Abstract
Background: Recently, biologic agents administered as a subcutaneous injection have been introduced as treatment options for atopic dermatitis (AD). Biologic treatments differ considerably from traditional topical and systemic anti-inflammatory treatments, and it is unclear how this may impact patient and physician preferences for treatments.
Objective: To examine the treatment preferences for new injection treatments of patients and physicians.
Methods: Discrete choice experiment methodology was used to quantify preferences for treatments via an online survey. Participants were presented with a series of choice scenarios; treatment options were described using the following attributes: add-on or replacement treatment, efficacy of improving rashes and itching, time until response, place of administration, injection site reaction, risk of mild-to-moderate and severe side effects, frequency of administration and cost.
Results: 76.67% of physicians and 46.24% of patients opted-in to the new treatment in the scenarios presented. Of those who opted-in to treatment, physicians were more likely to value the efficacy of treating rashes and were more concerned about cost than patients. Patients preferred add-on treatments and were against self-administering the treatment at home.
Conclusion: Overall patients and physicians differ in their preferences for AD treatments. These findings have implications for shared decision making and clinical practice.
Introduction
Atopic dermatitis (AD) is a chronic inflammatory skin condition characterized by pruritus, erythema and scaly lesions. AD is caused by a combination of underlying genetic and environmental factors. Current treatment and management options for AD include emollients; topical anti-inflammatory creams (e.g. topical corticosteroids (TCS)); and systemic anti-inflammatory medications (e.g. cyclosporine). For people with moderate-to-severe AD, these treatments may have limited or short-lasting efficacy. This may be compounded by poor treatment adherence due to negative feelings and beliefs about treatments and a fear of side effects from prolonged use, particularly with TCS, resulting in suboptimal outcomes (Citation1,Citation2). More recently, biologic agents such as dupilumab have demonstrated good clinical efficacy in treating AD (Citation3,Citation4), though patient preferences and adherence for new biologic agents are unclear.
Given the impact of patient preferences on adherence and treatment outcomes, it is important to understand patient treatment preferences and potential concordances/discordances between patient and physician preferences. Studies of treatment preferences have utilized a range of methodologies from simple surveys and ranking/rating approaches, to more complex empirical methods such as discrete choice experiments (DCEs). DCE methodology is favored over ranking/rating approaches as the tasks presented to participants are more consistent with how decisions are made in reality and is underpinned by economic theory (Citation5–7). DCEs are increasingly being used to evaluate treatment and health care preferences across medical disciplines (Citation8–11) and have been used to quantify patient and physician preferences for the treatments of dermatological conditions including psoriasis, melanoma and dermatological surgeries (Citation12–17). No such methods have been used to quantify preferences in AD treatment, nor has there been much research examining how patients and physicians evaluate the benefits and risks of treatment, or how they may differ, using other empirical methods. This study aims to examine and quantify the treatment preferences of patients with AD and physicians using DCE methodology.
Materials and methods
A 15–20-min online survey with a DCE component was conducted February–March 2018 to understand the treatment preferences of AD patients and physicians (dermatologists) in Japan. In the DCE task, participants are presented with a traditional treatment choice, focusing on the clinical benefits and associated risks. The decision-maker trades off attributes and selects the alternative that maximizes their ‘utility’ (i.e. satisfaction/value). By observing how decision-makers change their responses over choice situations, we can infer the importance they place on the attributes. The study was approved by the sponsor’s internal approval committee and was conducted in accordance with ethical and legal guidelines in Japan.
Participants
Patients with moderate to very severe AD and physicians (dermatologists certified by the Japanese Dermatological Association: JDA) who treat patients with AD were invited to participate in this study. Patients were eligible to participate if they satisfied the following criteria: aged 15 years and over; AD diagnosed by a health care professional; categorized as having moderate to very severe AD on the Patient Oriented Eczema Measure (POEM) scale (Citation18); and were either currently and/or previously using TCS as an AD treatment. To be eligible to participate, physicians were required to be certified in dermatology and treating patients with AD at the time of the survey. Physicians were classified as general physicians (GP) or hospital physicians (HP). HPs were separated into those who were practicing at a facility certified by the JDA in the use of biologic treatments for psoriasis and those who were practicing at a facility that was not certified. Participants were recruited nation wide across Japan through consumer (Rakuten AIP and Survey Sampling International) and specialist health care online research panels (M3 global research) and provided informed consent prior to participation.
