Abstract
Learning a foreign language is a long-term process requiring persistence and a willingness to engage in activities that will help develop communicative competence. An important role on the way to achieving linguistic proficiency is played by L2 grit. However, foreign language learners differ in the intensity of this trait. We identify the most important predictors of L2 grit in the online language learning context. Basing on a literature review, we first identify possible predictors in the generic process of L2 learning, including language mindsets as well as two general psychological dispositions: a sense of autonomy and curiosity, and subsequently one predictor specifically related to the virtual learning context, i.e., readiness for online learning. A multiple linear regression model built using questionnaire data collected from 615 learners from 69 countries enrolled in language courses taught in a remote or hybrid mode reveals that L2 grit is most determined by two dimensions of readiness for online learning, and to a lesser extent by learners’ autonomy and curiosity, and significantly and positively correlates with these factors. The regression model explains 48% of variance in the dependent variable. A subsequent psychological network analysis, one of the first applications of this method in the field of SLA, permitted estimating the relative importance of these factors in the complex network of associations, indicating equally strong direct connections between L2 grit and both dimensions of readiness for online learning, and a much weaker edge linking L2 grit with autonomy. The findings have practical implications for language teachers in remote and hybrid settings.
Introduction
Learning a foreign language constitutes a long-term, dynamic process involving many challenges, with numerous factors influencing its course and progress. Viewed from a complex dynamic systems perspective, these factors form complex, interrelated and interdependent systems that significantly shape the development of second language competence (Freeborn et al., Citation2022; Larsen-Freeman & Cameron, Citation2008; Mercer, Citation2018; Oxford, Citation2016a, Citation2016b). Some of thee are closely related to the learners, their cognitive resources (e.g. memory, need for cognition, attention, learning strategies), motivation to learn, as well as personality traits and other psychological dispositions, especially those whose role in the field of foreign language acquisition is nowadays being emphasised by positive psychology. Existing research reveals that these may include grit, creativity, courage, language mindsets, basic psychological needs, emotional intelligence, engagement in learning and flow experience, positive emotions and learning enjoyment, alongside others that affect learners’ wellbeing (Dewaele et al., Citation2019; MacIntyre et al., Citation2019). These factors constitute the internal resources of the learner.
Learning a second language takes place under exposure to the wider context and external factors, such as the learning environment, access to existing resources, relationship with the teacher, opportunities to practise the language (Mercer, Citation2018; Oxford, Citation2016a), and the mode of learning—remote, on-site, or hybrid. The latter factor has become particularly important in the recent years, with the significant increase in the use of online language learning solutions, leading to a “ubiquitous” autonomous learning experience through either mobile-assisted language learning (MALL) or informal learning on the one hand (Kalyaniwala & Ciekanski, 2019), and post-COVID normalisation of online language courses on the other. The new learning settings to which stakeholders have gradually been adapting present new challenges and requirements for both learners and teachers.
Successful and rewarding L2 learning seems to involve possessing and/or developing particular psychological dispositions. Among these, increasing attention has recently been paid to grit (e.g., Changlek & Palanukulwong; 2015; Feng & Papi, Citation2020; Khajavy et al., Citation2021; Khajavy & Aghaee, Citation2022; Sudina & Plonsky, Citation2021; Teimouri et al., Citation2022; Wei et al., Citation2019), which denotes perseverance in pursuing specific goals, combined with consistency of interest, particularly stability thereof (Duckworth et al., Citation2007).
Grit tends to be an important individual trait, which enables a person to pursue more demanding goals and challenges to foster personal growth (Duckworth et al., Citation2007). Research suggests that grit may predict success better than cognitive ability (Duckworth et al., Citation2007; Duckworth & Quinn, Citation2009). With the development of the concept of language-domain-specific grit (Teimouri et al., Citation2022), more research has emerged on the role of grit in language learning. However, knowledge on the subject is still limited, leading to controversies around the definition of this psychological disposition and its measurement (Elahi Shirvan, Lou, Shahnama & Yazdanmehr, Citation2021; Khajavy et al., Citation2021; Li & Yang, Citation2023; Oxford & Khajavy, Citation2021). Although a handful of studies have provided some insight into the contribution of L2 grit to the development of L2 competence, we still do not know the reasons why learners demonstrate different levels of this trait and subsequently the extent of their L2 achievement (Elahi Shirvan & Alamer, Citation2022; Teimouri, Sudina & Plonsky, Citation2021).
In this study, we identify the most important predictors of L2 grit in the online and hybrid language learning condition, an environment which presents many new challenges for learners. Based on a literature review, we distinguish a group of putative antecedents of L2 grit and investigate their relative role in its development. Lastly, to provide a deeper insight into the complex system of the interconnected factors, we apply psychological network analysis (Epskamp et al., Citation2018), whose application—like computational social network analysis (Paradowski et al., Citation2021a,b, Citation2022)—in SLA research has until recently been relatively rare.
Literature review
From domain-general grit to language-domain-specific grit
Grit is a positive personality disposition and non-cognitive skill which contributes to personal growth, lifelong learning and educational attainment. It consists of two dimensions: persistence of effort (PE) and consistency of interest (CI). Persistence of effort represents an individual’s tendency to work hard to achieve long-term goals despite challenges and failures, whereas consistency of interest refers to persistent and voluntary activity to achieve these long-term goals and develop specific competencies. Both are important contributing factors to performance (Duckworth et al., Citation2007, Citation2019; Duckworth & Quinn, Citation2009).
