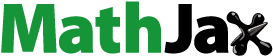
ABSTRACT
Two kinds of design ideation process may be distinguished in terms of the problems addressed: (i) solution-focused, i.e. generating solutions to address a fixed problem specifying a desired output; and (ii) exploratory, i.e. considering different interpretations of an open-ended problem and generating associated solutions. Existing systematic analysis approaches focus on the former; the literature is lacking such an approach for the latter. In this paper, we provide a means to systematically analyse exploratory ideation for the first time through a new approach: Analysis of Exploratory Design Ideation (AEDI). AEDI involves: (1) open-ended ideation tasks; (2) coding of explored problems and solutions from sketches; and (3) evaluating ideation performance based on coding. We applied AEDI to 812 concept sketches from 19 open-ended tasks completed during a neuroimaging study of 30 professional product design engineers. Results demonstrate that the approach provides: (i) consistent tasks that stimulate problem exploration; (ii) a reliable means of coding explored problems and solutions; and (iii) an appropriate way to rank/compare designers’ performance. AEDI enables the benefits of systematic analysis (e.g. greater comparability, replicability, and efficiency) to be realised in exploratory ideation research, and studies using open-ended problems more generally. Future improvements include increasing coding validity and reliability.
1. Introduction
Ideation is a salient area of interest for design researchers, and a considerable body of work now exists on the topic. In order to analyse design ideation empirically, we need two key elements: (1) design tasks that stimulate the kind of ideation processes we wish to investigate; and (2) a suitable procedure for analysing the processes. In this respect, a major approach for analysing ideation in design is the systematic, output-based approach originating in the work of Shah, Smith, and Vargas-Hernandez (Citation2003). This focuses on what may be termed solution-focused ideation – that is, ideation involving the generation of different solutions to a fixed problem, which is stimulated under experimental conditions using tasks that convey an output to be produced (e.g. ‘design a vehicle for moving people’) and functional requirements to be met (e.g. contain people and propel people). The ideation process is analysed using performance metrics, which quantify the extent to which the output solutions proposed by each designer (e.g. a car, a helicopter, and a teleporter) constitute new and different ways of addressing the requirements.
The systematic approach originating from Shah, Smith, and Vargas-Hernandez (Citation2003) is perceived to have benefits over other kinds of output-based ideation assessment, e.g. expert ratings. For instance, its methodical, consistent procedures may produce more comparable and replicable results (Shah, Smith, and Vargas-Hernandez Citation2003). It may also be more efficient in the context of larger samples (Sluis-Thiescheffer et al. Citation2016), which are important for the generalisability of results. However, a limitation is that the approach does not deal with the open-ended problems that are frequently encountered in design (Sosa Citation2018). Here, the nature of both the output to be produced and the specific requirements are unclear – e.g. ‘reduce food waste in the home.’ Rather than generating solutions to a fixed problem, the designer explores different ways of interpreting the open-ended problem and generates solutions (S) to address each of these problem interpretations (P). For instance: a waste monitoring system (S) to discourage people from creating waste (P); a composting system (S) to facilitate waste recycling (P); and single-portion food packaging (S) to discourage excessive food purchases (P). This may be termed exploratory ideation, and is particularly associated with the early, creative stages of conceptual design (Dorst and Cross Citation2001; Murray et al. Citation2019) where the problem tends to be fuzzy and abstract (Gero and McNeill Citation1998; Suwa, Gero, and Purcell Citation2000).
The solution-focused systematic approach outlined above does not accommodate and account for the exploration of different problem interpretations in addition to the generation of solutions. To enable the benefits of systematic analysis to be realised in studies on exploratory ideation and open-ended problems, a new approach that meets these requirements is needed. In this paper, we provide a means to systematically analyse exploratory ideation for the first time by presenting such an approach: Analysis of Exploratory Design Ideation (AEDI). AEDI fundamentally differs from existing solution-focused approaches (e.g. Shah, Smith, and Vargas-Hernandez (Citation2003) and derivatives) in two ways: (i) the use of tasks focusing on open-ended design problems as stimuli; and (ii) the analysis of ideation in terms of explored problem interpretations and solutions rather than just the latter alone. The approach consists of three key components, illustrated in Figure :
Open-ended design tasks. Designers are asked to generate concepts in response to open-ended problems, which encourage the exploration of different interpretations when proposing solutions. Data on generated concepts are gathered in the form of annotated sketches.
Identification of explored problems and solutions. Concept sketches are systematically classified using a qualitative coding process to identify the problem interpretations considered and corresponding solutions proposed by each designer during ideation.
Evaluation of exploratory ideation performance. Each designer’s performance is quantified in terms of: (i) how many different problem interpretations they considered (breadth of exploration); and (ii) the extent to which the solutions they generated are new compared to the solutions produced by others designers in the study (solution novelty).
We developed and applied AEDI to analyse ideation in a neuroimaging study of 30 professional product design engineers, who produced a sample of 812 concept sketches in response to 19 open-ended ideation tasks. The results (Section 4) demonstrate that the approach provides: (i) a consistent set of tasks for studying exploratory ideation, which stimulate problem exploration alongside solution generation; (ii) a reliable systematic process for interpreting problems and solutions from concept sketches via coding schemes; and (iii) an appropriate way to rank and compare designers based on exploratory ideation performance. As discussed in Section 5, two future improvements to be made are: (1) assessing the reliability of coding scheme development as well as application; and (2) finding efficient and effective ways to ensure that the coding is valid with respect to the intentions of the designers under study. That is, representative of the problems and solutions actually explored during ideation.
The remainder of the paper is organised as follows. In Section 2, we explore the scope of existing systematic ideation analysis approaches and highlight the need for a new approach focusing on exploratory ideation. In Section 3 we provide details on the neuroimaging study from which the AEDI approach originated. Section 4 outlines the elements of the approach in detail, and presents the results obtained from applying it in our study. Key observations and future work are discussed in Section 5, and the paper concludes with a summary of the work in Section 6.
