Abstract
This paper continues efforts to establish a mutually informative dialogue between psychology and evolutionary robotics in order to investigate the dynamics of social interaction. We replicate a recent simulation model of a minimalist experiment in perceptual crossing and confirm the results with significantly simpler artificial agents. A series of psycho-physical tests of their behaviour informs a hypothetical circuit model of their internal operation. However, a detailed study of the actual internal dynamics reveals this circuit model to be unfounded, thereby offering a tale of caution for those hypothesising about sub-personal processes in terms of behavioural observations. In particular, it is shown that the behaviour of the agents largely emerges out of the interaction process itself rather than being an individual achievement alone. We also extend the original simulation model in two novel directions in order to test further the extent to which perceptual crossing between agents can self-organise in a robust manner. These modelling results suggest new hypotheses that can become the basis for further psychological experiments.
1. Introduction
A fundamental assumption of most cognitive science is that the individual agent is the correct unit of analysis for understanding mind, cognition and behaviour. However, the validity of this widespread ‘methodological individualism’ (cf. Boden Citation2006), i.e. an exclusive focus on individual agency whereby all social phenomena including social cognition is reducible without remainder to individual mechanisms, is starting to be questioned on the basis of research from a variety of disciplines. The idea that human cognition is at least partly constituted by social interactions has received support from cognitive anthropology (e.g. Hutchins Citation1995), developmental psychology (e.g. Tomasello Citation1999; Lindblom and Ziemke Citation2003), social studies (e.g. Pentland Citation2007), primatology (e.g. Tomasello Citation2000; Savage-Rumbaugh, Fields, Segerdahl and Rumbaugh Citation2005), interaction studies (e.g. Auvray, Lenay and Stewart Citation2009), the enactive approach to social cognition (De Jaegher and Di Paolo Citation2007; De Jaegher and Froese Citation2009), philosophical and phenomenological considerations (e.g. Zahavi Citation2001; Froese and Di Paolo Citation2009), as well as artificial life modelling (cf. Section 2). In terms of social cognition, this shift is expressed by positing embodied interaction as the primary mechanism of our understanding of other minds, rather than theoretical inference or empathic simulation (Gallagher Citation2001).
In response to this growing challenge of methodological individualism, Boden (Citation2006, p. 54) asks: ‘how can we drop it without descending into mysterianism about social forces, group minds, or personal interactions?’ She notes that the social behaviour of agents has become a practical issue in the field of artificial intelligence (AI), and cites Di Paolo's (2000) modelling work as an example of how methodological individualism may be rejected in a non-mysterious way. His models demonstrate how the unfolding of social behaviour can depend on the dynamical properties of the interaction process itself (i.e. entrainment does not happen with a non-responsive partner that performs pre-recorded actions). This general result has since been replicated by several other modelling studies, some of which are based on actual psychological experiments and have in turn cast new light on the interpretation of empirical data (e.g. Rohde Citation2008; cf. Section 2).
Here we continue this particular way of challenging methodological individualism by modelling a psychological study of minimalist perceptual crossing (Auvray et al. Citation2009), as well as some modified scenarios. The generated models support the non-individualistic explanation of the empirical results by means of dynamical analysis and through a novel behavioural task that shows that individuals cannot rely on individual cognition and yet manage to solve the task collectively. In both cases, these conclusions suggest novel empirical routes, demonstrating again the methodological advantage of minimal modelling, even for understanding and generating hypotheses for the case of human experiments.
2. Models of minimally social behaviour
Since its beginnings in the early 1990s, evolutionary robotics has established itself as a viable methodology for optimising dynamical controllers for physical robots (e.g. Nolfi and Floreano Citation2000), as well as for synthesising models of what has become known as ‘minimally cognitive behaviour’, namely the simplest behaviour that raises issues of genuine cognitive interest (cf. Beer Citation1996, Citation2003; Harvey, Di Paolo, Wood, Quinn and Tuci Citation2005). Within the context of this research framework there has also been a growing interest in using this synthetic method in order to investigate the interaction dynamics of social cognition (e.g. Di Paolo Citation2000; Quinn Citation2001; Iizuka and Ikegami Citation2004; Ikegami and Iizuka Citation2007; Iizuka and Di Paolo Citation2007; Williams, Beer and Gasser Citation2008; Di Paolo, Rohde and Iizuka Citation2008; Froese and Di Paolo Citation2008). As a specialisation of the evolutionary robotics methodology, we can conceptualise this kind of modelling research as investigations into the dynamics of ‘minimally social behaviour’.
What is especially interesting about some of these recent advances in evolutionary robotics is that the synthetic method has been used to create simulation models that are explicitly inspired by actual psychological experiments. Moreover, some of these models have been specifically designed to generate insights that have the potential to become the starting point for mutually informing collaborations between the field of artificial life and the traditional empirical sciences, especially social psychology (e.g. Ikegami and Iizuka Citation2007; Di Paolo et al. Citation2008; Rohde Citation2008). Here two popular experimental paradigms for such inter-disciplinary research will be reviewed briefly.
2.1. The double TV monitor paradigm
One promising study for such an endeavour is Murray and Trevarthen's Citation(1985) double TV monitor experiment. In this psychological study, 2-month-old infants were animated by their mothers to engage in coordination via a live double video link. However, when the live video of the mother was replaced with a video playback of her actions recorded previously, the infants became distressed or removed. These results, and those of a more rigorous follow-up study by Nadel, Carchon, Kervella, Marcelli and Réserbat-Plantey Citation(1999), indicate that 2-month-old infants are sensitive to ‘social contingency’, i.e. the mutual responsiveness during an ongoing interaction, and that this sensitivity plays a fundamental role in the unfolding of the ongoing coordination.
Traditional explanations of the infant's sensitivity to social contingency have focused largely on individual and internal cognitive abilities. For example, Gergely and Watson Citation(1999) have postulated the presence of an innate cognitive module that enables the detection of social contingency, and Russell Citation(1996) hypothesises that infants have an innate capacity to understand intentionality and to process agency. Are these postulations of innate cognitive capacities on the part of the individual infant actually necessary in order to explain the empirical results?
Iizuka and Di Paolo Citation(2007) used an evolutionary robotics approach to test whether simpler solutions for the detection of social contingency could also emerge from the dynamics of the interaction process itself. In their simulation model the evolved agents successfully acquired the capacity to discriminate between ‘live’ (two-way) and ‘recorded’ (one-way) interaction. Moreover, an analysis of the resulting dynamics suggests that this capacity cannot be reduced to the isolated individual agent, but that the interaction process itself plays an essential role in enabling this behaviour. These results have subsequently been generalised further by Froese and Di Paolo Citation(2008), and are also supported by other simulation studies of social interactions (e.g. Iizuka and Ikegami Citation2004; Ikegami and Iizuka Citation2007; Di Paolo et al. Citation2008).
In all these cases, it is either highly likely or actually demonstrated that individual interactors do not utilise any internal computational mechanisms, such as social contingency detection modules. Indeed, contrary to what would be expected by the framework of methodological individualism, their successful performance depends constitutively on dynamical properties of their mutual coupling, demonstrating that it is not necessary to postulate complex cognitive mechanisms to account for an agent's sensitivity to social contingency, and thus extending the spectrum of testable explanations that could be applied to the actual psychological cases.
2.2. Experiments in perceptual crossing
Another promising target for evolutionary robotics to show its relevance to issues in social psychology, and the one pursued in this paper, is Auvray et al.’s (2009) minimalist perceptual crossing experiment. The term ‘perceptual crossing’ denotes situations in which two perceptual activities of the same nature interact with each other (as in the case of mutual touch or catching one another's eye), and which thereby make it possible to recognise the presence of another intentional subject. More precisely, this study attempts to explore the most basic conditions necessary for participants to recognise each other through minimal technologically mediated interaction in a shared virtual space. As this study will be the target of the modelling experiments presented in this paper we shall describe it in a bit more detail here. A schematic of the overall experimental set-up, as originally described by Auvray and colleagues Citation(2009), is shown in .
Figure 1. The experimental set-up. Two participants face each other in a 600-unit-long 1-D environment that wraps around at the edges. Note that a participant's receptor field (white bar) can encounter three different objects (grey bars): a static object (located at 148 or 448, depending on the participant), the other participant's avatar (coinciding with the location of its receptor field) and the other's ‘shadow’ (attached to the other's avatar via a rigid link of 48 units). As all objects have the same width (4 units) they cannot be told apart by any inherent differences between their appearances (they all give rise to the same all-or-nothing tactile response whenever a participant's avatar makes contact with them).