In the field of SLA research, early scholarship was dominated by the perception of grit as a general personality trait. A pioneering study examining grit among language learners was conducted by Lake (Citation2013), who investigated the relationship between grit and a range of positive personality dispositions among female Japanese university students learning English. Those with higher grit were more willing to devote time and effort to L2 learning. Changlek and Palanukulwong (Citation2015) selected a group of Thai students exhibiting higher grit levels to investigate their motivational characteristics. They found that those with high academic performance were grittier and revealed lower levels of anxiety. Similar results were obtained by Wei et al. (Citation2019), who reported a relationship between grit intensity and English language performance in secondary school students in China. Interestingly, Yamashita (Citation2018) did not find a similar association among Japanese students. Recently, a study of Taiwanese EFL undergraduate students found that grit predicted willingness to communicate in the L2, with the relationship stronger in offline (in- and out-of-class) than in digital settings (Lee & Hsieh, Citation2019). All these studies used scales measuring domain-general grit (Duckworth et al., Citation2007; Duckworth & Quinn, Citation2009) without considering the specific context of L2 learning.
A turning point in grit research came with a finding by Cormier et al. (Citation2019) confirming Duckworth’s initial conjecture that grit is a context-dependent disposition related to individual areas of human functioning. This gave the impetus for the development of scales measuring language-domain-specific grit. The first such instrument, an adaptation of Duckworth and colleagues’ (Citation2007) scale, was developed by Teimouri et al. (Citation2022). They found that their new scale correlated better with L2 learners’ performance than the originally used general Grit-O scale, as evidenced by large attenuation-corrected correlations for L2 grit. A separate scale was proposed by Alamer (Citation2021), adapting not only the original Grit Scale (Duckworth et al., Citation2007), but also the Academic Grit Scale (AGS; Clark & Malecki, Citation2019).
It is important to note that studies that had measured domain-general grit showed either a weak (e.g., Wei et al., Citation2019) or no association (e.g., Yamashita, Citation2018) with L2 achievement. Conversely, research relying on measures of L2 domain-specific grit revealed its positive and significant associations with L2 achievement and proficiency (e.g., Alamer, Citation2021; Sudina & Plonsky, Citation2021; Sudina et al., Citation2021; Teimouri et al., Citation2022; Mikami, Citation2023). Recently, in a study of 289 Chinese learners of L2 German, Li and Yang (Citation2023) showed that when domain-general grit was combined with L2 grit in the same regression model, the former completely lost its predictive power on all dimensions of L2 achievement, while the latter remained a strong positive predictor. These results indicate that a domain-specific measure of grit increases its construct validity.
Existing small-scale research on L2 grit hardly addresses the dynamics of the relationship of this construct with other variables (Elahi Shirvan, Taherian & Yazdanmeh, Citation2021). Additionally, while some studies have looked at how instructional practices (such as implementing a flipped EFL reading classroom; Khabir et al., Citation2022) can improve learners’ grit, little is known about the determinants of its differing levels, or the role of this construct in online language learning. One recent study (Zarrinabadi et al., Citation2022b) found that persistence of effort positively predicted surplus value of CALL, while consistency of interest predicted the degree of exhibition to CALL, but otherwise there is a dearth of pertinent research in this field.
Language mindsets
The concept of language mindset implies that people have implicit theories about individual characteristics such as intelligence or personality traits (Dweck, Citation2006; Dweck & Leggett, Citation1988). One can manifest two flavours of mindset (implicit theories): a growth mindset or incremental theory, which assumes that a person’s ability can change and may be improved through extensive work and the use of appropriate strategies, and a fixed mindset or entity theory, rooted in the belief that abilities are permanent and cannot be modified (ibid.). The two systems are expected to lead to different patterns of motivation and learning behaviour (Lou, Chaffe & Noels, 2021).
In the field of language learning and teaching, the concept of mindsets appeared in the 2010s (Lou & Noels, Citation2017; Mercer & Ryan, Citation2010; Noels & Lou, Citation2015), when it was recognised that mindsets are not universal or general, but are dependent on a specific context or domain of human functioning (Dweck et al., Citation1995), meaning that it is possible to have a weak growth language mindset, but at the same time a strong growth mindset in other domains of intellectual activity, or vice versa (Ryan & Mercer, Citation2012). Language mindsets are related to, but also separate from, other mindsets, correlating weakly with mindsets related to general intelligence and other specific abilities, such as athletic activities or mathematics (Lou & Noels, Citation2017). Unlike general intelligence mindsets, language mindsets tend to be a more direct and stronger predictor of language learning motivation (Lou & Noels, Citation2017).
An increasing number of studies have focused on the impact of language mindsets on language learning and teaching (e.g., Lou & Noels, Citation2020a, Citation2020c; Lou & Zarrinabadi, Citation2022; Zarrinabadi et al., Citation2022a). The growth language mindset represents the belief that an individual’s language abilities are malleable and hence can be improved through effort and the use of appropriate learning strategies, whereas the fixed language mindset expresses the belief that language abilities are relatively stable and cannot be changed (Lou & Noels, Citation2019, Citation2020a, Citation2020c; Noels & Lou, Citation2015).
Autonomy
Autonomy is one of the three basic psychological needs outlined by the Self-Determination Theory (Deci & Ryan, Citation2000; Ryan & Deci, Citation2000, Citation2017). Satisfying these is intrinsically motivating and important for development and well-being (ibid.). Autonomy means acting in accordance with one’s own beliefs, authentic interests and values. The degree of autonomy associated with the regulation of behaviour is, in turn, of great importance for performance, persistence and well-being. It is thus an important aspect regulating motivation (Deci & Ryan, Citation2000; Ryan & Deci, Citation2000, Citation2017).
