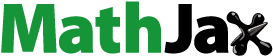
Abstract
We analyse the value of information in a stock market where information can be noisy and costly, using techniques from empirical game theory. Previous work has shown that the value of information follows a J-curve, where averagely informed traders perform below market average, and only insiders prevail. Here we show that both noise and cost can change this picture, in several cases leading to opposite results where insiders perform below market average, and averagely informed traders prevail. Moreover, we investigate the effect of random explorative actions on the market dynamics, showing how these lead to a mix of traders being sustained in equilibrium. These results provide insight into the complexity of real marketplaces, and show under which conditions a broad mix of different trading strategies might be sustainable.
1. Introduction
Markets play a central role in today's society and find wide application ranging from stock markets to consumer-to-consumer e-commerce (Angel, Citation2002; Bajari & Hortacsu, Citation2003). Success in market trading will greatly depend on traders having accurate market forecasts. There are two main types of trading strategies in today's markets: fundamentalists and chartists (Gehrig & Menkhoff, Citation2006; Taylor & Allen, Citation1992). Fundamentalists use a forecasting model that fits the actual economy and correctly identify the fundamental driving forces of the market. Technical analysts, also called chartists, use an autoregressive process to predict future price developments based on recent trends. In this paper we focus on fundamentalists, more specifically we look at the value of forecasting information by comparing the financial success of differently informed traders.
One might conjecture that more information is always better – if you know everything, you can act perfectly. However, previous work has shown that this does not need to be generally the case. In particular, it has been found both in simulation and in human experiments that averagely informed traders may be outperformed by uninformed traders that follow solely the current market price, and only insiders beat the market (Kirchler, Citation2010; Tóth, Scalas, Huber, & Kirchler, Citation2007). This effect leads to a so-called J-curve describing the value of information. One possible theory explaining this phenomenon is that more information helps during trends, whereas limited knowledge may be erroneous when the trend reverses; uninformed traders are safe from these systematic mistakes (Huber, Citation2007). These findings were recently confirmed in simulation for larger numbers of traders and different distributions over traders' information levels (Hennes, Bloembergen, Kaisers, Tuyls, & Parsons, Citation2012). Moreover, an evolutionary analysis of the market showed that indeed only insiders can prevail when information is freely available. When information comes at a price, a mix of differently informed traders may be sustained in equilibrium (Hennes et al., Citation2012).
Another factor that may greatly influence market outcomes is the presence of noise. Especially forecasting information may well be unreliable or imprecise, leading to a diminishing return on investment for information. In this paper we analyse the effect of noise by comparing different noise functions in a market with variously informed traders. Moreover, we compare the influence of noise and the influence of cost. Combining both cost and noise gives rise to a tipping point where the acquisition of additional information costs more than it pays off. Finally, we investigate the effect of exploration (or mutation, in evolution terms) on the market dynamics. In this setting, traders do not greedily pick the optimal action all the time, but with small probability choose randomly. This small change may yield qualitatively different dynamics, where trading strategies that are otherwise driven out of the market prevail.
The remainder of this paper is structured as follows. Section 2 briefly introduces auctions, evolutionary game theory and heuristic payoff tables, concepts necessary to understand the remainder of this work. The market model used in our experiments is detailed in Section 3. Experiments are described and results are presented in Section 4. Section 5 concludes.
2. Background
This section provides relevant background needed for the remainder of this paper. Firstly, auctions and their application in stock markets are detailed. Secondly, evolutionary game theory and in particular the replicator dynamics are described. Finally, heuristic payoff tables, forming the core of our evolutionary analysis, are introduced.
2.1. Auctions
Auctions are highly efficient match making mechanisms for trading goods or services. As such, they are employed by a number of real markets, such as telecommunication spectrum rights auctions or the New York Stock Exchange (NYSE) (Angel, Citation2002; McMillan, Citation1994). In practice, there is a variety of rules that may be used to conduct an auction. Each set of rules may result in different transaction volumes, transaction delays, or allocative market efficiency. One sided auctions, especially with one seller and many potential buyers, are popular in consumer-to-consumer e-commerce (Bajari & Hortacsu, Citation2003; Barrot, Albers, Skiera, & Schäfers, Citation2010). Here, we focus on double auctions, which essentially provide a platform for buyers and sellers to meet and exchange a commodity against money. A taxonomy of double auctions especially tailored to automated mechanism design can be found in Niu, Cai, Parsons, Fasli, and Yao (Citation2012).
