ABSTRACT
Desorptive capacity is the organisational ability to transfer internal knowledge to external actors to obtain competitive advantage. We distinguish two forms of desorptive capacity to investigate how organisations active in emerging technology domains explore and exploit opportunities to match internally developed technology to external needs. We also focus on management innovation – the ability to create or revise organisational structures or processes – as a potential antecedent of desorptive capacity. From a study of 84 synthetic biology organisations we found that explorative and exploitative desorptive capacity mediate the relationship between management innovation and external technology commercialisation. The study provides empirical evidence of the existence and interdependence of different managerial and organisational processes required for external technology commercialisation. We underscore how organisations can valorise technological assets strategically through management innovation and by achieving a fit between internal practices and the technological base of potential technology adopters.
1. Introduction
For organisations that are pursuing technological innovation in emerging domains, collaboration is a means of mitigating commercial risks. One specific form of collaboration relates to the commercialisation of internally developed technology through other organisations, a strategy also known as external technology commercialisation (ETC) (Masucci, Brusoni, and Cennamo Citation2020). Connecting with other organisations active in similar technology ecosystems is likely to increase external awareness of an organisation’s technology portfolio (Spigel Citation2017). While the organisational capacity to create and maintain relationships for ETC may be important, it is unclear how this capacity might be developed. Several studies have focused on the need for organisations to have the capability to externalise technologies (e.g. Hu, McNamara, and McLoughlin Citation2015; Lichtenthaler Citation2009), there are still questions regarding the nature of knowledge management processes that enable success in ETC, and also about particular capacities organisations should have to enable such processes (Denford and Ferriss Citation2018). In this study we investigate important aspects of these organisational capacities and their relationship to ETC. Applying the concept of desorptive capacity (DC) – defined as the organisational ability to transfer knowledge strategically to another party (Lichtenthaler and Lichtenthaler Citation2010) – we clarify different types of DC that are required to facilitate ETC. Our findings are particularly relevant for organisations that are active in emerging technology domains, where few external parties are available for ETC and dealing with scarce opportunities requires therefore a higher level of skills and attention.
This paper is structured as follows. First, we construct a theoretical framework in which we distinguish two forms of DC. Explorative DC, on the one hand, is based on having dedicated organisational structures and processes in place to help an organisation explore opportunities for ETC. Exploitative DC, on the other hand, is based on structures and processes oriented towards capitalising on opportunities for ETC that have already been identified. Then, we elaborate on our methodological approach in which we analyse data from a longitudinal survey in the emerging domain of synthetic biology. In this analysis we also test whether the ability of organisations to introduce new or revise organisational structures and processes – an ability referred to as management innovation (Birkinshaw, Hamel, and Mol Citation2008; Volberda, van den Bosch and Mihalache Citation2014) – is related to success in ECT.
2. Theory and hypotheses
2.1. The relationship between external technology commercialisation and desorptive capacity
History has shown that collaboration has often been central in driving technological innovation. Collaboration can provide various benefits for organisations, including lower R&D costs and access to external capital and complementary knowledge (Khanagha et al. Citation2020; Volberda, Oshri and Mom Citation2012). Collaboration can also help organisations to expand their innovation output, commercialise basic research in emerging areas, shorten their time to market, open up new markets, or give themselves greater flexibility in R&D (Gassmann and Enkel Citation2004).
External technology commercialisation (ETC) is the process by which organisations communicate or sell their interim technologies to other interested actors within their value network. ETC enables organisations to generate revenues through technology licensing, to improve their access to other external technologies through cross-licensing, and to develop and diffuse technologies by exploiting positive network externalities (Rivette and Kline Citation2000; Nambisan and Sawhney Citation2011). Additionally, ETC can limit the scope and cost of internal R&D, can positively influence technology standardisation and can provide organisations with feedback from other organisations that are exploiting the technology (Lichtenthaler Citation2006). It is also said to give them the capacity to change their innovation processes to make these more network-centric, and in turbulent environments it allows them to optimise value capture from technology (Gambardella, Giuri, and Luzzi Citation2007).
2.1.1. Knowledge exchange in external technology commercialisation
It has been argued that the soft elements involved in the technology complex should not be underestimated (Fleck and Howells Citation2001), which stresses the importance of knowledge management for realising ETC. Previous studies on open innovation have indicated that the amount of know-how transferred may affect how successful an organisation is at exploiting its intellectual property rights (Ziegler et al. Citation2013). Organisations can harness the power that resides in the transfer of specific knowledge across organisational boundaries (Chesbrough Citation2003) and its diffusion within networks (Müller-Seitz Citation2012). Transferring knowledge across organisational boundaries to inform external parties about an organisation’s technology portfolio can enhance organisational performance (Ahn, Mortara, and Minshall Citation2013). It has also been shown that organisations can strategically steer the complementary activities of external agents and can thus improve their own position in technology ecosystems (Cennamo Citation2018; Masucci, Brusoni, and Cennamo Citation2020). Moreover, ´selective knowledge revealinǵ can be a strategic mechanism to reshape collaborative behaviour in innovation, particularly when there is a high cost of coordination, a high level of uncertainty regarding available suitable partners, and unwillingness among existing partners to collaborate (Alexy, George, and Salter Citation2013).
A related concept that has been developed to understand and manage knowledge flows between organisations is desorptive capacity (Lichtenthaler and Lichtenthaler Citation2010). Complementing absorptive capacity (AC), which is associated with the inward transfer of knowledge that is external to the organisation (Cohen and Levinthal Citation1990), desorptive capacity (DC) is associated with the outward transfer of knowledge that is internal to the organisation. Desorptive capacity can improve access to new markets, increase visibility for an organisation’s technology portfolio, improve technological venturing and supply chain positioning, and influence technology standardisation processes (Lichtenthaler and Lichtenthaler Citation2010; Hu, McNamara, and McLoughlin Citation2015).
