Abstract
A humanoid service robot equipped with a set of simple action skills including navigating, grasping, recognising objects or people, among others, is considered in this paper. By using those skills the robot should complete a voice command expressed in natural language encoding a complex task (defined as the concatenation of a number of those basic skills). As a main feature, no traditional planner has been used to decide skills to be activated, as well as in which sequence. Instead, the SOAR cognitive architecture acts as the reasoner by selecting which action the robot should complete, addressing it towards the goal. Our proposal allows to include new goals for the robot just by adding new skills (without the need to encode new plans). The proposed architecture has been tested on a human-sized humanoid robot, REEM, acting as a general purpose service robot.
1. Introduction
Service robotics is an emerging research area for human-centred technologies. Even though several specific applications exist for this kind of robots, a general purpose service robot control is still missing, especially in the field of humanoid service robots (Haidegger et al., Citation2013). Hence, the main aim in this work is to endow service robots with a control architecture allowing them to generate and execute their own plan to accomplish a goal. The goal should be decomposable into several steps, each step involving a skill implemented in the robot. Furthermore, the system should openly be increased in goals by just adding new skills, without encoding new plans.
Typical approaches to the general control of service robots are mainly based on state machine technology, so all the steps required to accomplish the goal are specified and known by the robot beforehand. In those controllers, the list of possible actions that the robot can complete is exhaustively created, as well as all the steps required to reach the goal. The main drawback for this approach is that too much information must be specified beforehand, preventing the robot to react to either novel situations or new goals. A first alternative to the use of state machines are planners (Russell & Norvig, Citation2003). Planners, usually based on probabilistic approaches/criteria, determine at running time which is the best sequence of skills to be used in order to meet the specified goal. A different approach to planners is the use of cognitive architectures. Those are control systems trying to mimic some brain processes in order to generate decisions (Chen et al., Citation2010; Jones, Citation2004; Kelley, Citation2006; Laird & Wray, Citation2010; Langley, Laird, & Rogers, Citation2009; Pollack, Citation1993). Several cognitive architectures exist in the literature: SOAR (Laird, Kinkade, Mohan, & Xu, Citation2004), ACT-R (Anderson, Citation1996; Stewart & West, Citation2006), CRAM (Beetz, Mösenlechner, & Tenorth, Citation2010), and SS-RICS (Kelley, Citation2006; Wei & Hindriks, Citation2013), among others. From the enumerated architectures, only CRAM has been designed having in mind a specific robotic application: the generation of pancakes by two service robots (Beetz et al., Citation2011). However, at the time of designing our general purpose service robot control, CRAM is only able to build plans defined beforehand, that is, CRAM lacks the necessary skills to solve unspecified (novel) situations. Hence, the set of actions that the robot can perform is limited to the ones that CRAM had already encoded in itself.
However, SOAR is a general cognitive architecture selecting the required skill for the current situation and goal without having a predefined list of plans or situations. This feature is a key point for general purpose service robots when improvements in the human–robot interaction (HRI) are needed. Therefore, SOAR has been selected for our approach to control the human-sized humanoid robot REEM, equipped with a set of predefined basic skills. Moreover, SOAR has been recently applied to very simple navigation tasks for a simple wheeled robot (Hanford, Citation2011). Our work will extend this previous result to a complex general humanoid robot solving complex tasks.
The rest of the paper is organised as follows: the implemented architecture is introduced in Section 2; in Section 3, the robot platform, a general purpose service robot, is described; Section 4 highlights the main results obtained during experimentation; and finally, some conclusions and future research lines are offered.
2. Implementation
The proposed overall system is composed of four main modules connected to each other as shown in Figure . Firstly, a vocal command is sent to the robot, and it is translated to text by using an automatic speech recognition (ASR) system. Next, the semantic extractor module splits the received text into grammatical structures and a goal is generated with them. Then, the goal is compiled in the reasoner module and is sent to the cognitive architecture (SOAR). Finally, all the actions selected by the SOAR architecture are translated into skill activations. The required skills are activated through action nodes in the robotic operating system.
