Abstract
Although normatively irrelevant to the relationship between a cue and an outcome, outcome density (i.e. its base-rate probability) affects people's estimation of causality. By what process causality is incorrectly estimated is of importance to an integrative theory of causal learning. A potential explanation may be that this happens because outcome density induces a judgement bias. An alternative explanation is explored here, following which the incorrect estimation of causality is grounded in the processing of cue–outcome information during learning. A first neural network simulation shows that, in the absence of a deep processing of cue information, cue–outcome relationships are acquired but causality is correctly estimated. The second simulation shows how an incorrect estimation of causality may emerge from the active processing of both cue and outcome information. In an experiment inspired by the simulations, the role of a deep processing of cue information was put to test. In addition to an outcome density manipulation, a shallow cue manipulation was introduced: cue information was either still displayed (concurrent) or no longer displayed (delayed) when outcome information was given. Behavioural and simulation results agree: the outcome-density effect was maximal in the concurrent condition. The results are discussed with respect to the extant explanations of the outcome-density effect within the causal learning framework.
1. Introduction
The ability to perceive, learn and use causal relationships correctly between events is essential for humans. The available evidence suggests that we are quite accurate in detecting causal relationships based on a number of signals, such as the covariation or contingency between causes and effects (e.g. Allan and Jenkins Citation1980; Chatlosh, Neunaber and Wasserman Citation1985; Shanks and Dickinson Citation1987; Wasserman Citation1990a). If, other things being equal, a given outcome tends to occur more often in the presence than in the absence of a cue, we tend to perceive a causal relation between those two events. However, on some occasions we fail to identify correctly a potential causal relationship or, alternatively, we incorrectly perceive a causal relationship in a situation where a cue and an outcome are statistically independent (e.g. Smedslund Citation1963; Jenkins and Ward Citation1965; Alloy and Abramson Citation1979; Allan and Jenkins Citation1983; Shanks Citation1985, Citation1987; Kao and Wasserman Citation1993; Matute Citation1996; Allan, Siegel and Tangen Citation2005; Crump, Hannah, Allan and Hord Citation2007; see also Crocker Citation1981). These situations where our cognitive system is misled by the available information provide a valuable opportunity to gain a deeper insight into the mental processes involved in causal induction. Not surprisingly, a great effort has been made to explore such biases and to investigate the cognitive mechanisms underlying them (for a review, see Shanks Citation2007).
The most widely used paradigm to study causal induction is a very simple one where information on the presence or absence of a cue and on the presence or absence of an outcome is given to the participants on a trial-by-trial basis (e.g. Kao and Wasserman Citation1993). In each trial the cue is either present (C) or absent (∼C), and the outcome either occurs (O) or does not occur (∼O). For instance, participants can be asked to imagine that they are doctors who have to decide whether or not a patient is allergic to a food based on the information about whether or not the patient has eaten that food (cue) on several days and whether or not the patient has developed an allergic reaction (outcome) on each of those days. This situation allows the researcher to expose participants to four types of trial (see ): trials in which the cue and the outcome co-occur (type a trials); trials in which the cue is present but the outcome is absent (type b trials); trials in which the cue is absent but the outcome is nevertheless present (type c trials); and trials in which both the cue and the outcome are simultaneously absent (type d trials). After participants have been exposed to a given number of each of these trials, they are asked to provide a subjective rating of the perceived strength of the cue–outcome relationship, usually by means of a numerical scale.
Table 1. A simple 2×2 matrix for cue–outcome pairings.
In general, it is assumed that participants’ judgements should be based on a statistically normative index of the objective cue–outcome contingency. The Δ P index is usually considered as an appropriate normative measure against which participants’ judgements can be contrasted (Allan Citation1980; Cheng and Novick Citation1992). This index, defined as the difference between the probability of the outcome given the cue, p(O|C), and the probability of the outcome given the absence of the cue, p(O|∼C), can be easily computed from the information about the frequency of each of the above-mentioned trial types:
Among the many factors that are known to bias causal judgements, one of the most interesting is outcome density: although participants’ estimations should be sensitive only to the contingency, it has been observed that the overall probability of the outcome p(O) usually has an effect on causal judgements. Specifically, for a given Δ P value, participants’ ratings tend to increase with an increase in p(O). This effect of the outcome density was documented extensively in the 1980s and 1990s (e.g. Alloy and Abramson Citation1979; Allan and Jenkins Citation1983; Shanks and Dickinson Citation1987; Matute Citation1995; Wasserman, Kao, van Hamme, Katagiri and Young Citation1996) and has recently received renewed attention (e.g. Buehner, Cheng and Clifford Citation2003; Allan et al. Citation2005; Crump et al. Citation2007; Allan, Hannah, Crump and Siegel Citation2008).
Faced with the observation of increased subjective ratings of the perceived strength of the cue–outcome relationship when the outcome density was increased but the contingency (i.e. Δ P) kept constant, many researchers resorted to associative learning models to explain those results (e.g. Shanks and Dickinson Citation1987). The extremely influential Rescorla and Wagner Citation(1972) model (hereafter, RW) accounts for those observations by assuming that, when the probability of the outcome is high, the likelihood of fortuitous co-occurrences of the cue and the outcome is high, even in non-contingent situations. This phenomenon of fortuitous co-occurrences of the cue and the outcome would lead to the formation of a spurious association between the mental representation of the cue and the mental representation of the outcome. However, this explanation may have its limitations.
First, the RW model exhibits only a pre-asymptotic outcome-density effect: the outcome-density effect is predicted only early on during training. By the time the learning asymptote had been reached, the outcome-density effect would have disappeared. This is easy to see with Δ P=0: the strength of the spurious association vanishes as training proceeds because with further training the number of cases in which the cue and outcome values (present or absent) are the same end up being equal to the number of cases in which those values are different (i.e. one and only one is present). Transposed to the experiments that yielded an outcome-density effect, an explanation in terms of the RW model would imply that the outcome-density effect would disappear with further training.
Second, the RW model is capable of producing only positive outcome-density effects (i.e. contingency overestimation as the outcome density increases), failing to produce negative outcome-density effects (i.e. contingency underestimation as the outcome density decreases). Accordingly, researchers inspired by this model have, most of the time (with noticeable exceptions, e.g. Shanks and Dickinson Citation1987), emphasised the results obtained in high-density conditions (i.e. an increase in contingency judgement with an increase of the density of the outcome).