2.1.2. Explorative and exploitative desorptive capacity
A number of stages can be identified in connection to outward transfer of knowledge. To commercialise technologies externally, organisations require the capability to identify and evaluate opportunities for commercialisation. Then, it is important for them to have the capability to utilise such opportunities so that they can satisfy customer needs. This potential multidimensionality of desorptive capacity was also shown by other studies focusing on the relationship between ambidexterity and organisational performance in supply chains (Yang et al. Citation2014; Roldán Bravo et al. Citation2019). Desorptive capacity is not just about the actual transfer of knowledge, but it also concerns protecting that knowledge to secure core competitive advantages for an organisation. Moreover, processes such as opportunity identification, exploration, exploitation, and knowledge transfer are essential throughout the process (Lichtenthaler and Lichtenthaler Citation2010; Ziegler et al. Citation2013).
Thus, in the context of ETC, an organisation’s desorptive capacity can be divided into two distinct types:
Explorative desorptive capacity mainly concerns finding opportunities for ETC. Organisations need information on how and where ETC can be achieved. As there are relatively few organisations involved in these new technologies and their activities can be difficult to spot, organisations require the capacity to search for and identify opportunities for ETC. Organisations need to monitor external parties to understand more about the competences and resources other organisations require. Additionally, they must be able to present their technologies effectively to external parties to initiate potential ETC projects.
Exploitative desorptive capacity mainly concerns capitalising on the identified opportunities for ETC. Once the technology has been presented to external parties and new ETC projects have been initiated, organisations need to deepen their knowledge of potential partners. Organisational settings and mechanisms are managed to facilitate external technology commercialisation projects and develop relations with external partners for ETC. Through periodic meetings, the impact of ETC on the products and services of external parties can be discussed. Also, coordinated interaction with external parties helps organisations to refine the development of their internal technology so that they can meet evolving external technological needs and provide better customer value.
As it is likely that organisations active in emerging technology domains will have a strong incentive to realise ETC, they need dedicated structures and processes to both explore and exploit opportunities for ETC. We therefore hypothesise that, for organisations active in these domains, both explorative DC and exploitative DC will be positively related to success in ETC.
Hypothesis 1: For organisations involved in emerging technology domains, explorative desorptive capacity and exploitative desorptive capacity are positively related to successful external technology commercialisation.
2.2. The relationship between management innovation, desorptive capacity, and external technology commercialisation
Processes and structures that enable organisations to inform external parties about their technological offerings – i.e. explorative and exploitative desorptive capacity – are crucial for organisations oriented towards ETC. However, a changing external environment can influence the conditions under which organisations can collaborate and they might therefore need to reassess or revise the processes and structures used for this. For organisations active in emerging technology domains, which are by nature dynamic and unstable, the ability to adapt has been argued to be particularly important (Volberda Citation1996; Khanagha et al. Citation2018). Under such conditions, the notion of fit – the degree to which organisational practices involved in technology development are compatible with the technological base and requirements of potential adopters – needs to be considered.
Ensuring a fit with multiple heterogeneous receivers of knowledge requires continuous reconsideration of organisational systems, processes, and practices, which has been referred to as management innovation. Management innovation (MI) is the organisational ability to introduce managerial initiatives that result in new or revised organisational strategies, structures, administrative procedures, and systems (Damanpour and Aravind Citation2012). In the development of emerging technologies, MI is likely to become increasingly relevant (Khanagha, Volberda, and Oshri Citation2017). It has been argued not only to complement technological innovation (Khanagha et al. Citation2013) but even to make technological innovation work and to drive competitive advantage (Birkinshaw, Hamel, and Mol Citation2008; Volberda, van den Bosch and Mihalache Citation2014). The relationship between MI and open innovation has been explored in other contexts as well. For example, in a study of SMEs, it was shown that several characteristics of a CEO – such as positive attitude, entrepreneurial orientation, patience, or educational background – can play differing roles in facilitating various aspects of open innovation (Ahn, Minshall, and Mortara Citation2017).
2.2.1. Management innovation and environmental dynamism
MI might facilitate a dynamic fit between organisational routines and the market for knowledge, and may thus be important for organisational performance. As discussions on organisational fit have considered learning processes and knowledge transfer to provide ways of managing uncertainty (Bikhchandani, Hirshleifer, and Welch Citation1998), MI might be crucial and particularly for improving and maintaining the DC required for ETC. As ETC requires both communication and collaboration with a wide and changing range of partners, organisations will require the ability to assess and revise the processes and structures they use to identify stakeholder needs and bring on board potential partners. Organisations need to be able to assess their core capabilities and business models by evaluating what technological know-how they are importing or exporting, enabling them then to adjust those models and capabilities as needed to fit with changing business environments (Grönlund, Sjödin, and Frishammar Citation2010). It can therefore be argued that because MI can potentially enable organisations to critically assess and adjust their DC processes and structures, it is important for realising ETC.
While MI is often a response to environmental dynamism, the actions taken are focused mainly on internal operations. It is not likely that there is a direct causal relationship between MI and ETC; it is more likely that other abilities help to create a fit between them. Organisations often need knowledge-based capacities to link internally oriented abilities to those that are more externally oriented. For example, one study showed that organisational learning can mediate the relationship between investment in IT technology and organisational performance (Tippins and Sohi Citation2003). For ETC, processes related to explorative and exploitative desorptive capacity may benefit from a flexibility and orientation towards change that MI provides.