Double auctions maintain an open book of bids (offers to buy at a specified price) and asks (offers to sell at a specified price). Two principle forms are the clearing house auction and continuous operation auction. In a clearing house auction, orders are collected for a trading period (e.g. one day) and matched, or cleared, after the trading period is closed. This mode of operation allows for high allocative efficiency, but incurs delays in the transactions. In contrast, continuous operation immediately establishes a transaction as soon as some buyer is willing to pay more than a seller is asking for. This mode allows higher transaction rates at the cost of some allocative efficiency. Experiments in this article will use continuous operation mode, since it reflects the day-time operation mode of the NYSE (Angel, Citation2002).
2.2. Evolutionary game theory
Auctions provide a dynamic environment with a lot of traders (agents) that adapt to each other while competing for revenue. Learning in such multi-agent systems is generally complex and poses many challenges that inspire prescriptive, descriptive and normative research (Shoham, Powers, & Grenager, Citation2007). Evolutionary game theory provides a methodology to analyse multi-agent learning, replacing assumptions from game theory like rationality by evolutionary concepts such as pressure of natural selection (Tuyls & Parsons, Citation2007).
The evolutionary perspective considers a population of individuals, where each individual belongs to one of several species. These species generally relate to atomic strategies, or to information levels within this article. Two core concepts are the
replicator dynamics, describing how a population evolves, and evolutionarily stable states. The replicator dynamics formally define the population change over time, where
describes the population share of each of n species in the population:
(1)
(1) The payoff function
can be interpreted as the Darwinian fitness of each species i. Intuitively, Equation (Equation1
(1)
(1) ) describes how species that do better than average in the population thrive, whereas species that do worse decline. Evolutionarily stable state are such population distributions
that are fixed points of the replicator dynamics, that is,
, and where small perturbations
would be driven back to
by selection pressure, that is, by following the replicator dynamics.
The dynamical model of Equation (Equation1(1)
(1) ) only describes the evolutionary process of
selection. However, in many scenarios mutation also plays a role, where individuals not only reproduce, but may change their behaviour while doing so. Given a population
as defined above, we consider a mutation rate
indicating the propensity of species j to mutate into i (note the order of the indices), such that,
:
Adding mutation to Equation (Equation1
(1)
(1) ) leads to a dynamical model with separate selection and mutation terms (Hofbauer & Sigmund, Citation1998), given by
(2)
(2)
Previous research has demonstrated the viability of evolutionary game theory to analyse meta strategies in simulated auctions, and to compare clearing house against continuous double auctions (Kaisers, Tuyls, Parsons, & Thuijsman, Citation2009; Phelps, Parsons, & McBurney, Citation2005). We will follow a similar analysis procedure but our data is generated by a different model, described in Section 3.
2.3. Heuristic payoff tables
In order to apply the evolutionary game theoretic analysis to a complex system such as stock market trading, we need a way to estimate the payoff functions of the different trading strategies in relation to each other. We can do so by simulating the stock market with different distributions of trading strategies, and recording their relative market return. Payoff functions are typically continuous, and in particular the evolutionary model of Equation (Equation1(1)
(1) ) assumes an infinite population. We cannot derive the payoff for such a population directly, but we can approximate it from evaluations of a finite population.
All possible discrete distributions over k trading strategies can be enumerated for a finite population of n traders. Let N be a matrix, where each row contains one such distribution. The matrix will yield
rows. Using a simulated market model we can estimate the payoffs for each strategy in the distribution, returning a vector expected utilities,
. Let U be a matrix which captures the revenues corresponding to the rows in N, that is,
. A heuristic payoff table
is proposed by Walsh, Das, Tesauro, and Kephart (Citation2002) to capture the payoff information for all possible discrete distributions in a finite population. A stylised example of such a heuristic payoff table is given in . In this example, we have
different strategies, distributed over a population of
individuals. Each row in N specifies exactly how many individuals use each of the three strategy types, and each row in U specifies their estimated payoff based on the simulation results. If a discrete distribution
features zero individuals of type j, their payoff naturally cannot be measured, and we set
. For example, the third row in indicates a distribution consisting of three traders of type one, one trader of type two, and two traders of type three. Using simulations we find that (hypothetically) type one has an expected payoff of 0.3, type two gets 0.5, and type three gets 0.8.
