Abstract
Artificial intelligence (AI) and other AI-based applications are being integrated into firms’ human resource management (HRM) approaches for managing people in domestic and international organisations. The last decade has seen a growth in AI-based applications proliferating the HRM function, triggering an exciting new stream of research on topics such as the social presence of AI and robotics, effects of AI adoption on individual and business level outcomes, and evaluating AI-enabled HRM practices. Adopting these technologies has resulted in how work is organised in local and international firms, noting opportunities for employees and firms’ resource utilisation, decision-making, and problem-solving. However, despite a growing interest in scholarship, research on AI-based technologies for HRM is limited and fragmented. Further research is needed that analyses the role of AI-assisted applications in HRM functions and human-AI interactions in large multinational enterprises diffusing such innovations. In response to these combined issues—the fragmented nature of research and limited extant literature, we present a systematic review on the theme of this special issue and offer a nuanced understating of what is known, yet to be known, and future research directions to frame a future research agenda for international HRM. We develop a conceptual framework that integrates research on AI applications in HRM and offers a cohesive base for future research endeavours. We also develop a set of testable propositions that serve as directions for future research.
Introduction
The Fourth Industrial Revolution (4IR) marks an increased use of emerging technologies, such as artificial intelligence (AI), big data, machine learning, mobile technology, the Internet of Things, geo-tagging, virtual reality, speech recognition, and biometrics (Azadeh et al., Citation2018; Shank et al., Citation2019). The application of these advanced technologies transforms the way business is conducted locally or globally and has had a considerable impact on the way work is designed, workers are engaged, and workplace processes changed (Abraham et al., Citation2019; Agrawal et al., Citation2017; Duggan et al., Citation2020; Malik et al., Citation2020a; Citation2022; McColl & Michelotti, Citation2019). Indeed, serious concerns and reservations have been voiced regarding the role of AI in causing job destruction and humanity’s very basis and essence (Agar, Citation2019, Citation2020; Charlwood & Guenole, Citation2021; Malik et al., Citation2020b). Nevertheless, AI and other related intelligence-based applications bring opportunities for organisations to achieve optimal strategic business outcomes, such as enhancing service quality, productivity, cost-effective service excellence (CESE) (Wirtz, Citation2019), return on investment (Torres & Mejia, Citation2017), operational efficiency, customer engagement and loyalty (Prentice & Nguyen, Citation2020), employees’ service quality (Nguyen & Malik, Citation2022) and reducing considerable operational and capital cost (Wirtz, Citation2019). Moreover, such research also delivers positive individual-level outcomes, such as employee and talent experiences, intention to quit and job satisfaction (Malik et al., Citation2020c; Citation2021, Nguyen & Malik, Citation2022).
AI refers to a broad class of technologies that allows a computer to perform tasks that generally require human cognition, including adaptive decision-making (Tambe et al., Citation2019, p. 16). A growing debate in academic research examines different types of AI digital tools and techniques and whether firms can benefit from such business solutions (Aouadni & Rebai, Citation2017; Castellacci & Viñas-Bardolet, Citation2019). In this regard, the recent calls for academic scholarship on AI in HRM have received considerable attention in premier HRM journals, including other related disciplinary areas such as international management, information technology, and general management (see Budhwar & Malik, Citation2020; Buxmann et al., Citation2019; Jain et al., Citation2018; Kaplan & Haenlein, Citation2020; Meijerink et al., Citation2018). Thus, research at the interface of AI and HRM assumes an increasingly multidisciplinary character (Connelly et al., Citation2020). However, there is still limited understanding in the AI-HRM literature about how AI and related technologies can offer solutions for effective HRM and sub-functional areas and how AI-enabled HRM functions link to other operational tasks to deliver better results outcomes for their organisations (Agrawal et al., Citation2017).
Despite the limited knowledge on AI-HRM scholarship, a growing body of knowledge asserts that contemporary developments in automation technologies offer remarkable benefits for HRM (Bersin & Chamorro-Premuzic, Citation2019; Maedche et al., Citation2019; Prikshat et al., Citation2021). Further, organisations from local and multinational enterprises (MNEs) have understood the benefits of AI-based tools and techniques to enhanced employee satisfaction, commitment and job engagement (Castellacci & Viñas-Bardolet, Citation2019), productivity (Wirtz, Citation2019), job performance, HR cost-effectiveness (Azadeh & Zarrin, Citation2016); employee retention (Malik et al., Citation2020c, Citation2021; Nura & Osman, Citation2013), effective decision-making (Azadeh et al., Citation2018), while reducing HR-related and other operational costs (Torres & Mejia, Citation2017). The growing interest in examining AI and its impact on sub-functional areas of HRM is rising. For example, scholars argue that emerging AI-based HRM technologies can support talent acquisition, development, assessment, and retention in large technology MNEs (Bersin & Chamorro-Premuzic, Citation2019; de Kervenoael et al., Citation2020; Malik et al., Citation2021). It can also assist from recruitment to selection, assessing, and interviewing the most suitable candidates (Torres & Mejia, Citation2017; van Esch et al., Citation2019), including Industry 4.0 advertisements to take out new job profiles (Pejic-Bach et al., Citation2020) and assess employees’ training effectiveness (Sitzmann & Weinhardt, Citation2019). The above has implications for IHRM as contextual influences, such as linguistic, cultural, institutional differences across borders will need sufficiently diverse databases for AI applications to minimise any inherent biases in narrow databases and single country contexts.
Although the extant literature on AI-enabled HRM reports optimistic outcomes, others argue for examining the negative consequences of these advanced technologies for both organisations and employees (Huang et al., Citation2019). Not attending to adverse aspects may lead to unintended consequences, such as high employee turnover, decreasing job satisfaction, loss of customer satisfaction, incurring high costs, and eventually affecting organisations’ overall business performance and goodwill (Li et al., Citation2019). Furthermore, scholars point out that limitations usually happen when adapting AI in HRM due to the complex nature of HR phenomena, constraints of the small data sets, accountability questions associated with fairness and other ethical and legal issues, and possible adverse employee reactions to management decisions via data-based algorithms (Tambe et al., Citation2019).
Analysing the use of automation technologies in HRM suggests there is still limited knowledge of how AI-enabled HRM functions affect workers, their work outcomes, and overall organisational outcomes (Castellacci & Viñas-Bardolet, Citation2019). Furthermore, there is a need to show how these HR-focused AI applications improve positive outcomes while reducing negative consequences. Thus, we argue that the influence of the social-technological context, such as flexible organisational structure, proper training, dealing with fear and change management, and upskilling employees, can further strengthen to achieve favourable outcomes. We also argue that it is also vital to consider personal employee factors, such as personality and emotional intelligence, as they can influence business outcomes (Huang et al., Citation2019).
There is also an ongoing argument in the AI-HRM literature about identifying the attributes of employees when adapting AI and intelligence-based technologies in organisations to deploy these technologies effectively. However, despite the considerable opportunities provided by advanced technologies in HRM, employees are better able to perform several tasks that machines cannot do (Agrawal et al., Citation2017; Maedche et al., Citation2019). Thus, scholars argue that augmenting humans with AI applications rather than replacing them leads to optimised organisational benefits, as both AI and humans can collectively excel and perform well (Wilson et al., Citation2017). We argue that AI-enabled HRM produces favourable outcomes through Human-AI configuration mechanisms. The research base on AI applications from an IHRM perspective is relatively small, though there are some emerging signs of empirical evidence from single-country contexts (Nguyen & Malik, Citation2021, Citation2022; Pan et al., Citation2021; Suseno et al., Citation2021) or subsidiaries of large technology multinationals (Del Giudice et al., Citation2021; Jaiswal et al., Citation2021; Malik et al., Citation2020c, Citation2021).
Considering the above calls and limitations, a systematic review of the literature can provide some pathways for researchers. Taking a global view of the use of AI and advanced technologies in the field of HRM, we believe this systematic review provides a rigorous assessment of the extant literature for answering the following research questions:
What is the current knowledge of AI and intelligence-based technologies in the global business context in the field of HRM?
How do AI-enabled intelligence technologies affect employee and organisational outcomes in the global business context?
What are the main directions for consideration for future research?
This review aims to answer the above questions focusing on adopting AI applications in HRM functions in a global context. Second, this review contributes to AI literature on how automation and AI-based technologies affect HRM functions by studying outcomes at both employee and organisational levels, and considering the positive and negative consequences evident in the extant literature, thus proposing future research directions. Third, this review presents how key social-technical and personal factors influence positive outcomes at the workplace. Fourth, we highlight how Human-AI configurations play a vital role in strengthening positive individual and business unit outcomes. Fifth, by focusing on the themes of AI and its impact on HRM, we propose future research propositions to guide theory-building efforts. By focusing on the how and why of AI adoption, business and HR leaders must learn to effectively manage the opportunities and challenges posed by AI applications through appropriate social-technical and personal interventions and configurations. Sixth, we build a framework that shows the linkages between AI and intelligence technologies, HRM functions and their consequences on employee and organisational outcomes, and how some of these outcomes could be achieved through social-technical and personal factors by augmenting social-technical and personal factors.
Methodology
Employing a systematic literature review approach as our guiding research methodology (Snyder, Citation2019), this review aims to provide a thorough assessment of the extant literature on AI and advanced technologies in the field of HRM, which has implications for the management of HRs globally. Systematic reviews involve a process of collecting and critically analysing the literature and the themes emanating from the chosen studies that are within the scope of the research questions posed and to constitute a concrete foundation for advancing the knowledge and theory development on a given topic (Paul & Criado, Citation2020; Snyder, Citation2019). Furthermore, such an approach allows a repeatable and transparent process for synthesising findings that ensure overall reliability (Tranfield, Denyer & Smart, Citation2003). Therefore, guided by the suggestions of Tranfield et al. (Citation2003) and Snyder (Citation2019) for undertaking a systematic literature review in business and management research, we decided on a systematic approach as the appropriate method for this review to provide comprehensive coverage of the literature and to locate emerging themes while ensuring its repeatability.