DCE survey
The survey consisted of the DCE with additional questions in both the patient and physician surveys on diagnosis; burden and impact of AD; experience with treatments; and demographics. The patient survey included the Dermatology Life Quality Index (DLQI) (Citation19) and the Treatment Satisfaction Questionnaire for Medication (TSQM-9) (Citation20) to measure quality-of-life and treatment satisfaction.
A generic design was used for an injection treatment, with treatment profiles labeled as ‘Injection Treatment A’ and ‘Injection Treatment B’ and a ‘none’ alternative as an opt-out (). The DCE attributes and corresponding levels identified () were derived through interrogation of existing literature, evidence from clinical trials and currently funded treatments, as well as consultation with technical (health economics and medical experts) advisors and physicians. The experimental design followed good practice guidelines (Citation21), and the combinations of levels presented in the tasks were generated using Bayesian efficient design (Citation22) in Ngene (software used to design DCE experiments).
Table 1. DCE attributes and levels.
The DCE scenarios consisted of 12 choice tasks, focusing on clinical benefits and potential risks of treatments and non-health outcomes, e.g. frequency of administration and cost of treatment. To determine whether physicians’ preferences differed by disease severity, physicians were asked to respond to six choice tasks for patients with moderate AD and six choice tasks for patients with severe–very severe AD (). Each treatment scenario presented a different combination of the attribute levels, based on the design.
Analysis
The data was cleaned to remove participants who had incoherent open-text responses, had a completion time of less than 5 min or indicated a poor understanding of the survey based on a scale-based measure (understanding levels of less than 3/10).
Descriptive statistics were used to examine the participant demographic and treatment characteristics. The scores for each question on the DLQI were summed to give a total score from 0 to 30 with higher scores indicating a higher (negative) impact on patients’ quality of life. The TSQM-9 was scored across three domains: global satisfaction, effectiveness and convenience, each scored between 0 and 100 with higher scores indicating higher levels of satisfaction.
For the DCE data, participants were first categorized into two groups based on their treatment choices into those that opted out for all scenarios (i.e. participants who selected their current treatments for all scenarios) and those who opted in (i.e. participants who traded between treatments across the scenarios). Participants who opted out for all scenarios could have been included in the choice model but would have added no information about the attributes and only influenced the magnitude of the constant representing the opt-out. A binary logit model was used to determine the demographic and treatment characteristics associated with opting-in or out of the new treatment. Bivariate correlations were calculated to examine associations among demographic and treatment variables for patients (gender, age, working status, income, disease severity, current number of treatments, treatment costs, needle fear, TSQM-9 domain scores and DLQI scores) and physicians (gender, age, type of physician, average number of mild, moderate, severe–very severe patients seen per month and biologics certification). Variables that were not significantly correlated were then included in binary logit models.
DCE data for patients and physicians who opted in for the new treatment were analyzed together using a Mixed Multinomial Logit (MMNL) model. The MMNL accommodates heterogeneity (i.e. differences in preferences between groups) and allows for the determination of the value of the treatment attributes examined. The data was pooled to check for differences between the patients and physicians by exploring heterogeneity in the estimated mean of the random parameters. Statistical analyses were performed in NLOGIT version 6 (Econometric Software, Inc.) and IBM SPSS version 25 (IBM), and p < .05 criteria was used to determine statistical significance. The adjusted McFadden Pseudo R-squared was used to assess model fit.
To operationalize the DCE models in a manner that is meaningful for stakeholders, a dashboard was developed, which enables users to perform ‘what if’ scenarios based on hypothesized changes to treatment attributes. The dashboard was constructed in R Shiny and provides a comparison of patient and physician preferences for AD treatments, based on the treatment attributes examined in the DCE (). The comparisons were segmented by disease severity (moderate AD and severe–very severe AD).
