. doi:10.1108/01443570210412097.
- Allahverdi, Ali, Jatinder N. D. Gupta, and Tariq Aldowaisan. 1999. “A Review of Scheduling Research Involving Setup Considerations.” Omega 27 (2): 219–239. doi:10.1016/S0305-0483(98)00042-5.
- Aytug, Haldun, S. Bhattacharyya, G. J. Koehler, and J. L. Snowdon. 1994. “A Review of Machine Learning in Scheduling.” IEEE Transactions on Engineering Management 41 (2): 165–171. doi:10.1109/17.293383.
- Benavides, Marcos Buestán, and Hendrik Van Landeghem. 2015. “Implementation of S-DBR in Four Manufacturing SMEs: A Research Case Study.” Production Planning & Control 26 (13): 1110–1127. doi:10.1080/09537287.2015.1015060.
- Berglund, Martina, and J. Karltun. 2007. “Human, Technological and Organizational Aspects Influencing the Production Scheduling Process.” International Journal of Production Economics 110 (1–2): 160–174. doi:10.1016/j.ijpe.2007.02.024.
- Bertrand, J. W. M. 1983. “The Effect of Workload Dependent Due-Dates on Job Shop Performance.” Management Science 29 (7): 799–816. doi:10.1287/mnsc.29.7.799.
- Bertrand, J. W. M., and H. P. G. Van Ooijen. 2002. “Workload Based Order Release and Productivity: A Missing Link.” Production Planning & Control 13 (7): 665–678. doi:10.1080/0953728021000026276.
- Bidot, Julien, Thierry Vidal, Philippe Laborie, and J. Christopher Beck. 2009. “A Theoretic and Practical Framework for Scheduling in a Stochastic Environment.” Journal of Scheduling 12 (3): 315–344. doi:10.1007/s10951-008-0080-x.
- Blazewicz, Jacek, Moshe Dror, and Jan Weglarz. 1991. “Mathematical Programming Formulations for Machine Scheduling: A Survey.” European Journal of Operational Research 51 (3): 283–300. doi:10.1016/0377-2217(91)90304-E.
- Board of Governors of the Federal Reserve System. 2022a. “Capacity Utilization: Manufacturing (NAICS).” Federal Reserve Bank of St. Louis. https://fred.stlouisfed.org/series/MCUMFN
- Board of Governors of the Federal Reserve System. 2022b. “Industrial Production: Manufacturing (SIC).” https://fred.stlouisfed.org/series/IPMANSICS
- Boysen, Nils, Stefan Bock, and Malte Fliedner. 2013. “Scheduling of Inventory Releasing Jobs to Satisfy Time-Varying Demand: An Analysis of Complexity.” Journal of Scheduling 16 (2): 185–198. doi:10.1007/s10951-012-0266-0.
- Branke, Jürgen, Torsten Hildebrandt, and Bernd Scholz-Reiter. 2015. “Hyper-Heuristic Evolution of Dispatching Rules: A Comparison of Rule Representations.” Evolutionary Computation 23 (2): 249–277. doi:10.1162/EVCO_a_00131.
- Branke, Jurgen, Su Nguyen, Christoph W. Pickardt, and Mengjie Zhang. 2016. “Automated Design of Production Scheduling Heuristics: A Review.” IEEE Transactions on Evolutionary Computation 20 (1): 110–124. doi:10.1109/TEVC.2015.2429314.
- Buxey, Geoff. 1989. “Production Scheduling: Practice and Theory.” European Journal of Operational Research 39 (1): 17–31. doi:10.1016/0377-2217(89)90349-4.
- Carvalho, Andréa N., Luiz Felipe Scavarda, and Leonardo J. Lustosa. 2014. “Implementing Finite Capacity Production Scheduling: Lessons from a Practical Case.” International Journal of Production Research 52 (4): 1215–1230. doi:10.1080/00207543.2013.848484.
- Chakravorty, S. S., and J. Brian Atwater. 2006. “Bottleneck Management: Theory and Practice.” Production Planning & Control 17 (5): 441–447. doi:10.1080/09537280600682752.
- Cheevaprawatdomrong, Torpong, and Robert L. Smith. 2004. “Infinite Horizon Production Scheduling in Time-Varying Systems under Stochastic Demand.” Operations Research 52 (1): 105–115. doi:10.1287/opre.1030.0080.
- Child, John. 1972. “Organizational Structure, Environment and Performance: The Role of Strategic Choice.” Sociology 6 (1): 1–22. http://www.jstor.org/stable/42851133.