Table 1. Stylised example of a heuristic payoff table for 
strategies and a finite population of 
individuals.
In order to approximate the payoff for an arbitrary mix of strategies in an infinite population distributed over the species according to , n individuals are drawn randomly from the infinite distribution. The probability for selecting a specific row
can be computed from
and
:
The expected payoff
is then computed as the combination of the payoffs in all rows, weighted by their probability given
:
This expected payoff can be used in Equation (Equation1
(1)
(1) ) to compute the evolutionary population change according to the replicator dynamics.
3. Market model
The market is based on a continuous double auction with open order book, in which all traders can place bids and asks for shares. We closely follow the market model as described by Hennes et al. (Citation2012), Huber, Kirchler, and Sutter (Citation2008), and Tóth and Scalas (Citation2008) in order to be comparable. The intrinsic value of the shares is determined by a dividend stream that follows Brownian motion:
where
denotes the dividend in period t with
, and ε is a normally distributed random term with
and
, that is,
.
We simulate the market over 30 trading periods, each lasting time steps, where n is the number of traders present. All traders start with 1600 units cash and 40 shares, each worth 40 in the beginning. At the beginning of each period, all traders can put a bid or ask in the book (opening call). Hereafter, at every time step a trader is selected at random who can then either accept an open order, or place a bid or ask according to its trading strategy (see below). At the end of each period, dividend is paid based on the shares owned, and a risk free interest rate (0.1%) is paid over cash. The performance of the traders is measured as their total wealth after the 30 periods, that is, each share is valued according to the discounted future dividends (see below) and added to the cash reserves.
3.1. Dividend discount model
Let us assume that we require a certain rate of return on our investment. For example, if
, a share must return
per trading period for it to be a worthwhile investment. Rate r is also called the discount rate. A future dividend
at time t has a current discounted value of
If we intend to hold the share indefinitely, the value of a share is equal to the sum of future discounted dividends:
Gordon's growth model (Gordon, Citation1962) assumes that dividends grow at a constant rate g. If
is the current dividend payout, the current stock value can be computed as follows:
Let us assume the dividends are constant over time, that is,
and
. The stock value simplifies to:
(3)
(3) The infinite series Equation (Equation3
(3)
(3) ) converges to
as
with
. For example, a stock that pays a constant dividend of
per share has a current value of
.
The different information levels are implemented by varying the amount of knowledge that traders have about future dividends. In trading period , traders of information level
know dividend
and in general traders of information level
know
. Therefore, the discounted dividend payoff that is guaranteed with information level
is
and the future discounted dividends for
are estimated according to Equation (Equation3
(3)
(3) ) with a constant
:
(4)
(4) As Equation (Equation4
(4)
(4) ) estimates future discounted dividends from period
on, Equation (Equation4
(4)
(4) ) itself must be discounted by
to adjust payouts to current value prices. The complete stock value estimate for information level
is thus:
(5)
(5) To put it intuitively, a trader of information level
knows j future dividends and assumes dividends stay fixed from that point on.
3.2. Trading strategies
We use two different trading strategies in our experiments. Fundamentalist use their knowledge of future dividends ( to
) to estimate the current value of the stock and base their trading decision on that estimate. Traders without any information (
) use the
zero-information strategy that only makes use of the current market price of the shares.
3.2.1. Fundamentalists
Fundamentalists completely rely on the information they receive. The fundamentalist strategy is explained in Algorithm 1 (see also Tóth & Scalas, Citation2008; Tóth, Scalas, Huber, & Kirchler,Citation2006). In essence, they compare their estimated present value (Equation (Equation5
(5)
(5) )) with the current best bid and ask in the book. If they find a bid (ask) with a higher (lower) value than their estimate, they accept the offer. Otherwise, they place a new order between the current best bid and ask prices. Naturally, the trader should own enough shares or cash to accept or place an order.