Figure 1. Structure of the overall system developed to translate high-level commands into robotic actions.
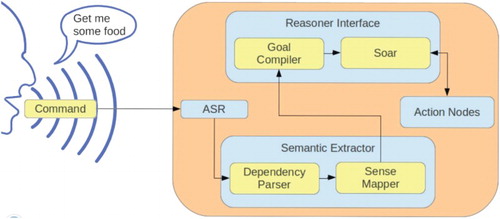
2.1. The ASR module
In order to allow natural voice communication, the system incorporates an ASR module processing speech signals and returns them as text for subsequent semantic analysis. Hence, a natural way of HRI is provided. The ASR is the element allowing the translation of voice commands into written sentences. The ASR software employed in our architecture is based on the open source infrastructure Sphinx (Ravishankar, Citation1996) developed at Carnegie Mellon University. A dictionary of 200 words has been considered. In case the robot receives a command with an unknown word, it will not accept the command and will request for a new command.
2.2. The semantic extractor module
The semantic extractor module is in charge of processing the imperative sentences received from the ASR module, extracting and retrieving the relevant knowledge from it. The robot can be commanded using two types of sentences:
Category I. The command is composed by one or more short, simple and specific sub-commands, each one referring to a very concrete specific action. For instance, ‘go to the kitchen, search a person and introduce yourself’. Please check if this is ok. Allowed sub-commands for the robot are the ones defined in Table .
Category II. The command is under-specified and requires further information from the user. The command could either, have missing information or be composed of categories of words instead of specific objects. Examples of sentences are ‘bring me a Coke’ or ‘bring me a drink’. The first example does not include information about where the drink is. The second example does not explain which kind of drink the user is asking for.
The implemented semantic extractor extracts the sub-commands contained in the command, when these actions are connected in a single sentence by conjunctions (‘and’), transition particles (‘then’) or punctuation marks. It must be noted that, given that the output comes from an ASR software, all the punctuation marks are omitted.
It is supposed that commands are expressed with imperative sentences. In this form, it is explicitly denoted that the aim of the speaker is that the robot performs a certain action. Actions are always represented by a verb. Although a verb may convey an occurrence or a state of being, as in ‘become’ or ‘exist’, in the case of imperative sentences or commands the verb must be an action. Moreover, it is also assumed that any command will ask the robot to do something and these actions might be performed involving a certain object (‘grasp a Coke’), location (‘navigate to the kitchen table’) or a person (‘bring me a drink’).
In commands from Category I, the semantic extractor extracts from the heard sentence the specific robot action and the object, location or person that this action has to act upon. In Category II, commands do not necessarily contain all the information for their execution. The semantic extractor figures out which is the action and identifies which information is missing in order to accomplish it.
For semantic extraction a parser was built using the Natural Language ToolKit (NLTK) (Bird, Citation2005). A context-free grammar (CFG) was designed to perform the parsing. Other state-of-the-art parsers like the Stanford Parser (Klein & Manning, Citation2003) or the Malt Parser (Hall, Citation2006) were discarded because they do not have support for imperative sentences, having been trained with deviated data or needing to be beforehand trained. The semantic extractor analyses dependencies, prepositional relations, synonyms and, finally, co-references.
Using this CFG, the knowledge retrieved from each command by the parser is stored on a structure called parsed-command. Each parsed-command contains the following information:
Which action is needed to perform.
Which location is relevant for the given action (if any).
Which object is relevant for the given action (if any).
Which person is relevant for the given action (if any).
The parsed-command structure is enough to identify most of the goals for a service robot at home, like [grasp – coke] or [bring – me – coke]. For multiple goals (like in the sentences from Category I), an array of parsed-commands is generated, each one populated with its associated information.