With the accumulation of new experimental data, these limitations of applying the RW model to explain the outcome-density effect have become more conspicuous. More recent studies have indeed reported, for a situation of null contingency, persistent and even increasing positive (e.g. Allan et al. Citation2005) and negative (e.g. Allan et al. Citation2005; Crump et al. Citation2007; see also Shanks and Dickinson Citation1987) causal judgements for high and low values, respectively, of the density of the outcome. These results are contrary to the pre-asymptotic and the only-positive predictions of the RW model.
A very different model that could explain the outcome-density bias is the causal power theory of causal induction (Cheng Citation1997). This theory offers a conceptualisation of the connection between the observation of covariation and the deduction of a causal link that is very different from that of the RW model. For a single causal candidate (the simplest case, the one that will be discussed here), this theory proposes that people compute the causal power of the potential cause by adjusting Δ P for the base-rate of occurrence of the effect when the candidate cause is absent, P(O|∼C). Specifically, the model assumes that the generative causal power of a cue, p c, is computed following the equation
Moreover, in situations where Δ P=0, the model cannot account for the outcome-density effect, because in such a situation whatever the value of the denominator, pc is zero, as the numerator, Δ P, is zero. For the model to predict an increase in p c (and thus in the causal judgements) with an increase in outcome density, one would have to make the further assumption that participants might slightly misperceive a small positive contingency. This may be a sound assumption, but the model would then still not explain the phenomena it is supposed to explain. Indeed, one would still have to specify how and why participants misperceived a small positive contingency in a situation of Δ P=0. Finally, another shortcoming of the causal power model is that it is an asymptotic model, which means that its prediction concerns the final result of causal learning. Unlike the RW model, whose short-run dynamics are available and can be compared with those of human participants, the causal power model offers only one prediction, which is the final, asymptotic, predicted value.Footnote1
Recently, Allan and her collaborators (Allan et al. Citation2005, Citation2008; Crump et al. Citation2007) proposed a different explanation of the outcome-density effect. Their proposal is that manipulations of the density of the outcome do not affect the correct perception of a causal relationship but generate a response bias that would play at the time of making the causal judgement (i.e. higher and positive judgements with high outcome density; lower and even negative judgements with low outcome density). In support of their signal-detection theory (hereafter SDT) account, Allan et al. Citation(2008) showed that participants’ sensitivity towards the cue–outcome contingency (measured by the SDT parameter d′) is not affected by outcome density manipulations, whereas the response bias (measured by the SDT parameter β) does change depending on the manipulation of outcome density, with a liberal bias in the case of high outcome density and a conservative bias in the case of low outcome density. Before that, Crump et al. Citation(2007) made a similar point by showing that participants’ estimates of the frequency of occurrence of each cue–outcome pair were not affected by the manipulation of outcome density, whereas the latter influenced participants’ contingency ratings. In these papers, however, the experimental paradigm was totally different from the one discussed here. Interestingly, Allan et al. Citation(2005) used the same paradigm as the one discussed here, and the SDT parameters d′ and β they computed from the predictive yes/no responses that the participants made during training seem to support the same conclusion. This issue deserves to be addressed more thoroughly, so we leave it for the general discussion.
One of the purposes of this paper is to explore an alternative explanation according to which the incorrect estimation of causality is grounded in the processing of cue–outcome information during learning. In this sense our connectionist models are process models. In the simulations that are presented, a model comparison approach is adopted in order to test what assumptions are to be made under this account for the outcome density to influence the results. The general framework of the approach that is offered here bears a general similarity to the RW model, as it assumes that outcome density manipulation affects the encoding of the objective contingency during learning. However, our account is actually very different from that offered by the RW model, because it aims to encompass the findings of outcome-density effects that are persistent with extensive training, and those findings of negative causal judgements that the RW model does not predict. Our proposal is also different from that of Allan and her collaborators – actually it runs counter to some of their results, as we shall comment on in the final discussion. Indeed, it is proposed here that outcome density manipulation affects the encoding of the objective contingency at learning. In proposing that, we do not deny the possibility that there may be other biases at the time of making a causal judgement (as those biases are documented; see Crocker Citation(1981) for a review), but we test whether the extant results may be explained under the account that outcome density manipulation affects the encoding of the objective contingency during learning.
After delineating the conditions for producing the outcome-density effect within the framework considered here, we present a behavioural experiment inspired by simulation results.
2. Overview of the distributed neural network simulations
The simulations described below investigate what the simplest connectionist architecture should comprise in order to encompass the results obtained in humans when manipulating outcome density. Their general aim is to simulate a contingency estimation that is biased, as a function of outcome density, owing to the processing of cue–outcome information during learning.
A question of importance then is whether processing of the cue–outcome relationship is sufficient for the simulations to yield the outcome-density effect, or whether a deep processing of cue information is also required. Simulations will thus consider these possibilities in turn. The first simulation will test whether a manipulation of the outcome density alone – or, in neural networks terms, whether a manipulation of the structure of the outputs (representing the outcome) – is sufficient to produce the outcome-density effect. If this fails to produce the effect under examination, then another possibility is that for the effect to manifest itself a deep processing of cue information is required in addition to the joint processing of cues and outcomes. The second simulation will test for this possibility.
We opted for the use of distributed multilayered neural networks trained by a gradient descent learning procedure. However, it is not intended that other types of neural network would not be able to simulate the outcome-density effect. Within the general type of neural network considered here, each specific architecture corresponds to a different explanation of the outcome-density effect, and it is tested which architecture is able to simulate the extant behavioural data is tested.
The architectures used in both simulations are three-layer networks comprising an input layer that is fully forward-connected to a hidden layer, itself fully forward-connected to a hetero-association layer. Typically, a set of input–target pairs (the to-be-learned training base, corresponding to the trials that are presented in a behavioural experiment) is repeatedly presented to the network. At each presentation of a pair, all the network's connection weights are differentially modified so as to minimise an error function based on the error between the output activation actually computed by the network in response to the input, and the target provided for each pair. The usual backpropagation learning algorithm that minimises the cross-entropy cost function (Hinton Citation1989) is used to associate input to target patterns.