Two adult participants, acting under the same conditions, can move a cursor left and right along a shared one-dimensional (1-D) virtual tape that wraps around. They are asked to indicate the presence of the other partner by clicking a mouse button. The participants are blindfolded and all they can sense are on/off tactile stimulations on a finger when their cursor crosses an entity on the tape. Apart from each other, participants can encounter a static object on the tape, or a displaced ‘shadow image’ of the partner, which is strictly identical to the partner as regards size and movement characteristics. Thus, each participant can encounter three different types of object in the shared environment:
(1) The four-unit wide ‘avatar’, i.e. sensory receptor field, of the other participant. When any of the four sensors of a participant overlaps with any sensors of the other participant, both of them receive sensory stimulation. This possibility of ‘perceptual interaction’, or shared perception, represents the way in which an embodied perceiver cannot observe someone else without also at the same time being perceivable in some manner, at least in principle (e.g. mutual gaze or touch). | |||||
(2) A four-unit wide static object that is placed at a specific location. There are two static objects, one for participant ‘up’ and one for participant ‘down’, which are located between 148–152 units and 448–452 units, respectively. Each participant can perceive only its specific static object. The objects were chosen to be participant-specific and placed maximally apart in the environment in order to encourage the participants’ exploration within the whole 1-D space. | |||||
(3) A four-unit wide ‘shadow’ object, whose position tracks that of the other participant at a fixed distance. The shadow object reproduces the exact same movement as the avatar of the other participant, but is displaced by 48 units. In contrast to an actual inter-individual encounter, it does not give rise to the possibility of two-way or mutual perceptual interaction. Thus, if a participant encounters the other's shadow object, it will indeed receive the same sensory stimulation as if it had encountered the other participant. However, as the other participant does not receive any corresponding sensory stimulation, there is only the possibility of ‘one-way’ interaction; thus, no reciprocal movement results from this encounter. |
The results of the psychological study show that, at least under the minimalist conditions of this experiment, the successful recognition of an ongoing interaction with another person is based not only on individual capacities, but also on certain properties that are intrinsic to the joint perceptual activity itself. The important issue is that the scanning of an encountered object will stabilise only when both partners are in perceptual contact with each other – if interaction is only one way, between a participant and the other's shadow, the shadow will eventually move away because the participant it is shadowing is still engaged in searching activity: two-way mutual scanning is the only globally stable condition. Therefore, in contrast to the expectations of a methodological individualist framework, the solution to the task does not rely on an individual ability to detect social contingency, but emerges from the mutual perceptual activity of the subjects that are oriented towards each other.
Di Paolo et al. Citation(2008) used evolutionary robotics to generate a simulation model of the perceptual crossing experiment, and successfully replicated the results while at the same time gaining some additional insights into the dynamics of the interaction process. For example, the problems that their agents had with avoiding interactions with their respective static objects led them to predict similar difficulties for human participants. This prediction was already supported by the empirical data presented in the work by Auvray and colleagues, but had previously gone unnoticed. Moreover, Di Paolo et al. found it practically impossible to evolve artificially a robust behavioural strategy without introducing temporal delays into the simulation, thereby leading to the additional hypothesis that timing has a crucial role between external stimulation in the participants’ behaviour.
In the cognitive sciences this work on perceptual crossing supports recent developments of the interactionist approach to social cognition (Gallagher Citation2008, pp. 162–166), as well as the enactive approach to cognitive science (De Jaegher and Froese Citation2009; Froese and Di Paolo Citation2009). Here we shall continue the modelling research in a novel direction. We begin by using a similar simulated experimental set-up as presented by Di Paolo and colleagues, and provide a comprehensive analysis of the evolved behavioural strategy by means of a set of psycho-physical tests. We then modify the original task in a couple of ways in order to test further the extent to which successful individual behaviour depends on the global dynamics of social interaction. This leads to new hypotheses that could be verified by additional psychological experiments. Finally, we use the psycho-physical studies of the evolved agents to derive a basic hypothesis about the sub-personal processes that give rise to their behaviour. However, a detailed analysis of the internal dynamics of the agents refutes this hypothesis in favour of one that instead focuses on temporality and the interaction process. Our inability to predict the internal operations of even such extremely simple dynamical systems serves as a warning against similar attempts to understand sub-personal mechanisms on the basis of behavioural observations in the case of humans.
3. Methods
This methodology section is divided into two parts. First, the simulated model environment and the model ‘agents’ are described (Section 3.1). Then, details are provided of the evolutionary algorithm that was used in order to optimise the behaviour of these agents for the perceptual crossing task (Section 3.2).
3.1. Simulation model
The simulation model includes two agents that, following Auvray et al. Citation(2009), face each other in a 1-D environment (in agent ‘up’ is located at 70 and agent ‘down’ is located at 340 units of space). The 1-D environment wraps around on itself after 600 units of space (i.e. the environment is a circle with a circumference of 600 units). In the simulation model all distance and time units are of an arbitrary scale. Each model agent can control the horizontal movement of its ‘avatar’, i.e. the position of its receptor field that occupies a total of 4 units of space. The sensory input of an agent is activated (set to unity) when its receptor field overlaps with another object in the 1-D space, otherwise the input remains off (set to zero).
The position and velocity of the agents are represented by continuous variables. The behaviour of each agent is determined by a continuous-time recurrent neural network (CTRNN), as described by Beer Citation(1995). Each CTRNN consists of fully interconnected nodes with self-connections. No symmetry is imposed on the network structure. The time evolution of node activation y
i
is determined by EquationEquation (1):
If node i is an ‘output’ node, then the velocity of the associated motor is that node's sigmoid output value z i (velocity range [0, 1]). No output gains were used. The overall speed of the agent is calculated as the difference between the left and right velocities. No noise was applied to any part of the simulation. The time evolution of the simulation environment and each agent's CTRNN controller is calculated by using Euler integration with a time step of 0.1. This time step has been chosen because the step size is still small enough such that, given the range of velocity and the size of the objects, all encounters within the 1-D space will give rise to at least some sensory stimulation, even if the agents move at maximum speed.
Finally, it should be emphasised that Di Paolo et al. Citation(2008) introduced a time delay between the activation of an agent's receptor field, i.e. due to an encounter in the 1-D environment, and the perturbation of the agent's CTRNN. This was apparently needed in order to evolve more robust and dynamic solutions to the task. As a CTRNN should in principle be capable of incorporating such a delay within its own network structure, we have spent a considerable amount of effort trying to evolve similarly robust and dynamic solutions without the external imposition of such a delay. However, this effort supported the findings of Di Paolo and colleagues that in practice such an approach does not seem to generate solutions that robustly generalise over all initial conditions. Accordingly, we introduced a delay of 25 units of time into the simulation, which finally made the evolution of more adaptive solutions possible. This delay is an integral part of the success of these solutions. Some preliminary tests with shorter delays showed that the agents described in Section 4 are capable of dealing with small alterations to some extent, but shortening the delay below 20 units makes them completely incapable of distinguishing between an encounter with a static object and the other agent. The practical need for appropriate delays in these models indicates that they might play a similar role in the adaptive performance of human participants, and whether this is indeed the case could be the focus of future psychological studies.
3.2. Evolutionary algorithm
The behaviour of the agents is optimised by using a simple genetic algorithm (GA) that is based on the ‘microbial GA’, a steady-state GA with tournament selection (cf. Harvey Citation2001). Until some termination criterion is reached, two members of the population are chosen at random, both have their fitness evaluated, and while the ‘winner’ of the tournament remains unchanged in the population, the ‘loser’ is replaced by a slightly mutated copy of the ‘winner’. Each member is a clonal pair of agents whose overall performance will be tested in a given experimental set-up. In this GA a generation is defined as the number of tournaments required to generate a number of offspring equal to the population size, which in this case has been set to 100. An evolutionary run finishes at a maximum of 5000 generations, though it is sometimes terminated manually before then if solutions are sufficiently good.
Niching
In order to enhance the evolvability of the CTRNNs, the standard microbial GA has been extended with a simple ‘geographical’ method to allow different subpopulations to evolve semi-independently within the overall population (cf. Spector and Klein Citation2006; Izquierdo, Harvey and Beer Citation2008). A minimalist, 1-D wrap-around ‘geography’ was used. This was implemented by running 10 evolutionary runs in parallel, each with a population size of 10 solutions. Thus, for a particular evolutionary run , after every generation two solutions are selected at random to compete against the best, most recent solutions of the two neighbouring subpopulations that are being evolved by runs
and
. The winner of each competition remains in the subpopulation of
for the next generation.
Genetic encoding
All CTRNN parameters and gains are encoded by a real-valued vector (gene range [0, 1]). At the start of the GA the gene vector is initialised with random values drawn from a uniform distribution (range [0, 1]). The mutation operator changes each gene by a random value drawn from a Gaussian distribution (μ=0; ) with reflection at the gene boundaries. Before every fitness evaluation, each gene is decoded linearly to the corresponding parameter range, except for the time constants, which are exponentially scaled.
Evaluation function
When the desirability of a solution is evaluated, it is tested for a total of 100 trial runs. This relatively large number of trials is beneficial for the evolutionary process because the behaviour of the CTRNN solutions is highly susceptible to initial conditions, i.e. the respective starting positions of the agents. Accordingly, the 100 trials are spread out evenly across the set of possible initial conditions: 10×10 trials over different possible starting positions, where the difference in positions is determined by a step size of
units of space. Moreover, to prevent the CTRNNs from simply learning how to deal with an arbitrary set of starting positions, for each evaluation the whole set of trials is adjusted by a general position offset drawn from a uniform random distribution (offset range [0, 60]), and each particular position is also displaced by a random value drawn from a Gaussian distribution (μ=0; σ2=30). Each trial run consists of 800 units of time. At the start of each trial, both agents have their internal node activations set to zero.
Fitness weighting
Even with the increased genetic diversity due to ‘geographical’ niching, and the random spread of the initial conditions, it is still the case that evolutionary runs are highly susceptible to getting stuck in local optima of the search space, especially because the difficulty associated with the starting positions is highly variable. For example, agents can easily locate each other when they begin a trial in each other's proximity, but fail when they start near their respective static objects. Under these conditions a typical evolutionary run will simply start optimising solutions for starting positions that are most easily optimised, because this will result in at least some improvement. However, eventually the solutions will become too specialised to be adapted to generalise over all starting positions. This problem was overcome by using a weighted measure of the overall success of a solution, whereby the contribution of a trial's score was inversely proportional to its ranking among all of the trials for that evaluation. This arrangement dynamically ensures that those starting positions that are difficult are given more weight.