Results
Demographics
Data from 28 patients and 3 physicians were removed during data cleaning. The final sample consisted of 323 patients (N = 222 moderate patients, N = 101 severe–very severe patients) and 121 physicians (N = 61 GPs, N = 44 HPs at a biologic-certified facility and N = 16 HPs at a non-biologic-certified facility). Demographic and clinical characteristics by patient disease severity are shown in . Demographic and clinical characteristics of physicians are displayed in .
Table 2. Patient demographic and clinical characteristics by disease severity.
Table 3. Physician demographic and clinical characteristics.
Patient-reported outcomes
Patients’ scores on patient-reported outcomes around disease severity (POEM), treatment satisfaction (TSQM-9) by the three subdomains global satisfaction, effectiveness and convenience, impact on quality of life (DLQI) and fear of needles are displayed in .
Table 4. Patient-reported outcomes by severity.
DCE results
Participants were categorized as either opting-in to new treatment (traded between treatment alternatives in the DCE scenarios) or opting-out of new treatment (i.e. always preferred their current treatments). Overall, 46.24% of patients (N = 129) opted in to new treatment and 53.76% of patients (N = 150) opted out of new treatment. Open-text responses revealed that patients opted out of the new treatment because of high costs (53.10%), uncertainty around the efficacy of the new treatment (13.79%) and dislike of injection treatments (12.41%). Conversely, 77.67% of physicians (N = 87) opted in to new treatment and 22.33% of physicians opted out of new treatment. Open-text responses revealed that physicians opted out of the new treatment because of cost (68.00%), the perception that current treatments were sufficient (16.00%), concerns about side effects and personal concerns (or perceptions of patient concerns) about injection treatments (16.00%). Binary logit models were used to examine demographic and clinical characteristics associated with participants opting-in or opting-out of the new treatment.
Patient binary logit model
Gender, age, current treatment costs, needle fear, TSQM-9 convenience scores and job status were included as binary explanatory variables in the model. As per accepted statistical methods, other demographic and clinical characteristic variables including disease severity, income, the number of current treatments, other TSQM-9 domain scores and DLQI scores were not included in the model due to a high level of correlation between variables which biases the model results (p < .05).
The output of the model with the best fit is displayed in . Patients with high treatment costs, low needle fear and low satisfaction on the TSQM-9 Convenience scale and working full time had significantly higher odds of opting-in to the new treatment (p < .05).
Table 5. Binary logit model output for patients.
Physician binary logit model
Age, gender and working at a biologic-certified facility were included as binary explanatory variables in the model. Other demographic and clinical characteristic variables (type of physician and the average number of mild, moderate and severe–very severe patients seen per month) were not included in the model due to a high level of correlation between variables which induces model bias (p < .05).
The output of the model with the best fit is displayed in . There was a trend for physicians who worked at a facility certified in the use of biologics to have higher odds of opting-in to the new treatment (p < .10).
Table 6. Binary logit model output for physicians.
Mixed multinomial logit model
The best fitting model was a MMNL with add-on to current treatment, efficacy of treating itching, efficacy of treating rashes, length of time to response, place of administration, injection site reaction, mild–moderate side effects, severe side effects and annual treatment costs as random normal parameters and a current treatment constant for patients and physicians. The current treatment constant was significant (p < .05) and negative, indicating both patients and physicians were more likely to choose the new injection treatment, all else being equal. The severity of patients considered for the physician scenarios was entered into the utility function as a nonrandom parameter. Given the extensive model output, for the readers’ convenience and ease of interpretation, the differences between patients and physicians in their preferences for the treatment attributes and levels observed in the MMNL model were simplified and displayed in (see Supplementary material Citation1 for full model output)
Table 7. Simplified MMNL model output – heterogeneity (differences) between patients and physiciansTable Footnote*.
As shown in , results from the sources of heterogeneity indicate that there was significant heterogeneity between patients and physicians in their preferences for add-on/replacement treatments, efficacy of improving the severity of rashes, length of time until response, place of administration and treatment costs (p < .05). More specifically, the efficacy of improving rashes and treatment costs was more important to physicians than patients, while the time until response was more important to patients. While patients preferred new treatments that were an add-on to current treatments, physicians preferred treatments that reduce current treatments. Patients and physicians also differed in their preferred place of administration. While patients preferred to receive treatments at clinics (administered by physicians), physicians preferred at-home treatments (self-administered by patients). All else being equal, physicians were also more likely to choose a new treatment than patients.