Researchers have confirmed the relationship between autonomy and motivation in the L2 learning process (e.g., Fukuda et al., Citation2011; Spratt et al., Citation2002; Ushioda, Citation1996, Citation2013), especially in online spaces (Godwin-Jones, Citation2019), with autonomy being a more important predictor of proficiency than language anxiety and motivation (Liu, Citation2012). Digital learning contexts have been posited to both require (Reinders & White, Citation2016) and have the potential to enhance learners’ autonomy, with the affordances ranging from access to resources “anytime, anywhere,” to raising students’ awareness of the learning process (Smith & Craig, Citation2013), to enhancing positive attitudes towards autonomous learning itself (Sato et al., Citation2020), although the potential danger has also been pointed out of technology giving students a false sense of development (Reinders & White, Citation2011).
Curiosity
Curiosity represents an important aspect of human motivation (Izard, Citation1977) and is one of the fundamental strengths and personality traits (Peterson & Seligman, Citation2004). It is defined as the desire to acquire new information and experiences that motivates people to explore their physical and social environment. Curiosity is seen as a derivative or component of intrinsic motivation (Ryan & Deci, Citation2000). Kashdan et al. (Citation2009) propose that curiosity can be defined as the recognition, acceptance and pursuit of knowledge and new experiences, and identify its two components: the motivation to seek knowledge and new experiences (stretching) and the willingness to accept new and unpredictable situations (embracing).
In the domain of second language acquisition, the role of curiosity has not yet been thoroughly investigated. A limited number of studies have reported its positive relationship with willingness to communicate, reading comprehension, and L2 development in the linguistic, socio-cultural and pragmatic areas (e.g., Gurning & Siregar, Citation2017; Mahmoodzadeh & Khajavy, Citation2018; Takkaç-Tulgar, Citation2018; Lee, Citation2022).
Readiness for online learning
A remote learning environment entails many challenges, markedly distinct from face-to-face learning settings. Not only does the learner need to possess technological skills to be able to efficiently use online platforms and educational tools, but in-class interactions within this context also tend to be quite different.
On the one hand, online learning settings provide students with flexibility in terms of where and when they study, and—compared with the face-to-face mode—give them more control over how they complete their courses. On the other, in a remote learning environment the students often have to control the learning process themselves in terms of learning pace and intensity, the amount of time they spend studying, content scope, and the type of media they use. They also have to control to what extent they can concentrate during lessons and actively participate in them without getting distracted. Remote study, therefore, requires a variety of dispositions or traits, which together constitute readiness for online learning (Hung et al., Citation2010; Hung, Citation2016; Joosten & Cusatis, Citation2020; Qin et al., 2022).
Readiness for online learning is now perceived as one of the most important preconditions for effective learning and achievement in an online environment. Researchers distinguish several constituent dimensions (Hung et al., Citation2010; Hung, Citation2016), which reflect a highly developed self-regulation, although the online learning environment may require a higher level of autonomy than face-to-face classes (Cheon et al., Citation2021; Guri-Rosenblit, Citation2018; Jarynowski et al., Citation2021). Keramati et al. (Citation2011) found that readiness for online learning was a moderator in the relationship between learning-related factors and online learning performance, with self-directed learning the most important predictor of achievement from among the many dimensions (Kırmızı et al. Citation2015). Torun (Citation2020) similarly found two dimensions of readiness for online learning—self-directed learning and motivation—to be effective predictors of academic performance.
Self-directed learning is conceptualised as a process in which individuals take the initiative in identifying their learning needs, setting learning goals, determining the resources needed for learning, selecting and implementing appropriate learning strategies, and evaluating learning outcomes (Knowles, Citation1975; Knowles et al., Citation2020). A self-directed learner is eager to engage in challenging activities and consciously seeks to develop personal knowledge and skills for effective use (Gibbons, Citation2002). Thus researchers (e.g., Knowles et al., Citation2020; Du Toit-Brits, Citation2020) point out that a self-directed individual is at the same time a person who exhibits a high level of autonomy and perseverance in learning, without feeling discouraged when faced with problems, which they instead perceive as challenges. Such a learner displays a high level of curiosity, is goal-oriented, and enjoys learning. Song and Hill (Citation2007) perceive self-directed learning as both a personal trait and a learning process.
Motivation likewise has a significant impact on students’ attitudes and behaviour (Ryan & Deci, Citation2000). Learning proceeds through an interplay between cognitive factors, personality traits, and motivation, and they tend to be inseparable (Pintrich & Schunk, Citation2014; Stefanou & Salisbury-Glennon, Citation2002). Having a motivational orientation—whether intrinsic or extrinsic—has a significant impact on learners’ academic performance. In computer-supported learning, Saadé et al. (Citation2007) found that intrinsic and extrinsic motivation plays an important role in whether the learner achieves L2 progress or experiences failure. As a dimension of readiness for online learning, motivation can considerably contribute to learners’ efforts to follow their own desires and strengthen their ability to learn and acquire information. Motivation to learn ensures that, when faced with a transition from a face-to-face to a remote learning environment, students remain eager to learn.
The present study
Learning a foreign language is a long-term process that requires systematic effort in order to make progress. In this process, it is thus important to be persistent and willing to engage in activities that will help develop communicative competence. On the way to achieving linguistic proficiency, L2 grit, therefore, plays an extremely important role. However, foreign language learners differ in the intensity of this trait. Pawlak, Csizér, Kruk and Zawodniak (2022) found such, albeit insignificant, differences in separate age groups of students. This motivates a search for factors that would explain this variation among the psychological dispositions that are active in the process of L2 learning.
Until now, little research has been conducted on the role of grit in the context of online learning. A study by Aparicio et al. (Citation2017) indicated that in a remote learning environment, grit significantly correlated with learners’ performance and satisfaction with this mode of learning. These results were confirmed by McClendon et al. (Citation2017) revealing that grit, as well as mindsets, positively correlated not only with learner performance, but also engagement in online activities. Similar results were obtained by Liu et al. (Citation2021), who found that grit was significantly associated with academic performance in mobile EFL learning. Lan and Moscardino (Citation2019) found that grit was a core determinant of learning success and found it to be an important psychological mechanism that sparked and guided learners’ activity, while Yang (Citation2021) reported that higher levels of learners’ grit fostered higher class enjoyment, which in turn was associated with a sense of students’ needs being met.