Selection of articles and qualitative assessment
Driven by our abovementioned research questions for this review, we included studies that have focused on the use of AI or advanced technologies in the field of HRM across the international boundaries and a temporal boundary of the last decade as this is a relatively new phenomenon that has gained prominence since 2010 (2010–2020). A ten-year time frame was also decided to capture the recent development of studies that explored AI-advanced technologies and HRM as the field of AI, and intelligence-based technologies have significantly developed in recent years. Based on the focus, a range of specific keywords relating to AI, robotics and other intelligence-based technologies and the HRM discipline were included and combined in the search string using the Boolean operators, ‘OR’ and ‘AND’. The keyword search algorithm applied for this review is as follows:
(“Artificial Intelligence” OR “AI” OR “robotics” OR “bots”) AND (“human resource management” OR “HRM” OR Human resource management functions” OR “HRM functions” OR “HRM cost efficiency” OR employee-level outcomes OR “individual outcomes” OR “organisational outcomes” OR “firm level outcomes” OR “human-collaboration” OR “employee-experience”).
The next critical decision was to decide which relevant electronic databases to use as search engines. Given that the scope of the review is in the context of management discipline and technology-related fields, we selected three well-known search engines that are widely used by management and business scholars, such as ABI/ProQuest, SCOPUS and Web of Science. Having decided on the three databases, we applied the search algorithm on each search engine and filtered the initial research results for full-text, peer-reviewed articles in the English language and keeping the time frame of 2010–2020. Then, an initial screening based on the relevance was performed for the titles, keywords or abstracts to identify the papers and subsequently screened using inclusion and exclusion criteria decided for the review. Following other comprehensive systematic reviews conducted in management (e.g., Christofi et al., Citation2021), we excluded non-peer-reviewed journal articles, papers not in English (due to language limitations), books, book chapters, editorials, commentaries, executive summaries and (peer reviewed) conference papers. In addition, we only included papers from the top journals listed in the Australian Business Deans Council (ABDC – A* and A) rankings as a proxy and signalling frame for selecting high-quality peer-reviewed articles we included papers published in journals which are ranked high in a combination of leading journal ranking lists such as the Australia’s ABDC and the UK’s CABS list.
Like with any research design, researchers have managed the trade-offs of time, effort and quality in terms of the number of data points (outputs) to include in the analysis. We believe the rigour of reviewing in top-tier journals is much more robust and demanding than other categories of outputs. This is particularly true for an emerging field which faces challenges in being accepted in the mainstream premier outlets. Thus, in our bid to focus more on high-quality outputs, we may have lost some excellent papers published in the lesser ranked journals and other outputs identified above as well as some additional themes. Nevertheless, we performed a manual cross-check to screen the papers for the inclusion criteria further. Moreover, due to different approaches and perspectives contributed to this emerging field of AI and advanced technologies and limited papers in the literature, we decided to include all three types—empirical, conceptual and review papers for the review.
Having screened one hundred and ten (110) papers based on the inclusion and exclusion criteria, and after removing the duplicate papers, a qualitative approach was undertaken to further refine the articles by reading the full text of these papers. Following a preferred guideline for using two reviewers to select the final sample of articles (Snyder, Citation2019), two authors of the research team were deployed to mutually decide on the final sample of articles for this review, ensuring the quality and the reliability of the search protocol. During the qualitative assessment, two authors from the authorship team rated the 110 papers for relevance on an individual basis after full-text reading and then compared the relevance with each other. Any deviations in ratings was resolved at discussion meetings with the rest of the team for including the papers in the final sample. Out of 110 papers, forty (40) papers were eliminated during the qualitative assessment, as these papers did not focus on the central questions posed by this literature review. The process applied for the review is depicted in . A final search yielded a total of seventy (70) papers that were published in 38 journals. A list of journals and the frequencies of articles published has been presented in .
Figure 2. An integrated conceptual framework of the influence of AI-based applications technologies on employee and organisational level outcomes. Note. Items in italics and asterisks are indicative of areas which need to be tested in future research.
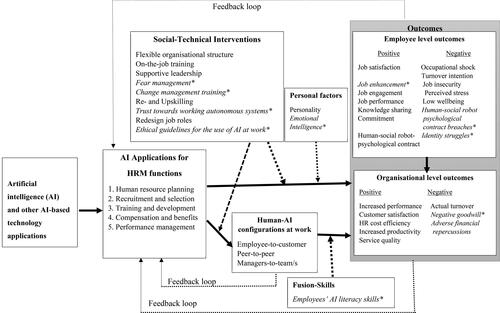
Table 1. List of journals and the frequencies of articles published.
Data coding and analysis
Relevant data from the selected articles were extracted into (Tranfield et al., Citation2003) an Excel spreadsheet containing simple categorical data, such as the article details, year of publication, author details, type of paper, methodology, research context (industry), key findings and future research directions that aided easy reading and forming the descriptive analysis (Tranfield et al., Citation2003). Following Ererdi et al. (Citation2021), the coding was conducted using two authors from the authorship team independently to ensure the inter-coder reliability and any coding discrepancies were discussed at research meetings for this review. The agreement between the two authors was 96% and the discrepancies were resolved by mutual consensus. Once extraction and coding were completed, in-depth thematic analysis was conducted to locate themes emerging. As a result, we identified a few common categories of articles on micro-foundation of AI/robotic collaboration, AI/advanced technology influence on HRM functions leading to the individual, team and organisational level outcomes, a range of intervention factors that influence the use of AI/robotics in the field of HRM and organisational settings. In addition, several articles had an international focus and included research on the adoption of AI for HR and related activities by multinational firms (e.g., Dwivedi et al., Citation2021; Li et al., Citation2016; Oesterreich et al., Citation2019; Pejic-Bach et al., Citation2020; Suen et al., Citation2019).
Key themes
To visualise the comprehensive picture of how AI and AI-based technologies affect HRM and human-machine configurations at work and their influences on employee and organisational level outcomes, we develop an overarching conceptual framework through this review. This is presented in and aligns with the above-identified themes in this review.
Theme 1: AI and intelligent technologies in HRM functions
A key emphasis has been on how AI and other related intelligence-based tools and techniques could impact the HRM function as a whole and its sub-functional domains. Analysing the literature enabled us to focus on AI-enabled HRM functions by concentrating on sub-functional domains, such as human resource (HR) planning, recruitment and selection, training and development, compensation and benefit and performance management, and analyse how these AI-enabled digitalised functions provided novel opportunities for firms and employees.
HR planning and recruitment and selection
Assigning the right person for the right job is the major challenge for HR planning. However, AI and other automation technologies make this task even easier in organisations. Mainly, AI assists in HR planning by determining future employee needs and making effective recruitment decisions (Karatop et al., Citation2015). It is also evident that AI-enabled recruitment and selection play a crucial role in attracting and selecting the most talented work pool to the organisations, as these advanced technologies can access data and make decisions at a speedy pace and can handle large volumes of information in a time that far exceeds human capacity (Torres & Mejia, Citation2017). As a result, AI algorithms can improve job candidate identification, that is who is most interested and suited for the job and provide better communication of the job opening. Influencing job seekers’ technology increases their participation in AI-enabled recruiting (van Esch et al., Citation2020). AI also assists in making the job interview process more effective, so that the interview process is now changed from face-to-face to internet-based interviews, such as asynchronous video interviews (AVIs) (Torres & Mejia, Citation2017). Moreover, Pessach et al. (Citation2020) found in their studies that using a hybrid decision-support tool helped HR professionals in the recruitment and placement processes and increased the impact of recruiters and maximised organisational return on investment. AI Algorithms allowed HR professionals to identify suitable profiles for job vacancies, eliminating cognitive biases of race, gender and sexual orientation that mar human judgement in recruiting activities.
Training and development
The literature highlights that AI supports the training and development of employees more effectively. Most importantly, systems could save each current employee’s electronic resumes that provide the organisation with an electronic inventory of its employees. This can help to track shortages in skills or to develop suitable training programmes. It can also help organisations to search for an appropriate candidate within the organisation. Moreover, employees could use these systems in order to manage their prospective careers. If employees lack any skills, these AI systems help them identify their training needs and complete the required courses. Further, online or virtual training provides a couple of benefits for both the organisation and employees. AI assists HR managers to assess training effectiveness and making decisions on employee competency, including emotional and intellectual capabilities and experiences level, in order to assign the right employee with the corresponding talents to the correct positions (Karatop et al., Citation2015; Sitzmann & Weinhardt, Citation2019).
Compensation and benefits
Our analysis presents that automation in payroll systems supports HR professionals in efficiently handling all HR payroll and related value-added activities. This is because AI technologies can track all types of employee data, including employee personal details, changes in personal information such as dependants, or marriage and beneficiary changes (Bussler & Davis, Citation2002). Moreover, the skill supply and demand gap obtained from the databases helps determine an organisation’s compensation and benefits plans (Pessach et al., Citation2020). AI systems also assist managers and experts in collecting the most pertinent information about required employees’ compensation and benefits systems. Specifically, these systems help to calculate and determine the salary parameters of employees concerning their jobs (Mehrabad & Brojeny, Citation2007)
Performance management
AI-enabled performance management tools and techniques also provide multiple opportunities for both employees and organisations. For instance, a fuzzy multi-attribute decision-making tool leads to a fair evaluation of employees. Notably, this tool helps identify employees who need further improvements in some factors and the magnitude of improvements needed (Manoharan et al., Citation2011). Moreover, digital performance tools assist managers to assess employee performance or to recommend any required improvements and take corrective actions for an employee based on expert opinion such as providing training, talent enhancement and further qualification wherever required (Azadeh et al., Citation2018; Manoharan et al., Citation2011) .