- Christensen, Clayton M. 2006. “The Ongoing Process of Building a Theory of Disruption.” Journal of Product Innovation Management 23 (1): 39–55. doi:10.1111/j.1540-5885.2005.00180.x.
- Cigolini, R. 1999. “Implementing New Dispatching Rules at SGS-Thomson Microelectronics.” Production Planning & Control 10 (1): 97–106. doi:10.1080/095372899233479.
- Comstock, Mica, Kerstin Johansen, and Mats Winroth. 2004. “From Mass Production to Mass Customization: Enabling Perspectives from the Swedish Mobile Telephone Industry.” Production Planning & Control 15 (4): 362–372. doi:10.1080/0953728042000238836.
- Conway, Richard, William Maxwell, and Louis Miller. 1967. Theory of Scheduling. Boston, MA: Addison-Wesley.
- Crawford, S. Vincent, and C. S. Wiers. 2001. “From Anecdotes to Theory: A Review of Existing Knowledge on Human Factors of Planning and Scheduling.” In Human Performance in Planning and Scheduling, edited by Bart L. MacCarthy and John R. Wilson, 15–42. London: Taylor & Francis.
- De Snoo, Cees, Wout Van Wezel, and René J. Jorna. 2011. “An Empirical Investigation of Scheduling Performance Criteria.” Journal of Operations Management 29 (3): 181–193. doi:10.1016/j.jom.2010.12.006.
- Dios, Manuel, and Jose M. Framinan. 2016. “A Review and Classification of Computer-Based Manufacturing Scheduling Tools.” Computers & Industrial Engineering 99: 229–249. doi:10.1016/j.cie.2016.07.020.
- Donaldson, Lex. 2001. The Contingency Theory of Organizations. Thousand Oaks, CA: SAGE Publications, Inc. doi:10.4135/9781452229249.
- Dong, Yachao, and Christos T. Maravelias. 2021. “Terminal Inventory Level Constraints for Online Production Scheduling.” European Journal of Operational Research 295 (1): 102–117. doi:10.1016/j.ejor.2021.02.029.
- Framinan, Jose M., Victor Fernandez-Viagas, and Paz Perez-Gonzalez. 2019. “Using Real-Time Information to Reschedule Jobs in a Flowshop with Variable Processing Times.” Computers & Industrial Engineering 129: 113–125. doi:10.1016/j.cie.2019.01.036.
- Framinan, Jose M., and Paz Perez-Gonzalez. 2015. “On Heuristic Solutions for the Stochastic Flowshop Scheduling Problem.” European Journal of Operational Research 246 (2): 413–420. doi:10.1016/j.ejor.2015.05.006.
- Framinan, Jose M., and Rubén Ruiz. 2010. “Architecture of Manufacturing Scheduling Systems: Literature Review and an Integrated Proposal.” European Journal of Operational Research 205 (2): 237–246. doi:10.1016/j.ejor.2009.09.026.
- Framinan, Jose M., and Rubén Ruiz. 2012. “Guidelines for the Deployment and Implementation of Manufacturing Scheduling Systems.” International Journal of Production Research 50 (7): 1799–1812. doi:10.1080/00207543.2011.564670.
- Fuchigami, Helio Yochihiro, and Socorro Rangel. 2018. “A Survey of Case Studies in Production Scheduling: Analysis and Perspectives.” Journal of Computational Science 25: 425–436. doi:10.1016/j.jocs.2017.06.004.
- Gómez Paredes, Fernando José, Moacir Godinho Filho, Matthias Thürer, Nuno O. Fernandes, and Charbel José Chiappeta Jabbour. 2022. “Factors for Choosing Production Control Systems in Make-to-Order Shops: A Systematic Literature Review.” Journal of Intelligent Manufacturing 33 (3): 639–674. doi:10.1007/s10845-020-01673-z.
- Gosling, Jonathan, and Mohamed M. Naim. 2009. “Engineer-to-Order Supply Chain Management: A Literature Review and Research Agenda.” International Journal of Production Economics 122 (2): 741–754. doi:10.1016/j.ijpe.2009.07.002.
- Graham, R. L., E. L. Lawler, J. K. Lenstra, and A. H. G. Rinnooy Kan. 1979. “Optimization and Approximation in Deterministic Sequencing and Scheduling: A Survey.” Annals of Discrete Mathematics 5: 287–326. doi:10.1016/S0167-5060(08)70356-X.
- Gross, Donald, John F. Shortle, James M. Thompson, and Carl M. Harris. 2008. Fundamentals of Queueing Theory. 4th ed. Hoboken, NJ: Wiley-Interscience.