3.2.2. Zero-information traders
The zero-information trading strategy only takes the current market price into account when deciding whether to accept or place an order. Specifically, these traders use the same Algorithm 1 with where
is the market price at time k.
3.3. Cost and noise
Three types of cost function and noise are used in our experiments. In line with Hennes et al. (Citation2012) we use a fixed cost function, where each fundamentalist pays the same fixed amount per trading period, and a quadratic cost function that is based on the idea that it gets increasingly difficult to obtain more information. Whereas some forecasting information may be acquired by reading financial news papers, a more detailed outlook may require hiring experts. Additionally we add a linear cost function, providing a middle ground between the former two. (a) shows these three different cost functions.
Similarly, we employ three different types of noise functions to model uncertainty in forecasting data, depicted in (b). Noise is added to each trader's value estimate when executing Algorithm 1, drawn randomly from . In the case of fixed noise, each trader experiences the same level of uncertainty. More realistically, the uncertainty increases with the amount of forecasting, especially when, for example, step-by-step prediction is used (Cheng, Tan, Gao, & Scripps, Citation2006). This inspires the exponential noise function. Again, a linear function is used as well as compromise between these two.
4. Results
This section details the experiments and lists their results. Two types of experiments are conducted to highlight the effect of both cost and noise on the relative return for differently informed traders. First, the population of traders is kept fixed, allowing us to visually investigate the market result when all information levels are present. Next, traders are allowed to switch their strategy if this is profitable. Evolutionary analysis of the resulting dynamical system indicates which strategy or information level is strongest from a natural selection point of view. Moreover, this analysis shows how the market evolves, and which strategy or strategies are economically viable in the long run under different scenarios.
4.1. Fixed market simulations
Previous work has shown that the value of information does not necessarily increase monotonically for traders in a stock market, but rather follows a J-curve (Hennes et al., Citation2012; Kirchler, Citation2010; Tóth et al., Citation2007). Here we extend these results to situations in which information can be costly or subject to uncertainty. We simulate the market (see Section 3) with traders, 10 for each of the information levels
. Different scenarios are investigated using the various noise and cost functions described previously in Section 3.3. To reduce the effect of randomness we run 100 sessions of 100 simulations for each scenario; the dividend stream is fixed for each session. Results are given as the relative performance with respect to the market average plotted against the information levels.
shows the relative return over information level for different cost functions. When no cost is involved, the market behaves as expected based on previous work (Hennes et al., Citation2012; Kirchler, Citation2010; Tóth et al., Citation2007). Traders with little information are not able to make any profit, and would be better off not using their fundamental information at all. Both zero-information traders and averagely informed traders perform at market average, and only highly informed insiders beat the market. Clearly, insiders are able to exploit those traders that rely fully on their limited forecasting abilities, whereas they are actually missing important trends that insiders are aware of. Moreover, the fact the uninformed traders achieve market average performance indicates that this strategy is indeed a safe choice. Adding fixed cost or linear cost does not change the result dramatically, and the J-curve is preserved. However, zero-information traders profit from not having to pay any cost; as a result their performance is now significantly above market average. The situation changes when quadratic costs are incurred. Here, a tipping point can be observed when acquiring additional information costs more than it adds, as also reported in Hennes et al. (Citation2012).
Figure 2. Relative return over information level, for a market with traders, 10 for each information level. Different cost functions are used; the noise is kept zero.
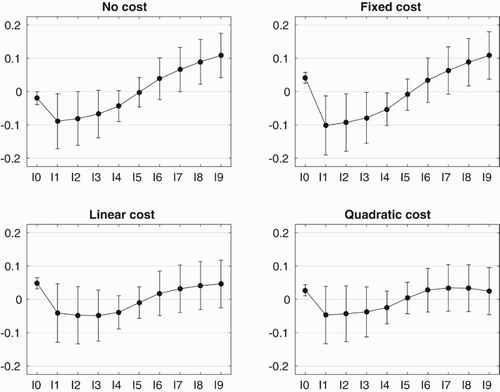
Adding noise has a similar effect, as is shown in . Insiders still win under both fixed noise and linear noise, the situation is in fact very similar to the case of fixed and linear noise reported above. However, since noise does not influence the trader's monetary wealth directly, zero-information traders are not able to profit in this case. Instead, the noisy information affects the realised market price through the fundamentalists' trading behaviour, thereby influencing the zero-information traders indirectly as well. When exponential noise is applied, the picture changes again. Similarly, we observe a tipping point where the increased uncertainty for longer forecasts outweighs their value. In other words, the signal-to-noise ratio of the forecasting information decreases with the length of forecasting. As a result, insiders are no longer able to make a profit, and perform well below market average in this case.