2. Scope of existing systematic ideation analysis approaches
The predominant solution-focused systematic approach, originally outlined by Shah, Smith, and Vargas-Hernandez (Citation2003), has been applied (e.g. Wilson et al. Citation2010; Verhaegen et al. Citation2011; Sun, Yao, and Carretero Citation2014; Toh, Miller, and Okudan Kremer Citation2014; Sluis-Thiescheffer et al. Citation2016; Toh and Miller Citation2016) and modified (e.g. Nelson et al. Citation2009; Peeters et al. Citation2010; Verhaegen et al. Citation2013; Sluis-Thiescheffer et al. Citation2016; Fiorineschi, Frillici, and Rotini Citation2018a, Citation2018b) by a multitude of authors over the past fifteen years. However, to provide a clear overview of the fundamental elements, we briefly outline the procedures as described in the original publication below.
Firstly, during the study, designers are presented with a task(s) that conveys a desired output to be produced and functional requirements to be met during ideation. For instance, Shah, Smith, and Vargas-Hernandez (Citation2003, 127) ask design students to produce ideas for ‘a semi-autonomous device to collect golf balls from a playing field and bring them to a storage area.’ The functional requirements to be met include ‘pick/collect balls’ and ‘transport/manoeuvre device.’ As conveyed on p.128, although there is structural and behavioural variation in the solutions generated by the students, they are all ‘devices for moving over distance’ – i.e. they all ultimately address the same problem. The problem remains essentially ‘fixed’ whilst the solutions vary.
After data collection, performance metrics are used to analyse the ideation processes of designers. Out of the set of four metrics originally proposed by Shah, Smith, and Vargas-Hernandez (Citation2003), the novelty and variety metrics have become particularly prolific. Novelty provides information on the extent to which a designer generated new solutions to address the problem requirements, and variety provides information on the extent to which they generated a range of different solutions. To compute the metrics, generated concepts are firstly interpreted to identify the nature of the solutions used to address each requirement of the problem – i.e. what each solution is, and how it differs from other solutions the designer generated. Scores are then calculated using quantitative data on the number of solutions and differences according to formulae outlined on p.117–129 of Shah, Smith, and Vargas-Hernandez (Citation2003). The procedures involved in computing the two metrics as presented by the authors are briefly summarised in Table .
Table 1. Procedures involved in the evaluation of novelty and variety metrics as presented in Shah, Smith, and Vargas-Hernandez (Citation2003).
In this paper, we report on a study examining exploratory ideation. Unlike solution-focused ideation, this involves open-ended problems where the nature of the desired solution and requirements is unclear (Sosa Citation2018). In this context, rather than generating solutions to a fixed problem, the designer explores different ways of interpreting the open-ended problem and generates solutions to address each of these problem interpretations. Exploratory ideation may be understood in terms of exploration-based models of designing, where the designer is considered to explore two knowledge spaces in parallel: the problem space, encompassing possible problem requirements; and the solution space, encompassing possible artefact solutions/parts thereof (Maher and Tang Citation2003). As shown in Figure , exploratory ideation operates within and across both spaces. In contrast, solution-focused ideation is restricted to a limited part of the solution space, corresponding with a fixed set of requirements in the problem space (Figure ). This is more reflective of search-based models of designing, where the focus of the problem does not change over time (Hay et al. Citation2017).
Analysing exploratory ideation requires a different approach to the solution-focused approach outlined above. Firstly, the fixed problems that tend to be used as stimuli in studies of solution-focused ideation are unlikely to stimulate exploration of different problem interpretations during ideation. The lack of ambiguity leaves little room for alternative perspectives. To stimulate problem exploration under experimental conditions, we need to use open-ended tasks – that is, tasks focusing on a broad design ‘challenge’ to be tackled (Sosa Citation2018, 3), rather than a specific output and requirements. Secondly, the procedures and metrics used to analyse solution-focused ideation (i.e. Shah, Smith, and Vargas-Hernandez (Citation2003) and derivatives) focus solely on the novelty and variety of solutions generated. To analyse exploratory ideation, we need some way of quantifying a designer’s performance in terms of both the solutions and problem interpretations they explored. In turn, this means we need a way of reliably identifying both of these attributes from the concepts generated during ideation. To address these needs, we developed the AEDI approach reported in this paper.
3. Study details
Key details on the neuroimaging study from which the AEDI approach originated are provided in the following sub-sections. The remainder of the paper focuses on the components of AEDI and the ideation analysis results rather than the neuroimaging results, which are reported in Hay et al..
3.1. Overview of study design and participants
The study aimed to investigate the brain regions activated during open-ended (exploratory) and constrained ideation in professional product design engineers. A sample of 30 designers (25 male and 5 female) were asked to complete a series of 20 design ideation tasks and 10 mental imagery control tasks, while we scanned their brain activity using functional magnetic resonance imaging (fMRI). Participants were aged 24–56 years (mean = 31.8, SD = 8.4), and all had at least two years of professional design experience (mean = 7.5 years, SD = 7.0). Of the twenty ideation tasks, ten focused on open-ended problems and ten on more constrained problems. The open-ended tasks are the focus of this paper (Section 3.2), but further details on all tasks are included in Hay et al. Each designer received a different set of 10 open-ended tasks in a random order, and a total of 19 different tasks were used across the sample.
During the ideation tasks, the designers were asked to generate up to three concepts to address a given design problem within a maximum timeframe of 85 s. These parameters were determined through behavioural pilot studies with 35 designers (24 professionals and 11 students), who completed the experimental tasks while sitting at a laptop. The average response times and number of concepts generated were analysed to identify appropriate maximum values for the fMRI protocol, that would also minimise the overall time participants spent in the scanner (to mitigate for the effects of fatigue and physical discomfort).
During fMRI scanning, body and head movements should be minimised to avoid negative effects on data quality. Furthermore, actions such as drawing and talking may activate additional brain regions not associated with the processes under study, yielding misleading results (Abraham Citation2013). As such, we did not allow participants to sketch during the ideation tasks. Instead, at the end of each task we audio recorded a brief verbal summary of the concepts the participant had generated (with these portions of the fMRI data excluded from the analysis). Participants were given 25 s per task for summarising. During this time, they were able to provide sufficient information on their concepts for use as an aid to recalling and sketching them after the scanning session. Again, it was necessary to minimise the length of the verbalisation periods in order to keep the total scanning time to a minimum, and this was optimised during the pilot studies.