Attribute importance
Relative attribute importance, which demonstrates the importance of attributes to participants, was calculated using the attribute coefficients presented in Supplementary material 1. Cost was removed from the comparison as it was dominant for physicians and is based on their assumption of patients’ willingness to pay. The top three attributes of importance for patients were in rank order: (i) risk of mild side effects, (ii) time until response and (iii) efficacy of reducing itching. For physicians, the top three attributes were (i) risk of mild side effects, (ii) efficacy of reducing itching and rashes and (iii) add on or replacement treatment (see )). Differences between patients and physicians as discussed above (see MMNL section) are also shown visually in by comparing the size of the bars in the chart. As demonstrated in the figure, patients place a different value on add-on/replacement treatments, efficacy of the treatments in treating rashes, length of time until response and place of administration compared to physicians.
Predicted treatment uptake
The MMNL findings were inserted into the dashboard (see ). The dashboard can be manipulated to provide estimates of uptake of any injectable treatment compared to current treatment by patients and physicians, based on their preferences. This can be done by adjusting the individual attributes for each treatment and observing the resultant change in preference share. For example, the dashboard was set to reflect treatment attribute levels of a new biologic treatment available in Japan to estimate its uptake (shown in ). The predicted uptake for the new injection treatment described, in the context of the proportion of patients and physicians who generally opted in to treatment, is displayed in .
Figure 4. Proportion of opting-in to treatment and predicted uptake of a new injection treatment by A) patients and B) physicians.
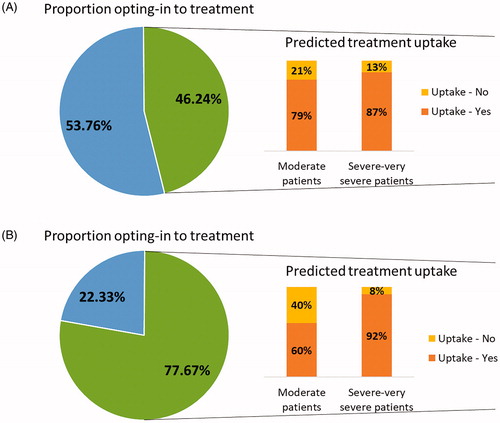
Table 8. New biologic treatment attribute levels.
Discussion
This study compared the treatment preferences of patients with AD and physicians. Overall, results suggest that while the majority of physicians would opt-in (i.e. consider the new injectable treatment as an option), only approximately half of all patients surveyed would opt-in to the new treatment. Of the participants who opt-in to treatment, there are also differences between patients and physicians in the value they put on various treatment attributes.
One key factor likely to be influencing patients’ decision to opt-out is the higher cost and the unfamiliar mode of administration of the new treatments relative to what AD patients are familiar with. Consistent with this, patients with lower needle fear and higher current treatment costs are more likely to choose the new treatment. Other patient characteristics associated with the likelihood of supporting a new treatment include poor satisfaction with the convenience of their current treatment and working full time.
In the context of the increasing development and introduction of biologic injectable treatments for AD, it is interesting that only approximately half of patients surveyed would opt-in to a new injection treatment. This has important implications not only for clinical practice, but also for the development of future treatments. Given the strong influence of needle fear and cost on patients’ preferences, physicians could seek to educate patients on the injection experience to alleviate their concerns and justify the higher treatment costs based on increased efficacy, better disease control and reduced medications in the long term. The development of future biologic treatments could also consider injection devices that are more acceptable to patients; research in diabetes shows that injection pens are associated with greater acceptance and patient adherence than syringe injections (Citation23,Citation24). Based on the patient characteristics associated with a preference for new injectable treatments, physicians can also target their recommendation of biologic treatments to patients who value convenience, e.g. full-time workers and those who are dissatisfied with their current treatment convenience.