4. Experiments
In this section, three sets of experiments are described. First, we replicated the results presented by Di Paolo et al. Citation(2008). In other words, the task performance of the simulated agents was evaluated in terms of the experimental set-up of the original psychological study (Section 4.1). We then introduce two novel studies that are specifically aimed at investigating the extent of the self-organising properties of the interaction process. In the first instance we tested the robustness of the solution that was evolved for the standard task by exchanging the input signals of the receptor fields between the two agents, as this significantly limits their ability for engaging in individual sensorimotor exploration (Section 4.2). Then we changed the experimental set-up to one in which the ‘intentions’ of the individual agents and the overall interaction dynamics were in conflict with each other, namely by evolving agents that were rewarded to interact with each other's shadow object (Section 4.3).
4.1. Experimental set-up 1
As a first step we tried to replicate the modelling work that has already been done in this direction by Di Paolo et al. Citation(2008). Their simulation model is relatively faithful to the details of Auvray et al.’s (2009) experimental set-up, with one significant difference: whereas the original task for the participants was to click a mouse whenever they encountered each other, the task for the model agents is to locate the partner agent and spend as much time as possible as close to each other as possible. Implicit in this task is the requirement for the agents not to become ‘trapped’ by the static object or shadow object of the other agent. Accordingly, in their model the fitness score F of each trial is calculated to be inversely proportional to the average distance between the two agents (it is therefore the same for both). The score F for a trial is determined by the following equation:
The advantage of this evaluation function is that it simplifies the task for the model agents, as they do not have to engage in any additional explicit classification response (i.e. some form of ‘clicking’). Moreover, because the score is a continuous measure this increases the evolvability of the solutions. In contrast to the discrete evaluation measure of a number of successful clicks, here every approaching behaviour is rewarded in a proportional manner, even if the agents do not happen to find each other (i.e. they do not actually need to cross).
There are drawbacks, however, in using the average distance between the agents as a measure of the desirability of the evolved solutions. Most importantly, this measure fails to distinguish properly between: an agent's general exploratory movements and interactions; and the explicit distinction of an ongoing interaction as an encounter with the receptor field of the other agent. By contrast, it is possible for a human participant in the original psychological experiments to spend a considerable amount of time engaging in interactions with the static and/or shadow object of the other, which is often the case, but then still decide against a mouse click response. Nevertheless, owing to the fact that this fitness measure turned out to be much more evolvable than equivalent measures based on ‘clicking’ accuracy, we chose to retain it for this study. Future work could investigate whether the inclusion of an explicit ‘clicking’ ability changes the general behavioural strategies of the agents.
We explored a range of CTRNN network sizes, starting with 11 nodes (the maximum size used by Di Paolo and colleagues), but were able to find robust solutions with as few as four nodes. We then chose the highest scoring solution out of the population that had achieved the highest average fitness out of the 10 different evolutionary runs for further testing. During testing the duration of each trial was doubled to 1600 units of time in order to assess better the general robustness of the selected solution.
In addition, we were able manually to prune several connections in the evolved CTRNN without significantly affecting its performance. Indeed, this pruning eventually allowed us to reduce the solution to a three-node CTRNN network, which is significantly smaller than the 11-node network obtained by Di Paolo and colleagues. This pruned CTRNN was then optimised further for 800 generations. The resulting CTRNN is shown in .
Figure 2. The CTRNN controller used for experimental set-up 1. Circles represent CTRNN nodes with time constants τ i , square-tailed arrows represent bias connections b i , diamond-tailed arrows represent weighted input connections Iw i , normal arrows represent motor outputs z i (L-M, left motor; R-M, right motor) and circle-headed lines represent weighted inter-node connections w ij (including self-connections). Negative connections are depicted as dashed lines, whereas positive connections are solid. The size of the arrows is roughly proportional to the weight strength of the connection, while the line width of the circles is roughly proportional to the decay speed of the node.
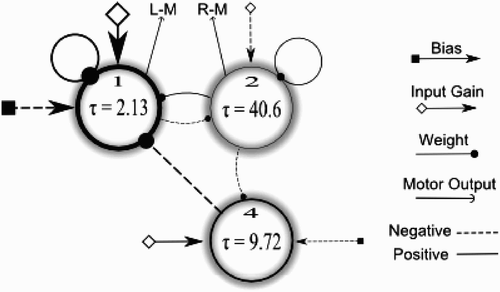
In order to get an initial rough idea of what the different fitness scores mean in terms of agent behaviour for this solution, it is helpful to consider the following illustrative cases.
(1) A fitness score of zero is an absolute limit point that is only ever attained when evolving solutions without delay, which often produces agents that suddenly stop moving when their receptor field becomes activated. Thus, when these agents start the trial on their respective static objects, in this case they will not move for the rest of the trial, and remain maximally distant from each other (the static objects are located 300 units apart). | |||||
(2) A fitness score of 0.5 is obtained, for example, when permanently turning the receptor field of the evolved agents, which then continually circle the 1-D environment until the end of the trial. The behaviour is also often displayed by randomly initialised CTRNNs that do not yet respond to perturbations. In these cases the agents move continuously in opposite directions around the 1-D environment, and therefore spend equal amounts of time near the maximally distant and maximally close positions. | |||||
(3) A fitness score of unity is another absolute limit point that is only ever attained by agents that have been evolved without delay, and which immediately stop moving when their receptor field is activated. Thus, when these agents start the trial on top of each other, they will not move for the rest of the trial, they remain maximally close to each other, and thereby receive perfect scores for this fluke. |
In order to obtain a comprehensive overview of this solution's performance, we tested it for each possible combination of starting positions of the two agents ( trials). The results of these tests are depicted graphically in .
Figure 3. Graphical representation of fitness scores achieved at each possible combination of starting positions for agent ‘up’ (x-axis) and agent ‘down’ (y-axis). Note that the axes wrap around owing to the 1-D circular shape of the virtual environment. Fitness scores range from 0.60 to 0.96 with an average of 0.87. See text for an explanation of the different regions.
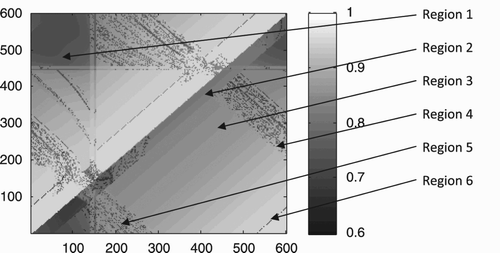
We can identify several salient regions in that enable us to make some general comments about the evolved solution. First, it should be noted how well the model agents manage to generalise over all initial conditions; no trial resulted in a fitness score of less than 0.60. Second, the evolved solution is not strictly symmetrical. However, this is not surprising because we did not enforce any structural symmetry on the CTRNN controller. Third, there are some regions of clear qualitative changes indicated by sharp grey-scale differences. These regions have been marked as 1–6 in for ease of reference. A brief description of the behaviour of the agents when initially starting from these different regions gives an overview of their general behavioural domain:
(1) The fitness in this region is relatively lower because both agents are perturbed by their respective static objects at the beginning of the trials. This will cause them briefly to oscillate around the object. After a few contacts the agents proceed to break out of the oscillation, continue to move on, and eventually come into contact. From this point onward they engage in perceptual crossing until the end of the trial. | |||||
(2) The low fitness diagonal region on the right-hand side going from starting position (0, 0) to (600, 600) can be explained as follows: agent ‘up’ (x-axis) moves rightwards without any input, while agent ‘down’ (y-axis) moves leftwards. This means that when agent ‘up’ starts within 52 units of space to the right of agent ‘down’, it will encounter the other agent's shadow object. This will trigger its receptor field and cause it to turn back briefly. However, agent ‘down’ has not been perturbed by this encounter and continues moving leftwards. Agent ‘up’ will thus not make any further contact and continue moving rightwards. In sum, what happens in this region is that agent ‘up’ is delayed, and the agents thus spend more time apart from each other. This ceases to be a problem when agent ‘up’ starts further along the x-axis compared with the starting position of agent ‘down’. | |||||
(3) This whole region is relatively flawless. The agents move unperturbed past each other's static object, eventually come into contact, and engage in perceptual crossing until the end of the trial. The increasing fitness gradient towards the corner (600, 0) reflects the fact that the agents have to travel less distance to meet each other. | |||||
(4) This streak of uneven fitness distribution reflects the fact that the agents, when they start from this region, sometimes encounter each other in the vicinity of the static object of agent ‘up’ (located at x=148). This interference causes the agents to break off perceptual crossing eventually, at least under some conditions. | |||||
(5) This streak of uneven fitness distribution reflects the fact that the agents, when they start from this region, sometimes encounter each other in the vicinity of the static object of agent ‘down’ (located at y=448). This interference causes the agents to break off perceptual crossing eventually, at least under some conditions. | |||||
(6) This solid line of relatively low fitness represents cases where the agents encounter each other at the beginning of the trial, but eventually disengage, move on, and finally establish proper perceptual crossing until the end of the run. These cases are the only trials in which the two agents break off perceptual crossing even though there is no external interference present. Such spontaneous disengagement did not occur with the original four-node solution; the three-node solution is thus slightly less robust. The behaviour appears to be related to the way in which the agents initially engage each other. In certain cases they are unable to stabilise the interaction appropriately. |
In order to get a better understanding of this discriminatory capacity it is helpful to study a particular interaction in more depth. We chose a representative trial starting from position (100, 500), which is illustrated in . The fitness score was 0.74. During this trial the agents first move sideways (in opposite directions, as they are controlled by identical CTRNNs but face each other), which is the most commonly evolved initial searching strategy. It is also the default behaviour for the agents whenever an interaction breaks down. When searching in this manner, the agents will not change their direction of movement until they make contact with another object in their environment. In this particular trial, they first encounter their respective static objects (before 1000 time steps), and continue to oscillate around this object for some time (from 1000 to 3000 steps). The agents then disengage and continue searching (from 3000 to 4500 steps), finally locate each other (after about 4500 steps), and manage successfully to establish perceptual crossing until the end of this trial (16,000 steps). On this basis it is possible to investigate why the agents disengage from the interactions with their static objects, but continue to engage in perceptual crossing once they make contact with each other.