At the neural networks level, the difference between the architecture used in simulation 1 and that used in simulation 2 lies in the error that is backpropagated. The architecture used in simulation 1 is classically trained to associate each input to the correct hetero-associative output (e.g. associate cue information with its corresponding outcome information), so that the error includes the usual error between the hetero-association layer activation and the hetero-associative target (see . For simulation 2, the architecture is not only trained to associate each input to the correct hetero-associative output (as in simulation 1) but also to reproduce correctly each input (see . Thus, for simulation 2 the error that is backpropagated includes not only the usual error between the hetero-association layer activation and the hetero-associative target but also the error between the auto-association layer activation and the auto-associative target (the input pattern plays the role of an auto-associative target).
Figure 1. The networks used in the simulations: (a) simulation 1 used a hetero-associator; (b) simulation 2 used an auto-hetero-associator. Large arrows represent full connectivity with modifiable weights. See text for details.
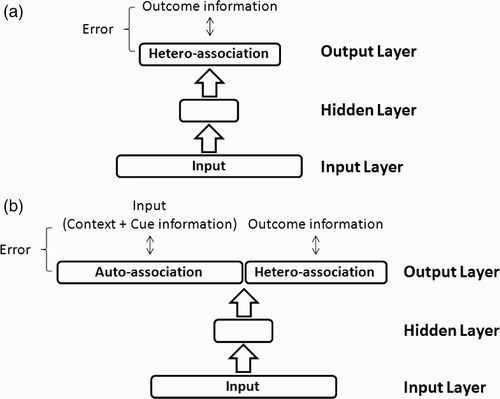
It is not intended here that these two architectures correspond strictly to known features of theories in the area of causal learning. Nor does the architecture of simulation 2 comprise an auto-associative part because such architectures generally perform better (this is particularly true for neural networks that process temporally ordered information, e.g. Maskara and Noetzel Citation(1992) and Ans, Rousset, French and Musca Citation(2004); see also Ans and Rousset Citation(2000), Ans et al. Citation(2004) and Musca, Rousset and Ans Citation(2009) for a potential explanation of the success of neural networks that incorporate an auto-associative layer). Actually, the two architectures are presented from a model comparison viewpoint according to the following reasoning.
The learning process in distributed multilayered neural networks trained by a gradient descent learning procedure such as the ones used here is guided solely by the errors made while trying to give the correct output (e.g. the presence or absence of the outcome) given the input at hand (Rumelhart, Hinton and Williams Citation1986; see also Bishop Citation1995). As noted before, the error that is backpropagated in simulation 1 is that between the hetero-association layer activation and the hetero-associative target, that is, between the activation of the output layer and the expected outcome pattern. Thus, if simulation 1 yields an effect of the manipulation of the outcome density, then this bias is truly an effect of how the manipulation of the outcome density affects the processing of cue–outcome information given to the network.
For simulation 2, on the other hand, the error that is backpropagated includes both the usual error between the hetero-association layer activation and the hetero-associative target (corresponding to outcome information) and the error between the auto-association layer activation and the auto-associative target (corresponding to cue information). For this reason, the connection weights of the architecture used in simulation 2 will be shaped by taking into account the constraints imposed by both cues and outcomes. This introduces no specific bias with respect to simulation 1, it only forces the network to encode the input space, that is, to process cue information thoroughly.
Thus, within the framework considered here, if simulation 1 fails to yield an effect of the manipulation of the outcome density but simulation 2 does yield the effect, one can say that the outcome-density effect may arise because of an incorrect encoding of the objective contingency during learning due to outcome density, but that a thorough processing of cue information is mandatory for this to occur.
2.1. Simulation 1
Does the manipulation of outcome density affect a neural network that simply processes the cue–outcome information in such a way that it would incorrectly encode the contingency and yield the expected outcome-density effect? For the reasons mentioned above, in order to answer this question one has to resort to a classical hetero-associator that associates cue information (as input) to outcome information (as output). Simulation 1 will thus explore the ability of this architecture to simulate the outcome-density effect.
Method
Stimuli and apparatus.
An important part of the modelling is the translation of the problem into neural networks language. This involves creating a training set, in other words choosing the input and output vectors and the way they are related one to another. Moreover, when doing this, one has to take into account that learning contradictory information, such as ‘cue leads to outcome’ in some trials and ‘cue does not lead to outcome’ in other trials, is always problematic. We dealt with this problem by assuming that each trial occurs in a different ‘context’. This context does not necessarily need to be understood as a temporal context or the like in humans (though some theories in psychology suppose explicitly the existence of a constantly evolving temporal context, such that every single trial in any learning situation can be thought of as occurring in a different temporal context (Howard and Kahana Citation2002; Sederberg, Howard and Kahana Citation2008)). It is just a means we used in order to allow the network to associate a given input sometimes with an output, sometimes with the opposite of that output. Adding context to the inputs allows for disambiguating two such otherwise incompatible trials and makes it possible for the network to converge to a solution. In order to take this important feature into account, it is necessary to introduce a representation of the context, so that ‘cue–outcome’ and ‘cue–no outcome’ pairings can both occur in the training set, but in different contexts. With these considerations in mind, it was decided to introduce into the simulations as many different contexts as training trials (i.e. no training exemplar has the same context as another training exemplar). At the neural network level this is not the most economical solution, but has the advantage of avoiding the introduction of a bias through the sharing of context between trials. Indeed, context vectors are all orthogonal so they do not influence learning of cue–outcome pairs, but only make it possible for the network to learn two otherwise incompatible trials, such as ‘cue present–outcome present’ and ‘cue present–outcome absent’.
The training base comprises 100 training exemplars. The input for each exemplar is a 102-component vector made of two parts. The first part is a 100-component context vector that contains only one 1 component and 99 0 components in such a way that the context vectors of all the training exemplars are orthogonal (i.e. a vertical concatenation of the context vectors yields a diagonal matrix). The second part of the input vector is a two-component vector that codes for the cue, with 1 0 being cue present and 0 1 being cue absent. The output vectors are two-component vectors that code for the outcome, with 1 0 being outcome present and 0 1 being outcome absent.
