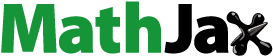
ABSTRACT
Temperature critically affects the performance of entomopathogenic fungi (EPF). Mathematical models are critical tools used in predictive microbiology but are less adopted for EPF. We selected eight nonlinear models to describe thermal biology; minimum (Tmin), optimal (Topt) and maximum (Tmax) thresholds; and maximal growth (Pmax) of EPF. Conidial germination and mycelial growth of Metarhizium anisopliae (ICIPE 7, ICIPE 20, ICIPE 62, ICIPE 69, ICIPE 78) and Beauveria bassiana (ICIPE 284) isolates incubated at 12, 16, 20, 24, 28, 32 and 36°C were measured and fitted to the models. The models were compared using the Akaike information criterion (AIC) and adjusted R2. The best–fitting models for germination of the isolates were the cardinal temperature model with inflection (CTMI), Ratkowsky 3 and the generalised β function, while the best–fitting models for growth were CTMI, Ratkowsky 3, Lactin 1 and generalised β function. Brière 1, Brière 2, Ratkowsky 2, and Van Der Heide least fitted most germination and growth datasets. Tmin, Topt, Tmax and Pmax ranged from 13.3–13.6°C, 26.3–28.1°C, 35.7–36.3°C and 95.4–100.0% for germination, and 3.7–13.7°C, 25.9–28.6°C, 35.4–37.2°C and 1.44–2.34 mm day–1 for growth, respectively. Topt were below temperatures of central bee brood areas and partly mirrored temperatures of the isolates’ regions of origin. The best–fitting models can be used to better match EPF with different regions’ temperatures for optimal performance against target pests.
Introduction
Entomopathogenic fungi (EPF) are increasingly promoted as alternatives to chemical pesticides in pest management practices (Abdelghany, Citation2015). In Africa, Metarhizium anisopliae (ICIPE 7, ICIPE 62, ICIPE 69 and ICIPE 78) constitute commercialised isolates against pests such as fruit flies, aphids, mealybugs, mites and ticks, while M. anisopliae ICIPE 20 and Beauveria bassiana ICIPE 284 isolates are about to be commercialised for management of fruit flies, the fall armyworm Spodoptera frugiperda Smith, the tomato leafminer Tuta absoluta Meyrick and the pea leafminer Liriomyza huidobrensis Blanchard (Akutse et al., Citation2020).
Products based on EPF are derived from isolates originating from different locations or hosts (Fargues et al., Citation1997; Ouedraogo et al., Citation1997). EPF may kill susceptible insects through contact toxicity. Under adequate temperature conditions, conidia germinate and penetrate the insect cuticle, and consequently develop into hyphae and mycelia that colonise the insect body (Mora et al., Citation2017). Temperature is, therefore, a critical factor determining the efficacy of EPF (Mora et al., Citation2017; Tumuhaise et al., Citation2018). Notably, most EPF are capable of growth at diverse temperatures different from those matching the geo–climatic conditions of their regions of origins (Davidson et al., Citation2003; Fargues et al., Citation1997; Rangel et al., Citation2005). The ability of EPF to remain viable and grow not only under conditions of the target (insect pests) but also under conditions of non–targets (e.g. insect pollinators) should be considered as part of the selection criteria of promising EPF candidates. In the advent of future registration and release in several countries, it is crucial to determine the optimum temperatures of EPF to optimise their efficacy and safety on non–target insects such as pollinators.
Best–fitting mathematical models have been suggested as an effective tool to describe and predict the growth of EPF. Numerous nonlinear models with varying complexity have previously been used to forecast the effect of different biophysical conditions, especially temperature, on microbial growth, with a bias towards bacterial species (Huang et al., Citation2011; Rosso et al., Citation1995; Zwietering et al., Citation1991). They include, among other models, the cardinal temperature model with inflection (CTMI) (Rosso et al., Citation1995), the square root Ratkowsky 2 (Ratkowsky et al., Citation1983), the modified Ratkowsky 3 (Zwietering et al., Citation1991), Lactin 1 (Lactin et al., Citation1995), Brière 1 and Brière 2 models (Briere et al., Citation1999). The Van Der Heide model (Van Der Heide et al., Citation2006) is a recent, simple nonlinear model developed to describe the growth of free–floating macrophytes as a function of temperature. The generalised β function was modified by Bassanezi et al. (Citation1998) to model the influence of temperature on the monocyclic components of the bean rust Uromyces appendiculatus Persoon and angular leaf spot Phaeoisariopsis griseola (Sacc.) Ferraris infecting bean Phaseolus vulgaris L. cultivars.
Comparatively few models have been used to characterise the growth of fungi under different temperature conditions, with more attention being given to food spoilage and mycotoxigenic fungi (Dantigny et al., Citation2011; Gougouli & Koutsoumanis, Citation2012, Citation2013; Peleg & Normand, Citation2013) than to EPF (Davidson et al., Citation2003; Fargues et al., Citation1997; Smits et al., Citation2003). However, studies using predictive models have shown that whenever fungi are maintained at specified temperature regimes, their conidial germination or mycelial growth is curvilinearly heterogenous or homogenous, and this is attributable to their thermal requirements (Fargues et al., Citation1997; Gougouli & Koutsoumanis, Citation2013). Conidial germination and mycelial growth are response metrics widely used in assessing inter– and intraspecific variations in thermal biology for EPF such as M. anisopliae and B. bassiana (Fargues et al., Citation1997; Ouedraogo et al., Citation1997).
Selection of Brière 1, Brière 2, CTMI, Lactin 1, Ratkowsky 2, Ratkowsky 3, Van Der Heide and the generalised β function as predictive models for this study was based on their ability to quantitatively describe at least three biologically meaningful and physically interpretable parameters including cardinal (minimum, optimum and maximum) temperatures, and maximal germination and growth of microbes. Models such as Brière 1, Brière 2 and Ratkowsky 3 have previously been used to model the effect of temperature on mycelial growth of the EPF M. anisopliae, B. bassiana and Paecilomyces fumosoroseus (Smits et al., Citation2003). Similarly, the generalised β function has been adopted to describe thermal requirements for mycelial growth of various EPF including M. anisopliae and B. bassiana isolates (García–Fernández et al., Citation2008; Quesada-Moraga et al., Citation2006). However, CTMI, Lactin 1, Ratkowsky 2 and Van Der Heide models have not yet been tested on EPF. The ability to accurately predict germination and/or growth of EPF isolates may differ among the models, requiring testing several predictive models on multiple EPF isolates to provide a comparatively best–fitting model or models.