Figure 4. Illustration of the behaviour of the agents during a representative trial starting from point (100, 500) with a score of 0.74. They first encounter their respective static objects, then continue searching, and finally locate each other and establish perceptual crossing until the end of the run (16,000 time steps). (a) The position of the agents and objects in the 1-D environment over time. (b) The sigmoid node outputs of agent ‘up’ over time. (c) The sensorimotor behaviour agent ‘up’ over time. Note that a change of receptor field status reaches the agent's controller only after a delay of 25 units of time (250 steps).
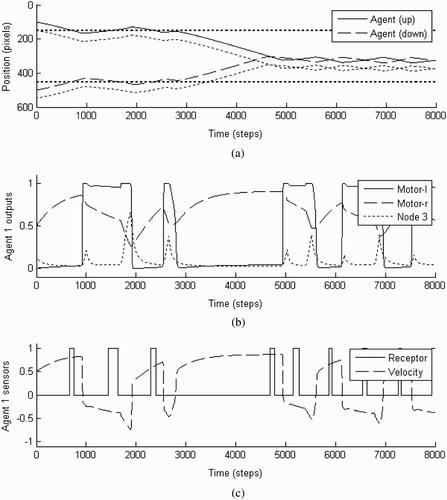
How does sensitivity to social contingency emerge in this system? The first thing to notice is that both agents disengage from their respective static objects after the third time that their receptor field has become activated. We shall therefore initially focus our analysis on this particular moment in time (the dynamical reason why discrimination happens only after three contacts will be given in Section 5, for now suffice to say that it has to do with the region of state space that the CTRNN is in). We know from Di Paolo and colleagues Citation(2008) that it is possible for the agents to base their behaviour on the duration of stimulation afforded by an encounter. Similarly, in this trial the third encounter between agent ‘up’ and its static object lasts 116 steps, while the third encounter with the other agent only lasts 56 steps. The static object therefore perturbs the agent almost exactly twice as long as the other agent. The reason for this, of course, is that the other agent moves with an opposite velocity, while the static object remains stationary. Do the agents make use of this difference in stimulus duration?
In order to determine whether this is indeed the case we performed some psycho-physical tests. By altering the size of the objects within the 1-D environment, it is possible to vary systematically the length of stimulation encountered by the agents. Similarly, we can also alter the size of the body of the agents. Thus, if the sensitivity of the agents relies on this temporal factor (duration of contact), then it should be possible to alter their behaviour accordingly. To explore this hypothesis two tests were conducted:
(1) We started the trial from the same initial conditions as before. However, just before the third interaction with the static object (at 2100 steps) we decreased the size of the static object to 3 units of space (from the usual 4 units). In this case the performance of the agents was drastically altered (score 0.06); both agents failed to disengage from their static objects and continued to oscillate around them until the end of the trial. As expected, the decrease in static object size entailed a shorter stimulation during the third contact (101 rather than 116 steps). | |||||
(2) We started the trial from the same initial conditions as the original set-up. However, just before the third perceptual crossing (at 5500 steps) we increased the size of the agents to 10 units of space (from the usual 4 units). In this case the performance of the agents dropped significantly (score 0.59). After making the third contact they drifted apart. As expected, the increase in agent size entailed a longer stimulation during the third encounter (108 rather than 56 steps). |
4.2. Experimental set-up 2
It could be argued on the basis of experimental set-up 1 that the agents actually employ an individual strategy to solve the task, namely by distinguishing between two different ranges of duration of contact. To be sure, part of the basis of this distinction, namely the duration of contact between agents, is co-determined by their different velocities. However, as long as both agents are simply moving at relatively different speeds, the possibility of making this distinction effectively remains the same, and no mutual interaction is necessary to establish it in the first place. On this view, the role of the interaction process would be marginalised to making interactions between an agent and the other's shadow unstable, thereby removing it as a possibility for further entrainment. The distinction between a static object and the other agent would then be largely a matter of individual accomplishment, as would be predicted by a methodological individualist stance. But is this all there is to the interaction process?
In order to test the hypothesis we modified the experimental set-up slightly, namely by switching the inputs to the receptor fields between the two agents. Under this set-up both agents still control the location of their respective receptor fields, but their CTRNN controllers receive the perturbations due to the other agent's field. In this manner we have severely disrupted the individual sensorimotor correlations, but crucially the possibility for engaging in mutual interactions leading to the establishment of perceptual crossing has remained unchanged from the original experiment. Consequently, if the agents in addition to their individual capacities also rely on mutually responsive interaction in order to distinguish between a static object and each other, they should still be able to perform the task even if they are incapable of using reliable sensorimotor correlations at the individual level. The results of a comprehensive test of this experimental set-up are shown in .
Figure 5. Graphical representation of fitness scores achieved at each possible combination of starting positions for agent ‘up’ (x-axis) and agent ‘down’ (y-axis). Note that the axes wrap around owing to the 1-D circular shape of the virtual environment. Fitness scores range from 0.40 to 0.965 with an average score of 0.87.
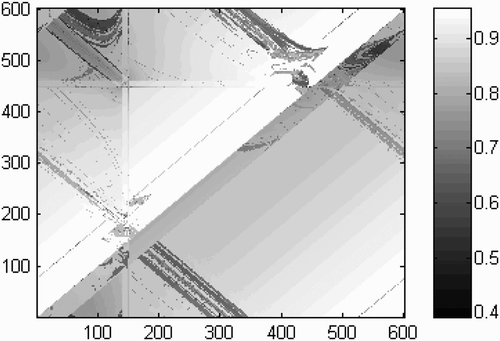
As predicted, the average fitness of this modified condition (0.87) is not different from that of the normal condition (0.87). Moreover, even the fitness distribution of the comprehensive results of this modified condition look strikingly similar to those of the normal condition shown in . To be sure, some of the salient patterns and asymmetries have shifted slightly, but these general differences might be expected, especially considering that we have effectively cross-wired the sensorimotor loops of the two agents. How are the agents able to cope with the distortion of their sensorimotor coupling? As a comparison it is helpful to examine again the conditions that led to the trial shown in , but with switched input parameters. A trace of the movement of the ‘input-switched’ agents is shown in .
Figure 6. Illustration of the behaviour of the agents during a representative trial starting from point (100, 500). The experimental set-up is the same as in , except that the input to the receptor fields of the agents has been exchanged between them. Fitness score: 0.71.
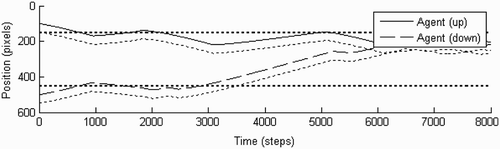
At the beginning of the trial the agents encounter similar situations, thereby providing each other with relatively matching simulation. At 3000 time steps, however, agent ‘down’ moves across its static object but, owing to the input switching, remains unperturbed, while agent ‘up’ is stimulated and turns back, does not find anything, and continues moving rightwards. Finally, the agents encounter each other and engage in perceptual crossing until the end of the trial. The switched inputs might thus even be helpful in some circumstances because most of the time the agents do not cross their respective static objects at the same time. Thus, when an agent turns back after being perturbed by the other agent who has just passed its own static object, it does not find anything there and is not held back any further. Similarly, the other agent will have remained oblivious to this occurrence as well. Moreover, the only time when there will be no interference from the swapped receptor fields at all is when the agents engage in mutual interaction, as this interaction results in identical (matching) receptor activations.
In sum, by modifying the original experimental set-up in the current manner we have thus demonstrated that the interaction process not only makes interaction with the shadow object unstable, thereby removing it as a possibility for further entrainment, but that it also plays a role in making perceptual crossing a stable possibility. Even without any consistent sensorimotor correlations as a basis for individual behaviour, the agents essentially negate this lack of individual control by means of mutually responsive interactions. In this manner successful perceptual crossing still self-organises out of the interaction process. On this basis we can hypothesise that if we changed the psychological study accordingly, human participants would similarly be able to continue to accomplish the task successfully (preliminary tests support this hypothesis).
4.3. Experimental set-up 3
It could be argued that the only reason why perceptual crossing emerges under the modified conditions of experimental set-up 2 is because the two agents actively establish the interaction process. After all, this is precisely what they were originally evolved for, and the success of their strategy might therefore still be better accounted for by appealing to their individual behavioural efforts rather than to the self-organising dynamics of the interaction process. How can we separate out the contribution of these two factors?
It is certainly the case that in the original experimental set-up of Auvray et al.’s psychological study, both the individual interactors and the interaction process are essentially ‘cooperating’ together in the successful completion of the task: Equation(1) if one individual finds the other's shadow, then the other will still be looking and the shadow will move away, thereby preventing further interactions; and Equation(2)
if one individual finds the receptor field of the other, the other has effectively found that individual too, thereby entailing further interactions. It follows that the stability of the interaction process in the experimental situation and the intentions of the individual interactors are reciprocally reinforcing. But just how important is the organisation of the interaction process for its own stability? Is its existence as a process largely supported by the behaviour of the individual agents or does it also possess some self-organising efficacy of its own?