The dependent variable that was used, called perceived contingency, is computed according to the following reasoning. After training, connection weights are no longer changed and the network is probed without any context (i.e. with a context vector of 100 components of value zero, so that this part of the input does not contribute any activation to the network), because using a particular context already used during training would bias the results towards the particular training trial corresponding to that context. The activation of the first output node is recorded, once with the cue present input (i.e. 1 0) and once with the cue absent input (i.e. 0 1). The ‘perceived contingency’ for the first output node is the difference in activation (‘act’ in EquationEquation (3)) between the ‘cue present’ input case and the ‘cue absent’ input case. Inspired by the Δ P index (see EquationEquation (1)
), this index is computed as:
Design and procedure.
The covariational information given to the networks corresponds to a non-contingent situation (i.e. Δ P=0), with an outcome density that was varied (low: 40%; high: 60%). In terms of the frequency of each trial type (see ), the low outcome density condition corresponds to a=32, b=48, c=8, d=12, and the high outcome density condition corresponds to a=48, b=32, c=12, d=8.
Starting with random connection weights – uniformly sampled between -0.5 and 0.5 – the three-layer hetero-associator with 102 input units, 10 hidden units and two output units was trained with a learning rate of 0.1, a momentum of 0.7 and the activation of the bias cell set to unity. Fifty replications were run. For each replication the starting connection weights were identical for the two conditions that were contrasted (i.e. low versus high outcome density).
The dependent variable perceived contingency was computed at three points during training: after little training was given, which corresponds to a root mean squared error (RMSE) on the training set of 0.075, after reasonable training was given (RMSE = 0.01), and finally after extensive training (RMSE = 0.001).
Results
As the results depicted in show, be it with little or extensive training, the hetero-associative network failed to exhibit an outcome-density effect that would be positive for high outcome density and negative for low outcome density. Owing to a much higher variability being observed between the replications after little training (i.e. at a RMSE of 0.075) than after reasonable (RMSE = 0.01) and extensive (RMSE = 0.001) training, the results of the simulations are analysed in two steps.
Figure 2. Simulation results. Perceived contingency in a non-contingent situation as a function of the outcome density manipulation and of training: (a) by a hetero-associative neural network (simulation 1); (b) by an auto-hetero-associative neural network (simulation 2). Amount of training on the training base increases from left to right, with the more extensive the training, the lower the RMSE. Whiskers represent the limits of the 0.95 confidence interval. See text for details.
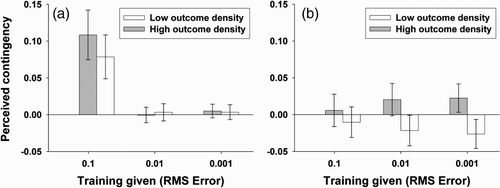
The first step is concerned with the results obtained after little training (RMSE = 0.075). At that point during learning the perceived contingency was significantly different from zero and positive for both the high, t(49)=6.400, p<0.0001, and the low, t(49)=5.247, p<0.0001, outcome density conditions. Moreover, the results are not different between these two conditions, t(49)=1.237, p=0.222. To sum up, at this first step a positive perceived contingency is obtained for both the high and the low outcome density conditions.
As the variances between the replications after reasonable (RMSE = 0.01) and extensive (RMSE = 0.001) training are similar, the second step of the analysis consists of an analysis of variance with the factors ‘density of the outcome’ (low versus high) and ‘training’ (reasonable versus extensive). This evidenced a main effect of training, F(1, 49)=4.762, p<0.05, with a higher perceived contingency after extensive learning (though never significantly different from zero; see , no main effect of the density of the outcome, F(1, 49)=0.025, p=0.876, and an interaction between these factorsFootnote2 that misses significance, F(1, 49)=3.388, p=0.072.
Importantly, with reasonable (RMSE = 0.01) and extensive (RMSE of 0.001) training the fact that the hetero-associative network failed to exhibit the expected outcome-density effect is confirmed by Student's t-tests comparing the perceived contingency at the two training times considered: the difference between the low and high outcome conditions is not significantly different, be it after reasonable, t(49)=0.576, p=0.567, or after extensive training, t(49)=0.355, p=0.724. Moreover, not only do the results not differ between the two outcome conditions, but also the perceived contingency is never statistically different from zero (see .
To summarise, with little training the perceived contingency was significantly different from zero and positive in both conditions. The positive perceived contingency in the low outcome density condition signs a failure of the network to simulate the phenomenon of negative perceived contingency that has been evidenced in humans. Reminiscent of the RW model, with little training a positive perceived contingency is obtained in the high outcome density condition, and this positive perceived contingency disappears with further training (see . This is not surprising, as the change of the strength of the association between a potential cue and a potential outcome after each learning trial is achieved in the RW model following an equation that Sutton and Barto Citation(1981) have shown to be formally equivalent to the delta rule (Widrow and Hoff Citation1960) used to train two-layer distributed neural networks. In other words, the results of the simulation with the present architecture are a pre-asymptotic and only positive perceived contingency that fade away with further training.
In conclusion, the manipulation of outcome density does not affect the encoding of cue–information pairs in a network that simply processes the cue–outcome information. Such a network, though it learns the cue–information pairs, does not yield the expected outcome-density effects.
2.2. Simulation 2
Simulation 2 was conducted with an auto-hetero-associator, an architecture that is similar to the architecture used in simulation 1, except that it also induces an active processing of the cue information. As discussed above, in this architecture both cues and outcomes are deeply processed and have the power to influence the way the learning of cue–outcome pairs occurs.
Method
Except for the use of an auto-hetero-associator neural network instead of a hetero-associator, all stimuli, apparatus, design and procedure details are the same as in simulation 1.
Results
As the variability observed between the replications after little (i.e. at a RMSE of 0.075), reasonable (RMSE = 0.01) and extensive (RMSE = 0.001) training was very similar, the results of the simulations were analysed through an analysis of variance. This analysis, with the factors ‘density of the outcome’ (low versus high) and ‘training’ (little, reasonable, extensive) evidenced a main effect of the density of the outcome, F(1, 49)=15.662, p<0.00025, with positive perceived contingencies in the high outcome density condition and negative perceived contingencies in the low outcome density condition. As these opposed perceived contingencies cancel each other at each training time considered (see , the main effect of training is not significant, F(2, 98)=0.023, p=0.978. The significant interaction between the two factors, F(2, 98)=3.203, p<0.05, shows that training is indeed responsible for the apparition of an effect of the density of the outcome, with a perceived contingency that becomes more and more positive as training proceeds in the high outcome density condition, and more and more negative as training proceeds in the low outcome density condition.