It is therefore likely that MI will have an indirect effect on ETC that is mediated by DC. Organisations require the capacity to purposefully extend their resource base and reconfigure their existing knowledge interfaces to adapt to changing environments and external needs (Helfat et al. Citation2007; Volberda, Foss and Lyles Citation2010). Therefore, we argue that:
Hypothesis 2: For organisations involved in emerging domains, explorative desorptive capacity and exploitative desorptive capacity mediate the relationship between management innovation and external technology commercialisation.
3. Data and methodological approach
3.1. Operationalisation of the conceptual model
The conceptual model was operationalised using one dependent variable (external technology commercialisation), one independent variable (management innovation), two mediating variables (explorative and exploitative desorptive capacity) and two control variables (organisation size and organisation age) (Appendix, ). Constructs were based on literature review and expert assessment (Bagozzi Citation1994), and were partly adapted from existing related scales. Feedback was obtained from various experts who internally reviewed and pre-tested the designed constructs. This feedback was used to optimise the comprehensiveness and interpretation of the final set of items.
The designed constructs were validated to determine their quality (Churchill Citation1979; Gerbing and Anderson Citation1988; Straub and Gefen Citation2004). Convergent and discriminant validity tests were performed using multi-trait scaling. Analysing the correlations of items within and between constructs enabled us to optimise the selection of items used for structural equation modelling (, Appendix). Additionally, we carried out Kaiser-Meyer-Olkin (KMO) tests to measure sampling adequacy by assessing the degree of collinearityFootnote1 of the designed constructs (Tabachnick and Fidell Citation2012). Cronbach’s alpha was calculated to measure internal consistency (Cronbach Citation1961). To determine the fit of the measured constructs (Cheung and Rensvold Citation2002), a confirmatory factor analysis was conducted using the comparative fit index (CFI) and the Tucker Lewis index (TLI). The root mean square error of approximation (RMSEA) was measured to evaluate the fit of the data to the constructs (Hu and Bentler Citation1999).
An analysis of non-response bias was performed by comparing the sample data to other external data sources on the characteristics of the sampled organisations. Common method bias was assessed using a Harman’s single factor score to test whether variations in the data collected could potentially be caused by our data collection instrument (Podsakoff et al. Citation2003).
All items used a five-point scale, ranging from (1) ‘totally disagree’ to (5) ‘totally agree’. Variables were calculated by averaging the combined items, including a correction for reversed items.
shows the model, including the hypothesised relationships.
Figure 1. Conceptual model regarding the relationship between management innovation, desorptive capacity, and external technology commercialization.
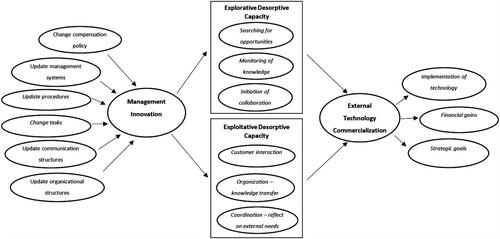
3.1.1. Dependent variable – external technology commercialisation
For ETC, we designed a construct that covered three key aspects:
Implementation: The commercialisation of technology has been shown to vary substantially across organisations and industry sectors (Chesbrough Citation2003) and to be influenced by the presence of technology brokers and by an organisation’s access to knowledge and intellectual property rights (Howells Citation2006).
Financial gains: ETC can generate revenues for an organisation through technology licensing (Rivette and Kline Citation2000), and can also capture technology-based value in highly turbulent settings (Gambardella, Giuri, and Luzzi Citation2007).
Strategic goals: ETC has been argued to play a potentially important part within an organisation’s overall strategy (Arora, Fosfuri, and Gambardella Citation2001), especially in terms of how innovation networks are used (Nambisan and Sawhney Citation2011; Gambardella, Giuri, and Luzzi Citation2007).
These aspects were converted into three items (Appendix, ). Factor analysis (principle component analysis; KMO = 0.68) indicated a determinant of 0.26. The construct had a good reliability (α = 0.84).Footnote2 Confirmatory factor analysis indicated a good fit.
3.1.2. Independent variable – management innovation
For MI, we used a construct developed by Vaccaro et al. (Citation2012), building on an article by Birkinshaw, Hamel, and Mol (Citation2008). MI consisted of six items, representing six areas of organisational management. MI measured the extent to which these areas were changed or updated. Factor analysis (principle component analysis; KMO = 0.85) indicated a determinant of 0.09. Reliability of the construct was α = 0.85. Confirmatory factor analysis indicated a good fit (one factor, CFI = 0.998, TLI = 0.997, RMSEA = 0.02).
3.1.3. Mediating variables – explorative and exploitative desorptive capacity
Given the importance of two different forms of desorptive capacity (explorative and exploitative) for ETC, we designed two separate constructs. Both constructs were designed by adapting validated scales for measuring absorptive capacity (Jansen, van den Bosch, and Volberda Citation2005). We adapted certain items to address specifically the outbound transmission of internal knowledge.
For explorative DC, we designed a construct to measure how organisations acquire information on the availability and suitability of potential partners for collaboration, and to whom they can then transfer knowledge regarding opportunities for ETC. The construct also measured the intensity of interactions with potential collaboration partners, as well as the ability of those partners to analyse and comprehend the knowledge transferred to them. The construct covered:
Searching: To identify opportunities for ETC, organisations need to pursue knowledge (March Citation1991) and look out for potential collaboration partners.
Monitoring: Organisations need to screen the knowledge they obtain and interact with potential collaboration partners so that they can determine which ones will be best (Stiglitz Citation2002).
Initiation: Once suitable partners have been identified for ETC, organisations need to set up possible collaboration projects and disclose specific knowledge relating to the technology they are developing (Fontana, Geuna, and Matt Citation2006).
These aspects were converted into six items, but after multi-trait scaling the number was reduced to four (Appendix, and ). Factor analysis (principle component; KMO = 0.68) indicated a determinant of 0.44 and no multicollinearity. Reliability was α = 0.69. Confirmatory factor analysis indicated a good fit (one factor, CFI = 0.981, TLI = 0.942, RMSEA = 0.086).