The process works as follows: firstly, the sentence received from the ASR is tokenised, that is, the stream of text is broken into a token per word. The NLTK toolkit includes an already trained tagger that implements Part-Of-Speech (POS) tagging functions for English. Then, tagging functions tag all the previous tokens with tags that describe which is the more plausible POS for each word. In this way, by applying POS-tagging, the verbs are found, and the action field of the parsed-command is filled with it.
At this point the action or actions that are needed to eventually accomplish the goal in the command have been already extracted. The next step is to obtain their complements (object, location and/or person). In order to achieve this, a combination of two methods is used:
An ontology allows to identify from all the nouns in a sentence which words are objects, persons or locations.
Dependencies between words in the sentence are found. Having a dependency tree allows identification of which parts of the sentence are connected to each other and, in that case, identify which connectors do they have. So, a dependency tree allows to find which noun acts as a direct object of a verb. Additionally, looking for the direct object allows to find the item over which the action should be directed. It is the same with the indirect objects or even locative adverbials.
2.3. The knowledge about the world
There are five types of information that the robot encodes about the world in order to properly operate the following:
A map of the environment where the robot has to work. This map is used to navigate around the locations. Hence, the map also contains the information about the locations where the robot can perform actions. Example of locations include specific rooms like the kitchen, the entry or the main room, but also specific fixed objects where an action can be performed, like the main table, the trash bin or the entry door.
A very simplistic ontology that contains all the actions, names of objects, names of people and names of locations that the robot can understand through the speech module translated to the reasoner module. This ontology includes which actions are applicable to which elements (locations, people and objects) and a knowledge base including the default location of some objects and the category of some objects and locations (when applicable, an object could be classified as drink, food or kitchenary, while a location could be a seat, a room or a table).
A database (DB) with the models of the objects that the robot is able to recognise. This is a database of 2D/3D models of the objects that the robot is able to recognise and grasp.
A database of the faces that the robot is able to recognise.
A simple database, within the cognitive architecture, with the current knowledge about the state of the world including the state of the robot, objects and persons. This includes, but it is not closed to, where an item is located at present, if it has already been grasped, the current state of the robot gripper or the current location of the robot or a specific person. A virtual representation of the world, the desired world, is also included. It encodes only the specific states needed for a goal to be considered as accomplished.
2.4. The reasoner module
A compiler is firstly designed in the reasoner module producing goals from the received parsed-command in an understandable format for the SOAR sub-system, called compiled-goal. It may happen that the command lacks some of the relevant information to accomplish the goal (Category II). Hence, this module is responsible for checking that there exists at least the necessary information to complete the goal, as well as for asking the correct questions required to complete this missing information. For example, in the command ‘bring me a drink’, the robot will check in its ontology for the category [drink]. It will then generate a question asking for which kind of drink the speaker is asking for, checking that the answer matches any of the words in the ontology. Next, the program will check the ontology again to determine whether it knows the usual location of that object or it should get that information, too. Once all the required information is obtained, goals are compiled and they are sent to the SOAR module.
2.4.1. Selecting the cognitive architecture
A main goal in this work is to endow general purpose service robots with the capacity to generate new behavioural patterns without the specific intervention of humans. A number of cognitive architectures provide a framework with this aim, such as CRAM, SS-RICS, ACT-R or SOAR. Hence, it is a key point to describe which features are interesting for our work in order to choose among these architectures. The features to be evaluated when selecting the cognitive architecture are the following:
Generalisation. The architecture, given a specific problem, should be able to extrapolate behaviours to other similar problems, as a Case Based Reasoner does.
Learning. Given a new goal that was not specifically programmed to be solved, the selected architecture should find a solution and learn it for later occurrences of the same case.
Symbolic Reasoning. A symbolic reasoner is needed for high-level control. The actions are assumed to be already implemented in the robot, so the interest is on choosing the most appropriate action at every execution step.
Scalability. The architecture to be chosen should allow easy scaling to more goals and actions, with a very little programming effort.