Therefore, our study aimed at modelling the influence of wide ranges of temperatures on conidial germination and mycelial growth of five isolates of M. anisopliae (ICIPE 7, ICIPE 20, ICIPE 62, ICIPE 69 and ICIPE 78) and one isolate of B. bassiana (ICIPE 284). We selected these isolates based on their current and projected commercial use as microbial agents against insect pests occurring in Africa and elsewhere (Akutse et al., Citation2020). Our study focused on selecting and validating nonlinear models to describe and predict conidial germination and mycelial growth as a function of temperature. We also focused on establishing the best–fitting nonlinear models for conidial germination and mycelial growth as a function of temperature and determining the cardinal thermal thresholds for each EPF isolate.
Materials and methods
Nonlinear models for the study
Equations of eight nonlinear models used to describe the effect of temperatures on conidial germination and mycelial growth of EPF isolates are illustrated as follows:
Brière 1 (Briere et al., Citation1999):
(1)
(1)
(2)
(2) Brière 2 (Briere et al., Citation1999):
(3)
(3)
(4)
(4) Ratkowsky 2 (Ratkowsky et al., Citation1983):
(5)
(5)
(6)
(6) Ratkowsky 3 (Zwietering et al., Citation1991):
(7)
(7)
(8)
(8) Lactin 1 (Lactin et al., Citation1995):
(9)
(9)
(10)
(10) Van Der Heide (Van Der Heide et al., Citation2006):
(11)
(11)
(12)
(12) CTMI (Rosso et al., Citation1995):
(13)
(13) Generalised β function (Bassanezi et al., Citation1998):
(14)
(14) In these models, µm represents conidial germination (%) or mycelial growth (mm day–1) at a specific temperature (T). Tmin, Topt and Tmax are the theoretical minimum, optimum and maximum temperature, respectively. Pmax is a specific maximal growth response at Topt, which is also equivalent to parameter µopt in the equation (13) and TYopt in equation (14). Equations with asterisks (*) are derivations to reparametrize the original models through mathematical substitution of redundant parameters to provide additional parameters Topt and Pmax (Adams et al., Citation2017). In equations (1) and (3), a is the empirical constant while b in equation (3) is a parameter describing the shape of the nonlinear curve (Briere et al., Citation1999). In equations (5) and (7), parameters b and c are the so–called Ratkowsky parameters °C–1 h–0.5 and °C–1, respectively, where b represents the regression coefficient of the square root of the growth rate and c is a curve–fitting parameter (Ratkowsky et al., Citation1983). In equation (14), TB3 is a shape parameter. Parameter a in equations (9) and (11) is an empirical constant.
Fungal isolates
Six fungal isolates for this study were obtained from the International Centre of Insect Physiology and Ecology (icipe), Nairobi, Kenya (). Before the study, the fungal isolates had been preserved in liquid nitrogen vapour as stock cultures. Each fungal isolate was revived by passage in 7th instar larvae of the susceptible insect host Galleria mellonella L. The larvae were injected with 5 µL water containing about 5,000 conidia. After 7 days of incubation at 25 ± 2°C in the dark, conidia from sporulating cadavers were harvested and inoculated on routine fungal cultivation media in 95 mm (diameter) × 15 mm (height) plastic Petri dishes. Metarhizium anisopliae and B. bassiana were inoculated on Sabouraud dextrose agar (SDA) (Oxoid, Hampshire, UK) and potato dextrose agar (PDA) (Oxoid) according to Ibrahim et al. (Citation2002) and Dale and Shinde (Citation2017), respectively. Before dispensing, 0.25 g L–1 of a selective antimicrobial agent (streptomycin sulphate) was added to the media to restrict the potential growth of other microbes.
Table 1. Characteristics of entomopathogenic fungal isolates that were evaluated for conidial germination and mycelial growth as a function of temperature.
After 3 weeks of incubation at 25 ± 2°C in the dark, conidia were harvested by flooding the fungal culture with 10 mL sterile 0.05% Triton–X–100 (Triton, Darmstadt, Germany). The resulting suspension was transferred into a 25 mL universal bottle containing 4 sterile small glass beads (1–3 mm diameter) and vortexed for 3 min at 700 rpm to form a homogenous suspension. Concentrations of stock suspensions were determined by serial dilution (10–2) and conidia present in the dilutions were microscopically enumerated using an improved Neubauer Hemocytometer (Marienfeld Germany, Lauda–Königshofen, Germany).
Assessment of in vitro conidial germination
A suspension of 3 × 106 conidia mL–1 was prepared by adjusting stock suspension with sterile 0.05% Triton–X–100 and then an aliquot (0.1 mL) was spread–plated on SDA (for M. anisopliae) or PDA (for B. bassiana) in Petri dishes. Inoculated Petri dishes were sealed with parafilm and incubated in dark at constant temperatures; 12, 16, 20, 24, 28, 32 and 36°C. Five replications were made for each combination of fungal isolate and temperature. Conidial germination was stained at 18 h post–inoculation by flooding with 2 mL of lactophenol blue. Four microscope coverslips were placed on the culture surface and germination assessed by randomly counting 100 conidia under each coverslip using a light microscope (× 400 magnification). Only conidia with a germination tube longer than its width were considered to have germinated.