Further analyses showed that the difference in the perceived contingency between the two outcome conditions was not present early on during training (RMSE = 0.075), t(49)=1.281, p=0.206, but appeared after reasonable training (RMSE = 0.01), t(49)=3.456, p<0.002. Interestingly, after extensive training (RMSE = 0.001), not only was the perceived contingency different between the two outcome conditions, t(49)=4.382, p<0.0001, but it was also significantly different from zero in both conditions, t(49)=2.633, p<0.011 (low density condition) and t(49)=2.317, p<0.025 (high density condition), thus yielding negative and respectively positive illusory correlations.
Taken together, these results suggest that this architecture is perfectly able to simulate the outcome-density effect with all its features that posed a problem in simulation 1. The current simulation yields a positive perceived contingency in the high outcome density condition and a negative perceived contingency in the low outcome density condition. Moreover, these effects need some training to arise, but become stronger and stronger as training proceeds. As discussed in the Introduction, all these features are consistent with the recent human data that challenge an interpretation in terms of many extant models.
Within the context of our proposal, that is, the idea that contingency estimation could be influenced by an outcome density manipulation because of an incorrect encoding of the objective contingency during learning, these results plead for a necessary role of the processing of both cue and outcome information for an outcome-density effect to appear.
3. Experiment
Taken together, the two simulations suggest that the deep processing of cue information plays an important role during the acquisition of cue–outcome information in order to produce the outcome-density effect. The experiment that follows was designed to test empirically how the joint processing of cue and outcome information influences the outcome-density effect. The experiment is inspired by the simulations in that it aims to test the hypothesis that the outcome-density effect would be maximal in a situation where the joint processing of cue and outcome information is made possible. Such a situation, which we call hereafter concurrent condition, would correspond to that of simulation 2. However, there is no obvious way to design an experimental condition that would correspond strictly to the situation of simulation 1, as it is impossible for humans to process the relationship between cue information and outcome information while not processing the cues. Therefore, the experimental condition that will be opposed to the concurrent condition is one where the joint processing of cue and outcome information is experimentally put at a slight disadvantage, the delayed condition. As this disadvantage should not prevent learning of the cue–outcome information, the manipulation that we introduce is shallow. Therefore, it is not expected that the manipulation introduced in the delayed condition abolishes completely the outcome-density effect. However, if our hypothesis is correct, the outcome-density effect should be significantly reduced in the delayed condition as compared with the concurrent condition.
The experimental manipulation that we chose leaves unchanged the contingency (Δ P), the cue density, p(O| C) and p(O|∼C). The only difference it introduces is that for some participants cue information is still on the screen when outcome information is presented (concurrent condition) whereas for the other participants cue information is cleared from the screen before outcome information is presented (delayed condition). Both ways of presentation of cues and outcomes that define the two cue–outcome processing conditions are very common in conditioning and associative learning research.
Method
Participants, stimuli and apparatus
One hundred and twenty anonymous Internet users voluntarily took part and were randomly assigned to one of the four groups. There were 60 participants in the high outcome density (hereafter High) condition and 60 participants in the low outcome density (hereafter Low) condition. Out of the 60 participants of each condition, 29 participants were randomly assigned to the concurrent condition and the remaining 31 to the delayed condition.
The experimental programme used is an adaptation of the allergy task that has been used extensively in contingency learning experiments (e.g. Wasserman Citation1990b). The experiment was run on the Internet, implemented as an HTML document dynamically modified with JavaScript that any computer connected to the World Wide Web with a standard Internet browser can run. Previous experiments conducted with this task show that the results obtained over the Internet are virtually identical to those obtained under traditional laboratory conditions (e.g. Vadillo and Matute Citation2007).
Design and procedure
Participants were exposed to a sequence of 50 trials. Outcome density was manipulated between participants. For both experimental conditions (High and Low), cue density was kept constant at 0.8. As , the contingency was null (Δ P=0). In the High condition the outcome density was 0.8, with 32 a, eight b, eight c and two d trials, whereas in the Low condition the outcome density was 0.2, with eight a, 32 b, two c and eight d trials. To avoid any order-of-trials bias, roughly half of the participants of each condition and group performed the task with a different pseudorandom sequence of trials (with the constraint of no more than three consecutive trials of the same type).
The cover story was the following. A space alien from Mars was offered carrots, which it ate (C) or did not eat (∼C), and then the Martian felt sick (O) or did not feel sick (∼O). A translation from Spanish of the initial instructions given to participants reads as follows:
Imagine you are a scientist taking part in an investigation on a recently discovered alien from Mars. Apparently a protein that can only be found in carrots is very important, but we do not know if the Martian should eat carrots or should avoid eating carrots. Thus, your mission is to investigate how carrots agree with the Martian. To do that, you will be presented with a series of cards with information from a recent report. On each card you will first see whether the Martian ate or did not eat carrots, and you will be asked to indicate whether you think the carrots agreed with the Martian or not. To help you improve your knowledge of the Martian, upon your answer you will see the outcome of the eating/no eating of carrots by the Martian. Use the information on these cards to learn how the carrots agree with the Martian.
On completion of the training trials, participants were presented with the causal question, which read ‘Is eating carrots the cause of the Martian being sick?’ Below the question a 100-point scale ranging from zero to 100 was displayed, with zero labelled ‘Clearly NO’ and 100 labelled ‘Clearly YES’. Participants could answer the question through a click on the scale. On clicking, the value corresponding to their answer was displayed and remained visible. Participants had the opportunity to correct their answer as many times as they wanted.