For exploitative DC, we designed a construct to measure the extent to which organisations are able to strategically transform the knowledge acquired into opportunities for ETC, and the extent to which they are able to utilise the identified opportunities. The construct covered:
Customer interaction: Interaction with customers allows organisations to refine and add to their existing knowledge so that they can provide additional or better value to customers (March Citation1991).
Organisational setting and mechanisms: Organisations require specific systems and procedures for transferring knowledge and arriving at rational ways of dealing with operational uncertainty (Bikhchandani, Hirshleifer, and Welch Citation1998).
Coordination of personal tasks: To recognise the needs of external actors and facilitate internal reflection on such knowledge, the tasks of the staff involved need to be well coordinated (Cohen and Levinthal Citation1990).
These aspects were converted into seven items (Appendix, ). Factor analysis (principle component; KMO = 0.85) indicated a determinant of 0.070 and no multicollinearity. Reliability was α = 0.83. Confirmatory factor analysis indicated a good fit (one factor, CFI = 0.957, TLI = 0.935, RMSEA = 0.085).
3.1.4. Control variable
Organisation size was used as control variable. Respondents were asked how many employees work within the organisation (full-time equivalents). It is assumed that larger organisations are less adaptable than smaller organisations, implying a potential relationship between organisation size and MI in emerging technology domains. Due to such increased flexibility of smaller organisations, they are also more likely to adopt open innovation practices and have fewer barriers to knowledge sharing (Tranekjer and Knudsen Citation2012). Furthermore, as the development of emerging technology is relatively resource-intense, it is likely that larger organisations are more able to keep R&D processes in-house. Also, larger organisations might be expected to have more diverse portfolios, containing more established technologies, compared to smaller organisations.
3.1.5. Total measurement model
We conducted confirmatory factor analysis for the complete measurement model (four factors, CFI = 0.845, TLI = 0.820, RMSEA = 0.095). Given our sample size and the relatively small number of items measured within the constructs, the model fit can be considered adequate. We carried out an additional test to determine the multidimensional nature of desorptive capacity. For this, we analysed whether the model fit might be better if the variables measuring explorative and exploitative DC were combined into a single multidimensional construct. Analysis showed that with a multidimensional construct of this kind, the model fitted less well (three factors, CFI = 0.840, TLI = 0.818, RMSEA = 0.095).Footnote3
3.2. Data collection and research context
3.2.1. Survey methodology
A survey was used to collect the data (Tanur Citation1983). To reduce measurement error and social desirability, the survey was pre-tested and reformulated after being reviewed by experts (Bagozzi Citation1994). Data for this study were collected using the online survey tool Globalpark-Questback.Footnote4 The questionnaire was directed to collect data at the organisation level. Reflecting the emerging nature of synthetic biology and the distinctive technologies involved, conventional databanks containing organisational profiles do not offer an adequate way of sampling organisations working in synthetic biology. The LinkedIn Synthetic Biology Interest Group (SyBIG) was therefore used to identify and make contact with synthetic biology organisations.
3.2.2. Measurements and sample characteristics
As there can be both sequential and synchronous patterns in the relationship between MI and technological innovation (Damanpour Citation2014), we collected longitudinal data, taking two measurements approximately one year apart. Having a second measurement enables us to correct for potential time lags between MI, DC, and ETC. Moreover, using multiple measurements will reduce bias and response variability.
3.2.2.1. First and second measurements
The first measurement included all the variables and constructs relating to our model. All 1690 members of the LinkedIn SyBIG interest group were invited to participate in the survey, representing 961 organisations. In total 134 organisations responded (14%); representatives from 84 of these organisations subsequently completed all questions relating to the constructs analysed (completion rate of 63%). Non-response bias analysis indicated that the characteristics of respondents corresponded to the expected characteristics of actors involved in synthetic biology R&D (van Doren, Koenigstein, and Reiss Citation2013). provides an overview of the sample characteristics.
Table 1. Characteristics of the sample with organisations completing the first measurement (n = 84).
For the second measurement, we only approached those organisations that had completed the first measurement. Our second measurement only concerned ETC. In total, 48 of the 84 organisations that had completed the first measurement participated in the second measurement (56%). No significant difference between the two measurements of ETC was found. Also, the two measurements significantly correlated. As no additional explanatory power could be extracted from the second measurement, only the first measurement was used for later structural equation modelling.
3.3. Structural equation modelling
The conceptual model () was tested by means of covariance-based structural equation modelling. The mediation model was tested using Baron and Kenny’s approach; conditions for mediation were met and no evidence of feedback was found (Baron and Kenny Citation1986).Footnote5 To assign accuracy measures to the sample estimates (Tenenhaus et al. Citation2005), we undertook bootstrapping with 2000 bootstrap samples and 95% bias-corrected confidence intervals.
4. Results
gives an overview of the means, standard deviations, and correlations for the relevant variables. Given the high correlation between explorative DC and exploitative DC (0.72), we calculated the variance inflation factors (VIF). As the VIFs for both explorative DC (VIF = 2.1) and exploitative DC (VIF = 2.2) were below 5, we found no evidence of multicollinearity and both variables could be included in the structural equation model. and show the effect sizes and t-values for the estimated paths.
Figure 2. Structural equation model. Effect sizes: DE = direct effect; TE = total effect; IE = indirect effect. The total effect (consisting of both the direct and indirect effect) is given only when an indirect effect was measured. For the relationship between management innovation and external technology commercialization, only the indirect effect is given (the direct effect was found to be insignificant). *** = p < 0.001; **p < 0.01; * p < 0.05; † p < 0.1. Organization age was used to control the dependent variable.