Assessment of in vitro mycelial growth
About 0.1 mL of suspension (3 × 106 conidia mL–1) was spread–plated on SDA (for M. anisopliae) or PDA (for B. bassiana) in 95 mm (diameter) × 15 mm (height) plastic Petri dishes. Petri dishes were sealed and incubated in dark at 25 ± 2°C for 3 days. Petri dishes containing fresh media were prepared, and two cardinal lines intersecting perpendicularly at the centre were drawn at the bottom. A cylindrical media plug from the centre of the Petri dish was cut with a sterile 8–mm–diameter cork borer and replaced with cylindrical plugs cut from a 3–day old mycelial mat. Inoculated Petri dishes were sealed and incubated at constant temperatures; 12, 16, 20, 24, 28, 32 and 36°C for 15 days in the dark. Five replicates of EPF isolate–temperature combinations were prepared. Mycelial growth was measured radially at 24 h intervals along the two previously drawn cardinal lines. Radial growth for each EPF isolate was plotted against time and fitted to a linear regression model () and absolute mycelial growth (slope; mm day–1) obtained using Microsoft Excel 2016 for subsequent analyses.
Statistical analyses
Conidial germination and mycelial growth were averaged across the five replicates of each EPF isolate and fitted to the nonlinear models using R software version 4.0.2 (R Core Team, Citation2020). For each EPF isolate, the start values for the parameters to achieve convergence tolerance of the model were based on hypothetical estimations. We used the nls function for datasets with non–zero residual sum of squares and the nlsLM function from the minpack.lm package (Elzhov et al., Citation2016) for datasets with zero residual sum of squares to provide weighted least–square estimates of nonlinear model’s parameters. Tmin for the generalised β function was fixed at 8°C, a standard minimum temperature for mycelial growth of most fungal isolates from the tropics (Bayissa et al., Citation2017; Fargues et al., Citation1997; Ouedraogo et al., Citation1997; Smits et al., Citation2003). Based on our datasets, none of the tested isolates germinated at 12°C and we, therefore, fixed Tmin in the generalised β function to 12°C for conidial germination. We compared the fitted models using the goodness–of–fit criteria: AIC and adjusted R2 (adj. R2). R–squared (R2) was obtained by estimating the models’ accuracy using the rcompanion package (Mangiafico, Citation2020). Adj. R2 accounted for the degrees of freedom in the fitted models and was computed from pseudo R2 using the expression provided by Kvålseth (Citation1985):
(15)
(15) where n and k were the numbers of observations in dataset and model parameters, respectively. The model with the smallest AIC and the highest adj. R2 was considered best–fitting. Equally best–fitting models were established statistically according to Vuong’s (Citation1989) non-nested likelihood ratio test implemented in the nonnest2 package (Merkle & You, Citation2020). Whenever the generalised β function was ranked as the best–fitting based on the goodness–of–fit criteria, the second–best model was selected for the likelihood ratio test, because the generalised β function was restricted to predict only three biologically significant parameters: Topt, Tmax and TYopt. The results of statistical estimates for the parameters were presented as unweighted means.
Results
Comparison of the models for conidial germination and mycelial growth
All models provided statistical estimates for the parameters when fitted with conidial germination and mycelial growth datasets of EPF isolates. Curves plotted from fitted models’ predicted values for conidial germination and mycelial growth of EPF isolates against temperature showed obvious nonlinear patterns. Conidial germination for all EPF isolates started from around 10–14°C and reached a peak at near 25–30°C, followed by a precipitous decline reaching upper thresholds near 35–38°C (). Mycelial growth for all EPF isolates started from around 0–14°C with a peak at near 25–30°C, followed by a quick decline towards upper thresholds near 35–42°C ().
Figure 1. Graphical comparison of temperature–dependent models describing conidial germination of the Metarhizium anisopliae (ICIPE 7, ICIPE 20, ICIPE 62, ICIPE 69, ICIPE 78) and Beauveria bassiana (ICIPE 284) isolates. CTMI = cardinal temperature model with inflection.
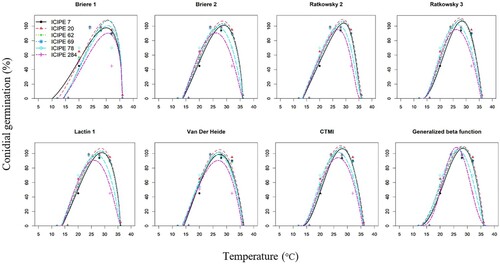
Figure 2. Graphical comparison of temperature–dependent models describing mycelial growth of the Metarhizium anisopliae (ICIPE 7, ICIPE 20, ICIPE 62, ICIPE 69 and ICIPE 78) and Beauveria bassiana (ICIPE 284) isolates. CTMI = cardinal temperature model with inflection.
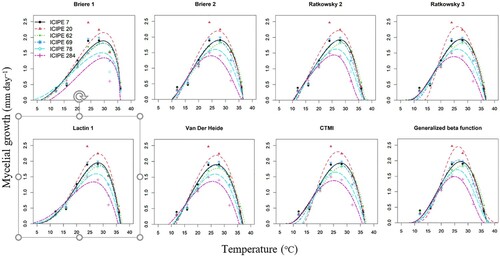
Statistics of goodness–of–fit of models describing the relationship of temperature with conidial germination and mycelial growth of the EPF isolates are presented in and , respectively. Generally, our results show that the models varied across the EPF isolates for conidial germination and mycelial growth in terms of goodness–of–fit.
Table 2. Goodness–of–fit metrics for the models describing the effect of temperature on conidial germination of the Metarhizium anisopliae (ICIPE 7, ICIPE 20, ICIPE 62, ICIPE 69, ICIPE 78) and Beauveria bassiana (ICIPE 284) isolates.
Table 3. Goodness–of–fit metrics for the models describing the effect of temperature on mycelial growth of the Metarhizium anisopliae (ICIPE 7, ICIPE 20, ICIPE 62, ICIPE 69, ICIPE 78) and Beauveria bassiana (ICIPE 284) isolates.
Based on adjusted R2 and AIC, we selected single best–fitting models and based on the likelihood ratio test, we established equally best–fitting models for conidial germination and mycelial growth of EPF isolates. Statistics of equally best–fitting and the least–fitting models for conidial germination and mycelial growth are presented in and , respectively.
Table 4. Comparison between the best–fitting model and other models for conidial germination of the Metarhizium anisopliae (ICIPE 7, ICIPE 20, ICIPE 62, ICIPE 69, ICIPE 78) and Beauveria bassiana (ICIPE 284) isolates.