- Halsall, David N., Alan P. Muhlemann, and David H. R. Price. 1994. “A Review of Production Planning and Scheduling in Smaller Manufacturing Companies in the UK.” Production Planning & Control 5 (5): 485–493. doi:10.1080/09537289408919520.
- Harjunkoski, Iiro. 2016. “Deploying Scheduling Solutions in an Industrial Environment.” Computers & Chemical Engineering 91: 127–135. doi:10.1016/j.compchemeng.2016.03.029.
- Harjunkoski, Iiro, Christos T. Maravelias, Peter Bongers, Pedro M. Castro, Sebastian Engell, Ignacio E. Grossmann, John Hooker, Carlos Méndez, Guido Sand, and John Wassick. 2014. “Scope for Industrial Applications of Production Scheduling Models and Solution Methods.” Computers & Chemical Engineering 62: 161–193. doi:10.1016/j.compchemeng.2013.12.001.
- Hayes, Robert H., and Steven C. Wheelwright. 1979. “Link Manufacturing Process and Product Life Cycles.” Harvard Business Review 57 (1): 133–140.
- Helkiö, Pekka, and Antti Tenhiälä. 2013. “A Contingency Theoretical Perspective to the Product‐Process Matrix.” International Journal of Operations & Production Management 33 (2): 216–244. doi:10.1108/01443571311295644.
- Hendry, Linda, Yuan Huang, and Mark Stevenson. 2013. “Workload Control: Successful Implementation Taking a Contingency‐based View of Production Planning and Control.” International Journal of Operations & Production Management 33 (1): 69–103. doi:10.1108/01443571311288057.
- Hopp, Wallace, and Mark Spearman. 2000. Factory Physics. 2nd ed. McGraw-Hill.
- Hvolby, Hans-Henrik, and Kenn Steger-Jensen. 2010. “Technical and Industrial Issues of Advanced Planning and Scheduling (APS) Systems.” Computers in Industry 61 (9): 845–851. doi:10.1016/j.compind.2010.07.009.
- Jackson, Sarah, John R. Wilson, and Bart L. MacCarthy. 2004. “A New Model of Scheduling in Manufacturing: Tasks, Roles, and Monitoring.” Human Factors 46 (3): 533–550. doi:10.1518/hfes.46.3.533.50393.
- Jalali, Hamed, and Inneke Van Nieuwenhuyse. 2015. “Simulation Optimization in Inventory Replenishment: A Classification.” IIE Transactions 47 (11): 1217–1235. doi:10.1080/0740817X.2015.1019162.
- Jayamohan, M. S., and Chandrasekharan Rajendran. 2000. “New Dispatching Rules for Shop Scheduling: A Step Forward.” International Journal of Production Research 38 (3): 563–586. doi:10.1080/002075400189301.
- Jeong, K.-C., and Y.-D. Kim. 1998. “A Real Time Scheduling Mechanism for a Flexible Manufacturing System: Using Simulation and Dispatching Rules.” International Journal of Production Research, 36 (9): 2609–2626. doi:10.1080/002075498192733.
- Jodlbauer, H., and A. Huber. 2008. “Service-Level Performance of MRP, Kanban, CONWIP and DBR Due to Parameter Stability and Environmental Robustness.” International Journal of Production Research 46 (8): 2179–2195. doi:10.1080/00207540600609297.
- Johri, Pravin K. 1993. “Practical Issues in Scheduling and Dispatching in Semiconductor Wafer Fabrication.” Journal of Manufacturing Systems 12 (6): 474–485. doi:10.1016/0278-6125(93)90344-S.
- Jonsson, Patrik, and Stig-Arne Mattsson. 2003. “The Implications of Fit between Planning Environments and Manufacturing Planning and Control Methods.” International Journal of Operations & Production Management 23 (8): 872–900. doi:10.1108/01443570310486338.
- Jordan, M. I., and T. M. Mitchell. 2015. “Machine Learning: Trends, Perspectives, and Prospects.” Science 349 (6245): 255–260. doi:10.1126/science.aaa8415.
- Jungwattanakit, Jitti, Manop Reodecha, Paveena Chaovalitwongse, and Frank Werner. 2008. “Algorithms for Flexible Flow Shop Problems with Unrelated Parallel Machines, Setup Times, and Dual Criteria.” The International Journal of Advanced Manufacturing Technology 37 (3–4): 354–370. doi:10.1007/s00170-007-0977-0.
- Kaçmaz, Özlem, Haci M. Alakaş, and Tamer Eren. 2019. “Shift Scheduling with the Goal Programming Method: A Case Study in the Glass Industry.” Mathematics 7 (6): 561. doi:10.3390/math7060561.