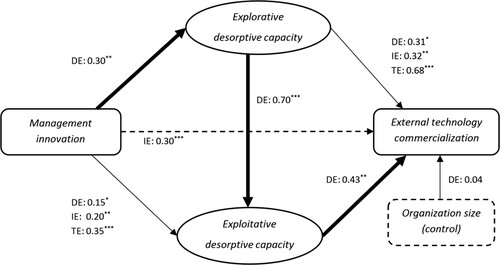
Table 2. Mean, standard deviation (S.D.), and correlations.
Table 3. Regression analysis.
5. Discussion
The analysis indicates that there is a positive relationship between DC and ETC for organisations involved in synthetic biology. This finding is in line with the notion that there are benefits to be gained from technological co-development (Carlson Citation2010), and also accords with similar partnering and clustering structures observed within the bio-pharmaceutical sector (Jones Citation2000). It is likely that many of these developments have co-evolved with organisational strategy oriented towards external relationships, the introduction of dedicated budgets for managing collaborations, and the reorganisation of internal practices to optimise external innovation activities (Bianchi et al. Citation2011).
The observed relationship between DC and ETC was especially strong in terms of the total effect of explorative DC on ETC. This finding shows that organisations in emerging technology domains give particular priority to identifying opportunities to collaborate on ETC. Various studies have indeed shown that organisations are more likely to invest only in developing those open innovation capacities that will meet organisational goals (Denford and Ferriss Citation2018). However, the strong relationship between explorative DC and exploitative DC – combined with exploitative DC’s significant effect on ETC – indicates that organisations also invest in how identified opportunities can be valorised, for example in technology commercialisation projects and meetings with interested partners.
The analysis also confirms that MI can indirectly influence organisational success in ETC. As we found it to have no direct effect on ETC, it provides evidence that DC processes play a mediating role in the relationship between MI and ETC. This finding has at least two implications First, it confirms the multidimensionality of MI in relation to other organisational variables (Volberda, van den Bosch, and Heij Citation2013), and also supports previous more implicit findings that there might be an interplay between processes relating to MI, DC, and ETC (Walker, Damanpour, and Devece Citation2010). Second, there seems a certain directionality in these relationships. Although MI was found to have a significant direct effect on both explorative and exploitative DC, the direct effect of MI on explorative DC was considerably stronger.
This suggests exploration to be an important prerequisite for exploitation in the context of ETC. Without processes that enable proper search and identification of appropriate opportunities for collaboration, organisations seem unlikely to be able to sufficiently assess, coordinate, or manage potential partnerships and to be successful in ETC. However, the analysis also indicated that explorative DC has a strong indirect effect on ETC. This emphasises the mediating role of exploitative DC. Although it is not necessarily essential for organisations to have exploitative DC to succeed at ETC, having this capacity does seem to increase their chances of success.
6. Contributions
In general terms, our study on the emerging technology domain of synthetic biology provides a valuable empirical contribution to overall research on open innovation. Although previous studies have discussed how the effectiveness and adoption of open innovation are likely to be dependent on industry characteristics and business environments (Chesbrough and Crowther Citation2006), many of these studies have been conducted in more established technological sectors. There are limited empirical and context-specific studies available to verify whether these arguments also hold for emerging technology domains.
More specifically, the insights from our analysis enable us to make several theoretical contributions to the research field of outbound open innovation. Inherent to the concept of DC is the notion that various processes and capabilities are required for organisations to transfer technological assets successfully to external parties. Our results show that there is a positive relationship between having such a capacity and being successful in ETC. Additionally, this study aimed to bring out the multidimensionality of DC. This makes some elements of DC more explicit for analysis and can give us better understanding how these elements interact with each other. By distinguishing between explorative and exploitative DC, we were able to identify new causal and mediating relationships between organisational processes. Also, our study shows that MI can have an important indirect effect on ETC. As MI is mainly about internal operation, our study shows that both explorative and exploitative DC can mediate its effect on ETC. Instead of merely exploiting existing partnerships, organisations are able to achieve fit by using MI to identify new opportunities for collaboration and to diffuse technological knowledge and distribute assets.
Our study has managerial implications. It shows that where organisations make a strategic decision to pursue ETC, their success will depend on having the capacity to explore and exploit relevant opportunities. This might require investment in developing dedicated organisational structures. To make internally developed technology suitable for others, an organisation must be able to critically assess prospective partners regarding their potential value for the organisation. Also, such partners should be taken on board early-on in the development of a technology to avoid the costs of its misalignment with stakeholder needs. In addition to building capacity for outbound transfer of knowledge and technology, managers interested in ETC should try to create flexible structures that allow for both organisational routines and more novel practices. By allowing existing processes to be adapted in line with changing environmental conditions, an organisation is likely to increase its chances of aligning its explorative and exploitative DC processes with the needs of potential clients, thereby increasing its chances of succeeding at ETC.
The study also indirectly indicates that organisations must think carefully about their decision to go for ETC. The strong relationship between MI, DC, and ETC means that success in ETC is likely to require considerable investment in both DC and MI. Particularly for organisations that want to follow an open innovation strategy but are not yet able to do so, managers need to assess carefully whether they will be able to invest sufficiently in the desorptive capacity required. In situations where there is a high degree of uncertainty, the short-term benefits of creating a capacity for open innovation within the organisation might not justify the investment needed to interact with potential partners (Khanagha, Volberda, and Oshri Citation2017).
7. Limitations
When considering our findings, one needs to note the specific context in which the study was conducted, and conclusions should therefore be treated cautiously. While the use of LinkedIn is widespread among individuals and organisations involved in business and technology development, its integration into research designs for sampling organisations is novel.