A comparison between the different above-mentioned cognitive architectures is shown in Table . It is clear that there is no so much difference between ACT-R and SOAR, but their abilities to work in low-level control tasks and its usability. In fact, ACT-R is easier to learn, but more complex to understand and debug than SOAR. CRAM and SS-RICS were discarded at the very first moment because their main purpose is reasoning at a sub-symbolic level, what would be useful to let the robot choose the trajectory of its arm in a grasping action, but this is out of the scope of this work. SOAR was finally selected over ACT-R for its simplicity and the available documentation.
Table 1. Comparison between different cognitive architectures.
Table 2. Table of skills available at the robot and their associated actions.
2.4.2. Using SOAR as the reasoner module
The SOAR module is in charge of determining which skills must be executed in order to achieve the compiled goal. A loop inside SOAR selects the skill that will push REEM one step closer to the goal. Each time a skill is selected, a petition is sent to an action node to execute the corresponding action. Each time a skill is executed and finished, SOAR selects a new one. SOAR will keep selecting skills until the goal is accomplished.
The set of skills that the robot can activate are encoded as a list of operators. Hence, for each possible action:
a rule proposes the operator, with the corresponding name and attributes;
a rule sends the command through the output link if the operator is accepted;
one or several rules, depending on the command response, fire and generate the necessary changes in the world.
Given the nature of the SOAR architecture, all the proposals will be treated at the same time and will be compared in terms of preferences. If one of the proposals is better than the others, this one is the only operator to be executed and a new deliberation phase will begin with all the new available data. It is important to know that all the rules that match the conditions are treated as if they are fired at the same time, in parallel. There is not a sequential order (Wintermute, Xu, & Laird, Citation2007). Once the goal or list of goals have been sent to SOAR the world representation is created.
SOAR requires an updated world state in order to produce the next decision. The world state is updated after each skill execution in order to show the robot interactions with the world. The world could be changed by either the robot itself or other existing agents. Changes in the world made by the robot actions directly reflect the result of the skill execution in the robot world view. When changes in the world are made by other agents, the robot could fail the execution of the current skill, provoking the execution of another skill that tries to solve the impasse (e.g. going to the place where the Coke is and finding that the coke is not there anymore, will trigger the Search for object skill to figure out where the Coke is).
Hence, after resolving the action, an object is returned to the SOAR module describing the success/failure of the action and the relevant changes it provoked. This information is used to change the current knowledge of the robot. For instance, if the robot detected a beer bottle and its next skill is to grasp it, it will send the command grasp.item = beer_bottle, while the action response after resolving should only be a ‘succeeded’ or ‘aborted’ message that is interpreted in SOAR as robot.object = beer_bottle. For example, during our experimentation, 10 different skills were implemented. The amount of rules checked in every loop step were 77.
It may also happen that there is no plan for achieving the goal. In those situations, SOAR implements several mechanisms to solve them:
Sub-goal capacity (Laird, Newell, & Rosenbloom, Citation1987) allows the robot to find a way to get out of an impasse with the available current actions in order to achieve the desired state. This would be the case in which the robot could not decide the best action in the current situation with the available knowledge because there is no distinctive preference.
Chunking ability (Howes & Young, Citation1997; Laird et al., Citation1987) allows the production of new rules that help the robot adapt to new situations and, given a small set of primitive actions, execute full featured and specific goals never faced before.
Reinforcement learning (Nason & Laird, Citation2005), together with the two previous features, helps the robot in learning to perform maintained goals such as keeping a room clean or learning by the use of user-defined heuristics in order to achieve not only good results like using chunking, but near-optimal performances.
The two first mechanisms were activated for our approach. The use of the reinforcement learning will be analysed in a future work. Those two mechanisms are especially important because, thanks to them, the robot is able to find its own way to get any reachable goal with the current skills of the robot. Moreover, chunking makes decisions easier when the robot faces early experienced similar situations. These strengths allow robot adaptation to new goals and situations without further programming but either by defining a goal or admitting the expansion of its capabilities by simply defining a new skill.