After completion of the fMRI scanning, participants were taken to a room where they were asked to listen back to their recorded verbalisations for each task, and produce rough sketches of the concepts generated as best they could remember them. They were asked to refrain from adding extra details. Sketches were produced on A4 response sheets (e.g. Figure ), and participants were asked to include annotations and/or a brief textual description of how the proposed product would work for clarity. One concept was recorded per sheet to ensure that individual concepts could be clearly distinguished by experimenters.
3.2. Open-ended ideation tasks
As discussed in Section 2, solution-focused ideation studies tend to use tasks with specified solutions and functional requirements. In our study, we created a set of open-ended tasks to stimulate the exploration of different problem interpretations alongside solution generation. Tasks were designed to meet the definition of a ‘problem-oriented’ task outlined by Sosa (Citation2018, 3) based on his recent review of tasks used in design ideation research – that is, focused on ‘a challenge such as removing paper or plastic for recycling.’ This may be contrasted with ‘solution-oriented’ tasks, which focus on ‘a desired output such as a seat for a cyber-café.’ Suitable open-ended challenges were identified from a variety of sources, including student design projects in the authors’ university department and publicly available information on design competitions. Task instructions were then written for each challenge, and matched in terms of structure and word count. Examples of the open-ended tasks are presented in Table . Each one provides brief contextual information (Domestic food waste is a serious problem due to global food shortages and socio-economic imbalances), accompanied by a short statement of the problem to be addressed (Generate concepts for products that may reduce unnecessary food wastage in the home). The full set of 19 open-ended tasks used in the study is included in the dataset linked at the end of the paper.
Table 2. Examples of open-ended ideation tasks for use in studies of problem-driven ideation
During the pilot studies mentioned in Section 3.1, the tasks were assessed in two dimensions: (i) applicability, i.e. whether designers could generate different solutions addressing several problem interpretations; and (ii) perceived difficulty, measured on a rating scale from 1 (very easy) to 7 (very difficult). Based on verbal summaries provided by the pilot participants, it was concluded that the majority of designers were able to generate a range of concepts addressing several problems in response to each task. The tasks were also consistently rated as moderately challenging: the mean difficulty rating was 3.76 (SD = 1.08) for professionals and 3.80 (SD = 0.74) for students.
3.3. Analysis of exploratory ideation
To determine what brain regions are activated during exploratory ideation, the fMRI data gathered during this condition can be statistically compared with the data from the other conditions. Regions showing significantly increased/decreased activation in the exploratory ideation condition may be interpreted as those likely to play a key role in this kind of ideation process. The soundness of the results and conclusions from such an analysis is dependent on (amongst other things) the extent to which the designers were engaged in the expected ideation process during the open-ended tasks – as opposed to some off-task activity. Thus, it was necessary to include measures of ideation task performance in the study, which can indicate whether or not participants were engaged in the tasks as expected at the appropriate points in the experiment.
Due to the restrictions on drawing and verbalising inside the MRI scanner, a systematic, output-based analysis approach was identified as the most appropriate means of obtaining the above measures. Given the limitations of the Shah, Smith, and Vargas-Hernandez (Citation2003) approach with respect to exploratory ideation, we developed and applied AEDI to analyse exploratory ideation using the sketches generated post-fMRI scanning. This involves:
Identifying the problem interpretations and solutions explored by the designers, by coding the concept sketches produced for each open-ended task in the study.
Evaluating each designer’s performance in terms of: (i) how many different problem interpretations they considered, i.e. breadth of exploration; and (ii) the extent to which the solutions they generated are new compared to the solutions produced by others designers in the study, i.e. solution novelty.
Section 4 describes each activity in more detail and presents the analysis results.
4. The AEDI approach
The 30 professional designers in our study generated a total of 859 concepts in response to the open-ended ideation tasks. They were able to recall and sketch 94.5% (812) of these after the fMRI scanning. The following sub-sections detail the procedures involved in identifying explored problems and solutions from these sketches (Section 4.1) and quantifying ideation performance (Section 4.2) using the AEDI approach, as well as the results obtained. Examples from the study are presented throughout to illustrate.
4.1. Identification of problem interpretations and solutions
As noted in Section 2, exploratory ideation performance should be quantified in terms of both the problem interpretations and solutions explored by a designer (as opposed to solutions alone). Clearly, to do this it is necessary to define formulae for computing performance scores based on these attributes (Section 4.2). However, in order for the performance scores obtained to be comparable across different studies, as well as replicable, it is important that there is a consistent, systematic procedure for reliably identifying the underlying attributes. Even if two studies use the same formulae for scoring, the scores are not necessarily comparable if the problem and solution attributes were identified through different processes.
Studies applying the solution-focused approach (see Section 2) do not always explain how design outputs were interpreted to obtain the data on solution attributes for performance scoring, and a procedure is not explicitly outlined by Shah, Smith, and Vargas-Hernandez (Citation2003). To provide researchers with a clear, systematic method in studies of exploratory ideation, in AEDI we have formalised a qualitative coding process for identifying problem interpretations and solutions from concept sketches. As shown in Section 4.1.1, this essentially consists of: (1) making inferences about problems and solutions from different sketches; and (2) gradually grouping together sketches that convey the same problems and solutions to identify common attributes.
It is also important to consider the (i) validity and (ii) reliability of the coding conducted. That is, the degree to which it (i) captures the actual intentions of the designers during ideation, and (ii) captures these in a consistent way under different conditions. This in turn affects the validity and reliability of performance scores computed from coding data, i.e. the extent to which they truly and consistently reflect designers’ ideation processes. Compared with quantitative research, it can be challenging to robustly establish validity in qualitative research dealing with subjective interpretations. A limitation of the AEDI approach in this respect, to be addressed in future work, is discussed in Section 5. Regarding (ii), we assessed inter-coder reliability (ICR), defined as the extent to which different researchers agree on the coding of the sketches. The ICR assessment process is described in Section 4.1.2.