Additionally, our sample was relatively small, giving us only limited data for analysis. Data availability challenges are inevitable when one wants to analyse emerging technology domains. There has been much discussion within the scientific community regarding required sample sizes (e.g. Tabachnick and Fidell Citation2012), with various studies arguing that there are conditions in which smaller sample sizes can be used for analysis when combined with bootstrapping (Preacher and Hayes Citation2004). Considering the relatively simple and unmoderated model underlying our hypotheses, the sample size is therefore unlikely to have influenced our key findings.
Another point that requires attention relates to the two forms of DC that were analysed. Although the analysis showed that both explorative and exploitative DC could be regarded as distinct forms of DC, it also showed a strong relationship between them. Future research could try to make an even finer distinction between the various organisational processes and structures relating to DC. This would help to specify the DC concept more precisely and would give organisations more insight into how they could optimise their DC.
8. Conclusion
Innovation has long been considered important in enabling organisations to improve their chances of survival and success. If one holds to the view that collaboration can help companies to mitigate the commercial risks associated with emerging technology, it seems likely that those working in these domains need to devise processes that make them better at realising outbound open innovation. Our analysis suggests that both MI and DC processes matter for the external commercialisation of technology. Our findings could be built on by studies that investigate the relationship between MI, DC, and ETC in both other emerging technology domains and mature technological domains. Through such efforts, the scientific basis for realising outbound open innovation is likely to strengthen.
Disclosure statement
No potential conflict of interest was reported by the author(s).
Additional information
Notes on contributors
Davy van Doren
Davy van Doren has an academic background in both biology and sustainable development. As a researcher, he has worked on a broad range of projects related to marine biodiversity, ecosystem conservation and climate change communication, as well as to transitions related to renewable energy and the bioeconomy. In recent years, his research has focused on technological and non-technological aspects associated to technological innovation, including issues related to public policy, organizational management and responsible research and innovation (RRI). His PhD research has a strong focus on the emerging domain synthetic biology. In this interdisciplinary research, he applies different methodological approaches for addressing various organizational, industrial, and socio-technological challenges.
Saeed Khanagha
Saeed Khanagha is Associate professor of Strategy at VU Amsterdam and his research is broadly focused on understanding the factors that determine success of organization in adoption of emerging digital technologies at the individual, team, organization, and ecosystem levels, using a variety of qualitative and quantitative data methods including single case study, multiple cases studies, survey, and experimental design. His ongoing research mostly explores the different dimensions of strategizing for digital technologies, particularly digital platforms and ecosystems. He has twice received prestigious Marie Curie Horizon 2020 grants, each 1.3 M Euro, and currently leads these projects. Dr. Khanagha has close collaborations with academic and industrial organizations, including having guest researcher positions at Rotterdam School of Management, Leeds University Business School, Ericsson, and Atos. His research has been published in outlets such as Strategic Management Journal, Journal of Management Studies, and Long Range Planning. He is a member of editorial board and currently serves as organizer and guest editor of a Long Range Planning special issue entitled Strategizing in a digital era.
Henk W. Volberda
Henk W. Volberda is Professor of Strategic Management & Innovation at Amsterdam Business School of the University of Amsterdam. Moreover, he is Director of the Amsterdam Centre for Business Innovation. He has held visiting scholarships at the Wharton School of the University of Pennsylvania, Duke University's Fuqua School of Business and at Cass Business School in London. Volberda holds various advisory positions such as member of the supervisory board of NXP Semiconductors Netherlands, expert member of the World Economic Forum and fellow of the European Academy of Management. His research on technological disruption, coevolution, new business models, strategic flexibility, and management innovation has led to an extensive number of publications in international peer-reviewed journals including the Academy of Management Journal, Journal of Management, Journal of Management Studies, Journal of International Business Studies, Management Science, Organization Science, and Strategic Management Journal. He is a member of the editorial boards of Long Range Planning, Journal of Strategy and Management, Management and Organization Review and Organization Studies.
Marjolein C. J. Caniëls
Marjolein C. J. Caniëls is Professor Organizational Learning at the Open Universiteit (the Netherlands). She is in charge of the research program “Learning” at the Faculty of Management. Furthermore, she is program director of the Master of Science in Management. Her main fields of interests are organizational learning, sustainability and technological development & economic growth. She has published a wide array of articles in many international peer-reviewed scientific journals including the International Entrepreneurship & Management Journal, Research Policy and Technovation.
Notes
1 We applied the rule of thumb of KMO being greater than 0.6 (e.g. see https://www.statisticshowto.datasciencecentral.com/kaiser-meyer-olkin/).
2 Calculating fit: CFI = 1.00, TLI = 1.00, RMSEA = 0.00. These fit measures occur since there are three variables (6 elements in the covariance matrix) measuring one latent variable (having 6 elements), implying 0 degrees of freedom. However, based on the internal consistency of the construct, it is assumed to be appropriate.
3 CFA of the multidimensional DC construct alone: one factor, CFI = 0.880, TLI = 0.850, RMSEA = 0.108. The decrease in fit is only modest, which can be explained by the relatively high correlation between explorative DC and exploitative DC ().
4 See https://www.questback.com/.
5 See also http://davidakenny.net/cm/mediate.htm and Kenny and Judd (Citation2014).
References
- Ahn, J. M., T. Minshall, and L. Mortara. 2017. “Understanding the Human Side of Openness.” R&D Management 47 (5): 727–740.
- Ahn, J., L. Mortara, and T. H. W. Minshall. 2013. “The Effects of Open Innovation on Firm Performance. Science.” Technology and Innovation Policy Review 4 (1): 79–93.
- Alexy, O., G. George, and A. J. Salter. 2013. “Cui Bono? The Selective Revealing of Knowledge and Its Implications for Innovative Activity.” Academy of Management Review 38: 270–291.
- Arora, A., A. Fosfuri, and A. Gambardella. 2001. Markets for Technology. Cambridge, MA: MIT Press.