Table 5. Comparison between the best–fitting model and other models for mycelial growth of the Metarhizium anisopliae (ICIPE 7, ICIPE 20, ICIPE 62, ICIPE 69, ICIPE 78) and Beauveria bassiana (ICIPE 284) isolates.
For conidial germination, CTMI was the best–fitting model for ICIPE 7, ICIPE 20, ICIPE 62 and ICIPE 69 while Ratkowsky 3 was the best–fitting model for ICIPE 78 and ICIPE 284. Equally best–fitting models were CTMI (for ICIPE 284), Ratkowsky 3 (for ICIPE 7, ICIPE 20, ICIPE 62 and ICIPE 69), Ratkowsky 2 (for ICIPE 20 and ICIPE 284), Brière 2 (for ICIPE 7 and ICIPE 20), Lactin 1 (for ICIPE 7 and ICIPE 20), the generalised beta function (for ICIPE 20, ICIPE 78 and ICIPE 284) and Van Der Heide (for ICIPE 20).
For mycelial growth, CTMI was the best–fitting model for ICIPE 7 and ICIPE 78, Ratkowsky 2 was the best–fitting model for ICIPE 69 and ICIPE 284, while Lactin 1 was the best–fitting model for ICIPE 62. However, we also established equally best–fitting models for mycelial growth of ICIPE 7 (Lactin 1, Ratkowsky 3 and the generalised β function), ICIPE 20 (CTMI, Lactin 1 and the generalised β function), ICIPE 62 (CTMI, Ratkowsky 3 and the generalised β function), ICIPE 69 (Lactin 1, Ratkowsky 3 and generalised β function) and ICIPE 78 (Lactin 1, Ratkowsky 2, Ratkowsky 3, Brière 2 and generalised β function). Except for above mentioned best–fitting and other fitting models for each isolate, the other models poorly described conidial germination and/or mycelia growth of the isolates as a function of temperature.
Cardinal estimates for conidial germination and mycelial growth
The extreme thermal range for conidial germination of all the EPF isolates was 7.5–38.3°C, while the range for optima was 26.0–30.4°C and maximal conidial germination was 86.8–100.0% (). On the other hand, mycelial growth of EPF isolates occurred between –2.6–42.3°C and the optima occurred between 24.6–29.9°C with maximal mycelial growth rates ranging between 1.22–2.45 mm day−1 (). Unlike the other models, Brière 1 had relatively low Tmin and the highest Topt estimates for conidial germination (10.8–13.1°C and 30.2–30.5°C, respectively), and mycelial growth (–1.6–7.5°C and 28.1–29.9°C, respectively). Additionally, Brière 1 had the shortest range between Topt and Tmax (conidial germination: 30.36.0°C, mycelial growth: 29.9–36.3°C) indicating a sharp decline in the rates of conidial germination and mycelial growth at temperatures above the optima. Brière 1 also gave similar Tmax estimates for conidial germination (36.0°) and mycelial growth (36.1°C) across the EPF isolates except for mycelial growth of ICIPE 62 (36.3°) and ICIPE 284 (36.0°).
Table 6. Cardinal estimates from models for conidial germination of the Metarhizium anisopliae (ICIPE 7, ICIPE 20, ICIPE 62, ICIPE 69, ICIPE 78) and Beauveria bassiana (ICIPE 284) isolates.
Table 7. Cardinal estimates from models for mycelial growth of the Metarhizium anisopliae (ICIPE 7, ICIPE 20, ICIPE 62, ICIPE 69, ICIPE 78) and Beauveria bassiana (ICIPE 284) isolates.
Tmin estimates by CTMI, Ratkowsky 2, Ratkowsky 3 and Brière 2 were relatively close (difference: ≤ 0.3°C) for conidial germination of all isolates and mycelial growth of most of the isolates. Comparatively, Lactin 1 had the lowest Tmin estimates across all isolates for conidial germination (7.5–11.6°C) and mycelial growth (–2.6–4.6°C), while Van De Heide had the highest Tmin estimates across all isolates for conidial germination (13.5–14.3°C) and mycelial growth (8.6–11.8°C). Except for Brière 1, Topt and Tmax estimates for the isolates did not show obvious trends. Pmax estimates also varied across all isolates but with no obvious trends among the models for conidial germination and mycelial growth.
The generalised β function was fairly symmetrical, indicating the rate of conidial germination or mycelial growth before and after the point of inflection was inversely equal. Topt estimates predicted by the generalised β function were relatively close to those predicted by CTMI and Ratkowsky 3 for conidial germination (difference: ≤ 0.4°C) and mycelial growth (difference: ≤ 0.5°C) for most of the isolates after fixing Tmin to 12°C (for conidial germination) and 8°C (for mycelial growth). Unlike the other models, Tmax estimates for the generalised β function were the highest for conidial germination and mycelial growth of some isolates; Tmax estimates for conidial germination of ICIPE 69 and ICIPE 284 were 37.0°C and 37.9°C, respectively, and for mycelial growth of ICIPE 20, ICIPE 69 and ICIPE 78 were 42.3°C, 38.7°C and 37.8°C, respectively.
Estimates of the single best–fitting model for each EPF isolate are reported here. Estimated Topt for conidial germination of ICIPE 7, ICIPE 20, ICIPE 62, ICIPE 69, ICIPE 78 and ICIPE 284 were 28.1, 27.6, 27.7, 27.9, 26.4 and 26.3°C, respectively, with corresponding Pmax for conidial germination at 100.0, 100.0, 100.0, 100.0, 100.0 and 95.4%, respectively.
Estimated Topt for mycelial growth of ICIPE 7, ICIPE 20, ICIPE 62, ICIPE 69, ICIPE 78 and ICIPE 284 were 27.6, 26.3, 28.6, 27.7, 26.5 and 25.9°C, respectively. At the optimum temperatures, the fastest growth rate (Pmax) was as follows: ICIPE 20 (2.34 mm day–1) > ICIPE 69 (2.00 mm day–1) > ICIPE 7 (1.94 mm day–1) > ICIPE 62 (1.85 mm day–1) > ICIPE 78 (1.65 mm day–1) > ICIPE 284 (1.44 mm day–1).