2.5. The action nodes module
Action nodes are robot operating system (ROS) software elements. They are modular pieces of software implemented to allow the robot to perform its abilities, defined in the SOAR module as possible skills. Every time that the SOAR system proposes a skill to be performed, it calls the action node in charge of that skill. When an action node is executed, it provides some feedback to the SOAR system about its success or failure. This feedback is captured by the interface, and it is sent to the SOAR in order to update the current state of the world. The set of skills implemented and their associated actions are described in Table .
3. The robot platform: REEM
REEM is the robot platform used for testing the developed system (Figure ). It is a 22 degrees of freedom humanoid service robot created by PAL Robotics, weighting about 90 kg and its autonomy is about 8 hours. REEM is controlled by open robot control software (OROCOS) for real-time operations and by ROS for skill depletion. Among other abilities, it can recognise and grasp objects, detect faces, follow a person and even clean a room of objects that do not belong to it. In order to include robust grasping and gesture detection, a Kinect sensor on a headset on its head has been added to the commercial version. The robot is equipped with a Core 2 Duo and an ATOM computer, which provide all the computational power required to perform all tasks control. Therefore, algorithms required to plan and perform all the abilities are executed inside the robot.
4. Experimentation and results
The whole architecture has been tested in an environment that mimics that of the RoboCup@Home League at the GPSR testFootnote1 (Figure ). In this test, the robot listens three different types of commands with increasing difficulty and executes the required actions (skills) to accomplish the command. For our implementation, only the two first categories have been tested, as described in Section 2.2.
Testing involves to provide the robot with a spoken command, and checking that the robot is able to perform the required actions to complete the goal. Examples of sentences the robot has been tested with (among others) are the following:
Category I. Complete sentences.
‘Go to the kitchen, find a Coke and grasp it’
Sequence of actions performed by the robot: understand command, go to kitchen, search for coke, grasp coke
‘Go to reception, find a person and introduce yourself’
Sequence of actions performed by the robot: understand command, go to reception, search person, go to person, introduce yourself
‘Find the closest person, introduce yourself and follow the person in front of you’
Sequence of actions performed by the robot: search person, go to person, introduce yourself, follow person
‘Point at a seating’
Sequence of actions performed by the robot: understand command, ask questions, acknowledge all information, go to location, search seating, point at seating
‘Carry a snack to a table’
Sequence of actions performed by the robot: understand command, ask questions, acknowledge all information, go to location, search snack, grasp snack, go to table, deliver snack
‘Bring me a drink’ (Figure )
Sequence of actions performed by the robot: understand command, ask questions about which drink, acknowledge all information, go to location, search energy drink, grasp energy drink, go to origin, deliver energy drink
Figure 4. Sequence of actions done by the REEM robot to solve the command ‘Bring me a drink’: Listen to command, request missing information and acknowledge full command, go to kitchen, look for objects, detect energy drink, grasp energy drink, return to master, deliver energy drink.
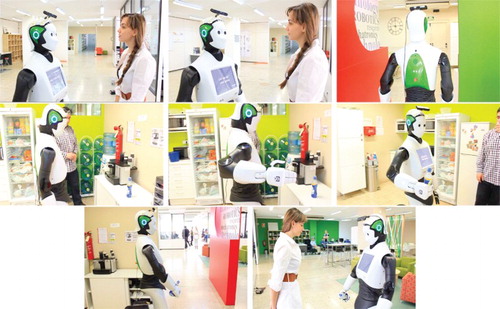
The system presented in this paper ensures that the actions proposed will lead to the goal, so the robot will find a solution, although it is not guaranteed that it leads to the optimal one. Due to the complexity of the tasks, sometimes the robot will face ambiguous situations in which SOAR must resolve ties between the best action to perform. Being SOAR a cognitive architecture, it will usually sub-goal to resolve for the best one but when there is no evidence on which is the best action, ties are resolved at random. For instance, in some situations, the robot moved to a location that was not the correct one, before moving on a second action step to the correct one. However, the completion of the task is guaranteed since the architecture will continue providing steps until the goal is accomplished.