Fortunately, it is also possible to investigate a ‘competitive’ situation in which the interaction process self-sustains even despite the individual intentions of the interactors. Consider, for example, the case of self-perpetuating arguments in which all participants actually want to stop arguing. Such a case could provide strong support for theories that propose that social interactions can be characterised by their autonomous dynamics (cf. De Jaegher and Di Paolo Citation2007). Note that the notion of a ‘competitive’ situation, as it is used here, refers to the competing goal-directedness of an individual interactor and the stability of the collective interaction process. Of course, this does not exclude the possibility that these interactors themselves also have conflicting intentions, but such inter-individual conflict is not necessary for an individual to be in conflict with the stability of the interaction process itself.
In order to investigate further the autonomous role of the interaction process within this particular experimental situation, we therefore need to change the basic set-up of Auvray and colleagues to give rise to this kind of ‘competitive’ situation. The task of detecting social contingency remains the same as before: the individuals must distinguish between those interactions that occur with the other's receptor field, and those that result from the mobile shadow object, as well as avoid any interaction with the static object. However, in contrast to the original psychological study, here the agents are required to stay with their partner's shadow object, rather than staying with the receptor field of their actual partner.
Owing to the asymmetry inherent in this set-up (i.e. agents face in opposite directions, but their shadows are displaced in the same direction), it is impossible for both participants to be interacting with each other's shadow at the same time. Therefore, in order to complete the task it is now necessary for the participants to avoid engaging in inter-individual interaction with each other. This will not be easy because: Equation(1) engaging in perceptual crossing is still a relatively stable behaviour, at least for as long as both interactors remain convinced that they are interacting with the other's shadow; and Equation(2)
crossing with the other's shadow remains inherently unstable, because that other participant will keep on looking for the shadow of its partner. In this manner we have created an experimental set-up in which the intentions of the individuals and the dynamics of the inter-individual interaction process are in direct conflict.
In order to implement this set-up in terms of a simulation model, we used the same parameters as for experimental set-up 1, but with the essential difference that the function to evaluate an agent's fitness (cf. EquationEquation (3)) now measures the average distance to the other agent's shadow. As the asymmetry of this set-up means that the evaluation function can now give different values for the two agents (i.e. they cannot be in contact with each other's shadow at the same time), we decided to have each agent controlled by a different CTRNN. In other words, whereas in the previous experiments we evaluated a solution by having two identical CTRNNs cooperating on the task, here we are evaluating two solutions by having them compete with each other during the trials.
It could be argued that this approach still does not represent proper competition between the solutions because of the likelihood of genetic convergence of the population (cf. Froese and Spier Citation2008). Genetic convergence could make the solutions almost identical, and therefore effectively turn this situation into a ‘cooperative’ one once again, at least at the genetic level. Nevertheless, this worry is unnecessary as several attempts to evolve agents to solve this task under these conditions did not succeed. The target solution appears to be too unstable to make such convergence possible, and even after thousands of generations the evolved behaviour is nowhere near as successful in terms of fitness as that evolved for experimental set-up 1. These difficulties indicate that there are limits to the ability of the interaction process to entrain the behaviour of the individual agents so as to sustain appropriate patterns of interaction.
In response to these difficulties of evolving a competitive scenario from scratch we chose a slightly more advantageous starting point for the evolutionary process, namely by seeding the populations with the best evolved agent from experimental set-up 1. In this case we still have two contrasting influences on the behaviour of the agents, i.e. a competitive fitness evaluation forcing the agents to disengage, and the entraining stability of the interaction process in the form of perceptual crossing. The results of comprehensive tests of the best agent taken from an evolutionary run that lasted almost 5000 generations are shown in . The results for the same agent, but with swapped receptor fields, are shown in . Note that for the purposes of these trials the agent competes against a clone of itself.
Figure 7. Graphical representation of fitness scores achieved at each possible combination of starting positions for agent ‘up’ (x-axis) and agent ‘down’ (y-axis). Note that the axes wrap around owing to the 1-D circular shape of the virtual environment. (a) Normal condition. Fitness scores range from 0.09 to 0.94 with an average of 0.80. (b) Swapped receptor fields. Fitness scores range from 0.06 to 0.94 with an average of 0.80.
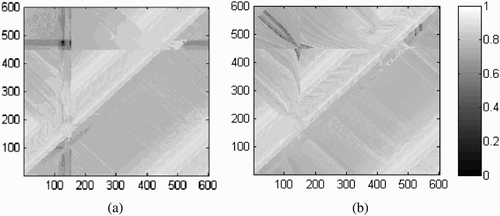
The comprehensive results shown in indicate that in terms of fitness scores the best evolved solution is in many respects qualitatively similar to the solutions found for the previous experiments, although there is a slight reduction in overall robustness. For example, there is a noteworthy exception that can be seen as a small black square in the top left corner of , an area where this solution received almost no score because both agents became stuck on their respective static objects. However, this particular problematic situation is largely resolved when we modify this set-up to the ‘swapped receptor field’ condition, as shown in . This result is consistent with what we have learned on the basis of experimental set-up 2. Of course, some points of low fitness remain, but this is to be expected considering the conflicting influences shaping agent behaviour.
How are these competing factors reflected in the behaviours and mutual interactions of the agents? We find that the original perceptual crossing evolved for experimental set-up 1 is retained across generations, although the precise strategy of the agents has adapted slightly to the new constraints. shows a sample trial run selected from .
Figure 8. Illustration of the behaviour of the agents during a representative trial starting from point (100, 500) with a score of 0.72. They first encounter their respective static objects, then continue searching, and finally locate each other and establish perceptual crossing until the end of the run (16,000 time steps). (a) The position of the agents and objects in the 1-D environment over time. (b) The node outputs of agent ‘up’ over time. (c) The status of the receptor field and the velocity of agent ‘up’ over time. Note that a change of receptor field status reaches the agent's controller only after a delay of 25 units of time (250 steps).
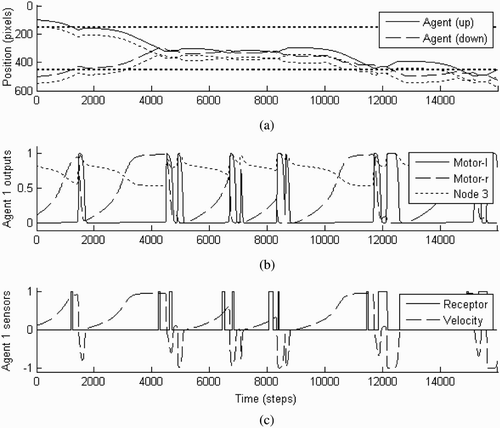
It is revealing to compare this trial with the one illustrated in . The scores of the two trials are almost identical, but there are some qualitative differences in behaviour. The first thing to notice is that in this modified set-up the agents make much less contact with the objects in their environment. For example, they make contact with their respective static objects only once (rather than three times) before moving on. Similarly, perceptual crossing is established with only two contacts (rather than periods of three). The second thing to notice is that the perceptual crossing is now characterised by a certain spatial drift, whereas before it was localised to one area of the environment. This is because of the asymmetry introduced by the competitive fitness function. For example, after engaging in basic perceptual crossing in between time steps 4000 and 8000, agent ‘down’ traces along the shadow object of agent ‘up’ until about 11,000 time steps. At this point the other agent, not having made any contact with agent ‘down’ for a while, starts to speed up its rightward exploratory movement, and the agents are eventually forced back into establishing perceptual crossing.
This inherent trade-off between staying in contact with the other's shadow and the self-organising stability of the interaction process is especially noticeable when agent ‘up’ starts the trial located between agent ‘down’ and its shadow (trial not depicted). In this case agent ‘up’ moves rightwards, makes contact with the other's shadow object, and traces its movement leftwards for a short period. However, as agent ‘down’ has not been perturbed during this activity it keeps increasing its leftward velocity until it eventually breaks away. In other words, lack of interaction makes this an unstable strategy and prevents the self-organising dynamics of the interaction process emerging. However, when the agents do make contact they generally engage in a pattern of interaction similar to that shown in , where short bursts of perceptual crossing are interspersed with periods of distancing. Here we thus have a situation in which the interaction process sustains itself, even though the individual agents have been specifically evolved to break this mutual interaction in favour of localising the other's shadow.
Of course, individual behaviour still plays an enabling role in this situation. It is the agents who make contact with each other, and thus allow the interaction process to take hold in the first place; and it is the agents who at times manage to break away from this entrainment as well. Nevertheless, it is the interaction process that constrains their behaviour most of the time, despite their individual goals. On this basis we can hypothesise that if we changed the psychological study accordingly, human participants would encounter great difficulty in accomplishing the task successfully. It is likely that they would still click more often on the other's receptor field than on the other's shadow. To some extent this hypothesis is already supported by the empirical data presented by Auvray and colleagues (Citation2009, p. 39), who found in their original psychological study that the probability of a participant's click after stimulation by either the other's active receptor field or the passive shadow object was not significantly different (as discussed in Section 2, the participants are still successful at solving the task collectively despite this ambiguity: mutually responsive interaction is the only globally stable condition, and thus participants find themselves quantitatively more often interacting with the other participant rather than the shadow object).