Temperature ranges of ICIPE 7, ICIPE 20, ICIPE 62, ICIPE 69, ICIPE 78 and ICIPE 284 required for conidial germination (13.6–36.0°C, 13.3–36.3°C, 13.4–36.1°C, 13.4–36.0°C, 13.4–36.0°C, and 13.6–35.7°C, respectively) were relatively shorter compared to those required for mycelial growth (8.0–36.8°C, 13.7–37.0°C, 3.7–37.2°C, 11.1–35.2°C, 13.0–36.7°C and 10.1–35.4°C, respectively).
Discussion
While it is well known that virulence is mostly used as a selection criterion of promising EPF candidates (Niassy et al., Citation2012; Onsongo et al., Citation2019), temperature remain a critical factor that may affect germination, growth, and consequently the infectivity of these fungi under conditions of the target (insect pests) and non–target (insect pollinators) population. This study shows that temperature has a characteristic nonlinear effect on the germination and growth of EPF, which is generally skewed to lower temperature thresholds and with a rapid decline from optima to upper–temperature thresholds. We found that the best–fitting models varied across the EPF isolates in describing the effect of temperature on conidial germination and mycelial growth. The variation indicates that a single model cannot adequately predict the growth of multiple EPF isolates. Also, the estimates of Tmin, Topt and Tmax for the EPF isolates varied depending on the models. We observed about 75% of Pmax estimates for conidial germination of the EPF isolates were 100% as predicted by the models, while Pmax estimates for mycelial growth of EPF varied across the isolates and the models. With fitted conidial germination datasets, the models often overestimated Pmax, by giving values that were slightly above 100%. We truncated these values to 100% and this may constitute a drawback of using the nonlinear model to predict Pmax for conidial germination.
The goodness–of–fit of the models may vary based on the number of model parameters, fungal species and isolates, and the number of observations in datasets fitted to the models. For instance, models with fewer parameters tend to have high fitness and vice versa (Zwietering et al., Citation1991). Whenever adjusted R2 is used as a basis of model comparison, the numbers of observations in datasets and model parameters based on the equation (15) may affect models’ variances. For example, the degree of explained variance (adjusted R2) decreases with the number of observations of datasets fitted to a model. Different fungal species and isolates have different thermal biology (Fargues et al., Citation1997; Ouedraogo et al., Citation1997) and can be best described by certain models (Davidson et al., Citation2003; Smits et al., Citation2003). Therefore, the suitability of models may vary based on these observations.
Statistically, the best–fitting models for conidial germination of the tested EPF isolates were CTMI and Ratkowsky 3 for all isolates. The best–fitting models for mycelial growth were CTMI, Ratkowsky 3, Lactin 1 and generalised β function for M. anisopliae isolates, and Ratkowsky 2, Van De Heide and generalised β function for the B. bassiana isolate. Other models, which could be selected as alternative predictive tools, showed some fitness specificity to the isolates. Brière 1 least fitted conidial germination and mycelial growth of all isolates. Brière 2 and Van De Heide least fitted mycelial growth datasets.
The generalised β function was used by Quesada-Moraga et al. (Citation2006) and García–Fernández et al. (Citation2008) as a temperature–dependent model to predict optimum temperatures, maximum temperatures and maximal growth rates of EPF. According to the authors, this model provided good predictions for the thermal biology of M. anisopliae and B. bassiana isolates based on unadjusted R2 values and standard errors of the parameters. In these studies, the model’s Tmin was fixed to a standard minimum temperature (5°C) for fungal isolates in temperate regions. In our study, this model was compared to other temperature–dependent models and we found it to be the best–fitting model for conidial germination of some isolates (ICIPE 20, ICIPE 78 and ICIPE 284) when Tmin was fixed to 12°C and mycelial growth of all isolates when Tmin was fixed to 8°C.
According to Zwietering et al. (Citation1991), Ratkowsky 3 (a modified form of Ratkowsky 2) was a comparatively suitable model to describe the effect of temperature on bacterial growth. Despite Ratkowsky 3 being a suitable model for conidial germination and mycelial growth of all tested isolates, we also consider Ratkowsky 2 as a suitable model to describe the effect of temperature on some isolates (conidial germination: ICIPE 20, ICIPE 284, and mycelial growth: ICIPE 69 ICIPE 78, and ICIPE 284).
As previously reported by Smits et al. (Citation2003), Brière 2 and Ratkowsky 3 were the best models to describe the effect of temperature on two isolates of M. anisopliae and two isolates of M. flavoviride, while Brière 1 was comparatively the least–fitting model. However, unlike Ratkowsky 3, Brière 2 was the least–fitting model for most of the tested isolates. Additionally, Smits et al. (Citation2003) lacked a statistical method to estimate Topt and Pmax for the Ratkowsky 3 model. In our study, all the models except CTMI and the generalised β function were reparametrized to provide additional parameters (Topt and Pmax). However, additional parameters may have contributed to a less degree of fitness (Zwietering et al., Citation1991). Brière 1, Lactin 1, Van Der Heide and CTMI models each have four parameters while Brière 2, Ratkowsky 2, Ratkowsky 3 and the generalised β function each have five parameters. Despite relatively fewer parameters, Brière 1 consistently least fitted most conidial germination and mycelial growth datasets.
Conidial germination is a critical initial step during the infection process of EPF. To our knowledge, the effect of temperature on conidial germination of EPF has never been modelled using herein described nonlinear models. We found isolates of M. anisopliae to have Tmin, Topt and Tmax for conidial germination with a range of 13.3–13.6°C, 26.4–28.1°C and 36.0–36.3°C, respectively, and with Pmax of 100%, while B. bassiana isolate had Tmin, Topt, Tmax and Pmax for conidial germination of 13.6°C, 26.3°C, 35.7°C and 95.4%, respectively. We established that M. anisopliae ICIPE 20 was more thermotolerant for conidial germination followed by ICIPE 62, ICIPE 69, ICIPE 78 and ICIPE 20, while the B. bassiana isolate ICIPE 284 was the least thermotolerant.