4.1.1. Coding of concept sketches
Coding is an analysis method that aims to identify common themes across a set of sources, where themes are represented by qualitative descriptions termed ‘codes’ (Krippendorff Citation2004). In the context of AEDI, the sources are concept sketches and the codes are descriptions of problem interpretations (P-codes) and solutions (S-codes). The output from coding consists of two elements:
a set of coded concept sketches, where each sketch is labelled with the inferred problem interpretation adopted by the designer (P-code) and type of solution generated (S-code); and
a set of P- and S-codes for each ideation task in the study (termed a ‘coding scheme’), which conveys the range of problem interpretations and solutions explored by the whole sample of designers.
To code the sketches produced in response to tasks in our study, we applied the following process to each individual sketch. The numbered steps below are illustrated in Figure , and the process is described with reference to the sketch in Figure :
Based on the visual and textual information provided by the designer, infer what product the sketch describes – this is the solution. For example, the visuals and annotations in Figure suggest that the solution may be described as fridge compartments that indicate what food should be used first.
Infer how the designer is likely to have interpreted the open-ended problem stated in the ideation task, i.e. the problem interpretation. This is based on the inferred solution, any other relevant elements of the sketch, and the task description provided to the designer during ideation. For example, considering the solution inferred from Figure in the context of the task (reducing food waste) suggests that the designer is likely to have interpreted this problem as how to organise food usage more effectively.
Decide whether the inferred problem interpretation and solution are the same as/similar to any of those identified from other sketches in the sample:
If different (or if this is the first sketch in the sample to be coded), the problem interpretation and solution are added to the coding scheme for the task as P- and S-codes, respectively. These codes are then used to label the sketch.
If same, label the sketch using existing P- and S-codes in the scheme that match the problem interpretation and solution inferred from the sketch.
If similar, adapt and/or merge existing codes to create new codes that better describe the sketch plus similar previously coded sketches.
Repeatedly applying this process yielded complete coding schemes of P- and S-codes for each ideation task. As an example, the coding scheme for task 1 (see Table for task description) is presented in Table . The coding was conducted by one coder (C1) with 10 years of experience in product design engineering (education and research), using the NVivo software system (QSR International Citation2018). To reduce the potential for bias towards the perspective of C1, the coding schemes were also reviewed and discussed at regular intervals by the coder’s research team (comprised of design and psychology academics/researchers) and refinements made where necessary. In total, 97.3% of the 812 sketches analysed were successfully coded with problem interpretations and solutions. In certain cases, it was not possible to assign a P- or S-code to sketches and one of four alternative classifications was applied:
Insufficient information to interpret concept: the sketch did not provide sufficient detail for interpretation (1.4% of sketches).
Multiple concepts: the participant had recorded multiple concepts on a single sheet and the sketch could not be uniquely categorised (0.1% of sketches).
Inappropriate task response: the concept was interpreted as unrelated to the task description (0.5% of sketches).
Service: a service rather than a product was generated (0.7% of sketches). Services were excluded from analysis due to the focus on product design engineering.
Table 3. Coding scheme defined for task 1 in Table (reducing domestic food waste).
4.1.2. Inter-coder reliability
Following completion of the coding process in Figure , we assessed the reliability of the P- and S-coding by testing ICR as noted previously. ICR quantifies the extent to which independent coders agree on the interpretation of the sketches given the same coding instrument (Krippendorff Citation2011) – in this case, the coding schemes developed by C1 and the research team. Higher levels of agreement indicate more reliable coding. Lower levels indicate less reliable coding, and suggest that the codes and/or coding process require alteration (Krippendorff Citation2004).
To test ICR (Figure ), the coding schemes for the ideation tasks were applied by two coders (C2 and C3) independently of C1 to interpret a subset of the sketches, termed the reliability sample (as a general rule, ∼10% of the total sample size (Campbell et al. Citation2013)). C2 was a design professional with over 30 years of industrial experience, and C3 was a PhD student with 1.5 years of experience in design cognition. C2 and C3 were firstly trained in the coding process on small subsets of sketches (∼3% of the total sample), using a coding manual developed by C1. They then independently coded a reliability sample consisting of ∼16% of the total sketch sample (selected using a random number generator). The process for ICR testing differed from the iterative process involved in the initial coding of sketches (Section 4.1.1) – the focus was on interpreting sketches using the defined coding schemes, and noting any shortcomings within these. C2 and C3 were instructed to apply the following process to each sketch in the reliability sample:
Based on the visual and textual information provided by the designer, infer what solution the sketch describes. Label the sketch with the most appropriate S-code from the coding scheme.
Infer how the designer is likely to have interpreted the open-ended problem stated in the ideation task, based on the inferred solution, any other relevant sketch elements, and the task description provided during ideation. Label the sketch with the most appropriate P-code from the coding scheme.
If no appropriate code can be found within the coding schemes in steps 1 and 2, propose an addition or amendment.
The codes applied to sketches in the reliability sample by C1, C2, and C3 were then reviewed to determine instances of agreement and disagreement. Using this information, ICR was quantified using a statistical measure of agreement called Krippendorff’s alpha (Hayes and Krippendorff Citation2007). A procedure for computing this measure in SPSS is available online (e.g. De Swert Citation2012), along with guidance on how to interpret the results. In general, alpha yields a value between 0 and 1, where 1 indicates perfect agreement and 0 indicates no agreement above what would be expected by chance (Krippendorff Citation2011). Krippendorff’s alpha = ∼0.80 was achieved for both coding variables (i.e. P and S), which indicates relatively strong agreement between coders and acceptable ICR (Krippendorff Citation2004). Identified disagreements and areas for refinement were addressed to arrive at a final agreed interpretation of the concept sketches. Key figures and results from the coding and ICR testing are summarised in Table .
Table 4. Key figures and results from coding and ICR testing.