4.1. Including new goals
One of the main features of this SOAR-based system is how easy is to solve new goals without having to encode new plans. Our implementation of SOAR includes a representation of a desired state of the world. This desired state is compiled from the output of the semantic extraction module, while a process that compares the real and the desired states of the world detects when the goal is fulfilled. By the simple fact of adding to SOAR a new desired state of the world, it will use the available skills in order to get closer to this new goal.
The addition of this new desired state of the world is achieved through the following two steps:
First of all, the grammar for the ASR and the semantic extractor must be updated. This step is required in order to make the robot understand the new goal and identify it as an action to be performed. The grammar is updated by just adding the word in the list of verbs defined in the grammar.
As a second step, the world state for the new goal must be defined. The goal compiler sub-module is in charge of producing the goal in a format understandable by SOAR from the received parsed-command. Consequently, this module has to be updated by adding the new goal with the new desired state of the world in the goal compiler.
As an example, the robot is provided with a new goal to be accomplished, that is, ‘empty the kitchen table’.
For the purpose of this example, the initial state of the world was modified including the existence of a kitchen table with a marmalade jar on it. A new location named ‘trash bin’ was defined in the robot navigation map. This location is where the robot has to deliver all the objects removed from the table.
Following the provided example, the two steps to be completed are the following:
add the word ‘empty’ in the list of verbs defined in the grammar;
add into the goal compiler a new goal named empty and define this goal as a world state where no object is located in the given location.
Once these steps were completed, the robot was asked to clean the table. The sequence of actions performed by the robot was the following: go to the kitchen table, search objects, grasp marmalade, go to the trash bin, deliver marmalade.
Therefore, the new goal of cleaning the table was achieved by using skills already endowed in the robot (go to, search, grasp, etc.).
To sum up, it is clear now that this architecture is flexible enough to compile new goals without having to encode neither new plans nor new skills by just performing minor updates.
4.2. Robot agnostic
The introduced architecture is robot agnostic provided that the skills required are implemented for the robot in which the architecture is going to be used. It means that the whole architecture can be used in any other robot without changing the architecture. The only requirement is that the skills of the robot provide the appropriate inputs and outputs in the expected format by the architecture.
In fact, once the speech recognition skill provides the heard sentence, the semantic extractor and the reasoner interface (as defined in Figure ) do not depend on any particular aspect of the robot architecture. Only the part that actually calls the action node is robot dependent, that is, the part that calls the different skills of the robots.
If the architecture is going to be used in another robot, the only requirement is that the skills must implement two things:
The ability of the robot to perform the required skill (e.g. allow the robot to grasp or recognise speech).
The proper interface in the skill to be called from the cognitive architecture. This interface is implemented using a standard ROS-action-server interface.
5. Conclusions and further research
A cognitive architecture based on SOAR has been introduced for the control of general purpose service robots. This architecture allows to command a commercial humanoid robot (the PAL Robotics REEM robot in the experimentation) to perform several tasks as a combination of skills. As a key issue, how basic skills should be combined in order to solve the task is not beforehand specified. The whole approach avoids artificial intelligence planning in the classical sense and uses instead a cognitive approach (SOAR) based on solving the current situation faced by the robot. By solving the current situation skill by skill the robot finally reaches the goal (if it is reachable). Given a goal and a set of skills, SOAR itself will generate the necessary steps to fulfil the goal using the skills (or at least try to reach the goal). Hence, it can be said that it can easily adapt to new goals, effortlessly.
The original SOAR architecture cannot detect whether the goal requested to the robot is reachable or not. If it is not, SOAR will keep trying to reach it, and it will send skill activations to the robot forever. In our implementation, the set of goals that one can ask the robot are restricted by the speech recognition system. Our system ensures that all the accepted vocal commands are reachable by an SOAR execution.