Results and discussion
Planned comparisons showed an effect of the outcome density manipulation on causal judgements both in the concurrent condition, F(1, 116)=30.724, p<0.0001 and in the delayed condition, F(1, 116)=7.251, p<0.01. More importantly, however, an interaction was obtained between the factors cue–outcome processing (concurrent versus delayed) and outcome density (low versus high), F(1, 116)=4.461, p<0.05, with a stronger effect of the outcome density manipulation in the concurrent condition (see ). This interaction shows that, as expected, the effect of the manipulation of the outcome density depends on the deep processing of cue information: The outcome-density effect is largest in a situation where the joint processing of cue and outcome information is possible, and weakened when a mere delay is introduced between the processing of the cue and that of the outcome. Thus, as predicted by the simulations, the outcome-density effect is maximal in a situation where cue processing and outcome processing occur concurrently.
4. General discussion
Perceiving contingency between potential causes and outcomes is of crucial importance in order to understand, predict and control our environment. While generally humans’ covariation judgements correspond to the objective covariation to which they are exposed (e.g. Shanks Citation2004), there are many factors that are known to bias these judgements (for a review, see Allan Citation1993; López, Cobos, Caño and Shanks Citation1998). As with many other cognitive phenomena, one way to gain a better understanding in order to develop an integrative theory of humans’ ability to make causal judgements is to find variables that affect it in a systematic way (such as the outcome density manipulation), and explain their action.
In this paper, we have explored the outcome-density effect, an effect whereby the outcome base-rate unduly influences contingency estimation, in order to increase the understanding of its possible conditions of manifestation. We started with a brief review of the available explanations of this effect, and highlighted their successes and failures. The RW model (Rescorla and Wagner Citation1972) is a process model that explains the outcome-density effect by pre-asymptotic fortuitous co-occurrence of cue and outcomes. It thus predicts that the outcome-density effect should wear off with training. It yields (though only pre-asymptotically) the outcome-density effect in a non-contingent situation (i.e. Δ P=0), but is unable to simulate negative outcome-density effects under conditions of low outcome density, and outcome-density effects that persist with training, though these results have been obtained in humans. The causal power model (Cheng Citation1997) is an asymptotic rational model that can explain positive outcome-density effects, but makes only one, asymptotic prediction, thus it lacks short-run prediction ability. Also, it does not predict negative outcome-density effects or any outcome-density effect in a non-contingent situation (i.e. Δ P=0). Finally, the explanation proposed by Allan and her collaborators (Allan et al. Citation2005, Citation2008; Crump et al. Citation2007) is that outcome density induces a response bias at the time of making the contingency estimation, so the higher (lower) the outcome density, the more liberal (conservative) the decision, so the higher (lower) the contingency estimate. As their proposal is a general one derived from the signal-detection theory, it applies to all values of Δ P (including zero) and has the potential to explain outcome-density data sets that pose a problem to the other theories. However, just as in the signal-detection theory, this account does not explain the mechanisms whereby learning influences how people set the decision criterion (from more or less liberal to neutral to more or less conservative), or how the acquisition of the information takes place.
The account that we propose here is based on neural network process models. It shows that under some conditions a bias in contingency estimation can arise because contingency is incorrectly encoded during training owing to the outcome density manipulation. First, a comparison of two distributed neural network simulations was presented. It showed that an effect of the manipulation of the density of the outcome on the perceived contingency is not obtained in an architecture that is sensitive only to outcome density (simulation 1), although this architecture correctly learned the cue–outcome information. On the contrary, an architecture whose learning is affected by the processing of both cue and outcome information (simulation 2) did indeed yield different results as a function of the outcome density while correctly learning the cue–outcome information.
Second, a behavioural experiment was presented. Inspired by the simulations, it was based on the idea that the outcome-density effect will be maximal in a situation where the joint processing of cue and outcome information is possible. A shallow manipulation of cue information that leaves unchanged all the critical parameters, i.e. the contingency (Δ P), cue density, p(O|C) and p(O|∼C), was introduced by manipulating in each and every trial whether cue and outcome information could be processed jointly: cue information was concurrent (concurrent condition) or was not concurrent (delayed condition) with outcome information. As expected, different results were obtained as a function of the outcome density in both cue display conditions (i.e. concurrent and delayed). Crucially, the difference in causal judgements between the two outcome density groups was reliably higher in the concurrent condition. This result is consistent with the results of the simulations.
We must acknowledge that the proposal for a potential explanation of the outcome-density effect articulated here is at odds with some human data sets. Indeed, whereas our proposal is that the contingency estimation can be biased (by a manipulation of outcome density) because the contingency is incorrectly encoded during learning, Allan and her collaborators (Allan et al. Citation2005, Citation2008; Crump et al. Citation2007) argued that manipulations of outcome density do not affect the correct encoding of contingency during learning but do induce a response bias at the time people estimate contingency. In support of their claim, Allan et al. Citation(2008) presented SDT analyses of contingency learning data that show that participants’ sensitivity towards the cue-outcome contingency (measured by the SDT parameter d′) is not affected by outcome density manipulations, whereas the response bias (measured by the SDT parameter β) does change depending on the manipulation of outcome density, with a liberal (conservative) bias in the case of high (low) outcome density.
In line with what Allan et al. Citation(2008) argue, however, we think that the SDT approach to contingency learning/assessment is poorly fitted to the standard paradigm that has been used the most up to now, including in the experiment we have presented here as well as in Allan et al. Citation(2005). The main reason is that with this paradigm, yes/no predictive responses that participants make during training are used to infer the values of SDT parameters d′ and β that correspond to what happens after training. In the words of Allan et al. (Citation2008, p. 228), ‘the discrete-trial contingency task is poorly suited for a SDT approach. Many cue–outcome presentations must be provided to the participant to ensure that sufficient information is given about the actual contingency [before computing d′ and β ]’. Moreover, again in the words of Allan et al. (Citation2008, pp. 227–228), ‘the application of a SDT analysis to the trial prediction responses is indirect in that it assumes that the prediction of the outcome on C and on ∼ C trials reflects the participant's assessment of the contingency on those trials’ (Allan et al. Citation2008, p. 228; see also Winman and Gredebäck Citation2006). Finally, within the discrete-trial paradigm, the only data that support the claim made by Allan et al. Citation(2005) come from the study of these authors, later criticised by Allan et al. (Citation2008, p. 228) on the grounds that responses were averaged over participants: ‘the pitfalls of estimating parameters and evaluating models on the basis of averaged data are well known (Wickens 2002)’. Given these known pieces of criticism against the application of a SDT analysis to the discrete-trial paradigm, we think that it has not yet been proven convincingly that in the discrete-trial paradigm (the one we used here) the manipulation of outcome density does not affect the correct encoding of contingency during learning. With this in mind, we think that the potential explanation that we offer for the outcome-density effect deserves consideration. It may be limited to the discrete-trial paradigm – though this is barely a limitation, given the extensive use that is being made of this paradigm. Importantly, we prefer to think of our explanation as a complementary explanation and not one that challenges the bias explanation proposed by Allan and her collaborators (it is undeniable that at least under some conditions biases occur at the decision stage). We acknowledge that the problems that plague the application of a SDT analysis to the discrete-trial paradigm are eliminated (or at least drastically reduced) with the application of the SDT made by Allan et al. Citation(2008) within the ‘streamed-trial’ paradigm presented in Crump et al. Citation(2007). Indeed, within this paradigm the application of the SDT is sensible because SDT parameters are computed after all information has been presented to the participants. Converging evidence within the framework of this paradigm comes from Crump et al. Citation(2007), who showed, without relying on a SDT analysis, that participants’ estimates of the frequency with which each cue–outcome pair occurred within a streamed trial were not affected by the manipulation of outcome density, while the latter influenced their contingency ratings.