Although these studies suggest that grit is important in the context of online learning, many of them measured general-domain rather than language-domain specific grit. Nor were the predictors of L2 grit analysed in the online learning context.
Learners’ language mindsets are related to their perceptions of what they can achieve by making an educational effort (Lou & Noels, Citation2017). Those who are convinced that they can develop their language skills believe that effort is an effective way to improve their proficiency. They feel that the more time they spend studying, the better their language performance will be. In contrast, those who assume that language ability is unchangeable and fixed, may believe that undue effort reflects their lack of natural ability, and that showing to others how much time they spend on learning can actually make them feel less capable. Since time spent studying is one of the strongest predictors of language achievement, positive beliefs about learning effort are considered a key motivational factor for language success (Csizér & Dörnyei, 2005). These beliefs about the meaning of the effort undertaken and one’s own L2 abilities may determine the level of L2 grit. Lou and Zarrinabadi (Citation2022:140) therefore suggest that in particular growth mindsets may “act as fuel to sustain grit for achieving long-term goals.” However, previous research has not provided a clear and unambiguous pattern of these relationships.
Language education has gradually been shifting towards a learner-centred approach. This means that the learner has more choice and is encouraged to take charge of their own development and pace of language progress. Self-directed learning is facilitated by the increasing availability of multimedia resources. As long as the learner perceives L2 learning as a personal choice and a self-determined goal with personally chosen activities, they will be able to make a persistent and active effort to achieve this learning objective. Consequently, they may have a stronger sense of autonomy. Jin and Kim (Citation2017) found grit to significantly and positively correlate with young adults’ satisfaction with their basic psychological needs being met, as well as with the need for autonomy and competence, along with subjective well-being. Being more autonomous may therefore explain a greater disposition to be gritty in meeting an L2 learning goal. Yet, little is known about the relationship between L2 grit and sense of autonomy.
L2 grit, manifest in perseverance in overcoming challenges, and consistent effort that will facilitate linguistic development, may stem from the desire to expand one’s knowledge, develop skills and acquire new experiences, i.e. from curiosity, which involves setting particular goals and tasks to fill the knowledge gap. So far, however, the role of curiosity in language learning has received little attention. Its relationship with L2 grit is also unknown.
The remote learning environment entails additional challenges, so it is important that the learner be equipped with appropriate skills and personal qualities. Two dimensions of readiness for online learning seem particularly important in this context: motivation and self-directed learning. Some previous studies have shown a positive relationship between motivation and L2 grit (e.g., Changlek & Palanukulwong, Citation2015; Teimouri et al., Citation2022). Yet, little is known about the association between L2 grit and self-directed learning, even though we may expect this factor to have an important influence.
Taking all these rationales into account, we pose the following research questions:
RQ1: Which characteristics from among language mindsets, curiosity, autonomy, and readiness for online learning measured by its two dimensions—self-directed learning and motivation—determine language-domain-specific grit in remote and hybrid learning settings?
RQ2: What are the relationships between L2 grit and these variables? Which of these factors are particularly important in the complex network of associations with L2 grit?
Materials and methods
Participants
A specially designed online survey was completed between October 2021 and July 2022 by 615 respondents who were learning 33 different foreign languages while participating in online or hybrid courses, with a 65.8% survey completion rate. The learners came from six continents; a map of respondents’ locations is provided in . The sample was dominated by female participants (almost 77%). The mean age was 29.9 years (SD = 14.04), range 18–84, with nearly 56% of the respondents under 25. More than 58% of the learners declared having no previous experience with remote language learning. The participants were most frequently learning in a synchronous (nearly 63%) or mixed mode (almost 29%). The largest group were learners at B2 level (almost 25%), according to the classification of the Common European Framework of Reference for Languages (Council of Europe, Citation2020), as well as beginners at A1 level (almost 20%). Almost 63% of the respondents declared learning a language other than English (LOTE). The participant sociodemographics are displayed in .
Figure 1. Map of respondents’ locations.
Eckert IV pseudocylindrical map projection of the Earth, with respondents’ locations plotted.
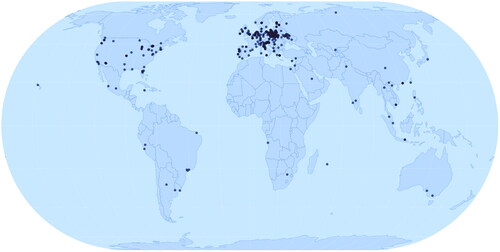
Table 1. Socio-demographic characteristics of the participants (N = 615).
Measures
To identify predictors of language-domain-specific grit in remote and hybrid learning settings from among the independent variables, we used a set of commonly used, reliable and valid scales along with one brand-new custom-made instrument constructed specially for this research (Appendix 1). All the scales were measured on a 6-point Likert scale ranging from “strongly disagree” (1) to “strongly agree” (6). The respondents were asked to indicate their native language(s) and subsequently to specify the language(s) they were learning remotely.