Implementation challenges
Although many studies highlight the need to adopt AI and automation intelligence technologies in HRM to obtain optimised benefits, how these systems are running is still a bottleneck in AI research. Most importantly, how organisations make decisions on employees based on the outputs provided by AI-based systems is not primarily transparent to employees (Connelly et al., Citation2020). There is an argument how employees like gig workers and workers, who work distantly from customers and organisations, monitor through AI-based technologies (Connelly et al., Citation2020). Scholars further claim that when employees do not understand how decisions have been made using AI-based systems or cannot accept these decisions, it leads them to adversarial behaviours in organisations (Tambe et al., Citation2019). However, only limited research is noted on examining how to diminish negative consequences due to the technology adaptions in HRM functions.
Ethical and legal issues
Employees need to know what data is collected about them and determine whether they are provided with the opportunity to verify their collected information produced by the relevant systems. Employees also need to know how these AI-based decisions affect their outcomes, including their attitudes and behaviours (Connelly et al., Citation2020). In this regard, employees need open and transparent communication to ensure the AI systems are working promptly, securely and reliably (Wilson et al., Citation2017). On the other hand, the literature points out that organisations communicate to employees what technologies are being used and how expert systems make employee-related decisions (Connelly et al., Citation2020). Furthermore, ethical issues from China and Global South-East Asian countries point to intensifying surveillance and ‘getting in workers’ heads’ (Houser, Citation2018; Kshetri, Citation2021; Wong & Liu, Citation2019), and there are several racist and sexist biases prevalent in some AI applications (Moosajee, Citation2019).
Way forward
Some studies emphasise that AI-enabled HRM functions, particularly e-based performance management and training and development, become more effective when improving communication (Bharadwaj & Shipley, Citation2020; Bititci et al., Citation2016). For instance, IBM uses clear dialogues between managers and employees on all valuable insights that have been obtained from analytics and AI capabilities, and they are readily available to both the HR division and employees (Kiron & Spindel, Citation2019). It is also argued that a feedback loop is needed for learning and innovation in organisations (Grønsund & Aanestad, Citation2020). Furthermore, Castellacci and Viñas-Bardolet (Citation2019) argue there is a need for developing automation-based communication technologies in organisations as these advanced algorithms facilitate communications and informal networks, enrich the information flow between employees and organisational managers and create new bonds with external agents. Thus, future research needs to focus on the how, why, and questions concerning the nature of feedback flow between organisations and employees related to AI-enabled HRM applications to minimise the impact of any unfavourable consequences at the workplace. Accordingly, we propose several propositions. For this theme we propose that:
Proposition 1.
A two-way, open and timely feedback incorporating inter-cultural and contextual knowledge in local and global organisations in the design and implementation of AI-enabled HRM applications can minimise the negative consequences of AI applications.
Theme 2: impact of AI-enabled HRM applications on business and employee outcomes
Positive individual outcomes
We found from the extant literature that AI-enabled HRM affects both employees and organisations. Most importantly, these AI-focused HRM create favourable employee outcomes such as job satisfaction, commitment, employee engagement, and participation, thereby increasing their performance (Aouadni & Rebai, Citation2017; Azadeh et al., Citation2018; Castellacci & Viñas-Bardolet, Citation2019). Employees can also use the internet to create realistic expectations and perceptions of their working conditions and to foster their competencies and training (Castellacci & Viñas-Bardolet, Citation2019). Moreover, AI applications can free up humans and their time for several predictable and routine tasks (Maedche et al., Citation2019). On the other hand, the literature also points out how these automated technologies can negatively affect employees. For instance, job insecurity, high employee turnover intentions, increased stress and negative attitudes and behaviours towards new technologies implemented are negative employee consequences that an organisation must confront. Moreover, employees’ interaction with internet use raises issues of employees’ well-being at the workplace (Castellacci & Viñas-Bardolet, Citation2019).
Positive business-level outcomes
Automation technologies in HRM impact not only employee-level outcomes but also influences business-level outcomes. The literature on AI-enabled HRM adoption suggests that it leads to productivity gains, cost reduction and operational efficiencies (e.g. flexibility, scalability, safety and reliability), customer engagement and loyalty (Botha, Citation2019; Lu et al., Citation2020; Prentice & Nguyen, Citation2020; Ransbotham et al., Citation2017; Tarafdar et al., Citation2019). AI can also yield greater returns on investment by providing cost-effectiveness to the organisation (Torres & Mejia, Citation2017). The other business-productivity outcome of the AI technology is cost-effective service excellence (CESE), which refers to organisations simultaneously among the best performers in their competitive market regarding customer satisfaction and productivity. For example, Amazon, a world-leading online retailer, and Singapore Airlines have achieved the CESE milestones. Emerging technologies such as AI, big data, machine learning, mobile technology, the Internet of Things, geo-tagging, virtual reality, speech recognition, and biometrics bring enormous opportunities for extensive service innovations that have the potential to enhance the customer experience, service quality and productivity richly, all simultaneously (Wirtz, Citation2019). Service robots and AI are the other examples that are also likely to provide exceptional economics of scale and scope, as they only incur bulk costs at their development stages. However, robots used at information counters incur a low cost, whereas entirely virtual robots (such as voice-based chatbots in an app or on a website) incur close to zero incremental costs. Robots can collect data from various sources: the internet, cameras, microphones, sensors, and organisational knowledgebase and CRM systems. The robot can identify a customer using biometrics (facial and voice recognition systems) and can provide highly customised and personalised service on a scale at a small marginal cost (Wirtz, Citation2019).
Negative individual outcomes
Although the positive consequences of advanced technologies are emphasised in the literature, several negative issues have also been identified. For instance, improper use of such technologies in HRM may lead to high employee turnover. Although service robots significantly contribute at the market level, employees are considered the most critical assets in people-intensive services. Moreover, service robots can only perform cognitive and analytical tasks that require low levels of emotional or social complexity.
On the other hand, services connected with high emotional or social complexity require emotional authenticity, which typically humans are more adept at displaying. Moreover, tasks that are highly complex and need high emotional-social skills need to be performed by humans. However, such tasks may be augmented by robots and AI. Therefore, service robots may not be a key source of competitive advantage beyond the short-to-medium term (Wirtz, Citation2019).
Adverse business-level outcomes
While the 4IR transforms the way of doing significant activities in organisations, it is still a debatable topic how effectively it impacts the organisation’s people, process, system, and structure it is still a debatable topic. More studies discuss the severe effects of adapting automation-based technologies in the workplace. For instance, Dwivedi et al. (Citation2021) claim that, by 2030, 70 per cent of business operations will have adopted some form of AI technology within their business or manufacturing processes. Scholars further predict that Smart Technology could replace 57% of existing jobs within the OECD, by AI, Robotics, and Algorithms, and most organisations are under pressure to make any progress on building AI data analytics capabilities (Brougham & Haar, Citation2020).
System-level challenges
Brougham and Haar (Citation2020) also found in their study that threat of technological disruptions leads to employee job insecurity and thereby increased turnover intentions. They also argue that employees feel fewer technological disruptions when they have fewer job mobility options. This study further asserts that, although employees leave organisations, turnover leads to adverse effects, including low employee job satisfaction. Thus, many studies assert that technological advancements in organisations increase fear among employees for their tasks and jobs, which may be adversely affected due to these advanced technologies. Moreover, employees’ negative attitudes towards technological evolutions are another significant barrier to successfully adopting and implementing advanced technologies in the workplace (Brougham & Haar, Citation2020). Thus, there is a need to answer how to alleviate fear among employees for the implementation of new technologies in HRM functions.
In this regard, researchers argue that proper and meaningful training is highly valued for reducing employees’ negative feelings towards new technological adaptions (Brougham & Haar, Citation2020). Furthermore, Tambe et al. (Citation2019) argue that organisational change practitioners need to accommodate change perspectives required to carry out technology innovation in organisations. Training also enables employees of organisations to learn routines, memorise relevant information and understand how to use IT systems (Wirtz, Citation2019). Thus, creating a new culture and organisational redesign helps overcome the technological obstacles at the workplace.
Also, scholars claim that appropriate organisational culture is required for the successful long-term implementation of automation technologies (Bititci et al., Citation2016). However, reducing fear among employees is not studied well in the literature. This needs further investigation. Therefore, we formulate the following proposition:
Proposition 2.
Lack of effective fear and change management training can rouse employees’ fear of AI-enabled HRM functions, thus increasing their intentions to leave their organisations or negatively impact their performance.
Theme 3: configurations of human-AI-enabled technologies interplay at the workplace
Use of digitalized co-workers
It is not uncommon to see different forms of AI-driven technologies deployed in organisational contexts, for example, involving logical decision-making and enhanced cognition, previously performed by humans (Grønsund & Aanestad, Citation2020). The extant literature provides limited evidence of such applications as the field is still evolving. However, there has been a noticeable surge in studies concerning human-AI collaboration in the last 5 years (2015–2020). Nevertheless, this is not steady and consistent, leaving more room for conceptual and empirical studies within AI/BOT applications and human interactions. Recent research on service employees, in particular studies on customer service frontline employees, offers evidence of utilisation of intelligent bots and automation (e.g. voice recognition assistants, chatbots and other service automation systems) to streamline processes and improve task efficiency (e.g. Henkel et al., Citation2020; Li et al., Citation2019; Prentice et al., Citation2020b).