Figure 3. Relative return over information level, for a market with traders, 10 for each information level. Different noise functions are used; the cost is kept zero.
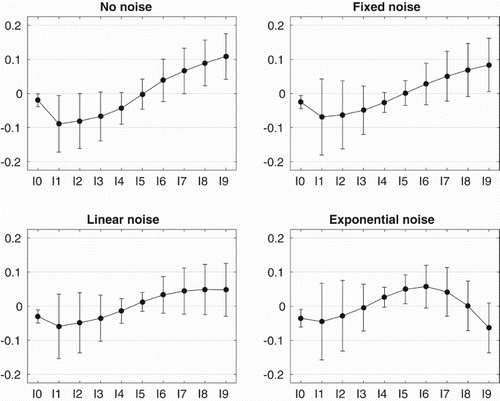
Finally, we combine cost and noise in order to see how their effects might add up. show the results. Where both linear cost and noise independently did not change traders' relative profits significantly, it is clear that their combined effect tips the scale: the relative return over information level shows again a tipping point, after which more information does not pay off anymore. Interestingly, zero-information traders outperform fundamentalists in this scenario, a fact that will be further investigated in Section 4.2. Other combinations of noise and cost show similar results, although zero-information traders no longer win. Rather, averagely informed traders do best in these settings. Exponential noise has the strongest effect, as could also be observed previously.
Figure 4. Relative return over information level, for a market with traders, 10 for each information level.
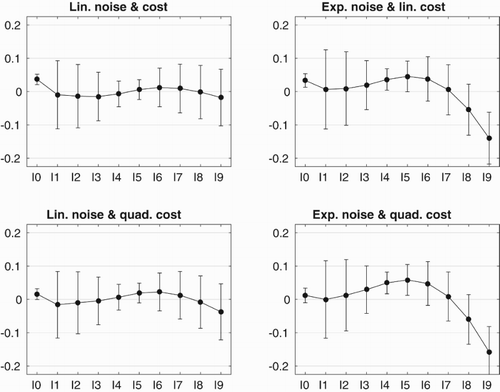
These results show that the value of information is not simply monotonically increasing. Instead, depending on factors such as noise and cost, there might be a tipping point where acquiring more information does no longer pay off. Either the cost involved becomes to large, or the increased uncertainty causes the new information to be detrimental rather than helpful.
4.2. Evolutionary analysis
So far, traders have not been able to choose their strategy, instead the distribution over information levels was kept fixed throughout the simulation. Realistically it can be assumed that traders may be inclined to switch strategy if they are currently performing poorly. In the following, we look at the dynamics of a market in which traders are free to change their strategy.
We compute heuristic payoff tables, as described in Section 2.3, for various scenarios using different cost functions and different types of noise. Each heuristic payoff table is computed using market simulations with traders of information levels
,
, and
, giving 91 rows in the payoff table for the various discrete distributions over these three types. We could have chosen different information levels, however previous work has indicated that this does not influence the results qualitatively (Hennes et al., Citation2012). For each row in the table we run the market simulation for 100 sessions of 10 simulations each. Again, the dividend stream is fixed for each session. As described in Section 2.3 we use the resulting payoff table to calculate expected fitnesses for an arbitrary mix of these strategies, and plug these in to the replicator dynamics Equation (Equation1
(1)
(1) ) yielding a dynamical system that can be inspected visually.
shows the resulting market dynamics for different cost functions. In the absence of cost the best strategy is to get as much foresight as possible, leading to a domination of traders over the entire interior of the simplex, in line with the J-curve reported earlier. Adding fixed cost gives a small boost to the zero-information traders (as can also be observed in ), allowing them to survive in equilibrium alongside the insiders. Both linear and quadratic costs give rise to an internal equilibrium where each type of trader can survive. Note however that the location of this interior is close to
, meaning that is consists of relatively many zero-information traders. The reason is again that zero-information traders are free from costs, which gives them an edge in this case.