- Kemppainen, Katariina, Ari P. J. Vepsäläinen, and Markku Tinnilä. 2008. “Mapping the Structural Properties of Production Process and Product Mix.” International Journal of Production Economics 111 (2): 713–728. doi:10.1016/j.ijpe.2007.03.020.
- Kerr, Roger M. 1992. “Expert Systems in Production Scheduling: Lessons from a Failed Implementation.” Journal of Systems and Software 19 (2): 123–130. doi:10.1016/0164-1212(92)90063-P.
- Ketokivi, Mikko, and Mikko Jokinen. 2006. “Strategy, Uncertainty and the Focused Factory in International Process Manufacturing.” Journal of Operations Management 24 (3): 250–270. doi:10.1016/j.jom.2004.07.011.
- Ketzenberg, Michael E., and Richard D. Metters. 2019. “Adapting Operations to New Information Technology: A Failed ‘Internet of Things’ Application.” Omega 92: 102152. doi:10.1016/j.omega.2019.102152.
- King, J. R. 1976. “The Theory-Practice Gap in Job-Shop Scheduling.” Production Engineer 55 (3): 137. doi:10.1049/tpe.1976.0044.
- Kjellsdotter, Linea. 2012. “Shop Floor Characteristics Influencing the Use of Advanced Planning and Scheduling Systems.” Production Planning and Control 23 (6): 452–467. doi:10.1080/09537287.2011.564218.
- Kjellsdotter, Linea, and Patrik Jonsson. 2010. “Problems in the Onward and Upward Phase of APS Implementation: Why Do They Occur?”
- Korytkowski, Przemysław, Tomasz Wiśniewski, and Szymon Rymaszewski. 2013. “An Evolutionary Simulation-Based Optimization Approach for Dispatching Scheduling.” Simulation Modelling Practice and Theory 35: 69–85. doi:10.1016/j.simpat.2013.03.006.
- Koufteros, Xenophon, Anto (John) Verghese, and Lorenzo Lucianetti. 2014. “The Effect of Performance Measurement Systems on Firm Performance: A Cross-Sectional and a Longitudinal Study.” Journal of Operations Management 32 (6): 313–336. doi:10.1016/j.jom.2014.06.003.
- Kusiak, Andrew. 2018. “Smart Manufacturing.” International Journal of Production Research 56 (1–2): 508–517. doi:10.1080/00207543.2017.1351644.
- Laengle, Sigifredo, José M. M. Merigó, Jaime Miranda, Roman Słowiński, Immanuel Bomze, Emanuele Borgonovo, Robert G. G. Dyson, José Fernando Fernando Oliveira, and Ruud Teunter. 2017. “Forty Years of the European Journal of Operational Research: A Bibliometric Overview.” European Journal of Operational Research 262 (3): 803–816. doi:10.1016/j.ejor.2017.04.027.
- Larsen, A., O. Madsen, and M. Solomon. 2002. “Partially Dynamic Vehicle Routing—Models and Algorithms.” Journal of the Operational Research Society 53 (6): 637–646. doi:10.1057/palgrave.jors.2601352.
- Lee, Young Hoon, Kumar Bhaskaran, and Michael Pinedo. 1997. “A Heuristic to Minimize the Total Weighted Tardiness with Sequence-Dependent Setups.” IIE Transactions 29 (1): 45–52. doi:10.1080/07408179708966311.
- Lee, Young Hoon, and Michael Pinedo. 1997. “Scheduling Jobs on Parallel Machines with Sequence-Dependent Setup Times.” European Journal of Operational Research 100 (3): 464–474. doi:10.1016/S0377-2217(95)00376-2.
- Lenstra, J., K. A. H. G. Rinnooy Kan, and P. Brucker. 1977. “Complexity of Machine Scheduling Problems.” Annals of Discrete Mathematics 1: 343–362. doi:10.1016/S0167-5060(08)70743-X.
- Leonardo, Dênis Gustavo, Bruno Sereno, Daniel Sant Anna da Silva, Mauro Sampaio, Alexandre Augusto Massote, and Jairo Celso Simões. 2017. “Implementation of Hybrid Kanban-CONWIP System: A Case Study.” Journal of Manufacturing Technology Management 28 (6): 714–736. doi:10.1108/JMTM-03-2016-0043.
- Lin, James T., and Chien-Ming Chen. 2015. “Simulation Optimization Approach for Hybrid Flow Shop Scheduling Problem in Semiconductor Back-End Manufacturing.” Simulation Modelling Practice and Theory 51: 100–114. doi:10.1016/j.simpat.2014.10.008.