To cause infection to a susceptible insect, germinated conidia have to actively grow into hyphae and form mycelia, which are also affected by temperature. Davidson et al. (Citation2003) showed that the Schoolfield (nonlinear Arrhenius) temperature model predicted the mycelial growth of six M. anisopliae isolates to have Topt and Pmax ranging between 25.6–28.3°C and 1.40–2.39 mm day–1, respectively, while growth of three B. bassiana isolates to have Topt and Pmax ranging between 26.2–29.8°C and 1.24–1.45 mm day–1, respectively. However, their study did not provide thermal thresholds for the fungi. In particular, mycelial growth of ICIPE 62 and ICIPE 69 have previously been reported to have Tmin, Topt and Tmax of 8.7–10.0°C, 29.1–29.3°C and 35.1°C, respectively, according to the Brière 1 model (Bayissa et al., Citation2017). Our study reports that M. anisopliae isolates had Tmin, Topt and Tmax for mycelial growth ranging between 3.7–13.7°C, 26.3–28.6°C and 35.2–37.2°C, respectively, and Pmax ranging between 1.65–2.34 mm day–1. We found that B. bassiana ICIPE 284 had the shortest thermal range for mycelial growth, and lowest Tmin (10.1°C), Topt (25.9°C), Tmax (35.4°C) and Pmax (1.44 mm day–1). For optimal mycelial growth, ICIPE 62 followed by ICIPE 20, ICIPE 7, ICIPE 78 and ICIPE 69 were more thermotolerant than ICIPE 284.
Across the EPF isolates, Topt for conidial germination were comparatively higher than Topt for mycelial growth, and this can be ascribed to the high requirement of activation energy for germination. Conidial germination of M. anisopliae and B. Bassiana isolates were comparatively less tolerant to low temperatures than mycelial growth, and this observation is consistent with previous studies (Bayissa et al., Citation2017; Ekesi et al., Citation1999; Tefera & Pringle, Citation2003). Although the tested EPF are mesophilic, specific thermal requirements for germination and growth varied among their isolates, and this can be ascribed to regions of origin. For example, M. anisopliae isolates used in this study originated from tropical regions, e.g. Kenya and DR Congo. The temperature ranges reported here match tropical conditions and, therefore, this may explain why most commercial EPF isolates in tropical countries are based on M. anisopliae. The tested isolate of B. bassiana originated from Mauritius and, therefore, its thermal biology as described here matches the temperatures commonly found in a mild tropical maritime climate.
The growth responses of EPF are best described at optimum temperatures where the fungi have optimal performance (Davidson et al., Citation2003). Our results indicate that all tested EPF isolates germinated optimally around 26.6–28.1°C and grew optimally around 25.9–28.1°C. These conditions are suitable for the occurrence and development of most insect pests found in tropical regions (Lehmann et al., Citation2020) and, therefore, these EPF can be successfully used to manage pests occurring in these geo–climatic conditions. However, pollinators such as the Western honey bee (Apis mellifera L.) or stingless honey bees such as Trigona denoiti Vachal visiting crops treated with EPF isolates during pest management practices could come into contact with conidia and return with them to their hives. Though, the conidia's ability to germinate and grow in central bee brood areas is unlikely to occur owing to highly thermoregulated hive conditions (Jarimi et al., Citation2020; Ramli et al., Citation2017). The optimal temperatures provided in our study do not match those of central brood areas of A. mellifera (32.0–36.0°C) or T. denoiti (31.0–32.0°C), although the upper–temperature thresholds where the growth of the EPF was nearly zero lies within ranges of conditions in central brood areas of these bee colonies (Fletcher & Crewe, Citation1981; Jarimi et al., Citation2020). Therefore, further research may be required to determine if the EPF can operate and negatively affect bee colonies under in vivo conditions.
Therefore, the best–fitting models can be routinely used to quantify and predict the growth of the EPF isolates during screening and re–evaluation for application as biocontrol candidates in regions with different temperatures. We also established the differences in cardinal temperatures between conidial germination and mycelial growth, which can be ascribed to the energy required for these growth responses. The thermal biology for the tested isolates partly mirrors the temperatures found in the regions of their origins. The study quantitatively describes the temperature requirements of several commercialised EPF isolates, which can be used as the basis for pest management and protection of non–target insects across different temperature conditions. However, further experiments using the predictive models should take into consideration the environmental fluctuating temperatures and thermoregulation capabilities of the target and non–target insects and other environmental factors such as humidity, pH and solar radiations that may determine their relationship with the viability, growth and infectivity of the EPF isolates.
Supplementary materials for this article can be found online and they include R software codes for the eight models provided at https://doi.org/10.6084/m9.figshare.16645231 and datasets provided at https://doi.org/10.6084/m9.figshare.16645774.
Acknowledgements
This work received financial support from the German Federal Ministry for Economic Cooperation and Development (BMZ) commissioned and administered through the Deutsche Gesellschaft für Internationale Zusammenarbeit (GIZ); Fund for International Agricultural Research (FIA), grant number 17.7860.4–001; Norwegian Agency for Development Cooperation, the Section for Research, Innovation, and Higher Education grant number RAF–3058 KEN–18/0005; UK’s Foreign, Commonwealth and Development Office (FCDO); the Swedish International Development Cooperation Agency (Sida); the Swiss Agency for Development and Cooperation (SDC); the Federal Democratic Republic of Ethiopia; and the Government of the Republic of Kenya. The funders had no role in the designs of the study; in data collection, analyses, or interpretation; in writing of the manuscript, or in the decision to publish the results.
Disclosure statement
No potential conflict of interest was reported by the author(s).
Additional information
Funding
References
- Abdelghany, T. M. (2015). Entomopathogenic fungi and their role in biological control. (1st ed.). OMICS Group. https://doi.org/https://doi.org/10.4172/978-1-63278-065-2-66.