The work presented here points to a complementary explanation: it is proposed that the outcome density manipulation can exert its action at the learning stage. Importantly, our work shows that deep processing of the cue enhances the outcome-density effect, a result that, to the best of our knowledge, none of the available models that we reviewed predicts. It is our hope that the simulation and experimental work presented here will inspire further experimental work that will contribute to increasing the understanding of causal learning in humans.
Acknowledgements
Support for this research was provided by Dirección General de Investigación of the Spanish Government (grant SEJ2007-63691/PSIC), Departamento de Educación, Universidades e Investigación of the Basque Government (grant PI2008-9), and Consejería de Innovación, Ciencia y Empresa of the Junta de Andalucía (grant P08-SEJ-03586). Fernando Blanco was supported by a post-doctoral fellowship granted by Departamento de Educación, Universidades e Investigación of the Basque Government (BFI08120.0). We thank Amanda Sharkey, Matt Crump and an anonymous reviewer for their suggestions that helped us improve the quality of the paper.
Notes
As an anonymous reviewer pointed out, comparing the RW model with the causal power model is not necessarily warranted, because these explanations are offered at different levels of description (the former being a process model, the latter an asymptotic model). However, while the causal power model should only be compared with another asymptotic model (e.g. Δ P), and the RW model to another process model (e.g. those proposed here), these two models have often been contrasted in the causal learning literature. For completeness sake, we must mention the very different model discussed in Danks, Griffiths and Tenenbaum Citation(2003). It is a dynamical model that uses the ‘noisy-OR/AND-NOT’ parameterisation (instead of the ‘sum of occurring strengths’ parameterisation used by the RW model). This model has the same benefits and drawbacks as the RW model while acting as a ‘causal power estimator’ that can accommodate the short-run deviances from zero when the causal power is zero. However, ‘the iterative algorithm [this model is based on] can only be applied if one knows whether each potential cause is potentially generative or preventive’ (Danks et al. Citation2003, p. 72).
We have no further comment on this potential interaction because it does not make much sense, as it is due to an increase in perceived contingency in the low outcome density condition between reasonable and extensive training that is higher than the one observed in the high outcome density condition.
References
- Allan , L. G. 1980 . A Note on Measurement of Contingency between Two Binary Variables in Judgement Tasks . Bulletin of the Psychonomic Society , 15 : 147 – 149 .
- Allan , L. G. 1993 . Human Contingency Judgments: Rule-based or Associative?’ . Psychological Bulletin , 114 : 435 – 448 .
- Allan , L. G. , Hannah , S. D. , Crump , J. C. and Siegel , S. 2008 . The Psychophysics of Contingency Assessment . Journal of Experimental Psychology: General , 137 : 226 – 243 .
- Allan , L. G. and Jenkins , H. M. 1980 . The Judgment of Contingency and the Nature of the Response Alternatives . Canadian Journal of Psychology , 34 : 1 – 11 .
- Allan , L. G. and Jenkins , H. M. 1983 . The Effect of Representations of Binary Variables on Judgment of Influence . Learning and Motivation , 14 : 381 – 405 .
- Allan , L. G. , Siegel , S. and Tangen , J. M. 2005 . A Signal Detection Analysis of Contingency Data . Learning & Behavior , 33 : 250 – 263 .
- Alloy , L. B. and Abramson , L. Y. 1979 . Judgements of Contingency in Depressed and Nondepressed Students: Sadder but Wiser?’ . Journal of Experimental Psychology: General , 108 : 441 – 485 .
- Ans , B. and Rousset , S. 2000 . Neural Networks with a Self-refreshing Memory: Knowledge Transfer in Sequential Learning Tasks without Catastrophic Forgetting . Connection Science , 12 : 1 – 19 .
- Ans , B. , Rousset , S. , French , R. M. and Musca , S. C. 2004 . Self-refreshing Memory in Artificial Neural Networks: Learning Temporal Sequences without Catastrophic Forgetting . Connection Science , 16 : 71 – 99 .
- Bishop , C. M. 1995 . Neural Networks for Pattern Recognition , Oxford : Oxford University Press .
- Buehner , M. J. , Cheng , P. W. and Clifford , D. 2003 . From Covariation to Causation: A Test of the Assumption of Causal Power . Journal of Experimental Psychology: Learning, Memory, and Cognition , 29 : 1119 – 1140 .
- Chatlosh , D. L. , Neunaber , D. J. and Wasserman , E. A. 1985 . Response–Outcome Contingency: Behavioral and Judgmental Effects of Appetitive and Aversive Outcomes with College Students . Learning and Motivation , 16 : 1 – 34 .
- Cheng , P. W. 1997 . From Covariation to Causation: A Causal Power Theory . Psychological Review , 104 : 367 – 405 .
- Cheng , P. W. and Novick , L. R. 1992 . Covariation in Natural Causal Induction . Psychological Review , 99 : 365 – 382 .