In sum, this modelling experiment is the first of its kind to show how individual behaviour can be coordinated by a self-sustaining inter-individual interaction process, despite the aims of those individuals to achieve the contrary. It is possible that this dynamical explanation for the presence of ‘unfit’ individual behaviour in this model can help to resolve outstanding puzzles in ethology. For example, it has been found that male fiddler crabs (Uca annulipes) engage in synchronous production of a conventional courtship signal, the movement of their single enlarged claw, even though there is no selective advantage in synchrony (Backwell, Jennions, Passmore and Christy Citation1998). It has been argued that the most plausible explanation is that synchrony is an ‘epiphenomenon’ of natural selection on male crabs who compete to be the first to signal, either because of some inherent physical constraint within the individuals or because of sexual selection (Reaney, Sims, Sims, Jennions and Backwell Citation2008). Here we thus have an example of methodological individualism in biology (i.e. why does an individual crab signal in synchrony with its neighbours?), which has created a blind spot for an explanation that takes into account the efficacy of the social domain. On the basis of the modelling experiment presented here we can hypothesise that synchronous signalling happens despite the differing goals of the individuals because of the coordinating organisation of an inter-individual interaction process.
5. Dynamical analysis
The results of these simulated experimental set-ups and the original empirical data presented by Auvray and colleagues undermine any attempt to attribute sensitivity to social contingency to the individual agents under these conditions. It is the collective interaction process, itself enabled by simple exploratory and discriminatory individual behaviour, which in turn enables and constrains appropriate ‘social’ behaviour on the individual level so as to self-sustain its entraining presence. We know, for example, that human participants of the study cannot tell from their perspective alone whether they are actually interacting with another responsive partner or merely with an unresponsive copy (no significant difference in clicking response to these two events). Yet their individual behaviour is clearly influenced by the presence of social contingency as such (mutual responsiveness enhances interactional stability). How can we explain this interaction between the individual and collective levels of dynamics?
In order to get a better understanding of how the individual and social levels relate to each other, let us for a moment entertain the validity of methodological individualism, i.e. we adopt the perspective that the individual agent is the only correct unit of analysis. The two behavioural tests described in Section 4.1 support the idea that an agent's discriminatory ability depends on the duration of the third contact. If this contact is too long it is a static object, if it is short enough then it is the other or the other's shadow. We have also seen that this ambiguity in the latter half of the discrimination is not a problem. Note, however, that already here we have to appeal to mutual responsiveness in order to discount the shadow as a serious possibility, as it affords only unstable interactions. Nevertheless, it still appears possible that the agent's controller is implementing a simple thresholding circuit that determines which of the two behaviours, i.e. oscillation or exploration, should become active depending on the length of stimulation. This hypothetical circuit is illustrated in .
Figure 9. Diagram of a hypothetical circuit that could explain the discriminatory behaviour of the agents. D1 and D2 are delays (total delay: 25 units of time), ∫ I dt is the integral of the input signal I for K units of time, T1 and T2 are thresholds, and G1 and G2 are output gains.
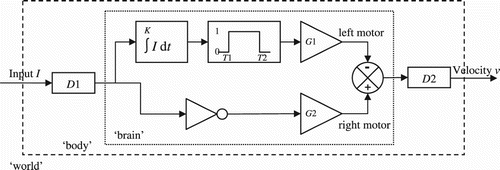
We know from the implementation of the agents that their controller is separated from their environment by a delay, which can be represented as part of their body. We also know that the velocity v of the agents is the difference between left and right motor activation. In addition, we have established that the crucial factor is duration of stimulation. Accordingly, we posit an integrator element, which takes the delayed input signal I and outputs the integral, calculated over K units of time. This integral is then passed through a threshold unit which becomes active (i.e. it outputs 1, rather than 0) when the integral falls between two thresholds T1 and T2. The initial behavioural tests described in Section 4.1 allow us to set T2 at about 105 time steps. The output of the threshold unit is then multiplied by the left motor gain G1 and subtracted from the agent's overall velocity. At the same time we know that when the agent receives no input (I=0) it continues to move rightwards. Accordingly, we posit another connection from the delayed input signal that passes through an inverter, is multiplied by the right motor gain G2, and is added to the agent's overall velocity.
Is it possible to determine the precise values for these parameters, especially T1 and T2? Let us consider a trial from the experimental set-up 1 in which the agents did not do very well in order to get an idea of the range of these values. We can hypothesise, for example, that the low fitness found in region 6 of can be explained in terms of a long contact time between the agents. To test this hypothesis we chose a representative trial starting from (557, 32). In this case the agents spontaneously disengage their interaction (after 2000 time steps) after having found each other at the very beginning of the trial. They then continue to explore the environment, finally re-establish perceptual crossing (after 10,000 time steps), and continue to interact appropriately until the end of the trial.
This initial coordination failure highlights the importance of co-regulation for perceptual crossing to be established. It is not enough for the agents simply to encounter each other, they also have to encounter each other with the right kind of velocity. It is also interesting to note that the decisive contact was the fourth encounter in this case rather than the usual third. Might this have something to do with the cause of the spontaneous failure? Initially this idea appears to be rejected by a quick test of decreasing the size of both agents to 3 units of space (instead of the normal 4 units) just before the fourth encounter, which results in a performance with an almost maximum score. It is a matter of stimulation length after all, but the number of contacts appears to be less important. Moreover, this modified contact lasts 55 steps, which is short even for the duration of normal contact with another agent. Might this be an indication for the value of T1? However, there is a serious problem for our hypothesis: the original final encounter between the two agents lasted only 64 steps as well. This value is still within the range of contact durations with the other agent that we determined in the previous series of tests (between T1 and T2). So why do the agents disengage? At this point we have to reject the possibility that we can explain the agent's sensitivity to social contingency in terms of an objective cut-off point of contact duration, and turn to a more dynamical explanation.
As a first step in this direction, it is important to get an idea of the general shape of the dynamical landscape of the CTRNN shown in . When the CTRNN is decoupled from the 1-D environment it is characterised by two fixed point attractors, depending on whether the input parameter I is set to zero or unity (see ). It turns out that the velocity of the agents is strongly coupled to the value of this parameter. The velocity of the agent at attractor0, when input I=0, is 0.867 units of space per unit of time, whereas for the attractor1, when I=1, the velocity is −0.963. This is indeed the basis for a tight sensorimotor coupling: the value of the input parameter is largely determined by the movement of the agent, and the movement of the agent is largely determined by the input parameter (for a similar result on the basis of a related task, see Froese and Di Paolo Citation2008).
Figure 10. The attractor landscape for the CTRNN shown in . The node activations were initialised 50 times to random activation values drawn from the trial shown in , and the network was allowed to settle for 8000 time steps. The axes (motor-l, motor-r, node 3) represent the sigmoid output of the three CTRNN nodes. The input was either (a) set to zero, which revealed a fixed point attractor at (0.04, 0.91, 0.02), or (b) set to unity, which revealed a fixed point attractor at (0.98, 0.02, 0.88). The attractors are represented by an asterisk. Input-driven switching between the two attractors results in a hysteresis of motor outputs.
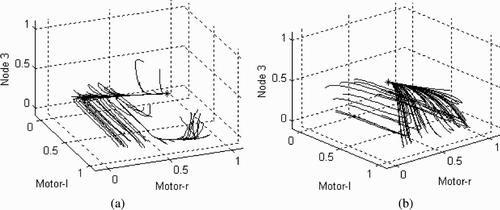
It is also noteworthy that the switching behaviour between the two attractors is characterised by a form of hysteresis (see ). The only way for the system to settle at attractor0 is for the output of the three nodes (motor-l, motor-r, node 3) to approach it from roughly (0, 0, 0) to (0, 1, 0). Thus, if I=0 and the system happens to be at (1, 1, 0), then it first needs to pass through (0, 1, 0) and (0, 0, 0) before finally reaching (0, 1, 0). Similarly, for the system to settle at attractor1 it must approach it from roughly (1, 1, 0) to (1, 0, 1). If I=1 and the system currently happens to be at attractor0 (0, 1, 0), then it first switches to (1, 1, 0) before shifting toward attractor1 (1, 0, 1).
Figure 11. Change of state between the left and right motors during the trial that was shown in . Arrows indicate direction and relative velocity of trajectories (i.e. long arrows, fast trajectories; short arrows, slow trajectories). For an explanation of regions 1–2 and 2–3 on the left-hand side of the state space, see the text.
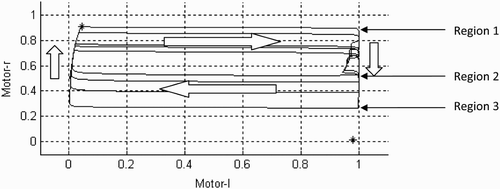
It is also important to note that there are significant differences in the trajectory speeds of the different regions of activation space, something that cannot be seen in (but cf. ). In the case of attractor0 the trajectories going from (1, 1, 0) to (0, 1, 0) are initially relatively slow; but when the output of the right motor node drops into the region of somewhere below 0.5, the system almost instantaneously switches off the output of the left motor node. Then the trajectories continue to make their way slowly from somewhere near (0, 0, 0) to the attractor0 at (0, 1, 0). In the case of attractor1, if the system happens to be near attractor0 at (0, 1, 0), then the system almost immediately switches on the left motor by going into state (1, 1, 0), and only then slowly begins to turn off the right motor as it approaches attractor1 at (1, 0, 1). In other words, the left motor can be switched on fully immediately from all states whenever I is set to unity, but it only switches off immediately when I is reset to zero from certain regions of state space, otherwise it lingers in the fully on state for a while.