The whole architecture is completely robot agnostic. It can be adapted to any other robot provided that the skills exist in the robot and are added to the interface. Moreover, adding and removing skills becomes as simple as defining their outcomes, as seen in Section 4.1. This feature makes this architecture extremely adaptable and easy to implement in any robot.
The current implementation can be improved in terms of robustness by solving two known issues. Firstly, if one of the actions is not completely achieved (e.g. the robot is not able to reach a position in the space because it is occupied, or the robot cannot find an object that is in front of it), the skill activation will fail. However, in the current implementation, the robot has no means to discover the reason for the failure. Hence, the robot will detect that the state of the world has not changed, and it will select the same action (retry) towards the goal accomplishment. This behaviour could lead to an infinite loop of retries. The second issue refers to the lack of this architecture to solve commands when errors in sentences are encountered (Category III in the GPSR Robocup@Home Test). Future versions of the architecture should include this feature by including semantic and relation ontologies like Wordnet (Miller, Citation1995) and VerbNet (Palmer, Kipper, Korhonen, & Ryant, Citation2006), making this service robot more robust and general.
Some preliminary experiments have been done in more realistic environments. We observed that the most limiting factors are still in the hardware, the control algorithms and the speech recognition of natural language. Although this high-level control system virtually allows the fulfilment of almost any order encoded as a goal, current robots present still severe difficulties to achieve with enough robustness each of the most basic skills. This makes the scalability still far from now. Although this, solving the aforementioned robustness issues, knowing the ability of this system to solve new tasks, would be the necessary step to allow the system to be scalable to real environments on any kind of robot. These additions would allow the robot to evaluate the achievability of the provided tasks and, although this does not guarantee that the robot will perform as desired, it could report to the user any problem or even solve it by other means.
Acknowledgements
This work was possible due to the support of Pal Robotics and all the members of its Robocup@Home team (the REEM@IRI team). J-Y. Puigbo and A. Pumarola contributed equally to this work.
Disclosure statement
No potential conflict of interest was reported by the authors.
Funding
This research was partly supported by the PATRICIA Research Project (TIN2012-38416-C03-01), funded by the Spanish Ministry of Economy and Competitiveness.
Notes
1. RoboCup@Home Rules and Regulations.
References
- Anderson J. R. (1996). ACT: A simple theory of complex cognition. American Psychologist, 51(4), 355–365. doi: 10.1037/0003-066X.51.4.355
- Beetz, M., Klank, U., Kresse, I., Maldonado, A., Mösenlechner, L., Pangercic, D., … Tenorth, M. (2011, October). Robotic roommates making pancakes. 11th IEEE-RAS international conference on humanoid robots, Bled, Slovenia (pp. 529–536).
- Beetz, M., Mösenlechner, L., & Tenorth, M. (2010, October). CRAM – a cognitive robot abstract machine for everyday manipulation in human environments. International Conference on Intelligent Robots and Systems (IROS), Taipei, Taiwan (pp. 1012–1017).
- Bird, S. (2005, June). NLTK: The Natural Language Toolkit. Proceedings of the ACL workshop on effective tools and methodologies for teaching natural language processing and computational linguistics, Philadelphia, PA (pp. 1–4).
- Chen, X., Ji, J., Jiang, J., Jin, G., Wang, F., & Xie, J. (2010, May). Developing high-level cognitive functions for service robots. Proceedings of the 9th international conference on autonomous agents and multiagent systems: Volume 1, AAMAS'10, Toronto, Canada (pp. 989–996).
- Haidegger, T., Barreto, M., Gonçalves, P., Habib, M. K., Ragavan, S. K. V., Li, H., … Prestes, E. (2013). Applied ontologies and standards for service robots. Robotics and Autonomous Systems, 61(11), 1215–1223. doi: 10.1016/j.robot.2013.05.008
- Hall, J. (2006). MaltParser – an architecture for inductive labeled dependency parsing (Technical Report 06050). Växjö University, School of Mathematics and Systems Engineering.
