Abstract
In recent days the quickly changing manufacturing environment has pushed organizations to accomplish more consumer satisfaction by improving item quality, lessening production cost, and acknowledging maintainability. Anomaly recognition impacts the nature of items and it is typically directed through visual quality assessment. The visual quality review of an item can be performed either physically or naturally. This research proposes a novel technique in manufacturing industry-based fault detection and control management using machine learning technique. Here the input data has been collected as manufacturing fault historical data by IoT (internet of things) module. This data has been processed for noise removal, normalization, and smoothening. The processed data features have been extracted by using kernel principal vector component analysis. Gaussian quadratic Kernelized Generative Adversarial Network has been used to manage the control of extracted features. The experimental analysis has been done in terms of RMSE, MAP, AUC, F-1 score, recall, accuracy, and precision. Upon reviewing our procedures after our research and testing, we discovered that Auto-Encoder Neural Network is the best effective algorithm for identifying a production line failure. One-Class SVM gives the highest accurate findings in our machine-based investigations.
Author contributions
Emad H. Abualsauod contributed to the design and methodology of this study, the assessment of the outcomes, and the writing of the manuscript.
Data availability statement
All data generated or analyzed during this study are included in the manuscript.
Additional information
Notes on contributors
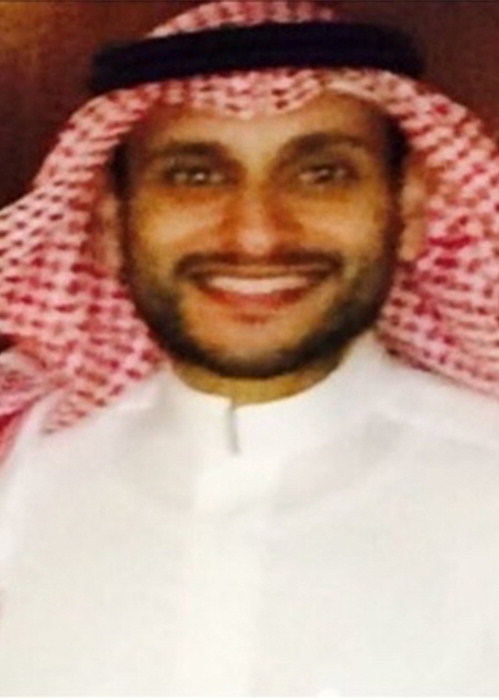
Emad H. Abualsauod
Emad Hashiem Abualsauod is an Associate Professor in Industrial Engineering at Taibah University in Saudi Arabia. He served as the vice dean for development and quality for the College of Engineering at Taibah University. He led the accreditation process for the College of Engineering and served as the chairman and one of the developers of Industrial Engineering Department at Taibah University for more than four years. He was a member of the Deanship for Scientific Research (DSR) council for four years. He is fellow to Advance Higher Academy in UK. He completed his master’s and PhD degrees in 2013 from the Department of Industrial Engineering at the University of Central Florida (UCF) in Orlando, Florida. He was the president of the American Society of Quality-UCF Chapter for four years 2009–2013. Dr. Abualsauod is certified Lean Six sigma Black Belt (CLSSBB), System Engineer (CSE), Project Engineer (CPE), and ISO 9001–2008. He is a member of Institute of Industrial and Systems Engineers (IISE) and Saudi Society for Systems and Industrial Engineering (SSSIE). His research interests lie in quality control, quality assurance, reliability, optimization, modeling, design of experiments, and total quality management and continuous improvement.