- Adams, M. P., Collier, C. J., Uthicke, S., Ow, Y. X., Langlois, L., & O’Brien, K. R. (2017). Model fit versus biological relevance: Evaluating photosynthesis-temperature models for three tropical seagrass species. Scientific Reports, 7(1), 39930. https://doi.org/https://doi.org/10.1038/srep39930
- Akutse, K. S., Subramanian, S., Maniania, N. K., & Dubois, T. (2020). Biopesticide research and product development in Africa for sustainable agriculture and food security – experiences from the International Centre of Insect Physiology and Ecology (icipe). Frontiers in Sustainable Food Systems, 4, 563016. https://doi.org/https://doi.org/10.3389/fsufs.2020.563016
- Bassanezi, R. B., Amorim, L., Filho, A. B., & Hau, B. (1998). Effects of bean line pattern mosaic virus on the monocyclic components of rust and angular leaf spot of Phaseolus bean at different temperatures. Plant Pathology, 47(3), 289–298. https://doi.org/https://doi.org/10.1046/j.1365-3059.1998.00239.x
- Bayissa, W., Ekesi, S., Mohamed, S. A., Kaaya, G. P., Wagacha, J. M., Hanna, R., & Maniania, N. K. (2017). Selection of fungal isolates for virulence against three aphid pest species of crucifers and okra. Journal of Pest Science, 90(1), 355–368. https://doi.org/https://doi.org/10.1007/s10340-016-0781-4
- Briere, J. F., Pracros, P., Le Roux, A. Y., & Pierre, J. S. (1999). A novel rate model of temperature-dependent development for arthropods. Environmental Entomology, 28(1), 22–29. https://doi.org/https://doi.org/10.1093/ee/28.1.22
- Dale, N. S., & Shinde, S. S. (2017). Growth, sporulation and biomass production of native entomopathogenic fungi Beauveria bassiana (balsamo) vuillemin on a suitable medium. International Journal of Entomology Research, 2(5), 93–98.
- Dantigny, P., Nanguy, S. P. M., Judet-Correia, D., & Bensoussan, M. (2011). A new model for germination of fungi. International Journal of Food Microbiology, 146(2), 176–181. https://doi.org/https://doi.org/10.1016/j.ijfoodmicro.2011.02.022
- Davidson, G., Phelps, K., Sunderland, K. D., Pell, J. K., Ball, B. V., Shaw, K. E., & Chandler, D. (2003). Study of temperature–growth interactions of entomopathogenic fungi with potential for control of Varroa destructor (acari: Mesostigmata) using a nonlinear model of poikilotherm development. Journal of Applied Microbiology, 94(5), 816–825. https://doi.org/https://doi.org/10.1046/j.1365-2672.2003.01871.x
- Ekesi, S., Maniania, N. K., & Ampong-Nyarko, K. (1999). Effect of temperature on germination, radial growth and virulence of Metarhizium anisopliae and Beauveria bassiana on megalurothrips sjostedti. Biocontrol Science and Technology, 9(2), 177–185. https://doi.org/https://doi.org/10.1080/09583159929767
- Elzhov, T. V., Mullen, K. M., Spiess, A. N., & Bolker, B. (2016). Minpack.lm: R interface to the levenberg-marquardt nonlinear least–squares algorithm. R package version 1.2-1. https://CRAN.R-project.org/package=minpack.lm
- Fargues, J., Goettel, M. S., Smits, N., Ouedraogo, A., & Rougier, M. (1997). Effect of temperature on vegetative growth of Beauveria bassiana isolates from different origins. Mycologia, 89(3), 383–392. https://doi.org/https://doi.org/10.1080/00275514.1997.12026797
- Fletcher, D. J. C., & Crewe, R. M. (1981). Nest structure and thermoregulation in the stingless bee, Trigona denoiti, hymenoptera: Apidae. Journal of the Entomological Society of Southern Africa, 44(2), 183–196.
- García–Fernández, P., Santiago–Álvarez, C., & Quesada–Moraga, E. (2008). Pathogenicity and thermal biology of mitosporic fungi as potential microbial control agents of Varroa destructor (acari: Mesostigmata), an ectoparasitic mite of honey bee, Apis mellifera (hymenoptera: Apidae). Apidologie, 39(6), 662–673. https://doi.org/https://doi.org/10.1051/apido:2008049
- Gougouli, M., & Koutsoumanis, K. P. (2012). Modeling germination of fungal spores at constant and fluctuating temperature conditions. International Journal of Food Microbiology, 152(3), 153–161. https://doi.org/https://doi.org/10.1016/j.ijfoodmicro.2011.07.030
- Gougouli, M., & Koutsoumanis, K. P. (2013). Relation between germination and mycelium growth of individual fungal spores. International Journal of Food Microbiology, 161(3), 231–239. https://doi.org/https://doi.org/10.1016/j.ijfoodmicro.2012.12.006
- Huang, L., Hwang, C.-A., & Phillips, J. (2011). Evaluating the effect of temperature on microbial growth rate–The Ratkowsky and a bělehrádek–type models. Journal of Food Science, 76(8), 547–557. https://doi.org/https://doi.org/10.1111/j.1750-3841.2011.02345.x
- Ibrahim, L., Butt, T. M., & Jenkinson, P. (2002). Effect of artificial culture media on germination, growth, virulence and surface properties of the entomopathogenic hyphomycete Metarhizium anisopliae. Mycological Research, 106(6), 705–715. https://doi.org/https://doi.org/10.1017/s0953756202006044
- Jarimi, H., Tapia-Brito, E., & Riff, S. (2020). A review on thermoregulation techniques in honey bees’ (Apis mellifera) beehive microclimate and its similarities to the heating and cooling management in buildings. Future Cities and Environment, 6(1), 1–8. https://doi.org/https://doi.org/10.5334/fce.81
- Kvålseth, T. O. (1985). Cautionary note about R2. American Statistician, 39(4), 279–285. https://doi.org/https://doi.org/10.1080/00031305.1985.10479448
- Lactin, D. J., Holliday, N. J., Johnson, D. L., & Craigen, R. (1995). Improved rate model of temperature-dependent development by arthropods. Environmental Entomology, 24(1), 68–75. https://doi.org/https://doi.org/10.1093/ee/24.1.68