- Hanford, S. D. (2011). A Cognitive robotic system based on the soar cognitive architecture for mobile robot navigation, search, and mapping missions (PhD thesis). University Park, PA, AAI3501001.
- Howes, A., & Young, R. M. (1997). The role of cognitive architecture in modeling the user: Soar's learning mechanism. Human-Computer Interaction, 12(4), 311–343. doi: 10.1207/s15327051hci1204_2
- Jones, R. M. (2004, July). An introduction to cognitive architectures for modeling and simulation. Proceedings of the Interservice/Industry Training, Simulation and Education Conference, I/ITSEC, Orlando, FL.
- Kelley, T. D. (2006). Developing a psychologically inspired cognitive architecture for robotic control: The symbolic and subsymbolic robotic intelligence control system. International Journal of Advanced Robotic Systems, 3(3), 219–222.
- Klein, D., & Manning, C. D. (2003, July). Accurate unlexicalized parsing. ACL '03 Proceedings of the 41st annual meeting on association for computational linguistics, Sapporo, Japan (Vol. 1, pp. 423–430).
- Laird, J. E., Kinkade, K. R., Mohan, S., & Xu, J. Z. (2004, July). Cognitive robotics using the soar cognitive architecture. Proceeding of the 6th international conference on cognitive modelling, Pittsburg, PA (pp. 226–230).
- Laird, J. E., Newell, A., & Rosenbloom, P. S. (1987). SOAR: An architecture for general intelligence. Artificial Intelligence, 33(1), 1–64. doi: 10.1016/0004-3702(87)90050-6
- Laird, J. E., & Wray, R. E., III (2010, March). Cognitive architecture requirements for achieving AGI. Proceedings of the third conference on artificial general intelligence, Lugano, Switzerland.
- Langley, P., Laird, J. E., & Rogers, S. (2009). Cognitive architectures: Research issues and challenges. Cognitive Systems Research, 10(2), 141–160. doi: 10.1016/j.cogsys.2006.07.004
- Miller, G. A. (1995). WordNet: A lexical database for English. Communications of the ACM, 38(11), 39–41. doi: 10.1145/219717.219748
- Nason, S., & Laird, J. E. (2005). Soar-RL: Integrating reinforcement learning with soar. Cognitive Systems Research, 6(1), 51–59. doi: 10.1016/j.cogsys.2004.09.006
- Palmer, M., Kipper, K., Korhonen, A., & Ryant, N. (2006, September). Extensive classifications of English verbs. Proceedings of the 12th EURALEX international congress, Torino, Italia (pp. 1–15).
- Pollack, J. B. (1993). On wings of knowledge: A review of Allen Newell's unified theories of cognition. Artificial Intelligence, 59, 355–369. doi: 10.1016/0004-3702(93)90200-U
- Ravishankar, M. K. (1996). Efficient algorithms for speech recognition (PhD thesis). School of Computer Science, Carnegie Mellon University, Pittsburgh. Available as CMU CS tech report CMU-CS-96-143.
- Russell, S. J., & Norvig, P. (2003). Artificial intelligence: A modern approach (2nd ed.). Englewood Cliffs, NJ: Pearson Education.
- Stewart, T. C., & West, R. L. (2006, April). Deconstructing ACT-R. Proceedings of the seventh international conference on cognitive modeling, Valencia, Spain (pp. 298–303).
- Wei, C., & Hindriks, K. V. (2013, June). An agent-based cognitive robot architecture. Programming Multi-Agent Systems (ProMAS) workshop affiliated with AAMAS 2012, Valencia, Spain (pp. 55–68).
- Wintermute, S., Xu, J. Z., & Laird, J. E. (2007, June). SORTS: A human-level approach to real-time strategy AI. Proceedings of the third artificial intelligence and interactive digital entertainment conference, AIIDE, Stanford, CA (pp. 55–60).