L2 grit scale
To assess language-domain-specific grit, we used an adapted version of the L2 grit scale developed by Teimouri, Plonsky and Tabandeh (2020). The scale is composed of 9 items and measures two components of L2 grit, i.e. L2 learners’ consistency of interest, and perseverance of effort in learning an additional language. Consistency of interest, assessed with 5 items, captures changes in learners’ interest in the course of L2 learning (e.g., “My interest in learning a foreign language changes from year to year”), whereas persistence of effort, assessed with 4 items, reflects the extent to which learners are persistent in achieving their L2 goals (e.g., “I will not allow anything to stop me from my progress in learning a foreign language”). The adaptation consisted in rephrasing two items, in consultation with one of the authors of the original scale: original “I have been obsessed with learning English in the past but later lost interest” replaced with “I was obsessed with learning a foreign language in the past but recently I have lost interest,” and “I put much time and effort into improving my English language weaknesses” replaced with “I put much time and effort into overcoming my foreign language weaknesses.” In this study, we obtained good internal consistency (Cronbach’s α and McDonald’s ωh = .84, Guttman’s λ6 = .87), surpassing that reported in the validation study by the original authors (Cronbach’s α = .80).
Language mindsets inventory
To measure language mindsets referring to beliefs about malleable or fixed ability to learn a foreign language, we used the Language Mindsets Inventory (LMI) by Lou and Noels (Citation2017). The 6-item scale comprises two dimensions, growth and fixed mindsets, each measured with three items (e.g., “I can always improve how good I am at learning new languages” for growth mindsets, and “To be honest, I can’t really change my ability to learn and use new languages” for fixed mindsets). In the current study, the growth language mindset subscale had good reliability (Cronbach’s α = .81, McDonald’s ωh = .82, Guttman’s λ6 = .75), with the fixed language mindset subscale characterised by a slightly lower but still acceptable internal consistency (Cronbach’s α and McDonald’s ωh = .77, Guttman’s λ6 = .69).
Curiosity and exploration inventory-II
Curiosity and Exploration Inventory-II (CEI-II, Kashdan et al., Citation2009) was applied to measure curiosity with its two dimensions, stretching, which reflects a motivation to seek knowledge and new challenging experiences (e.g., “In new situations, I actively seek out as much information as I can”), and embracing, which represents general willingness to be engaged in various activities (e.g., “I prefer jobs that are excitingly unpredictable”). The scale consists of 10 items (five for each dimension). In this study, curiosity was analysed as a single construct without dividing it into its two components. The scale was characterised by good internal consistency (Cronbach’s α, McDonald’s ωh and Guttman’s λ6 = .85), which was the same as that obtained by the original authors (Cronbach’s α = .85).
Autonomy scale
Autonomy was assessed with the subscale from the Basic Psychological Need Satisfaction and Frustration Scale (BPNSNF; Chen et al., Citation2015), which consists of 8 items measuring the general need to manage one’s own behaviour, choices and goals (e.g., “I feel a sense of choice and freedom in the things I undertake”). The scale demonstrated good internal consistency (Cronbach’s α and McDonald’s ωh = .81, Guttman’s λ6 = .84), very close to the values reported in Chen et al. (Citation2015) validation studies across four cultures (with Cronbach’s α ranging from .77 to .85 depending on country).
Readiness for online learning
To measure readiness for online learning, a brand new scale was constructed due to the fact that the existing instruments did not reflect technological development. Based on Hung and colleagues’ (2010) approach and measurement as well as a review of research and other scales assessing readiness for online learning (e.g., Cheon et al., Citation2021; Dray et al., Citation2011; Martin, Stamper & Flowers, 2021; Yu & Richardson, Citation2015), we identified four main dimensions: self-directed learning, online learning motivation, self-efficacy in using digital technologies, and self-efficacy in computer-mediated communication. The hypothesised structure was confirmed with an exploratory factor analysis performed with the maximum likelihood factor analysis and varimax rotation (Appendix 2). The present analysis focuses on two of four dimensions distinguished in the EFA, i.e. self-directed learning and online learning motivation. The first reflects taking the initiative by the learners in order to understand their own learning needs, establish learning goals, identify their learning resources, use appropriate learning strategies, and evaluate learning outcomes (e.g., “I set the specific goals in my learning”; “I can control my own learning progress”). This subscale is composed of 8 items and has acceptable reliability (Cronbach’s α = .74, McDonald’s ωh = .75, Guttman’s λ6 = .70). The online learning motivation scale, reflecting learners’ positive orientation towards the online learning process, consists of 10 items and has good internal consistency (Cronbach’s α, McDonald’s ωh and Guttman’s λ6 = .85).
Procedure
The survey was administered from October 2021 to July 2022 on the Alchemer platform, a commercial survey software tool that allows participation from regions where other solutions such as Google Forms are not easily accessible. The invitation to participate in the study was sent to university language instructors, lecturers in linguistics departments, as well as private language schools via mailing lists, private contacts, official e-mails to the heads of language centres, and via social media. Participants were also recruited using snowball sampling, the non-probabilistic sampling method where the respondents themselves share information about the study and invite others to participate. It is considered a method that allows research to be conducted among populations with specific desired characteristics, such as different L2 learners who would otherwise be difficult to contact (Ness Evans & Rooney, Citation2018). The study was preregistered (https://osf.io/zxnk8/), fully anonymous; the protocol had received IRB approval (University of Warsaw Committee for Research Involving Human Participants № 101/2021). Participation was fully voluntary and each potential respondent was informed in the invitation and immediately before starting to complete the survey about the purpose of the research. The survey was intended exclusively for adults. Completing the questionnaire took approximately between 15 and 19 minutes. The completion rate was 65.8%.