Key differences between patients and physicians in the value they place on various treatment attributes were identified. More specifically, physicians are more likely to value the efficacy in treating rashes and are more concerned about the cost of treatment than patients. In contrast, patients prefer an add-on treatment and are against self-administering treatments at home. These disparities demonstrate that factors beyond clinical efficacy are important to both patients and physicians preferences. Further, what physicians may consider an ‘optimal’ treatment may differ from what patients may consider an ‘optimal’ treatment, highlighting the importance of understanding patient preferences for treatments in the clinical context. Increasingly, trends in research and clinical practice are emphasizing the importance of shared decision making and tailoring treatments to patients’ preferences to optimize patient participation in their care, treatment satisfaction, adherence and outcomes (Citation25–28).
DCEs are a favored approach for examining treatment preferences and allows for the study of preferences for hypothetical and new treatments that patients and physicians may not yet have exposure to. Nevertheless, there are some limitations to the methodology and the study itself. Firstly, the patients and physicians surveyed were not matched (i.e. the patient was not matched to their treating physician); thus, no inferences can be made directly about differences in preferences for specific patient–physician pairings. Further, physicians were asked to make treatment decisions for patients on an aggregate level based on disease severity. These decisions do not take into account individual differences in patient circumstances. For example, a physician may prescribe a patient with moderate AD a more potent treatment typically used in more severe patients if the psychological impact of the disease is causing significant distress to the patient.
In summary, this study elucidates the treatment preferences of patients with AD and physicians in Japan. The findings have practical clinical implications, assisting physicians in matching treatments to patients based on demographic characteristics, discussions about treatment preferences and for shared decision making. This can contribute to increased patient participation in their clinical care and ultimately their treatment outcomes.
Supplemental Material
Download MS Word (17 KB)Acknowledgements
The authors would like to acknowledge Mr. Bruno Rossi for his assistance and advice with planning the study.
Disclosure statement
Y Okubo has been a consultant, scientific advisor and/or investigator for Eli Lilly Japan K.K., Kyowa Hakko Kirin Co., Ltd, Mitsubishi Tanabe Pharma Corporation, Maruho Co., Ltd, Celgene K.K., Janssen Pharmaceutical K.K., AbbVie GK, Eisai Co., Ltd, Torii Pharmaceutical Co., Ltd, Leo Pharma and Sanofi K.K., and KAH and SF have conducted research funded by Amgen, AstraZeneca, Celgene, Janssen, Roche, Sanofi, and Shire in the past 3 years. HF, Y Oki and YT are employees of Sanofi K.K. HF and Y Oki are also stockholders of Sanofi.
Data availability statement
All data generated in this study belong to Sanofi K.K. and are available upon reasonable request.
Correction Statement
This article has been republished with minor changes. These changes do not impact the academic content of the article.
Additional information
Funding
References
- Li AW, Yin ES, Antaya RJ. Topical corticosteroid phobia in atopic dermatitis: a systematic review. JAMA Dermatol. 2017;153:1036–1042.
- Snyder A, Farhangian M, Feldman SR. A review of patient adherence to topical therapies for treatment of atopic dermatitis. Cutis. 2015;96:397–401.
- Beck LA, Thaçi D, Hamilton JD. Dupilumab treatment in adults with moderate-to-severe atopic dermatitis. N Engl J Med. . 2014;371:130–139.
- Gandhi NA, Bennett BL, Graham NMH, et al. Targeting key proximal drivers of type 2 inflammation in disease [Review Article]. Nat Rev Drug Discov. 2016;online15:35.
- Thurstone LL. A law of comparative judgment. Psychol Rev. 1927;34:273–286.
- Lancaster KJ. A new approach to consumer theory. J Polit Econ. 1966;74:132–157.
- McFadden D. Conditional logit analysis of qualitative choice behaviour. In: Zarembka P, editor. Frontiers in econometrics. New York: Academic Press; 1974. p. 105–142.
- Ryan M, Scott DA, Reeves C, et al. Eliciting public preferences for healthcare: a systematic review of techniques. Health Techonol Assess. 2001;5:1–186.