Figure 5. Evolutionary dynamics of a market with three information levels and different cost functions for information. The noise is kept at zero.
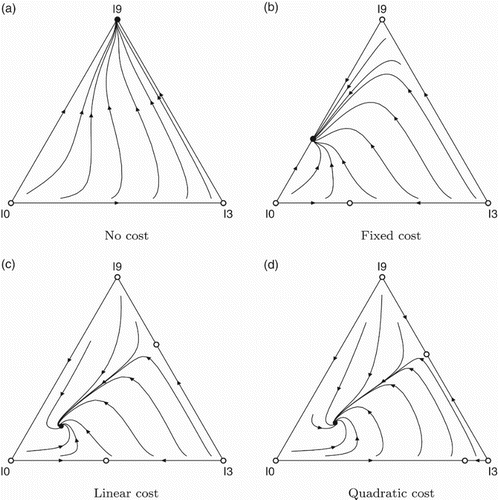
Slightly different results are obtained in the case of noise and presented in . Adding fixed noise does not change the evolutionary success of the insiders, and the pure
equilibrium is still the main attractor. Linear noise shifts the equilibrium to a mix of insiders and averagely informed traders; exponential noise leads to a situation where only averagely informed traders prevail – interestingly, in each scenario the zero-information traders are driven out of the market. Compared to cost scenarios described above, zero-information traders do not have an edge in this case. The noise alters the fundamentalists' trading decisions, thereby affecting the market price, which in turn affects the zero-information strategy. In effect, the zero-information traders indirectly receive the same unreliable information.
Figure 6. Evolutionary dynamics of a market with three information levels and different types of noise over information. Cost for information is zero.
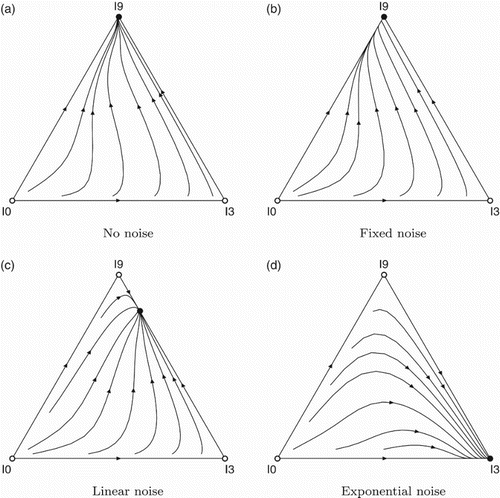
Finally, we again look at the situation where both cost and noise are present. shows the resulting dynamics. Linear noise and cost yield a situation where a mix of all three strategies survives in equilibrium. Moreover, there is an unstable mixed equilibrium of zero-information traders and averagely informed traders. The other combinations of cost and noise functions yield a mixed equilibrium where both zero-information and averagely informed traders prevail – in these cases, this mixed equilibrium is the main stable attractor. The presence of both cost and noise tips the scale in favour of the less-informed, as acquiring more information becomes too costly to pay off.
4.3. Evolutionary dynamics with mutation
So far we have only considered pure selection, as described by the replicator dynamics (Equation (Equation1(1)
(1) )). Here, we analyse the effect of mutation on the resulting market dynamics. Mutation can be thought of as random exploration by the trading agents, who now not only copy the strategy of successful peers, but try different strategies at random as well with some probability. A simple exploration strategy is ε-greedy, where agents choose their optimal action (selection) with probability
, and with probability ε they randomly choose any of their other actions (mutation). In our case we have three actions, yielding the mutation matrix
which is then used in the selection-mutation dynamics of Equation (Equation2
(2)
(2) ).
To show the effect of mutation on the resulting dynamics, we apply the selection-mutation model to the market scenario without cost and noise – note, however, that similar findings hold for the other scenarios as well. shows the market dynamics for different mutation rates ε. Clearly, mutation matters. As traders randomly choose their trading strategy with small probability, no information level dies out completely. The equilibrium moves to the interior of the simplex, where the full mix of trading strategies co-exists. Increasing the mutation rate strengthens this effect to the point where random mutation outweighs selection, and the strategy mix becomes uniform (e.g. see (d)). Also note that the three faces of the simplex (indicated with dashed lines in ) are repelling, and the pure equilibria disappear.