Data analysis – method
RQ 1: Multiple linear regression model
To address the first research question concerning the predictors of L2 grit in remote and hybrid learning settings, we built a multiple linear regression model using SPSS v. 28. Multiple linear regression requires meeting five assumptions: linearity of regression, normality of residuals, homogeneity of variance, homogeneity of regression slopes, and independence of error terms. The linearity assumption was tested on the basis of a visual analysis of scatterplots, which confirmed a normal distribution of the variables and the residuals. This method is customarily recommended for samples over 300 participants (Baguley, Citation2012), avoiding the tendency towards excessive conservatism and the poor power of normality tests such as the Kolmogorov-Smirnov (see e.g. Yap & Sim, Citation2011) or the Shapiro-Wilk, which are more appropriate with small samples (Field, Citation2018; Yap & Sim, Citation2011). In addition, normality in large sample distributions is usually guaranteed by the central limit theorem. Therefore, normality tests are not advised for large samples, because they can easily generate artefacts, recognising even small deviations from the null hypothesis (of meeting the assumption) as significant and thereby imposing its rejection (Field, Citation2018; Kozak & Piepho, Citation2018). The absence of multicollinearity was confirmed on the basis of two indicators: variance inflation factor (VIF), ranging from 1.05 to 2.16 (much below the threshold value of 5; Field, Citation2018) and tolerance, ranging from .46 to .95 (exceeding the threshold value of .10). The assumption of homoscedasticity was examined visually using a scatterplot of residuals versus predicted values. This analysis confirmed equal variances of the residuals. Taking into account the sample size and the assumption of the central limit theorem (Field, Citation2018), the graphical method of analysis was more relevant than Levene’s test. Lastly, the assumption of homoscedasticity was confirmed with the Durbin-Watson statistic testifying to the lack of correlation between the residuals (d = 1.88).
RQ 2: Pearson’s correlation coefficients and psychological network analysis
To answer the second research question about the relationships between L2 grit and its putative predictors, we first calculated Pearson’s correlation coefficients. To specify which of the factors are most important in the complex network of associations with L2 grit, we performed a psychological network analysis using JASP v. 0.16.4 (JASP Team, Citation2022). This analysis permits the simultaneous estimation of the relationships between all the variables included in the network without losing the complexity of the model. The associations among the variables are graphically represented in a network structure, which visualises them as nodes interconnected by edges. The connections represent coefficients derived from the model of the relationship between variables, such as correlation coefficients, partial correlation coefficients, or regression weights. The strength of the relationship between the variables is denoted by the thickness of the edges. Edge colour specifies the relationship direction (positive or negative). As a rule, software uses blue or green as the default colour for positive relationships and red for negative ones.
Another important indicator is the distance between the nodes and the amount of space they share. In the Fruchterman-Reingold (FR) algorithm, the closer the distance between the nodes, the stronger the relationship between them (Fruchterman & Reingold, Citation1991).
Following the suggestions of Epskamp et al. (Citation2018), we analysed the network of relationships between L2 grit and the remaining measured variables in three stages. In the first step, we estimated the statistical model, examining partial correlation coefficients using Gaussian Graphical Model (GGM; Epskamp et al., Citation2018). The GGM provides an estimation of polychoric correlations and is therefore used to analyse data collected with Likert-type scales (Fried et al., Citation2017). To eliminate the problem of failing to take into account the possible influence of the other variables—which takes place when network nodes and edges are estimated using zero-order correlations (such as Pearson’s correlation coefficients), resulting in inflated correlation coefficients and subsequent difficulties with interpretation of the results—we used a regularised partial correlation approach, calculating the conditional (in)dependence associations after all the other nodes have been taken into account, protecting against spurious associations (i.e., false positives). Given the frequently unclear form of complex networks, to minimise spurious edges we used GGM with the graphical least absolute shrinkage and selection operator (LASSO) regularisation technique. This algorithm reduces low partial correlations to 0, typically resulting in a sparse (more conservative) network that only shows the most important relationships between the variables (Epskamp et al., Citation2017; Hevey, Citation2018). The LASSO approach lowers the risk of false positives, increasing the confidence level of the edges occurring in the network (Krämer et al., Citation2009). Additionally, it uses a tuning parameter enabling to control sparsity and minimising the Extended Bayesian Information Criterion (EBIC), permitting the estimation of a ‘weighted’ matrix providing the optimal degree of network constraints (Epskamp et al., Citation2017; Epskamp et al., Citation2018). In this study, we applied the EBICglasso estimation method combining the LASSO algorithm with the EBIC tuning parameter, in line with the recommendation to create networks that balance specificity and interpretability with sensitivity (Epskamp & Fried, Citation2018). As a result, we received a network model which is sparser and more straightforward to interpret.
In the next step, we performed an analysis of the network structure. A network analysis provides not only an estimated strength of the relationship between specific variables, but also allows determining the importance each variable or their combination has in a given network structure (Hevey, Citation2018). The relative importance of a particular node can be calculated using centrality indices (Opsahl et al., Citation2010; Paradowski et al., Citation2021).
Finally, we assessed the accuracy and stability of the network parameters. Both these measures indicate the probability that the network with its effects will be replicated. Edge-weight accuracy can be evaluated on the basis of an estimation of 95% confidence intervals (CIs) applying non-parametric bootstrapped samples. Narrower CIs indicate more accurate edge estimation. Centrality stability may be estimated based on case-dropping subset bootstrapping. It consists in rejecting incremental percentages of observations to check whether centrality measures remain constant in each subset of observations. Correlation stability coefficient (CS) values enable assessing if the coefficients vary significantly between each incremental case-dropping subset; a CS over 0.5 represents adequate stability (Epskamp et al., Citation2018, Forbes et al., Citation2021).