- Bagozzi, R. 1994. “Measurement in Marketing Research.” In Principle of Marketing Research, edited by R. Bagozzi, 1–10. Oxford: Blackwell.
- Baron, R. M., and D. A. Kenny. 1986. “The Moderator-Mediator Variable Distinction in Social Psychological Research.” Journal of Personality and Social Psychology 51 (6): 1173–1182.
- Bianchi, M., A. Cavaliere, D. Chiaroni, F. Frattini, and V. Chiesa. 2011. “Organisational Modes for Open Innovation in the Bio-Pharmaceutical Industry.” Technovation 31 (1): 22–33.
- Bikhchandani, S., D. Hirshleifer, and I. Welch. 1998. “Learning from the Behavior of Others.” Journal of Economic Perspectives 12 (3): 151–170.
- Birkinshaw, J., G. Hamel, and M. J. Mol. 2008. “Management Innovation.” Academy of Management Review 33 (4): 825–845.
- Carlson, R. H. 2010. Biology is Technology. Cambridge, MA: Harvard University Press.
- Cennamo, C. 2018. “Building the Value of Next-Generation Platforms.” Journal of Management 44 (8): 3038–3069.
- Chesbrough, H. W. 2003. Open Innovation: The New Imperative for Creating and Profiting from Technology. Edited by H. W. Chesbrough, W. Vanhaverbeke, and J. West. Brighton, MA: Harvard Business School Press.
- Chesbrough, H. W., and A. K. Crowther. 2006. “Beyond High Tech: Early Adopters of Open Innovation in Other Industries.” R&D Management 36 (3): 229–236.
- Cheung, G. W., and R. B. Rensvold. 2002. “Evaluating Goodness-of-Fit Indexes for Testing Measurement Invariance.” Structural Equation Modeling: A Multidisciplinary Journal 9 (2): 233–255.
- Churchill, G. A. 1979. “A Paradigm for Developing Better Measures of Marketing Constructs.” Journal of Marketing Research 16 (1): 64–73.
- Cohen, W. M., and D. A. Levinthal. 1990. “Absorptive Capacity: A New Perspective on Learning and Innovation.” Administrative Science Quarterly 35 (1): 128–152.
- Cronbach, L. J. 1961. Essentials of Psychological Testing. New York: Harper and Row.
- Damanpour, F. 2014. “Footnotes to Research on Management Innovation.” Organization Studies 35 (9): 1265–1285.
- Damanpour, F., and D. Aravind. 2012. “Managerial Innovation: Conceptions.” Processes, and Antecedents. Management and Organization Review 8 (2): 423–454.
- Denford, J. S., and A. Ferriss. 2018. “Absorption, Combination and Desorption.” Journal of Knowledge Management 22 (7): 1425–1441.
- Fleck, J., and J. Howells. 2001. “The Technology Complex and the Paradox of Technological Determinism.” Technology Analysis and Strategic Management 13 (4): 523–531.
- Fontana, R., A. Geuna, and M. Matt. 2006. “Factors Affecting University-Industry R&D Projects.” Research Policy 35 (2): 309–323.
- Gambardella, A., P. Giuri, and A. Luzzi. 2007. “The Market for Patents in Europe.” Research Policy 36 (8): 1163–1183.
- Gassmann, O., and E. Enkel. 2004. Towards a Theory of Open Innovation: Three Core Process Archetypes. University of St.Gallen.
- Gerbing, D. W., and J. C. Anderson. 1988. “An Updated Paradigm for Scale Development Incorporating Unidimensionality and Its Assessment.” Journal of Marketing Research 25 (2): 186–192.
- Grönlund, J., D. R. Sjödin, and J. Frishammar. 2010. “Open Innovation and the Stage-Gate Process: A Revised Model for New Product Development.” California Management Review 52: 106–131.
- Helfat, C. E., S. Finkelstein, W. Mitchell, M. A. Peteraf, H. Singh, D. J. Teece, and S. G. Winter. 2007. Dynamic Capabilities: Understanding Strategic Change in Organizations. Oxford: Blackwell Publishing.
- Howells, J. 2006. “Intermediation and the Role of Intermediaries in Innovation.” Research Policy 35 (5): 715–728.
- Hu, L., and P. M. Bentler. 1999. “Cutoff Criteria for fit Indexes in Covariance Structure Analysis.” Structural Equation Modeling: A Multidisciplinary Journal 6 (1): 1–55.
- Hu, Y., P. McNamara, and D. McLoughlin. 2015. “Outbound Open Innovation in bio-Pharmaceutical out-Licensing.” Technovation 35: 46–58.
- Jansen, J. J. P., F. a J. van den Bosch, and H. W. Volberda. 2005. “Managing Potential and Realised Absorptive Capacity: How Do Organisational Antecedents Matter?” Academy of Management 48 (6): 16.
- Jones, O. 2000. “Innovation Management as a Postmodern Phenomenon: The Outsourcing of Pharmaceutical R&D.” British Journal of Management 11 (4): 341–356.
- Kenny, D. A., and C. M. Judd. 2014. “Power anomalies in testing mediation” Psychological Science. 25: 334–339.
- Khanagha, S., S. Ansari, S. Paroutis, and L. Oviedo. 2020. “Mutualism and the Dynamics of New Platform Creation: A Study of Cisco and Fog Computing.” Strategic Management Journal 31. 10.1002/smj.3147.
- Khanagha, S., M. T. Ramezan Zadeh, O. R. Mihalache, and H. W. Volberda. 2018. “Embracing Bewilderment: Responding to Technological Disruption in Heterogeneous Market Environments.” Journal of Management Studies 55 (7): 1079–1121.