This effectively means that the time taken to switch between output velocities not only depends on the current state of the input parameter, but is also determined by the current state of the system. In other words, the behaviour of the agents is not purely reactive to the input parameter but, owing to the hysteresis of the motor outputs with different trajectory speeds, depends crucially on their history of interactions. The hysteresis relationship between the different regions of state space effectively acts as a kind of short-term memory. The influence of this kind of dynamical internal state is completely missed by the hypothetical cybernetic circuit diagram that was shown in .
Moreover, this historical dimension in terms of the influence of previous interactions brings us to the next step of our dynamical analysis, as up to now we have only considered the CTRNN in isolation. How does the system behave when it is coupled to the 1-D environment and interacts with other objects, including the other agent? The change in state of the system during the first 8000 time steps of the trial starting from position (100, 500), as shown in , is of interest here. How does the dynamical analysis help us to understand better what is going on? Let us consider more closely the hysteresis relationship between the left and right motors (motor-l and motor-r) during this trial, as shown in . What make this hysteresis relationship special are the different velocities of the trajectory depending on which regions of state space the system is in. Observations of these trajectories have indicated some regions (all reached when motor-l ≈1 due to an input stimulation) to be of particular importance in explaining the discriminatory behaviour (cf. ). Between regions 1 and 2 the decrease in motor-r output is relatively slow, thereby delaying the moment when motor-l almost immediately decays (between regions 2 and 3). It is also important to note that the duration of stimulation (I=1) is proportional to the amount of inhibition of right motor output (the further down from region 1 the system state ends up).
How does this relate to the agent's observed behaviour? Before engaging in an interaction in the environment, the system is near attractor0 (top left) and thus with the output of the right motor on full power (motor-r ≈1). When the input parameter I changes from zero to unity the system immediately switches the left motor on to full power as well (motor-l ≈1) by jumping to region 1, thereby changing the direction of travel from rightward to leftward. This leftward velocity briefly increases, as the system starts to turn slowly down the right motor by passing down towards region 2. However, during this period both motors remain in competition and the agent's velocity is therefore slower on the leftward journey back to the source of the initial stimulation. This typically results in another, more extended contact with the object, which reduces further the output of the right motor by pushing the system's state further down towards region 3. From this region the output of the left motor node decays almost immediately by shifting the system's state left towards . This returns the agent again to its initial rightwards velocity, which is initially slow but continually increases as the system's state moves back upward to attractor0. A third contact with the other object ensues, switching the left motor back on to full power, but without the right motor output having fully recovered. This means that the system lands closer to region 2–3 than before. If it happens to be close enough, i.e. previous contacts sufficiently inhibited the right motor, then the left motor switches off almost immediately, and the agent returns to its rightward search without making another contact with the object (it disengages from the interaction). Otherwise, its receptor field catches the other object again and another hysteresis cycle is initiated (the interaction is sustained for another round).
This analysis shows that the three contacts that are made before the agent moves away (no social contingency) or engages in another round of oscillation (social contingency) do not result from some internal counting mechanism. Instead, the system is making use of these interactions to push itself into a different region of state space, from which discriminatory behaviour becomes possible. The third contact happens after the agent has made initial contact, turned around for another contact, and is now on its way to return to its original rightward velocity. In other words, the system is still tracing its way back up towards attractor0 when it is perturbed again. The duration of stimulation during these encounters determines how far down in the state space between regions 1 and 2 the system will go. The more stimulation, the further it shifts towards region 2–3, and the more quickly the activation of the left motor node will decay, rapidly shifting the state back leftwards. In this case the system will quickly resume its rightward velocity. In other words, a lengthy stimulation results in a significantly quicker return to rightward velocity, which prevents another contact with the object occurring and the agent therefore moves away. Otherwise, if the third simulation is short enough, for example because of the responsiveness of the other agent, the system will spend some time slowly moving down region 1–2, before eventually reaching region 2–3 and then switching quickly as before. This difference in time needed for the left motor to become deactivated, which is influenced by the responsive counter-movement of the other agent, is essentially at the basis of the agent's ability to distinguish between its static object and that other agent.
In summary, contrary to what would be expected from the perspective of methodological individualism, we found that the sensitivity to social contingency exhibited by the agents emerges only during interaction. The processes that drive the necessary internal state changes via appropriate input-switching are not internal to the agent, and in this case they are even partly constituted by the responsive behaviour of the other agent. An agent in an empty 1-D environment would be forever doomed to linear movement, lacking the ability to switch internally between the two attractor landscapes. Moreover, the hypothetical circuit of the agent's internal operations turned out to be wholly inadequate. A more detailed dynamical analysis failed to find internal thresholding mechanisms, but instead revealed the important role of different temporal scales distributed across the state space, as well as the constitutive role played by the agent's interactions with other objects (responsive and irresponsive). Note also that the behaviour of the agents was, contrary to common expectations, determined largely by dynamical transients rather than fixed attractors. Environmental interactions did not perturb the system along trajectories on a fixed state space, but instead caused the entire state space itself to switch between different attractor landscapes along which the system moved.
6. Discussion
The modelling experiments presented in this paper have provided support for the idea that the organisation of an inter-individual interaction process can enable and constrain individual behaviour in ways that are beneficial (and detrimental) for problem-solving. In experimental set-up 1 (cf. Section 4.1) we replicated the findings of previous studies (Di Paolo et al. Citation2008; Auvray et al. Citation2009), namely that interaction dynamics can enable the agents to complete a task that appears impossible from an individual's perspective. The emergence of ongoing perceptual crossing between two interacting agents gives rise to a form of coordination that depends on the ongoing interaction, and at the same time also makes it more likely for that kind of mutual interaction to persist. This reciprocal dependency between individual agent behaviour and overall coordination dynamics defines what has been called the constitutive autonomy of the interaction process (cf. De Jaegher and Di Paolo Citation2007).
The fact that the results of Auvray and colleagues can be replicated in a simulation model involving relatively simple interacting dynamical systems indicates that such autonomous interaction processes (and their enabling/constraining effects) might be much more pervasive than at first assumed. Thus, whereas De Jaegher and Di Paolo Citation(2007) illustrate their enactive approach to social cognition with examples drawn from human interactions, these models make a plausible case that more basic forms of life can also give rise to autonomous interaction processes. As such, it can be argued that the capacity of the interaction process to expand an agent's repertoire of behaviour can play an important explanatory role in defending the life-mind continuity thesis (cf. De Jaegher and Froese Citation2009). Indeed, it turns out that it is not even necessary for an agent involved in such an interaction to be aware that it is interacting with another agent. This finding has implications for our understanding of the relationship between interaction and intersubjectivity, as well as the relationship between a systemic approach and Husserlian phenomenology more generally (Froese and Di Paolo Citation2009).
In experimental set-up 2 (Section 4.2), we disrupted the sensorimotor loops of the modelled agents in such a way that they could no longer properly regulate their individual behaviours. However, engaging in reciprocal perceptual crossing remained a possibility within this modified set-up. The results show that the agents managed to complete the task in spite of this sensorimotor disruption. It turns out that their remaining interactional capacity was sufficient for the emergence of the relevant coordination dynamics, which then effectively organised the impaired individual behaviours appropriately. This is an indication that, given the right circumstances, interaction processes can enable and organise the individual capacities of the interactors in a beneficial manner without the need for external control.
Finally, in experimental set-up 3 (Sections 4.3) we modified the task of the agents in such a way that their individual behaviours and the overall interaction dynamics were in conflict, namely by requiring the agents to interact with the other's shadow (an inherently unstable situation in this set-up). The results for this experiment support De Jaegher and Di Paolo's (2007) claim that, under some circumstances, an interaction process can constrain the behaviours of the interacting agents such that it continues to persist despite the efforts of the individual interactors. In the simulation case, the agents ‘struggle’ to stay close to the other's shadow object, and nevertheless continually fall back into the more stable pattern of mutual perceptual crossing. This experiment indicates that we should be careful when assessing responsibility for an individual's behaviour. The outcome of intended actions can be unconsciously constrained in undesirable directions by certain processes in the social domain, thus leading to conflict despite our intention to behave otherwise.
Finally, a lesson to be learnt from the dynamical analysis presented in Section 5 is that we should be wary of using hypothetical circuits to explain observed behaviour. As Hurley wrote in relation to her own ‘shared circuits model’ (SCM) of cognition: ‘While SCM is described cybernetically, dynamic systems theory could represent interactions of its implementing neural processes and embodied activity over time as evolution of a phase space, and investigate its attractor structure’ (Hurley Citation2008, p. 20). Thus, at best, such models might help us to make the system's operations more intelligible, but there is the danger that we are merely providing a symbolic re-description of the behaviour that emerges from the system's interactions with its environment. It would be a mistake to reify such re-description as internal entities existing at the sub-personal level. In this simple example, for instance, we determined that the system was sensitive to the duration of a stimulus, and on this basis posited a comparator module as part of its operations, and finally had to concede that no such mechanism, as a reified unit of operation, exists within the actual system. Instead, the observed sensitivity is an emergent outcome of the shape of two different state spaces and the way interactions switch the system between them. It is the interaction process itself that shapes the agents’ response so as to sustain this interaction.
7. Conclusions
We have replicated a recent simulation model of a minimalist experiment in perceptual crossing and confirmed the results with significantly simpler artificial agents. A series of psycho-physical tests of their behaviour informed the creation of a hypothetical circuit model of their internal operation. However, a detailed study of the actual internal dynamics reveals this circuit model to be unfounded, thereby offering a tale of caution for those hypothesising about sub-personal processes in terms of behavioural observations. In particular, it has been shown that the behaviour of the agents emerges largely out of the interaction process itself rather than being an individual achievement alone. We have also extended the original simulation model in two novel directions in order to test further the extent to which perceptual crossing between agents can self-organise in a robust manner. These modelling results are the basis for new hypotheses that can be tested by further psychological studies. This paper thereby continues the ongoing efforts to establish a mutually informative dialogue between psychology and evolutionary robotics, especially in order to investigate the dynamics of social interaction.