Results
To answer the first research question and identify the predictors of language-domain-specific grit, we built a multiple linear regression model (). This showed that L2 grit is primarily determined by online learning motivation (β = .41, t(608) = 9.70, p < .001) and self-directed learning (β = .32, t(608) = 8.86, p < .001), thus the two aspects of readiness for online learning. The medium effect sizes of both dimensions (ηp2= .13 and .11, respectively) indicate a strong contribution of these variables to explaining L2 grit. In addition to these two factors closely connected to foreign language learning, L2 grit is also determined by learners’ two general personality dispositions, autonomy (β = .17, t(608) = 4.95, p < .001) and curiosity (β = − .09, t(608) = −2.52, p = .012). However, they are characterised by small effect sizes (ηp2= .04 and .01, respectively) and the confidence interval of the latter indicates that the effect is unstable. Interestingly, neither of the language mindsets has a significant impact on the explained variable. A test model controlling for participants’ age and gender found that these variables failed to contribute to the outcome, with null effect sizes and both confidence intervals crossing zero; these variables were consequently removed in the final analyses.
Table 2. Summary of multiple linear regression analysis for variables predicting L2 grit.
The regression model is significant (F6,608 = 97.28, p < .001) and predicts 48% of variance in language-domain-specific grit (), with a very large effect size (ηp2 = .49).
Table 3. The significance of the multiple linear regression model for variables predicting L2 grit.
To provide an answer to the first part of the second research question concerning the relationships between the variables, we first calculated Pearson’s correlation coefficients (). L2 grit correlated with readiness for online learning in the two measured dimensions: online learning motivation (r = .62, p < .001) and self-directed learning (r = .58, p < .001). It also positively correlated, though to a lesser extent, with the two general psychological dispositions not directly related to learning, i.e. sense of autonomy (r = .47, p < .001) and curiosity (r = .34, p < .001). Moreover, interest in language learning and persistency of effort correlated positively with growth language mindset (r = .31, p < .001), and negatively with fixed language mindset (r = −.21, p < .001). These relationships, although statistically significant, were nevertheless quite weak.
Table 4. Pearson’s correlation coefficients [CIs] between L2 grit and language mindsets, curiosity, autonomy, and readiness for online learning measured by its two dimensions: self-directed learning and online learning motivation.
To find the answer to the second part of the second research question and specify which of the factors are particularly important in the complex network of associations, we estimated partial correlations applying graphical LASSO regularisation combined with EBIC model selection and the tuning parameter set to 0.5 (Foygel & Drton, Citation2010; Epskamp et al., Citation2018). To check the accuracy and stability of the network, we followed nonparametric case-drop bootstrapping based on a 5,000 bootstrap sample.
After the regularisation, the initial network was reduced from 21 to 15 non-zero edges, with 12 representing positive relationships. The network () indicates equally strong direct edges connecting L2 grit and both dimensions of readiness for online learning, i.e. online learning motivation and self-directed learning (partial correlation of .32 for each). A much weaker edge links L2 grit with learners’ sense of autonomy (.17). The estimated network revealed no further direct connections with L2 grit. The graph shows an indirect path associating curiosity with L2 grit, mostly via online learning motivation and—to a lesser extent—autonomy, and only marginally via self-directed learning. A similarly complex pattern of relationships exists between L2 grit and growth language mindset. This association is primarily influenced by online learning motivation, and to a much lesser extent by the second component of readiness for online learning—self-directed learning. The graph includes the network’s weights indicating the strength of each connection. The matrix of the weights (p < .05) is shown in .
Figure 2. Estimated network structure of the relationships among L2 grit, language mindsets, curiosity, autonomy, and readiness for online learning.
Note: selfdir – self-directed learning, L2_gmindset – growth language mindset, L2_fmindset – fixed language mindset.
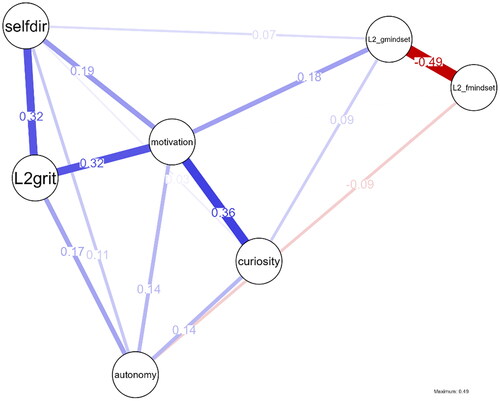
Table 5. Network’s weight matrix.
The accuracy of the edge weights, estimated after bootstrapping, is displayed in . The 95% CIs of most edge weights are below .12 and do not contain 0, thus are significant, with large values for quite a few edges. Relatively narrow CIs occur in the case of the edges connecting L2 grit with both components of readiness for online learning as well as linking other variables such as curiosity with self-directed learning, online learning motivation and growth language mindset. Conversely, considerably larger confidence intervals are found for edges combining L2 grit with a sense of autonomy, as well as the latter with curiosity and both components of readiness for online learning. Given the small deviations between the estimated edge weights (sample value) and the mean bootstrapped estimated edge-weights as well as the bootstrapped 95% CIs for the estimates, the network is of acceptable accuracy.
Figure 3. Accuracy of the estimated network. Lines on the y-axis show edges ordered from the highest mean bootstrapped edge-weight to the lowest mean bootstrapped edge-weight. The red line represents the estimated edge weights (sample value), and the grey area indicates the bootstrapped 95% CIs for the estimates. The black line shows the mean bootstrapped estimated edge-weights.
Note: selfdir – self-directed learning, L2_gmindset – growth language mindset, L2_fmindset – fixed language mindset.
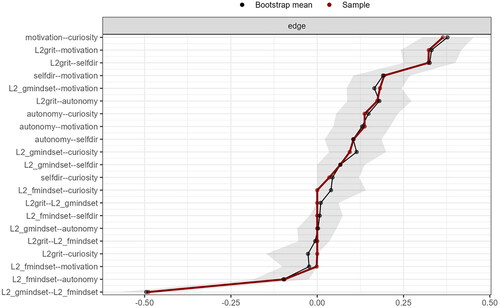
The analysis of the network structure shows that motivation for remote learning and L2 grit have the highest number of associations with the other variables included in the network and constitute the most central factors. Interestingly, online learning motivation turns out to be the strongest node in this dependency network, much stronger than L2 grit. Growth language mindset also displays multiple connections. The standardised estimates of centrality indices are presented in and .