- Pfarr C, Schmid A. Schneider U. Using discrete choice experiments to understand preferences in health care. In: Levaggi R, Montefiori M, editors. Health care provision and patient mobility: health Integration in the European Union. Milano: Springer Milan; 2014. p. 27–48.
- Gutknecht M, Schaarschmidt M-L, Herrlein O, et al. A systematic review on methods used to evaluate patient preferences in psoriasis treatments. J Eur Acad Dermatol Venereol. 2016;30:1454–1464.
- Fifer S, Rose J, Hamrosi KK, et al. Valuing injection frequency and other attributes of type 2 diabetes treatments in Australia: a discrete choice experiment. BMC Health Serv Res. 2018;18:675.
- Etzkorn JR, Tuttle SD, Lim I, et al. Patients prioritize local recurrence risk over other attributes for surgical treatment of facial melanomas: results of a stated preference survey and choice-based conjoint analysis. J Am Acad Dermatol. 2018;79:210–219.e3.
- Ashcroft DM, Seston E, Griffiths C. Trade-offs between the benefits and risks of drug treatment for psoriasis: a discrete choice experiment with U.K. dermatologists. Br J Dermatol. 2006;155:1236–1241.
- Schaarschmidt M, Schmieder A, Umar N, et al. Patient preferences for psoriasis treatments: process characteristics can outweigh outcome attributes. Arch Dermatol. 2011;147:1285–1294.
- Seston EM, Ashcroft DM, Griffiths CM. Balancing the benefits and risks of drug treatment: a stated-preference, discrete choice experiment with patients with psoriasis. Arch Dermatol. 2007;143:1175–1179.
- Essers BAB, Dirksen CD, Prins MH, et al. Assessing the public’s preference for surgical treatment of primary basal cell carcinoma: a discrete-choice experiment in the south of The Netherlands. Dermatol Surg. 2010;36:1950–1955.
- Essers BAB, van Helvoort-Postulart D, Prins MH, et al. Does the inclusion of a cost attribute result in different preferences for the surgical treatment of primary basal cell carcinoma?. PharmacoEconomics. 2010;28:507–520.
- Charman CR, Venn AJ, Williams HC. The patient-oriented eczema measure: development and initial validation of a new tool for measuring atopic eczema severity from the patients’ perspective. Arch Dermatol. 2004;140:1513–1519.
- Finlay AY, Khan GK. Dermatology Life Quality Index (DLQI)-a simple practical measure for routine clinical use. Clin Exp Dermatol. 1994;19:210–216.
- Bharmal M, Payne K, Atkinson MJ, et al. Validation of an abbreviated treatment satisfaction questionnaire for medication (TSQM-9) among patients on antihypertensive medications. Health Qual Life Outcomes. 2009;7:36.
- Bridges JFP, Hauber AB, Marshall D, et al. Conjoint analysis applications in health—a checklist: a report of the ISPOR good research practices for conjoint analysis task force. Value Health. 2011;14:403–413.
- Rose JM, Bliemer MCJ. Constructing efficient states choice experimental designs. Transport Rev. 2009;29:587–617.
- Anderson BJ, Redondo MJ. What can we learn from patient-reported outcomes of insulin pen devices? J Diabetes Sci Technol. 2011;5:1563–1571.
- Hyllested-Winge J, Sparre T, Pedersen LK. NovoPen Echo(®) insulin delivery device. Med Devices (Auckl.). 2016;9:11–18.
- Perfetto EM, Oehrlein EM, Boutin M, et al. Value to whom? The patient voice in the value discussion. Value Health. 2017;20:286–291.
- Barry MJ, Edgman-Levitan S. Shared decision making-pinnacle of patient-centered care. N Engl J Med. 2012;366:780–781.
- Kiesler DJ, Auerbach SM. Optimal matches of patient preferences for information, decision-making and interpersonal behavior: evidence, models and interventions. Patient Educ Couns. 2006;61:319–341.
- Umar N, Schaarschmidt M, Schmieder A, et al. Matching physicians’ treatment recommendations to patients’ treatment preferences is associated with improvement in treatment satisfaction. J Eur Acad Dermatol Venereol. 2013;27:763–770.