The simulation experiments presented in this paper lend support to the enactive approach to social cognition (De Jaegher and Di Paolo Citation2007; De Jaegher and Froese Citation2009; Froese and Di Paolo Citation2009). The results show that, under some circumstances, an interaction process can take on a self-organising identity of its own, and that such a process can effectively enable and constrain the behavioural repertoire of the individuals involved in the interaction. More precisely, the experiments indicate: Equation(1) that some interaction processes can beneficially extend the cognitive domain of individuals in novel directions without requiring any form of external supervision; and conversely Equation(2)
that some interaction processes can organise behavioural repertoires in ways that are in conflict with an individual's intended action. More research is needed in order to understand better the circumstances that lead to one or the other situation. Future work might eventually help us to structure our social environment better such that beneficial situations are more likely to emerge spontaneously.
Acknowledgements
We would like to thank Eduardo Izquierdo, Marieke Rohde and Peter Fine for the many helpful comments and discussions.
References
- Auvray , M. , Lenay , C. and Stewart , J. 2009 . Perceptual Interactions in a Minimalist Virtual Environment . New Ideas in Psychology , 27 : 32 – 47 .
- Backwell , P. , Jennions , M. , Passmore , N. and Christy , J. 1998 . Synchronized Courtship in Fiddler Crabs . Nature , 391 : 31 – 32 .
- Beer , R. D. 1995 . On the Dynamics of Small Continuous-time Recurrent Neural Networks . Adaptive Behavior , 3 : 471 – 511 .
- Beer , R. D. Toward the Evolution of Dynamical Neural Networks for Minimally Cognitive Behavior . From Animals to Animats 4: Proceedings of the 4th International Conference on Simulation of Adaptive Behavior . Edited by: Maes , P. , Mataric , M. J. , Meyer , J.-A. , Pollack , J. and Wilson , S. W. pp. 421 – 429 . Cambridge , MA : The MIT Press .
- Beer , R. D. 2003 . The Dynamics of Active Categorical Perception in an Evolved Model Agent . Adaptive Behavior , 11 : 209 – 243 .
- Boden , M. A. 2006 . Of Islands and Interactions . Journal of Consciousness Studies , 13 : 53 – 63 .
- De Jaegher , H. and Di Paolo , E. A. 2007 . Participatory Sense-making: An Enactive Approach to Social Cognition . Phenomenology and the Cognitive Sciences , 6 : 485 – 507 .
- De Jaegher , H. and Froese , T. 2009 . On the Role of Social Interaction in Individual Agency . Adaptive Behavior , 17 : 444 – 460 .
- Di Paolo , E. A. 2000 . Behavioral Coordination, Structural Congruence and Entrainment in a Simulation of Acoustically Coupled Agents . Adaptive Behavior , 8 : 25 – 46 .
- Di Paolo , E. A. , Rohde , M. and Iizuka , H. 2008 . Sensitivity to Social Contingency or Stability of Interaction? Modelling the Dynamics of Perceptual Crossing . New Ideas in Psychology , 26 : 278 – 294 .
- Froese , T. and Di Paolo , E. A. Stability of Coordination Requires Mutuality of Interaction in a Model of Embodied Agents . From Animals to Animats 10: Proceedings of the 10th International Conference on Simulation of Adaptive Behavior . Edited by: Asada , M. , Hallam , J. C.T. , Meyer , J. A. and Tani , J. pp. 52 – 61 . Berlin : Springer .
- Froese , T. and Di Paolo , E. A. 2009 . Sociality and the Life–Mind Continuity Thesis . Phenomenology and the Cognitive Sciences , 8 : 439 – 463 .
- Froese , T. and Spier , E. 2008 . Convergence and Crossover: The Permutation Problem Revisited . Cognitive Science Research Paper 596, University of Sussex
- Gallagher , S. 2001 . The Practice of Mind: Theory, Simulation or Primary Interaction? . Journal of Consciousness Studies , 8 : 83 – 108 .
- Gallagher , S. 2008 . Brainstorming: View and Interviews on the Mind , Exeter : Imprint Academic .
- Gergely , G. and Watson , J. 1999 . “ Early Social–Emotional Development: Contingency Perception and the Social Biofeedback Model ” . In Early Social Cognition: Understanding Others in the First Months of Life , Edited by: Rochat , P. 101 – 137 . Hillsdale , NJ : Lawrence Erlbaum .
- Harvey , I. Artificial Evolution: A Continuing SAGA . Evolutionary Robotics. From Intelligent Robotics to Artificial Life: Proceedings of the 8th International Symposium on Evolutionary Robotics . Edited by: Gomi , T. pp. 94 – 109 . Berlin : Springer .
- Harvey , I. , Di Paolo , E. A. , Wood , R. , Quinn , M. and Tuci , E. A. 2005 . Evolutionary Robotics: A New Scientific Tool for Studying Cognition . Artificial Life , 11 : 79 – 98 .
- Hurley , S. 2008 . The Shared Circuits Model (SCM): How Control, Mirroring, and Simulation can Enable Imitation, Deliberation, and Mindreading . Behavioral and Brain Sciences , 31 : 1 – 22 .
- Hutchins , E. 1995 . Cognition in the Wild , Cambridge , MA : The MIT Press .
- Iizuka , H. and Di Paolo , E. A. Minimal Agency Detection of Embodied Agents . Advances in Artificial Life: Proceedings of the 9th European Conference on Artificial Life . Edited by: Almeida e Costa , F. , Rocha , L. M. , Costa , E. , Harvey , I. and Coutinho , A. pp. 485 – 494 . Berlin : Springer .
- Iizuka , H. and Ikegami , T. 2004 . Adaptability and Diversity in Simulated Turn-taking Behavior . Artificial Life , 10 : 361 – 378 .
- Ikegami , T. and Iizuka , H. 2007 . Turn-taking Interaction as a Cooperative and Co-creative Process . Infant Behavior & Development , 30 : 278 – 288 .
- Izquierdo , E. , Harvey , I. and Beer , R. 2008 . Associative Learning on a Continuum in Evolved Dynamical Neural Networks . Adaptive Behavior , 16 : 361 – 384 .
- Lindblom , J. and Ziemke , T. 2003 . Social Situatedness of Natural and Artificial Intelligence: Vygotsky and Beyond . Adaptive Behavior , 11 : 79 – 96 .
- Murray , L. and Trevarthen , C. 1985 . “ Emotional Regulations of Interactions Between Two-month-olds and their Mothers ” . In Social Perception in Infants , Edited by: Field , T. M. and Fox , N. A. 177 – 197 . Norwood , NJ : Ablex Publishing .
- Nadel , J. , Carchon , I. , Kervella , C. , Marcelli , D. and Réserbat-Plantey , D. 1999 . Expectancies for Social Contingency in 2-month-olds . Developmental Science , 2 : 164 – 173 .
- Nolfi , S. and Floreano , D. 2000 . Evolutionary Robotics: The Biology, Intelligence, and Technology of Self-organizing Machines , Cambridge , MA : The MIT Press .
- Pentland , A. 2007 . On the Collective Nature of Human Intelligence . Adaptive Behavior , 15 : 189 – 198 .
- Quinn , M. Evolving Communication without Dedicated Communication Channels . Advances in Artificial Life: Proceedings of the 6th European Conference on Artificial Life . Edited by: Kelemen , J. and Sosik , P. pp. 357 – 366 . Berlin : Springer .
- Reaney , L. T. , Sims , R. A. , Sims , S. W.M. , Jennions , M. D. and Backwell , P. R.Y. 2008 . Experiments with Robots Explain Synchronized Courtship in Fiddler Crabs . Current Biology , 18 : 62 – 63 .
- Rohde , M. 2008 . Evolutionary Robotics Simulation Models in the Study of Human Behaviour and Cognition . unpublished D.Phil. thesis, University of Sussex
- Russel , J. 1996 . Agency: Its Role in Mental Development , Hove : Taylor & Francis .
- Savage-Rumbaugh , S. , Fields , W. M. , Segerdahl , P. and Rumbaugh , D. M. 2005 . Culture Prefigures Cognition in Pan/Homo Bonobos . Theoria: An International Journal for Theory, History and Foundations of Science , 20 : 311 – 328 .
- Spector , L. and Klein , J. 2006 . “ Trivial Geography in Genetic Programming ” . In Genetic Programming Theory and Practice III , Edited by: Yu , T. , Riolo , R. and Worzel , B. 109 – 124 . New York : Springer .
- Tomasello , M. 1999 . The Cultural Origins of Human Cognition , Cambridge , MA : Harvard University Press .
- Tomasello , M. 2000 . Primate Cognition: Introduction to the Issue . Cognitive Science , 24 : 351 – 361 .
- Williams , P. , Beer , R. and Gasser , M. Evolving Referential Communication in Embodied Dynamical Agents . Artificial Life XI: Proceedings of the 11th International Conference on the Simulation and Synthesis of Living Systems . Edited by: Bullock , S. , Noble , J. , Watson , R. and Bedau , M. pp. 702 – 709 . Cambridge , MA : The MIT Press .
- Zahavi , D. 2001 . Beyond Empathy: Phenomenological Approaches to Intersubjectivity . Journal of Consciousness Studies , 8 : 151 – 167 .