- Crocker , J. 1981 . Judgment of Covariation by Social Perceivers . Psychological Bulletin , 90 : 272 – 279 .
- Crump , M. J.C. , Hannah , S. D. , Allan , L. G. and Hord , L. K. 2007 . Contingency Judgements on the Fly . Quarterly Journal of Experimental Psychology , 60 : 753 – 761 .
- Danks , D. , Griffiths , T. L. and Tenenbaum , J. B. 2003 . “ Dynamical Causal Learning ” . In Advances in Neural Information Processing Systems 15 , Edited by: Becker , S. , Thrun , S. and Obermayer , K. 67 – 74 . Cambridge, MA : MIT Press .
- Hinton , G. E. 1989 . Connectionist Learning Procedures . Artificial Intelligence , 40 : 185 – 234 .
- Howard , M. W. and Kahana , M. J. 2002 . A Distributed Representation of Temporal Context . Journal of Mathematical Psychology , 46 : 269 – 299 .
- Jenkins , H. M. and Ward , W. C. 1965 . Judgement of Contingency between Responses and Outcomes . Psychological Monographs , 79 : 1 – 17 .
- Kao , S.-F. and Wasserman , E. A. 1993 . Assessment of an Information Integration Account of Contingency Judgment with Examination of Subjective Cell Importance and Method of Information Presentation . Journal of Experimental Psychology: Learning, Memory, & Cognition , 19 : 1363 – 1386 .
- López , F. J. , Cobos , P. L. , Caño , A. and Shanks , D. R. 1998 . “ The Rational Analysis of Human Causal and Probability Judgment ” . In Rational Models of Cognition , Edited by: Oaksford , M. and Chater , N. 314 – 352 . Oxford : Oxford University Press .
- Maskara , A. and Noetzel , A. Forced Simple Recurrent Neural Network and Grammatical Inference . Proceedings of the 14th Annual Conference of the Cognitive Science Society . pp. 420 – 425 . Hillsdale, NJ : Lawrence Erlbaum .
- Matute , H. 1995 . Human Reactions to Uncontrollable Outcomes: Further Evidence for Superstitions rather than Helplessness . Quarterly Journal of Experimental Psychology , 48 : 142 – 157 . Ser. B
- Matute , H. 1996 . Illusion of Control: Detecting Response–Outcome Independence in Analytic but not in Naturalistic Conditions . Psychological Science , 7 : 289 – 293 .
- Musca , S. C. , Rousset , S and Ans , B. 2009 . Artificial Neural Networks Whispering to the Brain: Nonlinear System Attractors Induce Familiarity with never seen Items . Connection Science , 21 : 359 – 377 .
- Rescorla , R. A. and Wagner , A. R. 1972 . “ A Theory of Pavlovian Conditioning: Variations in the Effectiveness of Reinforcement and Nonreinforcement ” . In Classical Conditioning II: Current Research and Theory , Edited by: Black , A. H. and Prokasy , W. F. 64 – 99 . New York : Appelton-Century-Crofts .
- Rumelhart , D. E. , Hinton , G. E. and Williams , R. J. 1986 . “ Learning Internal Representations by Error Propagation ” . In Parallel Distributed Processing: Explorations in the Microstructure of Cognition , Edited by: Rumelhart , D. E. , McClelland , J. L. and The PDP Research Group . 318 – 362 . Cambridge, MA : MIT Press .
- Sederberg , P. S. , Howard , M. W. and Kahana , M. J. 2008 . A Context-based Theory of Recency and Contiguity in Free Recall . Psychological Review , 115 : 893 – 912 .
- Shanks , D. R. 1985 . Continuous Monitoring of Human Contingency Judgment Across Trials . Memory & Cognition , 13 : 158 – 167 .
- Shanks , D. R. 1987 . Acquisition Functions in Contingency Judgment . Learning and Motivation , 18 : 147 – 166 .
- Shanks , D. R. 2004 . “ Judging Covariation and Causation ” . In Handbook of Judgment and Decision Making , Edited by: Koehler , D. J. and Harvey , N. 220 – 239 . Oxford : Blackwell .
- Shanks , D. R. 2007 . Associationism and Cognition: Human Contingency Learning at 25 . Quarterly Journal of Experimental Psychology , 60 : 291 – 309 .
- Shanks , D. R. and Dickinson , A. 1987 . “ Associative Accounts of Causality Judgment ” . In The Psychology of Learning and Motivation , Edited by: Bower , G. H. Vol. 21 , 229 – 261 . San Diego, CA : Academic Press .
- Smedslund , J. 1963 . The Concept of Correlation in Adults . Scandinavian Journal of Psychology , 4 : 165 – 173 .
- Sutton , R. S. and Barto , A. G. 1981 . Toward a Modern Theory of Adaptive Networks: Expectation and Prediction . Psychological Review , 88 : 135 – 170 .
- Vadillo , M. A. and Matute , H. 2007 . Predictions and Causal Estimations are not Supported by the Same Associative Structure . Quarterly Journal of Experimental Psychology , 60 : 433 – 447 .
- Wasserman , E. A. 1990a . “ Detecting Response–Outcome Relations: Toward an Understanding of the Causal Texture of the Environment ” . In The Psychology of Learning and Motivation , Edited by: Bower , G. H. Vol. 26 , 27 – 82 . San Diego, CA : Academic Press .
- Wasserman , E. A. 1990b . Attribution of Causality to Common and Distinctive Elements of Compound Stimuli . Psychological Science , 1 : 298 – 302 .
- Wasserman , E. A. , Kao , S.-F. , van Hamme , L. J. , Katagiri , M. and Young , M. E. 1996 . “ Causation and Association ” . In The Psychology of Learning and Motivation, Vol. 34: Causal Learning , Edited by: Shanks , D. R. , Holyoak , K. J. and Medin , D. L. 207 – 264 . San Diego, CA : Academic Press .
- Widrow , G. and Hoff , M. E. 1960 . Adaptive Switching Circuits. 1960 IRE WESCON Convention Record , 96 – 104 . NewYork : Institute of Radio Engineers .
- Winman , A. and Gredebäck , G. 2006 . Inferring Causality Assessments from Predictive Responses: Cue Interaction without Cue Competition . Quarterly Journal of Experimental Psychology , 59 : 28 – 37 .