Gradual job-replacement thesis
Firstly, in our observations of extant literature, scholars have acknowledged the role of AI and intelligent technologies in accelerating specific tasks and processes while noting that AI deployment resonates a risk of ‘human replacement’ (Li et al., Citation2019). In their theory of job replacement through AI, Huang and Rust (Citation2018) significantly contributed to the literature concerning this double-edged effect of AI in services as to conceptualise how AI reshapes service and how AI may replace service workers entirely, thus suggesting employees to develop specific skills—intuitive and empathetic skills that AI is not yet capable of holding to it. Furthermore, their theory asserts that while service employees focus on developing their intuitive and empathetic skills, AI may help take care of analytical tasks that save human time and resources.
Apart from this theory, only a handful of studies have theoretically or empirically investigated the effects of human-AI configurations, what tasks can be best performed by AI and intelligent technologies, and what skills and competencies employees need to develop in AI-driven organisational settings. A significant number of scholars have recently acknowledged more ethical and justice to consider the human worker in the loop where most of the studies encouraged augmenting employees, rather than a replacement as the technology itself in organisational contexts (e.g. Dwivedi et al., Citation2021; Glikson & Woolley, Citation2020; Grønsund & Aanestad, Citation2020; Maedche et al., Citation2019; Metcalf et al., Citation2019). These studies have alluded to different roles or acts or skills that employees should play and develop at work to remain in the loop and cope with their roles being augmented from AI/intelligent technologies for better organisational results. Several scholars agree that employees must acquire new skills—to work with AI or expand their existing skills focused on emotions, judgement, innovation, personal relationships and soft skills (e.g. Agrawal et al., Citation2017; Huang et al., Citation2019; Huang & Rust, Citation2020; Larivière et al., Citation2017; Rampersad, Citation2020; Ransbotham et al., Citation2017; Wilson & Daugherty, Citation2018). Doing so will help utilise their knowledge and skills in heterogeneous tasks organisational value addition activities. The role of AI and AI-enabled technologies can play a complementary rather than competing a competing role with humans, as it helps interact with customers or co-workers/team members and amplifies human capability by dealing with high volume analytical and routine tasks or offering efficient, personalised services on a scale (e.g. Agrawal et al., Citation2017; Huang & Rust, Citation2020; Wirtz et al., Citation2018). Building on different capabilities that human workers and AI/intelligent technologies can offer, we can develop a better human-AI configuration, and thus, the following propositions are offered:
Proposition 3a.
AI/intelligent technologies can augment human capacity by delivering routine (homogeneous) high volume analytical tasks while leaving the non-routine (heterogeneous), judgment-based low volume and non-analytical tasks to be performed by humans.
Proposition 3
b. With the increased augmentation of humans, HR managers can leverage AI/intelligent technologies to deliver transformative and innovative solutions for acquiring, developing and retaining talent, thus enhancing employee experience and engagement propositions.
Re-inventing and re-imaging self for better alignment
In further reaction to the above proposition, we assert our argument on employees’ particular skills to better fit human-AI configurations? The extant literature notes the importance of re-skilling, job redesign and training workers to use intelligent agents or AI technologies (e.g. Aleksander, Citation2017; Grønsund & Aanestad, Citation2020; Larivière et al., Citation2017; Ransbotham et al., Citation2017). Nevertheless, this stream of research tends to be less conclusive on which specific skills a worker should adopt or develop to work together with AI depending on the nature of AI technology(ies) being used at work. Wilson and Daugherty (Citation2018) introduced the term ‘fusion skills’—to define the skills that employees need to develop that enable them to work effectively in the human-AI interplays at work. They assert that organisations and the management teams should use the full potential machine and human intelligence through effective collaboration to transform their operations into the next level of business competition to capitalise on market opportunities. Malik et al. (Citation2021) found the presence of talent supply chain bots for acquiring, developing and retaining talent and enhancing their employee experience (EX) through hyper-personalisation of the talents’ EX. To this end, literacy on using algorithm-based systems and intelligent agents, we would call ‘AI literacy’, help human workers better collaborate with the systems and ensure the work is getting through AI/intelligent technologies. The underline notion that the positive effect of AI/intelligent technologies can be enhanced when employees have better fusion skills and literacy to work with AI/intelligent technologies, we propose the following:
Proposition 4a.
The positive effect of human-AI/intelligent technologies configurations will be stronger when employees have fusion skills and AI literacy.
Proposition 4
b. The positive effect of AI-assisted talent applications is likely to provide a positive employee experience for talents through hyper-personalised talent development opportunities.
AI-mediated social exchanges
Thirdly, we note that studies on employee-level outcomes as a result of human-AI interplay at work show positive empirical effects or conceptual viewpoints regarding the benefits through such exchanges (e.g. Hohenstein & Jung, Citation2020; Metcalf et al., Citation2019; Wilson & Daugherty, Citation2018), adverse effects (e.g. Brougham & Haar, Citation2020; Li et al., Citation2019) or mixed-effects (e.g. Henkel et al., Citation2020; Prentice et al., Citation2020a, Prentice et al., Citation2020b). The studies selected for this review examine employee outcomes such as perceived trust, performance, job satisfaction, affective well-being, turnover intention/retention, and job insecurity.
Research on frontline services can be found in a dominant context concerning human-social robot/intelligent machines that found a range of employee level outcomes, including customers’ perspective of service performances. For example, Henkel et al. (Citation2020) found mixed outcomes of service employees in two centralised call centres in the Netherlands, augmenting service employees with AI emotion recognition tool improved the effectiveness of controlling customer emotions and subsequently elevated their affective well-being. Nevertheless, it causes a higher level of stress at the initial phase of the technology. Further evidence suggests that despite employees integrating with AI and related technologies, a range of social support and inducement is needed to cope well with the nature and extent of changes in organisational workplaces. Li et al. (Citation2019) provide evidence that though the relationship between AI and robotic awareness and employee turnover intention is related, the strength of the relationships may moderate from the perceived organisational support and competitive psychological climate from a sample of hospitality employees of China. Investigating the extant literature, we build on a collective term - social-technical interventions, wherein scholars have noted the presence of flexible organisational structures, supportive leadership, opportunities for upskilling, job redesign, change management training and overcoming their fear and anxieties (e.g. Aleksander, Citation2017; Dwivedi et al., Citation2021; Li et al., Citation2019; Lu et al., Citation2020; Robert et al., Citation2020). Further, the high quality of AI-mediated exchanges can lead to high levels of EX, which can trigger the norm of reciprocity, such that positive EX of employees of such applications can result in greater employee commitment and job satisfaction (Malik et al., Citation2021; Nguyen & Malik, Citation2021). This approach can potentially elevate positive employee outcomes when using AI/intelligent technologies at work. Accordingly, we propose the following:
Proposition 5a.
The positive effect of AI and intelligent technologies on employee outcomes will be strengthened in the presence of supportive social-technical interventions at work.
Proposition 5
b. The quality of AI applications can positively affect EX, thus triggering a norm of reciprocity by employees in the form of high levels of employee commitment and job satisfaction.
Some AI scholars assert that employees are considered the most critical assets and can react better than machines (Agrawal et al., Citation2017; Wirtz, Citation2019). Moreover, researchers argue that personal factors, such as employees’ personality traits, affect people’s cognitive, affective, and behavioural outcomes (Abubakar et al., Citation2019). This is because most employment actions affect the socio-psychological status of employees, such as personal status and perceived fairness (Tambe et al., Citation2019). Moreover, some scholars argue that employees must emphasise their work’s empathetic and emotional dimensions (Huang et al., Citation2019).
Although service robots can perform cognitive and analytical tasks which require low emotional or social complexity, services connected with high emotional or social complexity require emotional authenticity, which a human can only display. Moreover, tasks that are highly complex and need high emotional-social skills need to be performed by humans and augmented by robots and AI. Henkel et al. (Citation2020) argue that when service employees augment with an AI emotion recognition tool lead to regulating emotions in customers and enhancing customer relationships. However, augmentation with AI technology leads to extra stress and requires roles changes.
Agrawal et al. (Citation2017) emphasise that demand for employees increases in the workplace, as they can engage well with customers using emotional intelligence and creating new opportunities. Thus, we put forward the following proposition: developing emotional intelligence and supporting specific employee personality types can lead to effective and productive customer interactions.
Proposition 6.
Developing emotional intelligence and identifying and supporting certain personality traits of employees can lead to effective perceptions of AI-enabled HRM functions and leads to positive and productive engagement of customers.
Many new relationships need to explore what contextual factors cause employee outcomes to turn into a positive or negative state, what boundary conditions would change the attitudinal and behavioural outcomes in human-AI interplays, and what perceptions are evolving in the use of human-AI collaborations in different industry settings. Future research could explore these emerging issues to expand the depth of extant literature.
Theme 4: ethical and legal challenges in using AI and intelligent technologies in an international HRM context
Ethical and moral issues
Beyond the increasing popularity of adopting AI-enabled intelligent applications and technologies in organisations worldwide, a central theme in the extant literature is to address the vital aspect concerning ethics, accountability, trust, fairness and legal implications of using AI-driven technologies and autonomous systems at workplaces. The issue of equity, diversity and inclusion (EDI) is also vital, as early applications of AI by large technology corporates such as Amazon revealed biases against women in hiring. In other tech firms, biases against people of colour in the promotion and career advancement decisions are also noted. A lot more work is needed to reduce biases by making high-quality AI applications.
Recent research by Nguyen and Malik (Citation2021, Citation2022) points to the importance of AI service quality and knowledge sharing in organisations to improve the experience of employees’ service quality and customer satisfaction. However, very few review studies have identified the above topics, such as reviews by Dwivedi et al. (Citation2021) and others (De Stefano, Citation2019; Glikson & Woolley, Citation2020; Robert et al., Citation2020; Tambe et al., Citation2019). Firstly, the responsibility of decisions made by AI/intelligent technologies and the gravity of such decisions against human value judgement remains a challenge, as discussed by Dwivedi et al. (Citation2021). Secondly, there are instances where moral values come into consideration that could be missing in the radar of AI-driven decision making. To further explain, Tambe et al. (Citation2019) discussed two associated challenges on accountability concerns and possible adverse employee outcomes in reaction to management decisions by algorithms. In particular, employee resistance to AI-driven decisions may be caused when the decision could be biased to certain groups of organisations based on data patterns and frequencies that AI captures may raise concerns in a diverse employee base in a multi-cultural organisation setting. However, AI/intelligent technologies perform better than human judgements in repetitive situations rather than heterogeneous case-based scenarios (Tambe et al., Citation2019). Thus, a careful delegation of work on what decisions algorithms may be responsible for should be determined to gain a mutual advantage of having accurate and effective decisions without confronting ethical and accountability concerns.