4.2. Ideation performance evaluation
As discussed in Section 2, the systematic variety and novelty metrics from the solution-focused approach described by Shah, Smith, and Vargas-Hernandez (Citation2003) have become particularly prolific in design ideation research (Table ). We used our data on P- and S-codes to define and compute comparable performance metrics for exploratory ideation, which account for the exploration of different problem interpretations alongside solutions. Figures and present a representation of how a designer might explore a particular problem and solution space (the same space in each figure). The shading and labels illustrate the conceptual differences between breadth (Figure ) and novelty (Figure ) evaluation in our exploratory approach vs Shah, Smith, and Vargas-Hernandez (Citation2003).
Figure 6. Breadth as measured for (i) ideation in response to fixed problems in Shah, Smith, and Vargas-Hernandez (Citation2003), and (ii) exploratory ideation in AEDI.
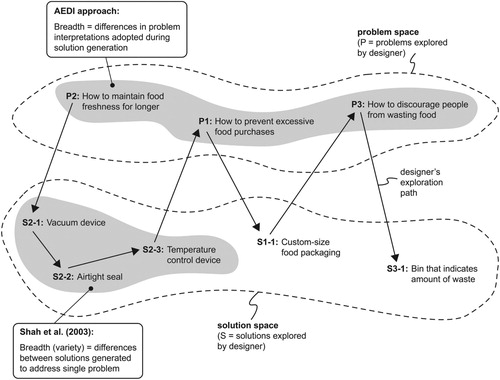
Figure 7. Solution novelty as measured for (i) ideation in response to fixed problems in Shah, Smith, and Vargas-Hernandez (Citation2003), and (ii) exploratory ideation in AEDI.
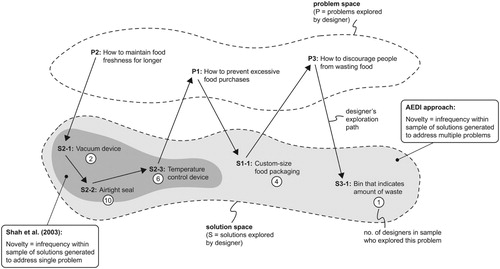
The variety metric used in Shah, Smith, and Vargas-Hernandez (Citation2003) measures the breadth of solutions generated in response to a fixed problem, as shown in Figure . As per Table , this is quantified in terms of differences in the physical/working principles, embodiments, and details of solutions. In exploratory ideation, differences in the problem interpretations explored by a designer seem to be a more appropriate measure of breadth than differences in specific solution attributes: as shown in Figure , each time the designer reinterprets the problem, they shift their attention to a different category of potential solutions. A designer who explores three different interpretations of the problem will therefore generate a broader set of solutions than another who fixates on a single interpretation. Using the coding data, we calculated a breadth score per task for each designer by counting the number of times they reinterpreted the problem. That is, the number of times the P-codes applied to their sketches for a given task can be differentiated, equal to the number of P-codes (np) minus 1. As per Equation (1), this is divided by the maximum number of differentiations achieved for the task across the sample of designers (np_diff_max) so that the maximum score is always 1. Note that the score is based on differentiations rather than the number of P-codes so that a designer who focused on a single problem interpretation (i.e. demonstrated no breadth) scores zero.
Equation (1): Formula for calculating breadth scores using data on P-codes
We also used the coding data to evaluate the novelty of solutions. As shown in Table and illustrated in Figure , Shah, Smith, and Vargas-Hernandez (Citation2003) compute the novelty of a solution to a particular requirement as its infrequency within the total sample of solutions generated (across all designers) for that requirement. That is, the less frequently a solution appears in the sample, the more novel it is. The overall novelty of a concept is computed as the sum of its solution-level novelty scores across all requirements of the problem. In AEDI, concepts generated through exploratory ideation are coded in terms of a problem interpretation (P-code) and associated solution that addresses this problem (S-code). To assign a novelty score to a concept in this context, we determined how frequently the S-code describing the solution is applied within the total sample of concept sketches produced for a particular task. That is, accounting for all possible interpretations of the problem (P-codes) as shown in Figure . A frequency-based novelty scoring method described in the psychology literature was adopted, where S-codes (and in turn, coded concepts) receive a novelty score of 2, 1, or 0 according to frequency thresholds (Mouchiroud and Lubart Citation2001; Chou and Tversky Citation2017):
an S-code applied to ≤ 2% of the sketches produced for a task scores 2 (most novel);
an S-code applied to 3–5% of the sketches scores 1 (moderately novel); and
an S-code applied to >5% of the sketches scores 0 (least novel).
To briefly illustrate the scoring of breadth and novelty in exploratory ideation using the methods outlined above, consider the set of three coded sketches from our study presented in Figure . These were generated by a single designer in response to task 1 in Table (reducing domestic food waste). A total of 65 concept sketches were produced for this task by the sample of designers in the study. Firstly, the sketches in Figure are coded with three different P-codes, i.e. the P-codes can be differentiated twice. The maximum number of P-code differentiations achieved for this task by any designer was 2. According to Equation (1), the participant therefore receives a breadth score of 1 for the task. Secondly, the frequency thresholds for scoring novelty are as follows:
2% of the sample = 0.02 × 65 = 1 → an S-code applied to 1 sketch in the sample scores 2 (most novel);
5% of the sample = 0.05 × 65 = 3 → an S-code applied to 2–3 sketches in the sample scores 1 (moderately novel); and
An S-code applied to more than 3 sketches in the sample scores 0 (least novel).
The S-codes for each sketch in Figure were applied to the following number of concepts in the overall sample for the task: S1 = 2; S2 = 1; and S3 = 1. Thus, concepts 2 and 3 (S2 and S3) are the most novel and score 2, whilst concept 1 (S1) is moderately novel and scores 1.
Table presents summary statistics for the breadth and solution novelty scores obtained via the above formulae for the 30 study participants. The results are visualised in frequency plots in Figures and . Participant scores were computed by averaging the scores obtained across tasks (for breadth) and individual concept sketches (for novelty). The scores for breadth were positively skewed indicating higher frequencies for lower breadth scores. For novelty the scores were normally distributed but limited in range, with the maximum recorded score of 1 out of a possible of 2. Overall, the results demonstrate that applying the metrics produces a distribution of scores that can be used to rank and compare designers with respect to their exploratory ideation performance.