- Khanagha, S., H. W. Volberda, and I. Oshri. 2017. “Customer Co-Creation and Exploration of Emerging Technologies.” Long Range Planning 50 (2): 221–242.
- Khanagha, S., H. W. Volberda, J. Sidhu, and I. Oshri. 2013. “Management Innovation and Adoption of Emerging Technologies.” European Management Review 10 (1): 51–67.
- Lichtenthaler, U. 2006. “External Technology Commercialisation as an Alternative Mode of Technology Marketing.” International Journal of Technology Marketing 1 (4): 411–430.
- Lichtenthaler, U. 2009. “Outbound Open Innovation and Its Effect on Firm Performance: Examining Environmental Influences.” R&d Management 39 (4): 317–330.
- Lichtenthaler, U., and E. Lichtenthaler. 2010. “Technology Transfer Across Organizational Boundaries: Absorptive Capacity and Desorptive Capacity.” California Management Review 53 (1): 154–170.
- March, J. G. 1991. “Exploration and Exploitation in Organizational Learning.” Organization Science 2 (1): 71–87.
- Masucci, M., S. Brusoni, and C. Cennamo. 2020. “Removing Bottlenecks in Business Ecosystems.” Research Policy 49 (1): 1–17.
- Müller-Seitz, G. 2012. “Absorptive and Desorptive Capacity-Related Practices at the Network Level.” R&D Management 42 (1): 90–99.
- Nambisan, S., and M. Sawhney. 2011. “Orchestration Processes in Network-Centric Innovation.” Academy of Management Perspectives 25: 40–57.
- Podsakoff, P. M., S. B. MacKenzie, J. Y. Lee, and N. P. Podsakoff. 2003. “Common Method Biases in Behavioral Research.” Journal of Applied Psychology 88 (5): 879–903.
- Preacher, K. J., and A. F. Hayes. 2004. “SPSS and SAS Procedures for Estimating Indirect Effects in Simple Mediation Models.” Behavior Research Methods, Instruments, and Computers 36 (4): 717–731.
- Rivette, K. G., and D. Kline. 2000. “Discovering New Value in Intellectual Property.” Harvard Business Review 78 (1): 54–66.
- Roldán Bravo, M. I., M. Stevenson, A. R. Moreno, and F. J. Lloréns Montes. 2019. “Absorptive and Desorptive Capacity Configurations in Supply Chains.” International Journal of Production Research 58 (7): 2036–2053.
- Spigel, B. 2017. “The Relational Organization of Entrepreneurial Ecosystems.” Entrepreneurship Theory and Practice 41 (1): 49–72.
- Stiglitz, J. E. 2002. “Information and the Change in the Paradigm in Economics.” American Economic Review 92 (3): 460–501.
- Straub, D., and D. Gefen. 2004. “Validation Guidelines for IS Positivist Research.” Communications of the Association for Information Systems 24: 380–427.
- Tabachnick, B. G., and L. S. Fidell. 2012. Using Multivariate Statistics. 6th ed. New York: Harper and Row.
- Tanur, J. M. 1983. “Methods for Large-Scale Surveys and Experiments.” Sociological Methodology 14: 1–71.
- Tenenhaus, M., V. E. Vinzi, Y. M. Chatelin, and C. Lauro. 2005. “PLS Path Modeling.” Computational Statistics and Data Analysis 48 (1): 159–205.
- Tippins, M. J., and R. S. Sohi. 2003. “IT Competency and Firm Performance: Is Organizational Learning a Missing Link?” Strategic Management Journal 24 (8): 745–761.
- Tranekjer, T. L., and M. P. Knudsen. 2012. “The (Unknown) Providers to Other Firms New Product Development.” Journal of Product Innovation Management 29: 986–999.
- Vaccaro, I. G., J. J. P. Jansen, F. A. J. van den Bosch, and H. W. Volberda. 2012. “Management Innovation and Leadership.” Journal of Management Studies 49 (1): 28–51.
- van Doren, D., S. Koenigstein, and T. Reiss. 2013. “The Development of Synthetic Biology.” Systems and Synthetic Biology 7 (4): 209–220.
- Volberda, H. W. 1996. “Toward the Flexible Form: How to Remain Vital in Hypercompetitive Environments.” Organization Science 7: 359–374.
- Volberda, H.W., N.J. Foss, and M.A. Lyles. 2010. “Absorbing the Concept of Absorptive Capacity: How to Realize Its Potential in the Organization Field.” Organization Science 21 (4): 931–951.
- Volberda, H.W., I. Oshri, and T. Mom. 2012. “Technology transfer: the practice and the profession.” Technology Analysis and Strategic Management, Special Issue 24 (9): 863–870.
- Volberda, H. W., F. A. J. van den Bosch, and C. V. Heij. 2013. “Management Innovation: Management as Fertile Ground for Innovation.” European Management Review 10 (1): 1–15.
- Volberda, H.W., F.A.J. Van den Bosch, and O. Mihalache. 2014. “Advancing Management Innovation: Synthesizing Processes, Levels of Analysis, and Change Agents.” Organization Studies 35 (9): 1245–1264.
- Walker, R. M., F. Damanpour, and C. A. Devece. 2010. “Management Innovation and Organizational Performance.” Journal of Public Administration Research and Theory 21 (2): 367–386.
- Yang, S. M., S. C. Fang, S. R. Fang, and C. H. Chou. 2014. “Knowledge Exchange and Knowledge Protection in Interorganizational Learning.” Industrial Marketing Management 43 (2): 346–358.
- Ziegler, N., F. Ruether, M. A. Bader, and O. Gassmann. 2013. “Creating Value Through External Intellectual Property Commercialization.” Journal of Technology Transfer 38 (6): 930–949.
Appendix
Table A1. Survey variables and construct items.
Table A2. Results of the multi-trait scaling.