Trust in AI. Researchers have raised the concern of trust as it has been commonly recognised that lack of trust and ethical dimensions of AI/intelligent technologies in data exchange and sharing. Featuring human trust in AI, Glikson and Woolley (Citation2020) proposed a framework that has elements in shaping users’ cognitive trust (rational thinking) and emotional trust (affect-based). According to authors, in building human’s cognitive trust in AI, role of tangibility (physical presence of robots), transparency (underlying rules of operation and rationale of the technology), reliability (technology trustworthiness for expected behaviour), task characteristics (functional abilities of robots) and immediacy behaviours (extent of physical/psychological closeness – social robots) are said to be important antecedents. For an emotional trust, the role of anthropomorphism (human-like features) and immediacy behaviours (responsiveness) have been identified, in addition to the role of tangibility (Glikson & Woolley, Citation2020). However, we should note a lack of empirical research on trust issues of adopting AI and autonomous related systems, as it is not investigated how trust could be engendered in different organisational settings (e.g. healthcare, finance, telecommunication and transportation).
Regulation and ethics
Finally, in terms of legal and fairness aspects of AI/intelligent technologies, the review identifies the studies of De Stefano (Citation2019) and Robert and colleagues (2020). The advent of advancing technologies has increased legal implications in setting new laws, issues related to termination/redundancies of employment, liabilities to workers (e.g. injuries) caused by bots and protection of personal information. De Stefano (Citation2019, p. 3) asserts on ‘jobs of the future’, which requires high technical skills, where regulators should allow employees to be equipped with relevant high-tech skills to be employed in modern roles of working and take measures to absorb ‘occupational shocks’ caused by workplace automation and intrusive business practices which could result from ‘management by algorithms’. Thus, studies such as Robert et al. (Citation2020) stress designing AI/BOT systems to support workplace fairness and ethics, ensuring such work organisation is mutually beneficial to humanity and organisational efficiency goals. Theoretical lenses of organisational justice (including three types of fairness: distributive, procedural and interactional) and utilitarian theories have been applied in the discussion of ethical and human aligned- AI/robotics work settings. (Robert et al., Citation2020).
Although originally developed for the EU, the establishment of the Global Data Protection Regulation (GDPR) as key legislation dealing with data protection on privacy issues is gradually finding its place in different countries, showing some evidence of harmonisation. Yet, the enforcement is problematic in the context of MNEs and the large IT MNEs who are developers of such AI applications and products, making the accountability of such applications and data ownership and privacy issues a complex problem. Taken together, the findings and conclusions of the studies suggest that potential adverse effects, i.e. ethical concerns on biases in algorithmic decisions, trust and reliability issues and other issues related to employment arise from using AI and intelligence technologies at work, could be minimised using context-specific, transparent guidelines on ethics and accountability. These guidelines should address all possible concerns in HRM functions organising work from human-human and human-machine collaborations that will positively engender individual and organisational level outcomes. Therefore, we propose;
Proposition 7.
The presence of transparent, ethical and accountability guidelines for using AI and AI-enabled intelligence technologies at work will positively influence AI-enabled HRM functions, human-AI configurations, and individual and organisational level outcomes.
Implications for theory and practice
Our review analysed the extant literature, presented opportunities and challenges of AI and other automated technologies for HRM, exploring how the automated HRM functions can impact employee and organisational outcomes. From the extant literature, we found that AI and other related automation technologies offer numerous opportunities for HRM functions, particularly in attracting the star performers, enhancing training effectiveness, identifying skill-gaps and recommending any required training and development for employees, assisting in employee payroll, effective decision-making on employees, while reducing the costs and time, cognitive biases made by humans in HRM activities (Karatop et al., Citation2015; Kshetri, Citation2021; Pessach et al., Citation2020; Torres & Mejia, Citation2017). Thus, AI and related intelligence technologies play a crucial role in strengthening HRM functions and activities.
Moreover, our review highlights that AI-enabled HRM functions can impact both employees and organisations. For this reason, there is a need to study some influential factors which may further strengthen favourable outcomes. Thus, we argue social-technical interventions and employee factors could influence positive consequences at the workplace. Furthermore, literature argued that AI and advanced robot technologies might take human jobs (Wirtz, Citation2019). However, some arguments of augmentation suggest that instead of automation, it is the importance of AI-human configurations in the effective use of AI-enabled HRM functions that will deliver better outcomes.
Although literature points to AI as an asset, the extent to which it can be classified is questionable and unclear, as AI and other automation technologies always need to adhere to privacy, legal, moral and ethical principles. Further, the service quality of AI applications, their design, and delivery attributes are vital for the ease of use and EX. Hence, further research is needed on developing measurements for AI service quality, AI attributes and AI-mediated knowledge sharing mechanisms. Though some progress has been made in developing measures for AI-service quality and its attributes (Nguyen & Malik, Citation2021), further work is needed for a range of AI inputs, processes and outputs to advance the field further. Thus, considering clear, transparent legal, ethical, and accountability guidelines are necessary for prompting favourable consequences due to the automation of HRM function at the workplace. Additionally, there is also a need to have sound principles and guidelines on analysing how effectively AI can augment humans and the possible impacts of these configurations. Finally, we believe that the model presented and propositions developed in this review will provide a pathway for future researchers to examine how AI-focused HRM functions enhance positive outcomes in organisations through some interventions and conditions.
In line with the opportunities provided by the intelligence technologies, this review also provides some practical implications for managers, practitioners and policymakers in organisations. As per this review, advanced automated technologies shape, assist and change the handling of HRM activities in organisations. As a result, AI and related automation technologies are not only helping attract and select high calibre, but they also help in providing prompt and effective training and development to employees in handling massive employee data and assisting managers to make better decisions for their employees. The supportive role provided by the technologies in HRM function eventually leads to cultivating favourable employee outcomes such as enhanced employee participation and job satisfaction, commitment and job performance. Thus, it is possible to boost organisational outcomes, such as enhanced performance, productivity, efficiency, cost-effectiveness, and customer satisfaction and service quality. However, on the other hand, it is the responsibility of organisations to ensure all legal, moral, and ethical guidelines on how to use these technologies in HRM, as they can affect workers and the working process.
Most importantly, it is vital to understand how employees perceive and behave in response to technological advancements. Thus, based on this review, we suggest that developing favourable and supportive social-technological interventions such as flexible organisational structure, supportive leadership, ethical workplace culture, change and fear management training, upskilling employees and redesigning job roles will lead to enhanced positive outcomes in the workplace. Moreover, we put forward that creating favourable personal factors of employees, such as personality and emotional intelligence, also assist in creating those positive results for organisations. Finally, our review also greatly recommends the importance of AI-human configurations at the workplace to optimise the benefits offered by technologies.
Despite the increasing attention among scholars on AI and intelligent based technologies in augmenting human potential and elevating organisational outcomes, detailed research concerning its effect, practices/techniques to effectively use AI-human configurations, and other related topics largely remain unexplored. Nevertheless, this systematic literature review unveiled many potential research areas and developed research propositions. For example, future studies might want to theorise what a priori conditions organisations (e.g. cultural and strategical influences) need to adopt for an AI-driven HRM function and then test their effects on employee and organisational level to verify its effectiveness.
We further suggest scholars examine organisational and HRM approaches by making choices regarding delegation of work and decision-making between humans and AI technologies for achieving a better combination between the human-AI interplay at work. Kshetri (Citation2021) provides ten case examples of AI usage in domestic, government and global multinational enterprises covering different aspects of HRM’s functional practices, such as recruitment and selection (e.g. in WeChat recruiting, DBS’s Jim, Talkpush’s Stanley and Ajingas’s talent management tool) and other talent management, development and utilisation tools (e.g., EY’s Goldie, Supa Agent’s DIANE, Leena’s AI chatbot and Daeyea’s ‘brain surveillance device). Therefore, it is crucial to investigate how job redesign and upskilling of employees can be utilised to facilitate changes in future organisational work. Specific areas of HRM functions, for example, predictions of human resource planning via AI-enabled HR analytics, personalised coaching on employee career development, a personalised recommendation for training and upskilling, could be vital applications for producing insights for HR practitioners to organise well in the future world of work. Apart from the positive effects, future studies might also look at potential conflicts at an employee and organisational level. These include conflicts, such as breaches between human and social robots’ psychological contract, identity struggles and cognitive dissonance of coping with non-humans and humanoid-based systems at work.
Papers in this special issue
We highlight the current state and potential areas of future research on the very topic from our systematic literature review in the first section that pave the path to this section, where we introduce cutting-edge research of challenges and opportunities of AI in the international HRM context. We received a total of 25 manuscripts but following a rigorous editorial and double-blind reviewing AQ1of papers by expert scholars in this emerging field, whom we sincerely thank, we included six exciting and rigorously executed studies relevant to research for an international HRM context. The contributions span 24 European countries, including data in different papers on subsidiaries of several Canadian, French, Irish, Swiss, the US and UK, multinational corporations (MNCs), including domestic firms and MNCs from India and China. The contributions include quantitative, qualitative and conceptual papers. The focus of these papers was on AI scholarship in IHRM, ranging on aspects such as higher-level debates on automation and augmentation, firm-level productivity and performance, the impact of AI-enabled applications on HR effectiveness and individual-level outcomes, the specific impact of AI-enabled HRM applications on individual outcomes, and the impact of a bundle of HRM practices on skills needed by employees and HR practitioners for managing change. A substantive review of the field is also included in this special issue.