- López-Santana, Eduyn, and Germán Andrés Méndez-Giraldo Ramiro. 2016. “A Knowledge-Based Expert System for Scheduling in Services Systems.” In Applied Computer Sciences in Engineering, edited by Juan Carlos Figueroa-García, Eduyn Ramiro López-Santana, and Roberto Ferro-Escobar, 212–224. Cham: Springer International Publishing.
- Lu, H. L., George Q. Huang, and H. D. Yang. 2011. “Integrating Order Review/Release and Dispatching Rules for Assembly Job Shop Scheduling Using a Simulation Approach.” International Journal of Production Research 49 (3): 647–669. doi:10.1080/00207540903524490.
- McKay, Kenneth N., and John Buzacott. 2000. “The Application of Computerized Production Control Systems in Job Shop Environments.” Computers in Industry 42 (2–3): 79–97. doi:10.1016/S0166-3615(99)00063-9.
- McKay, Kenneth N., Frank Safayeni, and John Buzacott. 1988. “Job-Shop Scheduling Theory: What is Relevant?” Interfaces 18 (4): 84–90. doi:10.1287/inte.18.4.84.
- McKay, Kenneth N., and Vincent C. S. Wiers. 1999. “Unifying the Theory and Practice of Production Scheduling.” Journal of Manufacturing Systems 18 (4): 241–255. doi:10.1016/S0278-6125(00)86628-5.
- McKay, Kenneth N., and Vincent C. S. Wiers. 2003. “Integrated Decision Support for Planning, Scheduling, and Dispatching Tasks in a Focused Factory.” Computers in Industry 50 (1): 5–14. doi:10.1016/S0166-3615(02)00146-X.
- Meyer, Heiko, Franz Fuchs, and Klaus Thiel. 2009. Manufacturing Execution Systems (MES): Optimal Design, Planning, and Deployment. 1st ed. New York, NY: McGraw-Hill.
- Mittal, Sameer, Muztoba Ahmad Khan, David Romero, and Thorsten Wuest. 2018. “A Critical Review of Smart Manufacturing & Industry 4.0 Maturity Models: Implications for Small and Medium-Sized Enterprises (SMEs).” Journal of Manufacturing Systems 49: 194–214. doi:10.1016/j.jmsy.2018.10.005.
- Morton, Thomas, and David W. Pentico. 1993. Heuristic Scheduling Systems: With Applications to Production Systems and Project Management. Hoboken, NJ: Wiley-Interscience. http://www.amazon.com/gp/redirect.html?location=/exec/obidos/ASIN/0471578193%7B&%7DAWSAccessKeyId=1TQA6S4ATNFN6EYN0H82
- Mukhopadhyay, S. K., and S. Shanker. 2005. “Kanban Implementation at a Tyre Manufacturing Plant: A Case Study.” Production Planning & Control 16 (5): 488–499. doi:10.1080/09537280500121778.
- Münch, Lars, John W. Fowler, and Scott J. Mason, eds. 2013. “Dispatching Approaches.” In Production Planning and Control for Semiconductor Wafer Fabrication Facilities: Modeling, Analysis, and Systems, 65–104. New York, NY: Springer. doi:10.1007/978-1-4614-4472-5_4.
- Novas, Juan M., and Gabriela P. Henning. 2010. “Reactive Scheduling Framework Based on Domain Knowledge and Constraint Programming.” Computers & Chemical Engineering 34 (12): 2129–2148. doi:10.1016/j.compchemeng.2010.07.011.
- Ouelhadj, Djamila, and Sanja Petrovic. 2009. “A Survey of Dynamic Scheduling in Manufacturing Systems.” Journal of Scheduling 12 (4): 417–431. doi:10.1007/s10951-008-0090-8.
- Parthanadee, P., and J. Buddhakulsomsiri. 2010. “Simulation Modeling and Analysis for Production Scheduling Using Real-Time Dispatching Rules: A Case Study in Canned Fruit Industry.” Computers and Electronics in Agriculture 70 (1): 245–255. doi:10.1016/j.compag.2009.11.002.
- Pegels, C. Carl, and Craig Watrous. 2005. “Application of the Theory of Constraints to a Bottleneck Operation in a Manufacturing Plant.” Journal of Manufacturing Technology Management 16 (3): 302–311. doi:10.1108/17410380510583617.
- Pickardt, Christoph W., and Jürgen Branke. 2012. “Setup-Oriented Dispatching Rules – A Survey.” International Journal of Production Research 50 (20): 5823–5842. doi:10.1080/00207543.2011.629634.