4.4. Discussion
The various simulations and results show a variety of outcomes depending on whether or not cost and noise are a driving factor of the market. Whereas insiders drive all other traders out of the market when no noise is present and costs are zero, the situation rapidly changes and other types of traders can prevail when different types of noise or cost are added. This shows that the J-curve that was presented in previous work (Hennes et al., Citation2012; Kirchler, Citation2010; Tóth et al., Citation2007) may be not so much a general rule as it is a special case. Moreover, we observe that adding a mutation (exploration) term alters the market dynamics as well. Mutation causes pure equilibria to disappear, in favour of a single interior equilibrium where all trading strategies co-exist. These findings show that a good understanding of the underlying dynamics are vital if any reasonable predictions about market outcomes are to be made.
5. Conclusion
Previous work has established the non-trivial relation between the amount of forecasting information available to a trader and his or her expected return. Specifically, it has been observed that averagely informed traders perform below market average and are outperformed by zero-information traders – only insiders prevail. We extend previous work by investigating the effect of noise or uncertainty with respect to forecasting information. Realistically, a longer forecast horizon may lead to higher uncertainty or noise. We observe that the presence of noise changes the market outcome in such a way that averagely informed traders may coexist with insiders, driving zero-information traders out of the market. Putting a price on information similarly changes the market outcome, this time yielding an equilibrium where each type of trader can prevail simultaneously. A combination of cost and noise may lead to a situation where additional information is too costly to obtain, driving insiders out of the market. Finally, we show the effect of exploration, where traders with small probability select a random trading strategy, alters the market dynamics in such a way that a mix of trading strategies is present in equilibrium.
As mentioned in the Introduction, real markets include not only fundamentalists, but technical analysts or chartists as well. A logical next step for future work is to include chartists in the market model as well. Moreover, it would be of interest to investigate whether the price signal resulting from the market model in equilibrium exhibits stylised facts found in real financial time series data (Aloud, Citation2013; Cont, Citation2001; Masry, Citation2013; Palit, Phelps, & Ng, Citation2012), and if so under which circumstances. This would further validate our approach.
Disclosure statement
No potential conflict of interest was reported by the authors.
Funding
This work was supported by the Netherlands Organisation for Scientific Research (NWO) [grant number 612.001.017].
References
- Aloud, M. (2013). Modeling the high-frequency FX market: An agent-based approach (PhD thesis). University of Essex.
- Angel, J. J. (2002). Market mechanics: A guide to U.S. stock markets (Technical report). The Nasdaq Stock Market Educational Foundation, Inc.
- Bajari, P., & Hortacsu, A. (2003). The winner's curse, reserve prices, and endogenous entry: Empirical insights from ebay auctions. RAND Journal of Economics, 34(2), 329–355. doi: 10.2307/1593721
- Barrot, C., Albers, S., Skiera, B., & Schäfers, B. (2010). Vickrey vs. ebay: Why second-price sealed-bid auctions lead to more realistic price-demand functions. International Journal of Electronic Commerce, 14(4), 7–38. doi: 10.2753/JEC1086-4415140401
- Cheng, H., Tan, P.-N., Gao, J., & Scripps, J. (2006). Multistep-ahead time series prediction. Proceedings of the 10th Pacific-Asia Conference on Advances in Knowledge Discovery and Data Mining, PAKDD'06, Berlin, Heidelberg: Springer-Verlag, pp. 765–774. ISBN 3-540-33206-5, 978-3-540-33206-0.
- Cont, R. (2001). Empirical properties of asset returns: Stylized facts and statistical issues. Quantitative Finance, 1(2), 223–236. doi: 10.1080/713665670
- Gehrig, T., & Menkhoff, L. (2006). Extended evidence on the use of technical analysis in foreign exchange. International Journal of Finance & Economics, 11(4), 327–338. doi: 10.1002/ijfe.301
- Gordon, M. J. (1962). The investment, financing, and valuation of the corporation: Irwin series in economics. Homewood, IL: Greenwood Press.