Presented in the order of propositions, the first paper by Del Giudice et al. (Citation2021) presents data from intercultural contexts (partially supports Proposition 1), analysing data from 24 European countries using the theoretical lens of service robot deployment model and organisational ambidexterity to investigate the impacts of robots in the workplace on firm productivity. Their distinctive contribution lies in analysing the indirect impact of robots deployment on productivity by modifying existing and creating new creative routines. Furthermore, the authors argue the vital role of leaders in managing this transition of automation and adoption of service robots by ensuring they maintain the balance between exploratory and exploitative routines.
The second paper by Pan et al. (Citation2021) explores AI use adoption in employee recruitment practices. Employing the technology, organisation and environment model and integrating it with transaction cost theory, the authors analyse the enablers and barriers in adopting AI and its usage for employee recruitment practices. The authors analysed data from 297 Chinese firms and found that various technological, organisational and environmental elements directly affect AI usage for recruitment in the surveyed sample. The authors found positive moderation effects for the interaction terms between transaction cost theory determinant of asset specificity in examining the relationship between technology competence on AI usage, indicating that the interaction was only significant for firms with high levels of asset-specificity. Similarly, support was also found for the moderation effects of uncertainty in examining the relationship between technical competence and AI usage, such that the interaction was significant for companies facing high uncertainty, thus partly supporting Proposition 3a.
The third paper by Malik et al. (Citation2021) presents an in-depth qualitative case study data of humongous global information technology consulting MNC with its subsidiary in India, focusing on delivering innovative and cutting-edge AI and disruptive technology solutions for several industries worldwide. The authors analyse the adoption and diffusion of several AI-enabled HRM applications by the global MNC and develop contextually relevant AI applications for its subsidiary operation in India. The case presents self-learning by different AI applications that deliver solutions and respond to HR queries for an Indian context. Other AI Bots addressed the diverse and specific needs of Indian employees for a range of routine, non-routine and somewhat complex HR queries. These HR-focused AI-applications’ ability to offer personalised, hyper-personalised responses, suggestions and problem-solving helped employees to receive individualised solutions. In addition, these bots collectively improved the overall HR cost-effectiveness in terms of the sheer volume of routine queries dealt with by these applications. Such an AI-mediated social exchange helped improve the overall employee experience proposition, leading to more significant commitment, satisfaction and a reduced intention to quit, thus partly supporting Propositions 3a, b, 4b and 5.
The fourth paper by Jaiswal and colleagues (2021) focuses on the crucial issue of upskilling employees to understand and utilise AI technologies, especially in light of the increased adoption and proliferation of AI-enabled applications and technologies at work. Employing the theoretical lens of dynamic skill, neo-human capital, and AI job replacement theories, the authors present qualitative data analysis of 20 experienced technical and managerial professionals working in subsidiaries of Canadian, Danish, French Irish, Indian, Swiss, the UK and US multinational corporations (MNCs) operating in India. Findings from their analysis highlight five critical core skills, including data analysis, digital, complex cognitive, decision making, and continuous learning, thus partly supporting Proposition 4a.
The fifth paper by Suseno et al. (Citation2021) examines the change readiness of HR managers for AI adoption. Specifically, the authors focused on HR managers’ beliefs about AI and their AI anxiety and examined its relationship with change readiness for AI adoption. Analysing data from 417 HR managers working in China and employing social cognitive theory, the authors found a significant negative relationship between HR managers’ AI anxiety on change readiness for AI adoption, while a positive and significant relationship between AI beliefs and change readiness. In addition, the authors found the moderating role of high-performance work systems (HPWS), such that HPWS attenuates the adverse effects of AI anxiety on change readiness for AI adoption, thus partly supporting Proposition 4.
The final paper by Vrontis et al. (Citation2021) presents a systematic review of 45 articles on the literature examining the emergent themes around intelligent automation in HRM and how it impacts firm performance and employment conditions and areas for future research. Among several themes, the authors found that the bulk of the intelligent automation research is in the areas of recruitment, training and job performance. Further, the emerging adoption has led to increasing job replacement thesis, human-bot interactions, and decision-making, including evidence of HRM algorithms showing adaptive and self-learning. The above studies point to several benefits to the employees, work processes and organisational effectiveness, leading to an overall more robust climate and culture for innovation, change, as noted in a recent study on elevating talent’s experience through AI-mediated social exchange in the presence of a strong culture of innovation (Malik et al., Citation2021).
Concluding remarks
Through these contributions and supplementing this with our review of the field and framing future research agenda based on a systematic literature review for the recent ten years, we expected to enhance the current knowledge in this emerging field. First, we extend the knowledge base on the drivers and consequences of the adoption of AI and AI-based intelligent technologies in international HRM and inform the research audience on the growing potentiality for further research. This research area is relatively emerging yet timely needed to be further explored through robust conceptual and empirical research keeping up with dynamic changes of technological advancement and changing business environment. Second, we reviewed the extant literature and derived the main focus areas as four key themes—and the propositions that we have developed for each theme can be explored further in future studies. Third, the theoretical framework we have developed from this review explicates the association of constructs in detail and points to new constructs that can be potentially sensible for further research to provide answers of concern under the review’s focus. This review and the articles of this special issue will contribute to the literature and lead business organisations and HR professionals towards facilitating dynamic changes to introduce intelligent technologies in gaining and securing competitive advantages.
Disclosure statement
No potential conflict of interest was reported by the authors.
Correction Statement
This article has been republished with minor changes. These changes do not impact the academic content of the article.
References
- Abraham, M., Niessen, C., Schnabel, C., Lorek, K., Grimm, V., Möslein, K., & Wrede, M. (2019). Electronic monitoring at work: The role of attitudes, functions, and perceived control for the acceptance of tracking technologies. Human Resource Management Journal, 29(4), 657–675. https://doi.org/https://doi.org/10.1111/1748-8583.12250
- Abubakar, A. M., Behravesh, E., Rezapouraghdam, H., & Yildiz, S. B. (2019). Applying artificial intelligence technique to predict knowledge hiding behavior. International Journal of Information Management, 49, 45–57. https://doi.org/https://doi.org/10.1016/j.ijinfomgt.2019.02.006
- Agar, N. (2019). How to be human in the digital economy. MIT Press.
- Agar, N. (2020). How to treat machines that might have minds. Philosophy & Technology, 33(2), 269–282. https://doi.org/https://doi.org/10.1007/s13347-019-00357-8
- Agrawal, A., Gans, J., & Goldfarb, A. (2017). What to expect from artificial intelligence. MIT Sloan Management Review, 58(3), 23-26. http://mitsmr.com/2jZdf1Y
- Aleksander, I. (2017). Partners of humans: A realistic assessment of the role of robots in the foreseeable future. Journal of Information Technology, 32(1), 1–9. https://doi.org/https://doi.org/10.1057/s41265-016-0032-4
- Aouadni, I., & Rebai, A. (2017). Decision support system based on genetic algorithm and multi-criteria satisfaction analysis (MUSA) method for measuring job satisfaction. Annals of Operations Research, 256(1), 3–20. https://doi.org/https://doi.org/10.1007/s10479-016-2154-z
- Azadeh, A., Yazdanparast, R., Abdolhossein Zadeh, S., & Keramati, A. (2018). An intelligent algorithm for optimising emergency department job and patient satisfaction. International Journal of Health Care Quality Assurance, 31(5), 374–390. https://doi.org/https://doi.org/10.1108/IJHCQA-06-2016-0086
- Azadeh, A., & Zarrin, M. (2016). An intelligent framework for productivity assessment and analysis of human resource from resilience engineering, motivational factors, HSE and ergonomics perspectives. Safety Science, 89, 55–71. https://doi.org/https://doi.org/10.1016/j.ssci.2016.06.001
- Bersin, J., & Chamorro-Premuzic, T. (2019). New ways to gauge talent and potential. MIT Sloan Management Review, 60(2), 1. https://mitsmr.com/2QLPcEN
- Bharadwaj, N., & Shipley, G. M. (2020). Salesperson communication effectiveness in a digital sales interaction. Industrial Marketing Management, 90, 106–112. https://doi.org/https://doi.org/10.1016/j.indmarman.2020.07.002
- Bititci, U., Cocca, P., & Ates, A. (2016). Impact of visual performance management systems on the performance management practices of organisations. International Journal of Production Research, 54(6), 1571–1593. https://doi.org/https://doi.org/10.1080/00207543.2015.1005770
- Botha, A. P. (2019). A mind model for intelligent machine innovation using future thinking principles. Journal of Manufacturing Technology Management, 30(8), 1250–1264. https://doi.org/https://doi.org/10.1108/JMTM-01-2018-0021
- Brougham, D., & Haar, J. (2020). Technological disruption and employment: The influence on job insecurity and turnover intentions: A multi-country study. Technological Forecasting and Social Change, 161, 120276. https://doi.org/https://doi.org/10.1016/j.techfore.2020.120276
- Budhwar, P., & Malik, A. (2020). Call for papers for the special issue on Leveraging artificial and human intelligence through Human Resource Management. Human Resource Management Review. Retrieved June 24, 2020, from https://www.journals.elsevier.com/human-resource-management-review/call-for-papers/leveraging-artificial-and-human-intelligence
- Bussler, L., & Davis, E. (2002). Information systems: The quiet revolution in human resource management. Journal of Computer Information Systems, 42(2), 17–20.