- Pickardt, Christoph W., Torsten Hildebrandt, Jürgen Branke, Jens Heger, and Bernd Scholz-Reiter. 2013. “Evolutionary Generation of Dispatching Rule Sets for Complex Dynamic Scheduling Problems.” International Journal of Production Economics 145 (1): 67–77. doi:10.1016/j.ijpe.2012.10.016.
- Pillac, Victor, Michel Gendreau, Christelle Guéret, and Andrés L. Medaglia. 2013. “A Review of Dynamic Vehicle Routing Problems.” European Journal of Operational Research 225 (1): 1–11. doi:10.1016/j.ejor.2012.08.015.
- Pinedo, Michael. 2016. Scheduling: Theory, Algorithms and Systems. 5th ed. New York, NY: Springer. doi:10.1007/978-1-4614-2361-4.
- Portougal, Victor, and David Robb. 2000. “Production Scheduling Theory: Just Where is It Applicable?” Interfaces 30 (6): 64–76. doi:10.1287/inte.30.6.64.11623.
- Priore, Paolo, Alberto Gómez, Raúl Pino, and Rafael Rosillo. 2014. “Dynamic Scheduling of Manufacturing Systems Using Machine Learning: An Updated Review.” Artificial Intelligence for Engineering Design, Analysis and Manufacturing 28 (1): 83–97. doi:10.1017/S0890060413000516.
- Qi, Xiangtong, Jonathan F. Bard, and Gang Yu. 2006. “Disruption Management for Machine Scheduling: The Case of SPT Schedules.” International Journal of Production Economics 103 (1): 166–184. doi:10.1016/j.ijpe.2005.05.021.
- Rajendran, Chandrasekharan, and Oliver Holthaus. 1999. “A Comparative Study of Dispatching Rules in Dynamic Flowshops and Jobshops.” European Journal of Operational Research 116 (1): 156–170. doi:10.1016/S0377-2217(98)00023-X.
- Rebecca, Duray. 2002. “Mass Customization Origins: Mass or Custom Manufacturing?” International Journal of Operations & Production Management 22 (3): 314–328. doi:10.1108/01443570210417614.
- Reisman, A., A. Kumar, and J. Motwani. 1997. “Flowshop Scheduling/Sequencing Research: A Statistical Review of the Literature, 1952–1994.” IEEE Transactions on Engineering Management 44 (3): 316–329. doi:10.1109/17.618173.
- Romero-Silva, Rodrigo, and Sander de Leeuw. 2021. “Learning from the past to Shape the Future: A Comprehensive Text Mining Analysis of or/MS Reviews.” Omega 100: 102388. doi:10.1016/j.omega.2020.102388.
- Romero-Silva, Rodrigo, and Gabriel Hernández-López. 2020. “Shop-Floor Scheduling as a Competitive Advantage: A Study on the Relevance of Cyber-Physical Systems in Different Manufacturing Contexts.” International Journal of Production Economics 224: 107555. doi:10.1016/j.ijpe.2019.107555.
- Romero-Silva, Rodrigo, Margarita Hurtado, and Javier Santos. 2016. “Is the Scheduling Task Context-Dependent? A Survey Investigating the Presence of Constraints in Different Manufacturing Contexts.” Production Planning & Control 27 (9): 753–760. doi:10.1080/09537287.2016.1166274.
- Romero-Silva, Rodrigo, and Erika Marsillac. 2019. “Trends and Topics in IJPR from 1961 to 2017: A Statistical History.” International Journal of Production Research 57 (15–16): 4692–4718. doi:10.1080/00207543.2018.1551638.
- Romero-Silva, Rodrigo, Javier Santos, and Margarita Hurtado. 2015. “A Framework for Studying Practical Production Scheduling.” Production Planning & Control 26 (6): 438–450. doi:10.1080/09537287.2014.919413.
- Romero-Silva, Rodrigo, Javier Santos, and Margarita Hurtado. 2018. “A Note on Defining Organisational Systems for Contingency Theory in OM.” Production Planning & Control 29 (16): 1343–1348. doi:10.1080/09537287.2018.1535146.
- Romero-Silva, Rodrigo, Sabry Shaaban, Erika Marsillac, and Margarita Hurtado. 2018. “Exploiting the Characteristics of Serial Queues to Reduce the Mean and Variance of Flow Time Using Combined Priority Rules.” International Journal of Production Economics 196: 211–225. doi:10.1016/j.ijpe.2017.11.023.
- Roser, C., M. Nakano, and M. Tanaka. 2002. “Shifting Bottleneck Detection.” In Proceedings of the Winter Simulation Conference. Vol. 2, 1079–1086. doi:10.1109/WSC.2002.1166360.