Figure 8. A set of coded concept sketches from our study, generated by a single designer in response to task 1 in Table (reducing domestic food waste). (colour online).
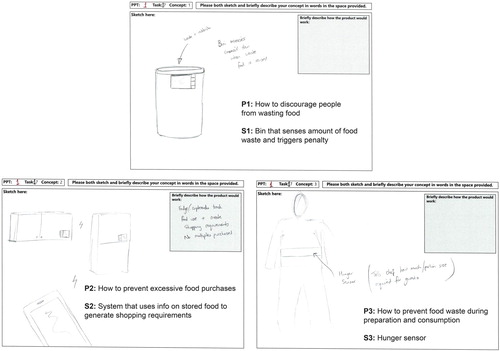
Table 5. Summary statistics for breadth and novelty scores in the study sample (N = 30).
5. Discussion
In this paper, we have addressed a lack of systematic approaches for analysing exploratory ideation by presenting and demonstrating AEDI. The approach includes three key elements: (1) open-ended ideation tasks; (2) identification of explored problems and solutions via coding of sketches; and (3) evaluation of exploratory ideation performance based on the coding data.
AEDI provides a means for researchers to study exploratory ideation using a systematic, output-based approach for the first time. It may be considered to complement the solution-focused approach originated by Shah, Smith, and Vargas-Hernandez (Citation2003) discussed in Section 2. Systematic approaches can have advantages over other output-based approaches, e.g. rating methods, in that they may produce more comparable and replicable results (Shah, Smith, and Vargas-Hernandez Citation2003) and be more efficient in the context of larger samples (Sluis-Thiescheffer et al. Citation2016). As discussed in Hay et al. (Citation2017), there is a need for larger-scale, quantitative experiments in design to build upon small-scale, qualitative studies. AEDI can contribute to addressing this challenge in the context of exploratory ideation, where research topics include the nature of exploration patterns (Murray et al. Citation2019) and their link with solution novelty (Finke, Ward, and Smith Citation1992) and other attributes of design outcomes (Studer et al. Citation2018). We used AEDI in an fMRI study of professional product design engineers, where an output-based analysis approach was necessitated by constraints on verbalisation and physical behaviour inside the MRI scanner. The approach may be useful in other kinds of neuroimaging technique with similar constraints (e.g. EEG), which are becoming increasingly popular in design research (e.g. Shealy, Hu, and Gero Citation2018; Goucher-Lambert, Moss, and Cagan Citation2019; Hay et al. Citation2019). More generally, AEDI may be useful in any empirical investigation of ideation employing open-ended design problems.
The AEDI approach provides a consistent set of tasks for studying exploratory ideation. The range of scores obtained for the breadth metric in our study (0.30–0.80, mean = 0.53, SD = 0.12), which is based on the number of times the problem was reinterpreted, indicates that the tasks do stimulate problem exploration as intended. The coding schemes can be used to obtain a reliable interpretation of problems and solutions from the concept sketches produced by designers, in the sense that multiple researchers are able to agree on how the coding schemes should be applied to interpret the sketches. Furthermore, relatively strong agreement was obtained between three coders with different design backgrounds and levels of experience (Krippendorff’s alpha = ∼0.80). This suggests that a range of researchers can be involved in the interpretation of sketches, as opposed to experts alone.
There are two key limitations which should be addressed in future work to further develop and refine the approach. Firstly, as discussed in Section 4.1.1, the coding schemes were initially defined by a single researcher who coded the full sketch sample. This decision was largely based on the availability of coders at the time we conducted the study. To minimise bias, we included the perspectives of multiple researchers in coding scheme development through regular team reviews and refinement of the emerging codes. However, whilst we measured how reliably the developed schemes can be applied to interpret sketches (Section 4.1.2), we did not measure the reliability of the initial development process. That is, the extent to which independent researchers would agree on what P- and S-codes should be defined initially based on the sketch data (Perry and Krippendorff Citation2013). If high ICR can be demonstrated in this part of the coding process, the efficiency of coding could be increased by dividing the sketch sample between several researchers coding in parallel. If reliability is low, this may suggest that changes are required in the manner that P- and S-codes are defined. Thus, this could constitute an important area for further investigation.
A second, more fundamental limitation is that we did not assess the validity of the coding. That is, the extent to which the P- and S-codes reflect the problems and solutions that were actually explored by designers during the study (Section 4.1). Although independent coders were able to agree on how to interpret the sketches using the codes (reliability), there is no guarantee that the codes are a true reflection of the designers’ intentions. In turn, it is not clear from the present work how much the breadth and novelty scores are determined by the participants’ ideation process versus the judgment of the coders. This limitation is not unique to AEDI – it is pervasive in ideation studies involving the interpretation of design outputs, including the work of Shah, Smith, and Vargas-Hernandez (Citation2003) and derivatives discussed in Section 2. It is particularly problematic when the outputs being interpreted are concept sketches, which are highly (and necessarily) ambiguous (Fish and Scrivener Citation1990; Bafna Citation2008). To address this, we could go back to the designers in our study and ask them how well the judgments made by coders match their recalled intentions during the study. However, this is not an effective way of ensuring validity in future applications of AEDI. A better solution in future may be to ask the designers to code their own sketches after producing them, i.e. describe in words the problem and solution they considered. The role of the researchers would then be to look for commonalities among the codes defined by designers, grouping similar codes together to obtain a more general set that could be used to compute breadth and novelty scores. We could omit sketching altogether and instead ask designers to describe their concepts in words only, which may be a more unambiguous but less natural mode of idea externalisation. The validity of the proposed exploratory ideation performance metrics is fundamentally dependent on the validity of the codes with respect to designer intentions. Thus, finding efficient and effective ways to ensure coding validity is a key area for future improvement in the AEDI approach.