- Buxmann, P., Hess, T., & Thatcher, J. (2019). Call for papers, issue 1/2021. Business & Information Systems Engineering, 61(4), 545–547. https://doi.org/https://doi.org/10.1007/s12599-019-00606-2
- Castellacci, F., & Viñas-Bardolet, C. (2019). Internet use and job satisfaction. Computers in Human Behavior, 90, 141–152. https://doi.org/https://doi.org/10.1016/j.chb.2018.09.001
- Charlwood, A., & Guenole, N. (2021). Can HR adapt to the paradoxes of AI? Human Resource Management Journal, ahead-of-print. https://doi.org/https://doi.org/10.1111/1748-8583.12433
- Christofi, M., Vrontis, D., & Cadogan, J. W. (2021). Micro-foundational ambidexterity and multinational enterprises: a systematic review and a conceptual framework. International Business Review, 30(1), 101625. https://doi.org/https://doi.org/10.1016/j.ibusrev.2019.101625
- Connelly, C. E., Fieseler, C., Černe, M., Giessner, S. R., & Wong, S. I. (2020). Working in the digitised economy: HRM theory & practice. Human Resource Management Review, 31(1), ahead-of-print. https://doi.org/https://doi.org/10.1016/j.hrmr.2020.100762
- de Kervenoael, R., Hasan, R., Schwob, A., & Goh, E. (2020). Leveraging human-robot interaction in hospitality services: Incorporating the role of perceived value, empathy, and information sharing into visitors’ intentions to use social robots. Tourism Management, 78, 104042. https://doi.org/https://doi.org/10.1016/j.tourman.2019.104042
- De Stefano, V. (2019). ‘Negotiating the algorithm’: Automation, artificial intelligence and labour protection. Comparative Labor Law & Policy Journal, 41(1), 15-46. https://ssrn.com/abstract=3178233
- Del Giudice, M., Scuotto, V., Ballestra, L. V., & Pironti, M. (2021, this issue). Humanoid robot adoption and labour productivity: A perspective on ambidextrous product innovation routines. The International Journal of Human Resource Management, ahead-of-print. 1–27. https://doi.org/https://doi.org/10.1080/09585192.2021.1897643
- Duggan, J., Sherman, U., Carbery, R., & McDonnell, A. (2020). Algorithmic management and app‐work in the gig economy: A research agenda for employment relations and HRM. Human Resource Management Journal, 30(1), 114–132. https://doi.org/https://doi.org/10.1111/1748-8583.12258
- Dwivedi, Y. K., Hughes, L., Ismagilova, E., Aarts, G., Coombs, C., Crick, T., Duan, Y., Dwivedi, R., Edwards, J., Eirug, A., Galanos, V., Ilavarasan, P. V., Janssen, M., Jones, P., Kar, A. K., Kizgin, H., Kronemann, B., Lal, B., Lucini, B., … Williams, M. D. (2021). Artificial Intelligence (AI): Multidisciplinary perspectives on emerging challenges, opportunities, and agenda for research, practice and policy. International Journal of Information Management, 57, 101994. https://doi.org/https://doi.org/10.1016/j.ijinfomgt.2019.08.002
- Ererdi, C., Nurgabdeshov, A., Kozhakhmet, S., Rofcanin, Y., & Demirbag, M. (2021). International HRM in the context of uncertainty and crisis: A systematic review of literature (2000–2018). The International Journal of Human Resource Management, 1–39. ahead-of-print. https://doi.org/https://doi.org/10.1080/09585192.2020.1863247
- Glikson, E., & Woolley, A. W. (2020). Human trust in artificial intelligence: Review of empirical research. Academy of Management Annals, 14(2), 627–660. https://doi.org/https://doi.org/10.5465/annals.2018.0057
- Grønsund, T., & Aanestad, M. (2020). Augmenting the algorithm: Emerging human-in-the-loop work configurations. The Journal of Strategic Information Systems, 29(2), 101614. https://doi.org/https://doi.org/10.1016/j.jsis.2020.101614
- Henkel, A. P., Bromuri, S., Iren, D., & Urovi, V. (2020). Half human, half machine–augmenting service employees with AI for interpersonal emotion regulation. Journal of Service Management, 31(2), 247–5818. https://doi.org/https://doi.org/10.1108/JOSM-05-2019-0160
- Hohenstein, J., & Jung, M. (2020). AI as a moral crumple zone: The effects of AI-mediated communication on attribution and trust. Computers in Human Behavior, 106, 106190. https://doi.org/https://doi.org/10.1016/j.chb.2019.106190
- Houser, K. (2018). Chinese surveillance is literally getting in workers’ heads. Futurism. https://futurism.com/china-emotional-surveillance
- Huang, M. H., & Rust, R. T. (2018). Artificial intelligence in service. Journal of Service Research, 21(2), 155–172. https://doi.org/https://doi.org/10.1177/1094670517752459
- Huang, M. H., & Rust, R. T. (2020). Engaged to a robot? The role of AI in service. Journal of Service Research, 24(1), 30-41. https://doi.org/https://doi.org/10.1177/1094670520902266
- Huang, M. H., Rust, R., & Maksimovic, V. (2019). The feeling economy: Managing in the next generation of artificial intelligence (AI). California Management Review, 61(4), 43–65. https://doi.org/https://doi.org/10.1177/0008125619863436
- Jain, H., Padmanabhan, B., Pavlou, P. A. & Santanam, R.T. (Eds). (2018). Call for papers—Special Issue of Information Systems Research—Humans, algorithms, and augmented intelligence: The future of work, organisations, and society. Information Systems Research, 29, 250–251.
- Jaiswal, A., Arun, C. J., & Varma, A. (2021, this issue). Rebooting employees: Upskilling for artificial intelligence in multinational corporations. The International Journal of Human Resource Management, 1–30. ahead-of-print. https://doi.org/https://doi.org/10.1080/09585192.2021.1891114
- Kaplan, A., & Haenlein, M. (2020). Rulers of the world, unite! The challenges and opportunities of artificial intelligence. Business Horizons, 63(1), 37–50. https://doi.org/https://doi.org/10.1016/j.bushor.2019.09.003
- Karatop, B., Kubat, C., & Uygun, Ö. (2015). Talent management in manufacturing system using fuzzy logic approach. Computers & Industrial Engineering, 86, 127–136. https://doi.org/https://doi.org/10.1016/j.cie.2014.09.015
- Kiron, D., & Spindel, B. (2019). Rebooting work for a digital era. MIT Sloan Management Review. https://sloanreview.mit.edu/IBM-case
- Kshetri, N. (2021). Evolving uses of artificial intelligence in human resource management in emerging economies in the global South: Some preliminary evidence. Management Research Review, 44(7), 970–990. https://doi.org/https://doi.org/10.1108/MRR-03-2020-0168
- Larivière, B., Bowen, D., Andreassen, T. W., Kunz, W., Sirianni, N. J., Voss, C., Wünderlich, N. V., & De Keyser, A. (2017). ‘Service Encounter 2.0’: An investigation into the roles of technology, employees and customers. Journal of Business Research, 79, 238–246. https://doi.org/https://doi.org/10.1016/j.jbusres.2017.03.008
- Li, J. J., Bonn, M. A., & Ye, B. H. (2019). Hotel employee’s artificial intelligence and robotics awareness and its impact on turnover intention: The moderating roles of perceived organisational support and competitive psychological climate. Tourism Management, 73, 172–181. https://doi.org/https://doi.org/10.1016/j.tourman.2019.02.006
- Li, J., Liu, M., & Liu, X. (2016). Why do employees resist knowledge management systems? An empirical study from the status quo bias and inertia perspectives. Computers in Human Behavior, 65, 189–200. https://doi.org/https://doi.org/10.1016/j.chb.2016.08.028
- Lu, V. N., Wirtz, J., Kunz, W. H., Paluch, S., Gruber, T., Martins, A., & Patterson, P. G. (2020). Service robots, customers and service employees: What can we learn from the academic literature and where are the gaps? Journal of Service Theory and Practice, 30(3), 361–391. https://doi.org/https://doi.org/10.1108/JSTP-04-2019-0088
- Maedche, A., Legner, C., Benlian, A., Berger, B., Gimpel, H., Hess, T., Hinz, O., Morana, S., & Söllner, M. (2019). AI-based digital assistants. Business & Information Systems Engineering, 61(4), 535–544. https://doi.org/https://doi.org/10.1007/s12599-019-00600-8
- Malik, A., Budhwar, P., Patel, C., & Srikanth, N. R. (2020c, this issue). May the bots be with you! Delivering HR cost-effectiveness and individualised employee experiences in an MNE. The International Journal of Human Resource Management, 1–31. ahead-of-print. https://doi.org/https://doi.org/10.1080/09585192.2020.1859582
- Malik, A., Budhwar, P., & Srikanth, N. R. (2020a). Gig economy, 4IR and artificial intelligence: Rethinking strategic HRM. In P. Kumar, A. Agrawal, & P. Budhwar (Eds.), Human & technological resource management (HTRM): New insights into revolution 4.0 (pp. 75–88). Emerald Publishing Limited.
- Malik, A., De Silva, M. T. T., Budhwar, P., & Srikanth, N. R. (2021). Elevating talents’ experience through innovative artificial intelligence-mediated knowledge sharing: Evidence from an IT-multinational enterprise. Journal of International Management, 27(4), 100871. https://doi.org/https://doi.org/10.1016/j.intman.2021.100871
- Malik, A., Sreenivasan, P., & De Silva, T. (2022). Artificial intelligence, employee engagement, experience and HRM. In A. Malik (Ed.), Strategic human resource management and employment relations: An international perspective (2nd ed.). Springer.
- Malik, A., Srikanth, N. R., & Budhwar, P. (2020b). Digitisation, artificial intelligence (AI) and HRM. In J. Crawshaw, P. Budhwar, & A Davis (Eds.), Human resource management: Strategic and international perspectives (pp. 88–111). Sage.