- Rossit, Daniel Alejandro, Fernando Tohmé, and Mariano Frutos. 2019. “Industry 4.0: Smart Scheduling.” International Journal of Production Research 57 (12): 3802–3813. doi:10.1080/00207543.2018.1504248.
- Ruiz, Rubén. 2016. “Scheduling Heuristics.” In Handbook of Heuristics, edited by Rafael Martí, Pardalos Panos, and Mauricio G. C. Resende, 1–24. Cham: Springer International Publishing. doi:10.1007/978-3-319-07153-4_44-1.
- Ruiz, Rubén, and Thomas Stützle. 2007. “A Simple and Effective Iterated Greedy Algorithm for the Permutation Flowshop Scheduling Problem.” European Journal of Operational Research 177 (3): 2033–2049. doi:10.1016/j.ejor.2005.12.009.
- Safizadeh, M. Hossein, Larry P. Ritzman, Deven Sharma, and Craig Wood. 1996. “An Empirical Analysis of the Product-Process Matrix.” Management Science 42 (11): 1576–1591. doi:10.1287/mnsc.42.11.1576.
- Salido, Miguel A., Antonio Garrido, and Roman Barták. 2008. “Introduction: Special Issue on Constraint Satisfaction Techniques for Planning and Scheduling Problems.” Engineering Applications of Artificial Intelligence 21 (5): 679–682. doi:10.1016/j.engappai.2008.03.007.
- Schonberger, Richard J., and Karen A. Brown. 2017. “Missing Link in Competitive Manufacturing Research and Practice: Customer-Responsive Concurrent Production.” Journal of Operations Management 49–51 (1): 83–87. doi:10.1016/j.jom.2016.12.006.
- Sharma, Pankaj, and Ajai Jain. 2014. “Analysis of Dispatching Rules in a Stochastic Dynamic Job Shop Manufacturing System with Sequence-Dependent Setup Times.” Frontiers of Mechanical Engineering 9 (4): 380–389. doi:10.1007/s11465-014-0315-9.
- Shen, Liji, Stéphane Dauzère-Pérès, and Janis S. Neufeld. 2018. “Solving the Flexible Job Shop Scheduling Problem with Sequence-Dependent Setup Times.” European Journal of Operational Research 265 (2): 503–516. doi:10.1016/j.ejor.2017.08.021.
- Slotnick, Susan A. 2011. “Order Acceptance and Scheduling: A Taxonomy and Review.” European Journal of Operational Research 212 (1): 1–11. doi:10.1016/j.ejor.2010.09.042.
- Sousa, Rui, and Christopher A. Voss. 2008. “Contingency Research in Operations Management Practices.” Journal of Operations Management 26 (6): 697–713. doi:10.1016/j.jom.2008.06.001.
- Tenhiälä, Antti. 2011. “Contingency Theory of Capacity Planning: The Link between Process Types and Planning Methods.” Journal of Operations Management 29 (1–2): 65–77. doi:10.1016/j.jom.2010.05.003.
- Tenhiälä, Antti, and Pekka Helkiö. 2015. “Performance Effects of Using an ERP System for Manufacturing Planning and Control under Dynamic Market Requirements.” Journal of Operations Management 36 (1): 147–164. doi:10.1016/j.jom.2014.05.001.
- Thürer, Matthias, Mark Stevenson, and Cristovao Silva. 2011. “Three Decades of Workload Control Research: A Systematic Review of the Literature.” International Journal of Production Research 49 (23): 6905–6935. doi:10.1080/00207543.2010.519000.
- Tomotani, João Vitor, Marco Aurélio de Mesquita. 2018. “Lot Sizing and Scheduling: A Survey of Practices in Brazilian Companies.” Production Planning & Control 29 (3): 236–246. doi:10.1080/09537287.2017.1409370.
- Uhlmann, Iracyanne Retto, and Enzo Morosini Frazzon. 2018. “Production Rescheduling Review: Opportunities for Industrial Integration and Practical Applications.” Journal of Manufacturing Systems 49: 186–193. doi:10.1016/j.jmsy.2018.10.004.
- Van de Ven, Andrew, and Robert Drazin. 1984. The Concept of Fit in Contingency Theory.
- Vieira, Guilherme E., Jeffrey W. Herrmann, and Edward Lin. 2003. “Rescheduling Manufacturing Systems: A Framework of Strategies, Policies, and Methods.” Journal of Scheduling 6 (1): 39–62. doi:10.1023/A:1022235519958.