- Hennes, D., Bloembergen, D., Kaisers, M., Tuyls, K., & Parsons, S. (2012). Evolutionary advantage of foresight in markets. Proceedings of the Genetic and Evolutionary Computation Conference (GECCO 2012), pp. 943–950.
- Hofbauer, J., & Sigmund, K. (1998). Evolutionary games and population dynamics. Cambridge: Cambridge University Press.
- Huber, J. (2007). “J”-shaped returns to timing advantage in access to information – Experimental evidence and a tentative explanation. Journal of Economic Dynamics and Control, 31(8), 2536–2572. doi: 10.1016/j.jedc.2006.09.003
- Huber, J., Kirchler, M., & Sutter, M. (2008). Is more information always better? Experimental financial markets with cumulative information. Journal of Economic Behavior and Organization, 65(1), 86–104. doi: 10.1016/j.jebo.2005.05.012
- Kaisers, M., Tuyls, K., Parsons, S., & Thuijsman, F. (2009). An evolutionary model of multi-agent learning with a varying exploration rate. Proceedings of The 8th International Conference on Autonomous Agents and Multiagent Systems, Budapest, Hungary.
- Kirchler, M. (2010). Partial knowledge is a dangerous thing – On the value of asymmetric fundamental information in asset markets. Journal of Economic Psychology, 21, 643–658. doi: 10.1016/j.joep.2010.04.005
- Masry, S. (2013). Event-based microscopic analysis of the FX market (PhD thesis). University of Essex.
- McMillan, J. (1994). Selling spectrum rights. The Journal of Economic Perspectives, 8(3), 145–162. doi: 10.1257/jep.8.3.145
- Niu, J., Cai, K., Parsons, S., Fasli, M., & Yao, X. (2012). A grey-box approach to automated mechanism design. Electronic Commerce Research and Applications, 11(1), 24–35. doi: 10.1016/j.elerap.2011.06.006
- Palit, I., Phelps, S., & Ng, W. L. (2012). Can a zero-intelligence plus model explain the stylized facts of financial time series data? Proceedings of the 11th International Conference on Autonomous Agents and Multiagent Systems, Valencia, Spain, pp. 653–660.
- Phelps, S., Parsons, S., & McBurney, P. (2005). An evolutionary game-theoretic comparison of two double-auction market designs. In P. Faratin & J. A. Rodríguez-Aguilar (Eds.), Agent-mediated electronic commerce VI. Theories for and engineering of distributed mechanisms and systems (pp. 101–114). New York, NY: Springer.
- Shoham, Y., Powers, R., & Grenager, T. (2007). If multi-agent learning is the answer, what is the question? Artificial Intelligence, 171(7), 365–377. doi: 10.1016/j.artint.2006.02.006
- Taylor, M. P., & Allen, H. (1992). The use of technical analysis in the foreign exchange market. Journal of International Money and Finance, 11(3), 304–314. doi: 10.1016/0261-5606(92)90048-3
- Tóth, B., & Scalas, E. (2008). The value of information in financial markets: An agent-based simulation. In J. Huber & M. Hanke (Eds.), Information, interaction, and (in)efficiency in financial markets. (pp. 95–114). Vienna: Linde.
- Tóth, B., Scalas, E., Huber, J., & Kirchler, M. (2006). Agent-based simulation of a double-auction market with heterogeneously informed agents. Potentials of complexity science for business, governments, and the media, Budapest, Hungary.
- Tóth, B., Scalas, E., Huber, J., & Kirchler, M. (2007). The value of information in a multi-agent market model. European Physical Journal B, 55, 115–120. doi: 10.1140/epjb/e2007-00046-2
- Tuyls, K., & Parsons, S. (2007). What evolutionary game theory tells us about multiagent learning. Artificial Intelligence, 171(7), 406–416. doi: 10.1016/j.artint.2007.01.004
- Walsh, W. E., Das, R., Tesauro, G., & Kephart, J. O. (2002). Analyzing complex strategic interactions in multi-agent systems. AAAI-02 Workshop on game-theoretic and decision-theoretic agents, Edmonton, Alberta, Canada.