- Manoharan, T. R., Muralidharan, C., & Deshmukh, S. G. (2011). An integrated fuzzy multi-attribute decision-making model for employees’ performance appraisal. The International Journal of Human Resource Management, 22(3), 722–745. https://doi.org/https://doi.org/10.1080/09585192.2011.543763
- McColl, R., & Michelotti, M. (2019). Sorry, could you repeat the question? Exploring video‐interview recruitment practice in HRM. Human Resource Management Journal, 29(4), 637–656. https://doi.org/https://doi.org/10.1111/1748-8583.12249
- Mehrabad, M. S., & Brojeny, M. F. (2007). The development of an expert system for effective selection and appointment of the jobs applicants in human resource management. Computers & Industrial Engineering, 53(2), 306–312.
- Meijerink, J. G., Boons, M., Keegan, A., & Marler, J. (2018). Call for papers for the special issue on Digitization and the transformation of human resource management. International Journal of Human Resource Management. https://doi.org/https://doi.org/10.1080/09585192.2018.1503845
- Metcalf, L., Askay, D. A., & Rosenberg, L. B. (2019). Keeping humans in the loop: Pooling knowledge through artificial swarm intelligence to improve business decision making. California Management Review, 61(4), 84–109. https://doi.org/https://doi.org/10.1177/0008125619862256
- Moosajee, N. (2019). Fix AI’s racist, sexist bias. 14 March, https://mg.co.za/article/2019-03-14-fix-ais-racist-sexist-bias/
- Nguyen, T. M., & Malik, A. (2021). A two‐wave cross‐lagged study on AI service quality: The moderating effects of the job level and job role. British Journal of Management. ahead-of-print. https://doi.org/https://doi.org/10.1111/1467-8551.12540
- Nguyen, T. M., & Malik, A. (2022). ‘Impact of knowledge sharing on employees’ service quality: The moderating role of artificial intelligence. International Marketing Review, ahead-of-print. https://doi.org/https://doi.org/10.1108/IMR-02-2021-0078
- Nura, A. A., & Osman, N. H. (2013). Gauging the effect of performance management and technology based human resource management on employee retention: The perspective of academics in higher educational institutions in Sokoto State Nigeria. Asian Social Science, 9(15), 295.
- Oesterreich, T. D., Teuteberg, F., Bensberg, F., & Buscher, G. (2019). The controlling profession in the digital age: Understanding the impact of digitisation on the controller’s job roles, skills and competences. International Journal of Accounting Information Systems, 35(C), 100432. https://doi.org/https://doi.org/10.1016/j.accinf.2019.100432
- Pan, Y., Froese, F., Liu, N., Hu, Y., & Ye, M. (2021, this issue). The adoption of artificial intelligence in employee recruitment: The influence of contextual factors. The International Journal of Human Resource Management, 1–23. ahead-of-print. https://doi.org/https://doi.org/10.1080/09585192.2021.1879206
- Paul, J., & Criado, A. R. (2020). The art of writing a literature review: What do we know and what do we need to know? International Business Review, 29(4), 101717. https://doi.org/https://doi.org/10.1016/j.ibusrev.2020.101717
- Pejic-Bach, M., Bertoncel, T., Meško, M., & Krstić, Ž. (2020). Text mining of industry 4.0 job advertisements. International Journal of Information Management, 50, 416–431. https://doi.org/https://doi.org/10.1016/j.ijinfomgt.2019.07.014
- Pessach, D., Singer, G., Avrahami, D., Chalutz Ben-Gal, H., Shmueli, E., & Ben-Gal, I. (2020). Employees recruitment: A prescriptive analytics approach via machine learning and mathematical programming. Decision Support Systems, 134, 113290. https://doi.org/https://doi.org/10.1016/j.dss.2020.113290
- Prentice, C., Dominique Lopes, S., & Wang, X. (2020a). The impact of artificial intelligence and employee service quality on customer satisfaction and loyalty. Journal of Hospitality Marketing & Management, 29(7), 739–756. https://doi.org/https://doi.org/10.1080/19368623.2020.1722304
- Prentice, C., Dominique Lopes, S., & Wang, X. (2020b). Emotional intelligence or artificial intelligence–an employee perspective. Journal of Hospitality Marketing & Management, 29(4), 377–403. https://doi.org/https://doi.org/10.1080/19368623.2019.1647124
- Prentice, C., & Nguyen, M. (2020). Engaging and retaining customers with AI and employee service. Journal of Retailing and Consumer Services, 56, 102186. https://doi.org/https://doi.org/10.1016/j.jretconser.2020.102186
- Prikshat, V., Malik, A., & Budhwar, P. (2021). AI-augmented HRM: Antecedents, assimilation and multilevel consequences. Human Resource Management Review, 100860. ahead-of-print.
- Rampersad, G. (2020). Robot will take your job: Innovation for an era of artificial intelligence. Journal of Business Research, 116, 68–74. https://doi.org/https://doi.org/10.1016/j.jbusres.2020.05.019
- Ransbotham, S., Kiron, D., Gerbert, P., & Reeves, M. (2017). Reshaping business with artificial intelligence: Closing the gap between ambition and action. MIT Sloan Management Review, 59(1), 1-17. https://search.proquest.com/docview/1950374030?accountid=10499
- Robert, L. P., Pierce, C., Marquis, L., Kim, S., & Alahmad, R. (2020). Designing fair AI for managing employees in organisations: A review, critique, and design agenda. Human–Computer Interaction, 35(5-6), 545–575.
- Shank, D. B., Graves, C., Gott, A., Gamez, P., & Rodriguez, S. (2019). Feeling our way to machine minds: People’s emotions when perceiving mind in artificial intelligence. Computers in Human Behavior, 98, 256–266. https://doi.org/https://doi.org/10.1016/j.chb.2019.04.001
- Sitzmann, T., & Weinhardt, J. M. (2019). Approaching evaluation from a multilevel perspective: A comprehensive analysis of the indicators of training effectiveness. Human Resource Management Review, 29(2), 253–269. https://doi.org/https://doi.org/10.1016/j.hrmr.2017.04.001
- Snyder, H. (2019). Literature review as a research methodology: An overview and guidelines. Journal of Business Research, 104, 333–339. https://doi.org/https://doi.org/10.1016/j.jbusres.2019.07.039
- Suen, H.-Y., Chen, M. Y.-C., & Lu, S.-H. (2019). Does the use of synchrony and artificial intelligence in video interviews affect interview ratings and applicant attitudes? Computers in Human Behavior, 98, 93–101. https://doi.org/https://doi.org/10.1016/j.chb.2019.04.012
- Suseno, Y., Chang, C., Hudik, M., & Fang, E. S. (2021, this issue). Beliefs, anxiety and change readiness for artificial intelligence adoption among human resource managers: The moderating role of high-performance work systems. The International Journal of Human Resource Management, ahead-of-print. 1–28. https://doi.org/https://doi.org/10.1080/09585192.2021.1931408
- Tambe, P., Cappelli, P., & Yakubovich, V. (2019). Artificial intelligence in human resources management: Challenges and a path forward. California Management Review, 61(4), 15–42. https://doi.org/https://doi.org/10.1177/0008125619867910
- Tarafdar, M., Beath, C. M., & Ross, J. W. (2019). Using AI to enhance business operations. MIT Sloan Management Review, 60(4), 37–44.
- Torres, E. N., & Mejia, C. (2017). Asynchronous video interviews in the hospitality industry: Considerations for virtual employee selection. International Journal of Hospitality Management, 61, 4–13. https://doi.org/https://doi.org/10.1016/j.ijhm.2016.10.012
- Tranfield, D., Denyer, D., & Smart, P. (2003). Towards a methodology for developing evidence‐informed management knowledge by means of systematic review. British Journal of Management, 14(3), 207–222. https://doi.org/https://doi.org/10.1111/1467-8551.00375
- van Esch, P., Black, J. S., & Ferolie, J. (2019). Marketing AI recruitment: The next phase in job application and selection. Computers in Human Behavior, 90, 215–222. https://doi.org/https://doi.org/10.1016/j.chb.2018.09.009
- van Esch, P., Stewart Black, J., Franklin, D., & Harder, M. (2020). AI-enabled biometrics in recruiting: Insights from marketers for managers. Australasian Marketing Journal. 29(3), 225–234. https://doi.org/https://doi.org/10.1016/j.ausmj.2020.04.003
- Vrontis, D., Christofi, M., Pereira, V., Tarba, S., Makrides, A., & Trichina, E. (2021, this issue). Artificial intelligence, robotics, advanced technologies and human resource management: A systematic review. The International Journal of Human Resource Management, 1–30. ahead-of-print. https://doi.org/https://doi.org/10.1080/09585192.2020.1871398
- Wilson, H. J., & Daugherty, P. R. (2018). Collaborative intelligence: Humans and AI are joining forces. Harvard Business Review, 96(4), 114–123.
- Wilson, H. J., Daugherty, P., & Bianzino, N. (2017). The jobs that artificial intelligence will create. MIT Sloan Management Review, 58(4), 14. http://mitsmr.com/2odREFJ
- Wirtz, J. (2019). Organisational ambidexterity: Cost-effective service excellence, service robots, and artificial intelligence. Organizational Dynamics, 49(3), 1–9.
- Wirtz, J., Patterson, P. G., Kunz, W. H., Gruber, T., Lu, V. N., Paluch, S., & Martins, A. (2018). Brave new world: Service robots in the frontline. Journal of Service Management, 29(5), 907–931. https://doi.org/https://doi.org/10.1108/JOSM-04-2018-0119
- Wong, S. L., & Liu, Q. (2019). Emotion recognition is China’s new surveillance craze. November 1, www.ft.com/content/68155560-fbd1-11e9-a354-36acbbb0d9b6