- Lehmann, P., Ammunét, T., Barton, M., Battisti, A., Eigenbrode, S. D., Jepsen, J. U., … Björkman, C. (2020). Complex responses of global insect pests to climate warming. Frontiers in Ecology and the Environment, 18(3), 141–150. https://doi.org/https://doi.org/10.1002/fee.2160
- Mangiafico, S. (2020). Rcompanion: Functions to support extension education program evaluation. R package version 2.3.25. https://CRAN.R-project.org/package=rcompanion
- Merkle, E., & You, D. (2020). Nonnest2: Tests of non–nested models. R package version 0.5-5. https://CRAN.R-project.org/package=nonnest2
- Mora, M. A. E., Castilho, C. A., & Fraga, M. E. (2017). Classification and infection mechanism of entomopathogenic fungi: Review article. Arquivos do Instituto Biológico, 84, 1–10. https://doi.org/https://doi.org/10.1590/1808-1657000552015
- Niassy, S., Maniania, N. K., Subramanian, S., Gitonga, L. M., Mburu, D. M., Masiga, D., & Ekesi, S. (2012). Selection of promising fungal biological control agent of the western flower thrips frankliniella occidentalis (pergande). Letters in Applied Microbiology, 54(6), 487–493. https://doi.org/https://doi.org/10.1111/j.1472-765X.2012.03241.x
- Onsongo, S. K., Gichimu, B. M., Akutse, K. S., Dubois, T., & Mohamed, S. A. (2019). Performance of three isolates of Metarhizium anisopliae and their virulence against zeugodacus cucurbitae under different temperature regimes, with global extrapolation of their efficiency. Insects, 10(9), https://doi.org/https://doi.org/10.3390/insects10090270
- Ouedraogo, A., Fargues, J., Goettel, M. S., & Lomer, C. J. (1997). Effect of temperature on vegetative growth among isolates of Metarhizium anisopliae and M. Flavoviride. Mycopathologia, 137(1), 37–43. 101023/A
- Peleg, M., & Normand, M. D. (2013). Modeling of fungal and bacterial spore germination under static and dynamic conditions. Applied and Environmental Microbiology, 79(21), 6764–6775. https://doi.org/https://doi.org/10.1128/AEM.02521-13
- Quesada-Moraga, E., Maranhao, E. A. A., Valverde-García, P., & Santiago-Álvarez, C. (2006). Selection of Beauveria bassiana isolates for control of the whiteflies bemisia tabaci and trialeurodes vaporariorum on the basis of their virulence, thermal requirements, and toxicogenic activity. Biological Control, 36(3), 274–287. https://doi.org/https://doi.org/10.1016/j.biocontrol.2005.09.022
- Ramli, A. S., Luqman, A. H., Basrawi, F., Oumer, A. N., Abd Aziz, A., & Mustafa, Z. (2017). A new cooling technique for stingless bees hive. MATEC Web of Conferences, 131, 3013. https://doi.org/https://doi.org/10.1051/matecconf/201713103013
- Rangel, D. E. N., Braga, G. U. L., Anderson, A. J., & Roberts, D. W. (2005). Variability in conidial thermotolerance of Metarhizium anisopliae isolates from different geographic origins. Journal of Invertebrate Pathology, 88(2), 116–125. https://doi.org/https://doi.org/10.1016/j.jip.2004.11.007
- Ratkowsky, D. A., Lowry, R. K., McMeekin, T. A., Stokes, A. N., & Chandler, R. E. (1983). Model for bacterial culture growth rate throughout the entire biokinetic temperature range. Journal of Bacteriology, 154(3), 1222–1226. https://doi.org/https://doi.org/10.1128/jb.154.3.1222-1226.1983
- R Core Team. (2020). R: A language and environment for statistical computing. R software version 4.0.3. R Foundation for Statistical Computing.
- Rosso, L., Lobry, J. R., Bajard, S., & Flandrois, J. P. (1995). Convenient model to describe the combined effects of temperature and pH on microbial growth. Applied and Environmental Microbiology, 61(2), 610–616. https://doi.org/https://doi.org/10.1128/aem.61.2.610-616.1995
- Smits, N., Brière, J. F., & Fargues, J. (2003). Comparison of non–linear temperature-dependent development rate models applied to in vitro growth of entomopathogenic fungi. Mycological Research, 107(12), 1476–1484. https://doi.org/https://doi.org/10.1017/S095375620300844X
- Tefera, T., & Pringle, K. (2003). Germination, radial growth, and sporulation of Beauveria bassiana and Metarhizium anisopliae isolates and their virulence to chilo partellus (lepidoptera: Pyralidae) at different temperatures. Biocontrol Science and Technology, 13(7), 699–704. https://doi.org/https://doi.org/10.1080/0958315031000151756
- Tumuhaise, V., Ekesi, S., Maniania, N. K., Tonnang, H. E. Z., Tanga, C. M., Ndegwa, P. N., … Mohamed, S. A. (2018). Temperature–dependent drowth and virulence, and mass production potential of two candidate isolates of Metarhizium anisopliae (metschnikoff) sorokin for managing maruca vitrata fabricius (lepidoptera: Crambidae) on cowpea. African Entomology, 26(1), 73–83. https://doi.org/https://doi.org/10.4001/003.026.0073
- Van Der Heide, T., Roijackers, R. M. M., Van Nes, E. H., & Peeters, E. T. H. M. (2006). A simple equation for describing the temperature dependent growth of free–floating macrophytes. Aquatic Botany, 84(2), 171–175. https://doi.org/https://doi.org/10.1016/j.aquabot.2005.09.004
- Vuong, Q. (1989). Likelihood ratio tests for model selection and non–nested hypotheses. Econometrica, 57(2), 30–333. https://doi.org/https://doi.org/10.2307/1912557
- Zwietering, M. H., De Koos, J. T., Hasenack, B. E., De Wit, J. C., & Van't Riet, K. (1991). Modeling of bacterial growth as a function of temperature. Applied and Environmental Microbiology, 57(4), 1094–1101. https://doi.org/https://doi.org/10.1128/aem.57.4.1094-1101.1991