6. Conclusion
Ideation is a salient area of interest for design researchers, and a considerable body of work now exists on the topic. With respect to output-based ideation analysis, systematic approaches are considered to have benefits over other methods (e.g. greater comparability and replicability of results, and higher efficiency in large samples). The predominant systematic approach, originating in Shah, Smith, and Vargas-Hernandez (Citation2003), focuses on what may be termed solution-focused ideation, i.e. the generation of solutions to address a fixed problem specifying a desired output and requirements. However, this approach does not deal with exploratory ideation, which involves the exploration of different interpretations of an open-ended problem and the generation of solutions to address these (Dorst and Cross Citation2001). To enable the perceived benefits of systematic analysis to be realised in studies on exploratory ideation, a new approach is needed that accounts for both the problem and solution spaces.
In this paper, we provide a means to systematically analyse exploratory ideation for the first time through a new approach: Analysis of Exploratory Design Ideation (AEDI). AEDI fundamentally differs from existing solution-focused systematic approaches in two ways: (i) the use of tasks focusing on open-ended design problems as stimuli; and (ii) the analysis of ideation in terms of explored problem interpretations and solutions rather than just the latter alone. AEDI consists of three key components: (1) open-ended ideation tasks; (2) identification of explored problems and solutions via coding of sketches; and (3) evaluation of exploratory ideation performance based on the coding data. We developed and applied the approach to analyse ideation in an fMRI study of 30 professional product design engineers, who produced a sample of 812 concept sketches in response to 19 ideation tasks. The results demonstrate that the approach provides: (i) a consistent set of tasks for studying exploratory ideation, which stimulate problem exploration alongside solution generation; (ii) a reliable means of interpreting problems and solutions from concept sketches via coding schemes; and (iii) an appropriate way to rank and compare designers based on exploratory ideation performance.
AEDI enables the perceived benefits of systematic ideation analysis to be realised in research on exploratory ideation, e.g. greater comparability and replicability of results (Shah, Smith, and Vargas-Hernandez Citation2003), and greater efficiency in large samples (Sluis-Thiescheffer et al. Citation2016). From this perspective, the approach can support larger scale quantitative studies on exploratory design ideation, helping to address a broader need for increased sample sizes in design research (Hay et al. Citation2017). More generally, the approach may be useful in any empirical investigation of ideation employing open-ended design problems. Two future improvements to be made to the approach are: (1) assessing the reliability of coding scheme development as well as application; and (2) finding efficient and effective ways to ensure that the coding schemes are valid representations of the problems and solutions actually explored by designers in a study. That is, reflective of the designers’ intentions.
Data statement
All data underpinning this publication are openly available from the University of Strathclyde KnowledgeBase at https://doi.org/10.15129/a82c32a8-689a-4be5-a61f-17d60eaad10c.
Disclosure statement
No potential conflict of interest was reported by the authors.
ORCID
Additional information
Funding
References
- Abraham, Anna. 2013. “The Promises and Perils of the Neuroscience of Creativity.” Frontiers in Human Neuroscience 7 (June): 1–9. doi:10.3389/fnhum.2013.00246.
- Bafna, Sonit. 2008. “How Architectural Drawings Work — and What That Implies for the Role of Representation in Architecture.” The Journal of Architecture 13 (5): 535–564. doi:10.1080/13602360802453327.
- Campbell, John L., Charles Quincy, Jordan Osserman, and Ove K. Pedersen. 2013. “Coding In-Depth Semistructured Interviews: Problems of Unitization and Intercoder Reliability and Agreement.” Sociological Methods & Research 42 (3): 294–320. doi:10.1177/0049124113500475.
- Chou, Yung-Yi Juliet, and Barbara Tversky. 2017. “Finding Creative New Ideas: Human-Centric Mindset Overshadows Mind-Wandering.” Proceedings of the 39th Annual Meeting of the Cognitive Science Society, London, UK, July 26th-29th , 1776–1781.
- De Swert, Knut. 2012. “Calculating Inter-Coder Reliability in Media Content Analysis Using Krippendorff’s Alpha.” http://www.polcomm.org/wp-content/uploads/ICR01022012.pdf.
- Dorst, Kees, and Nigel Cross. 2001. “Creativity in the Design Process: Co-Evolution of Problem–Solution.” Design Studies 22 (5): 425–437. doi:10.1016/S0142-694X(01)00009-6.
- Finke, Ronald A., Thomas B. Ward, and Steven M. Smith. 1992. Creative Cognition: Theory, Research, and Applications. Cambridge, MA; London, England: The MIT Press.
- Fiorineschi, L., F. S. Frillici, and F. Rotini. 2018a. “A-Posteriori Novelty Assessments for Sequential Design Sessions.” International Design Conference - Design 2018: 1079–1090. doi:10.21278/idc.2018.0119.
- Fiorineschi, L., F. S. Frillici, and F. Rotini. 2018b. “Issues Related To Missing Attributes in A- Posteriori Novelty Assessments.” International Design Conference - Design 2018: 1067–1078. doi:10.21278/idc.2018.0118.
- Fish, Jonathan, and Stephen Scrivener. 1990. “Amplifying the Mind’s Eye: Sketching and Visual Cognition.” Leonardo 23 (1): 117–126. http://www.jstor.org/stable/1578475?seq=1#page_scan_tab_contents. doi: 10.2307/1578475
- Gero, John S., and Thomas McNeill. 1998. “An Approach to the Analysis of Design Protocols.” Design Studies 19 (1): 21–61. doi:10.1016/S0142-694X(97)00015-X.
- Goucher-Lambert, Kosa, Jarrod Moss, and Jonathan Cagan. 2019. “A Neuroimaging Investigation of Design Ideation with and Without Inspirational Stimuli—Understanding the Meaning of Near and Far Stimuli.” Design Studies 60 (January): 1–38. doi:10.1016/J.DESTUD.2018.07.001.
- Hay, L., A. Duffy, S. J. Gilbert, L. Lyall, G. Campbell, D. Coyle, and M. Grealy. 2019. “The Neural Underpinnings of Creative Design.” In Cognitive Neuroscience Society Annual Meeting, 172. San Francisco: Cognitive Neuroscience Society.