- Wacker, John G. 1998. “A Definition of Theory: Research Guidelines for Different Theory-Building Research Methods in Operations Management.” Journal of Operations Management 16 (4): 361–385. doi:10.1016/S0272-6963(98)00019-9.
- Wezel, Wout Van, René J. Jorna, and Alexander M. Meystel. 2006. Planning in Intelligent Systems: Aspects, Motivations and Methods. Hoboken, NJ: John Wiley & Sons.
- Wiers, Vincent C. S. 1997. “A Review of the Applicability of or and AI Scheduling Techniques in Practice.” Omega 25 (2): 145–153. doi:10.1016/S0305-0483(96)00050-3.
- Wiers, Vincent C. S. 2009. “The Relationship between Shop Floor Autonomy and APS Implementation Success: Evidence from Two Cases.” Production Planning & Control 20 (7): 576–585. doi:10.1080/09537280903034289.
- Wiers, Vincent C. S., and A. (Ton). G. de Kok. 2018a. “The Implementation Project.” In Designing, Selecting, Implementing and Using APS Systems, 139–151. Cham: Springer International Publishing. doi:10.1007/978-3-319-65055-5_5.
- Wiers, Vincent C. S., and A. (Ton). G. de Kok. 2018b. “Definition and Context.” In Designing, Selecting, Implementing and Using APS Systems, 1–17. Cham: Springer International Publishing. doi:10.1007/978-3-319-65055-5_1.
- Wiers, Vincent C. S., and Tjerk Van der Schaaf. 1997. “A Framework for Decision Support in Production Scheduling Tasks.” Production Planning & Control 8 (6): 533–544. doi:10.1080/095372897234876.
- Xiong, Hegen, Huali Fan, Guozhang Jiang, and Gongfa Li. 2017. “A Simulation-Based Study of Dispatching Rules in a Dynamic Job Shop Scheduling Problem with Batch Release and Extended Technical Precedence Constraints.” European Journal of Operational Research 257 (1): 13–24. doi:10.1016/j.ejor.2016.07.030.
- Yang, Feng, Bruce E. Ankenman, and Barry L. Nelson. 2007. “Efficient Generation of Cycle Time-Throughput Curves through Simulation and Metamodeling.” Naval Research Logistics 54 (1): 78–93. doi:10.1002/nav.20188.
- Yang, T., Y. Kuo, and I. Chang. 2004. “Tabu-Search Simulation Optimization Approach for Flow, Shop Scheduling with Multiple Processors — A Case Study.” International Journal of Production Research 42 (19): 4015–4030. doi:10.1080/00207540410001699381.
- Zanda, Simone, Paola Zuddas, and Carla Seatzu. 2018. “Long Term Nurse Scheduling via a Decision Support System Based on Linear Integer Programming: A Case Study at the University Hospital in Cagliari.” Computers & Industrial Engineering 126: 337–347. doi:10.1016/j.cie.2018.09.027.
Appendix
Based on the sample of 50 manufacturing firms considered in Romero-Silva, Hurtado, and Santos (Citation2016), shows an example of the proposed characterization of manufacturing systems. Depending on the characteristics of each firm, values for Dyn, SCpx and PPSTCpx (represented by the y-axis, x-axis and values inside the circles in , respectively) were calculated and plotted. Moreover, as there were various firms with the same exact values for Dyn and SCpx, the frequency of each pair of values was represented by the size of the area of the plot circles in .
Figure A1. Classification and PPSTCpx values of 50 manufacturing firms according to their Dyn and SCpx values.
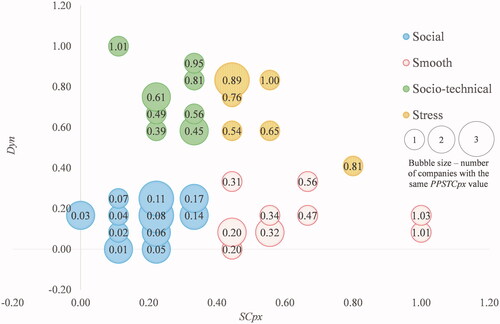
It is worth noting that for this exercise, the values for w and v weights from Equationequations (1)(1)
(1) and Equation(3)
(3)
(3) were equal to 1. In addition, the threshold values to set the combination of values between Dyn and SCpx that will define a type of manufacturing system were set to 0.4, resulting from the particular characteristics of this sample of firms.
In this sample, the Social shop was the most prevalent system, with 21 cases, followed by the Smooth shop (11), the Socio-technical shop (10) and the Stress shop (8). Interestingly, none of the firms considered in this sample had a PPSTCpx value near 2, a result that suggests that stress shops that have highly complex environments to schedule were rare for this particular sample.