- Hay, Laura, Alex H. B. Duffy, Chris McTeague, Laura M. Pidgeon, Tijana Vuletic, and Madeleine Grealy. 2017. “A Systematic Review of Protocol Studies on Conceptual Design Cognition: Design as Search and Exploration.” Design Science 3 (July): 1–36. doi:10.1017/dsj.2017.11.
- Hayes, Andrew F., and Klaus Krippendorff. 2007. “Answering the Call for a Standard Reliability Measure for Coding Data.” Communication Methods and Measures 1 (1): 77–89. http://afhayes.com/public/cmm2007.pdf. doi: 10.1080/19312450709336664
- Krippendorff, K. 2004. Content Analysis: An Introduction to Its Methodology. 2nd ed. Thousand Oaks: Sage.
- Krippendorff, Klaus. 2011. “Agreement and Information in the Reliability of Coding.” Communication Methods and Measures 5 (2): 93–112. doi:10.1080.19312458.2011.568376. doi: 10.1080/19312458.2011.568376
- Maher, Mary, and Hsien-Hui Tang. 2003. “Co-Evolution as a Computational and Cognitive Model of Design.” Research in Engineering Design 14 (1): 47–64. doi:10.1007/s00163-002-0016-y.
- Mouchiroud, Christophe, and Todd Lubart. 2001. “Children’s Original Thinking: An Empirical Examination of Alternative Measures Derived From Divergent Thinking Tasks.” The Journal of Genetic Psychology 162 (4): 382–401. doi:10.1080/00221320109597491.
- Murray, Jaclyn K., Jaryn A. Studer, Shanna R. Daly, Seda McKilligan, and Colleen M. Seifert. 2019. “Design by Taking Perspectives: How Engineers Explore Problems.” Journal of Engineering Education 108 (2): jee.20263. doi:10.1002/jee.20263.
- Nelson, Brent A., Jamal O. Wilson, David Rosen, and Jeannette Yen. 2009. “Refined Metrics for Measuring Ideation Effectiveness.” Design Studies 30 (6): 737–743. doi:10.1016/j.destud.2009.07.002.
- Peeters, J., P. Verhaegen, D. Vandevenne, and J. R. Duflou. 2010. “Refined Metrics for Measuring Novelty in Ideation.” Proceedings of IDMME - Virtual Concept 2010: 1–4.
- Perry, Gabriela Trindade, and Klaus Krippendorff. 2013. “On the Reliability of Identifying Design Moves in Protocol Analysis.” Design Studies 34 (5): 612–635. doi:10.1016/J.DESTUD.2013.02.001.
- QSR International. 2018. “Discover the NVivo Suite.” 2018. http://www.qsrinternational.com/nvivo/nvivo-products.
- Shah, Jami J., Steve M. Smith, and Noe Vargas-Hernandez. 2003. “Metrics for Measuring Ideation Effectiveness.” Design Studies 24 (2): 111–134. doi:10.1016/S0142-694X(02)00034-0.
- Shealy, Tripp, Mo Hu, and John Gero. 2018. “Patterns of Cortical Activation When Using Concept Generation Techniques of Brainstorming, Morphological Analysis, and TRIZ.” In Volume 7: 30th International Conference on Design Theory and Methodology, 1–9. ASME. doi:10.1115/DETC2018-86272.
- Sluis-Thiescheffer, Wouter, Tilde Bekker, Berry Eggen, Arnold Vermeeren, and Huib De Ridder. 2016. “Measuring and Comparing Novelty for Design Solutions Generated by Young Children Through Different Design Methods.” Design Studies 43 (March): 48–73. doi:10.1016/J.DESTUD.2016.01.001.
- Sosa, Ricardo. 2018. ““Metrics to Select Design Tasks in Experimental Creativity Research.” Proceedings of the Institution of Mechanical Engineers, Part C: Journal of Mechanical Engineering Science 0 (0): 1–11. doi:10.1177/0954406218778305.
- Studer, Jaryn A., Shanna R. Daly, Seda McKilligan, and Colleen M. Seifert. 2018. “Evidence of Problem Exploration in Creative Designs.” Artificial Intelligence for Engineering Design, Analysis and Manufacturing 32 (4): 415–430. doi:10.1017/S0890060418000124.
- Sun, Ganyun, Shengji Yao, and Juan A. Carretero. 2014. “Comparing Cognitive Efficiency of Experienced and Inexperienced Designers in Conceptual Design Processes.” Journal of Cognitive Engineering and Decision Making 8 (4): 330–351. doi:10.1177/1555343414540172.
- Suwa, Masaki, John Gero, and Terry Purcell. 2000. “Unexpected Discoveries and S-Invention of Design Requirements: Important Vehicles for a Design Process.” Design Studies 21 (6): 539–567. doi: 10.1016/S0142-694X(99)00034-4
- Toh, Christine A., and Scarlett R. Miller. 2016. “Choosing Creativity: The Role of Individual Risk and Ambiguity Aversion on Creative Concept Selection in Engineering Design.” Research in Engineering Design 27 (3): 195–219. doi:10.1007/s00163-015-0212-1.
- Toh, Christine A., Scarlett R. Miller, and Gül E. Okudan Kremer. 2014. “The Impact of Team-Based Product Dissection on Design Novelty.” Journal of Mechanical Design 136 (4): 041004. doi:10.1115/1.4026151.
- Verhaegen, Paul-Armand, Jef Peeters, Dennis Vandevenne, Simon Dewulf, and Joost R. Duflou. 2011. “Effectiveness of the PAnDA Ideation Tool.” Procedia Engineering 9 (January): 63–76. doi:10.1016/J.PROENG.2011.03.101.
- Verhaegen, Paul Armand, Dennis Vandevenne, Jef Peeters, and Joost R. Duflou. 2013. “Refinements to the Variety Metric for Idea Evaluation.” Design Studies 34 (2): 243–263. doi:10.1016/j.destud.2012.08.003.
- Wilson, Jamal O., David Rosen, Brent A. Nelson, and Jeannette Yen. 2010. “The Effects of Biological Examples in Idea Generation.” Design Studies 31 (2): 169–186. doi:10.1016/J.DESTUD.2009.10.003.