Figure 4. Centrality indices. The horizontal axis displays standardised centrality (z-scores). The vertical axis presents the particular variables. Those with higher centrality are more distant (further to the right) from the vertical axis.
Four panels illustrating the constructs’ centrality indices, showing highest values for online learning motivation, L2 grit, curiosity, and growth mindset.
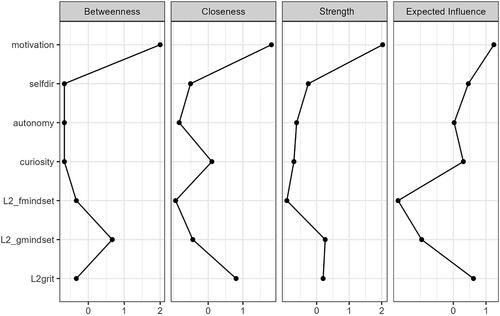
Table 6. Centrality measures per variable.
To examine the stability of the centrality indices, case-dropping bootstrapping was performed, with the results shown in . The graph demonstrates the correlation stability coefficient (CS) from subsets of data differing in the percentage of the total sample for all centrality indices. A slight decrease in the correlation between the subsample estimates and those from the original total sample is observed, as the subset sample is reduced from 95% of the original sample to 25% of the sample. However, the correlations for all the centrality indices remain above .75, with the highest value for strength centrality, and indicate good stability of the centrality indices (Epskamp et al., Citation2018). Similarly, good network stability is confirmed by the average correlations of edge weights in our network, significantly stronger than .75 ().
Figure 5. Stability of centrality indices. The average correlations between the network’s centrality indices received from case-dropping bootstrap and the network based on the original sample. The lines represent the means, while the shaded areas reflect the 95% confidence interval.
Note: selfdir – self-directed learning, L2_gmindset – growth language mindset, L2_fmindset – fixed language mindset.
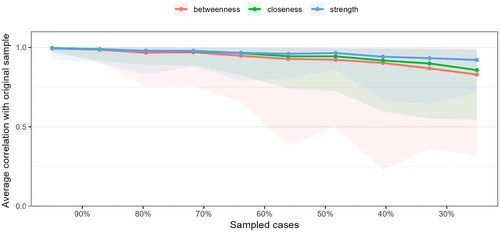
Figure 6. Stability of edge weights. The average correlations in edge weight between the original sample and the network received from case-dropping bootstrap (sampled cases).
Note: selfdir – self-directed learning, L2_gmindset – growth language mindset, L2_fmindset – fixed language mindset.
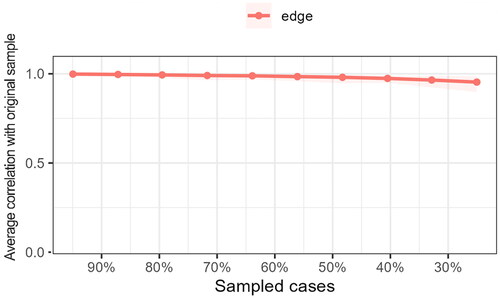
Discussion and conclusions
While studies in many fields of education confirm that grit plays an important role in educational goals and learning progress, the importance of this disposition in relation to second language learning has not yet been well explored. Even though emerging studies show a positive relationship between language-domain-specific grit and language learning achievement, little is known about factors responsible for the variance in the intensity of L2 grit among learners and about the predictors of this trait. Such dependencies and relationships are particularly consequential in online learning environments, which—while providing learners with many opportunities and freedom—entail new challenges and demands such as being easily distracted during lessons by other activities, for instance web browsing, social media, or instant messaging, and consequently loss of interest and poor engagement in lessons, technical difficulties and anxiety related to lack of sufficient skills in using digital technologies, limited interaction with other classmates and the teacher, and resultant frustration (Bani-Hani, Al-Sobh & Abu-Melhim, Citation2014; Gonzáles-Lloret, 2020; Jacobs, Citation2014; Keramati et al., Citation2011; Wang & Hsu, Citation2008).
This large-scale study permitted identifying the predictors of L2 grit. The multiple regression model revealed that both dimensions of readiness for online learning, i.e. learning motivation and self-directed learning, are the chief determinants of L2 grit in remote and hybrid contexts, with medium effect sizes approaching large values. This result is in line with the assumptions of complex dynamic systems theory, indicating the crucial role of the learning context—or, more specifically, learners’ dispositions strongly associated with a specific learning context—in developing learners’ L2 grit. Our findings are consistent with those reported by Torun (Citation2020), who showed that self-directed learning and motivation are the most effective predictors of academic performance in higher education from among various dimensions of readiness for online learning. However, also noteworthy is the role of two general psychological dispositions, autonomy and curiosity. The latter makes for a particularly interesting insight, as the effect of curiosity as a general personality trait or character strength has not yet been studied in connection with L2 learning. With regard to autonomy, our result echoes the one presented by Elahi Shirvan and Alamer (Citation2022). According to their SEM, autonomy is the only basic psychological need that can explain both components of L2 grit, i.e. persistence of effort and consistency of interest (although the latter in a small proportion). Autonomy allows the individual to make voluntary and deliberate decisions regarding their level of commitment in language learning and, consequently, the effort undertaken, consistent interest, and the pace of learning. However, contrary to the rationale behind theory and previous research, growth language mindset completely failed to affect L2 grit. In this point our results disconfirm the hypothesis by Elahi Shirvan, Taherian and Yazdanmehr (Citation2021) that changes in the intensity of L2 grit over time may be a function of learners’ mindset. However, their hypothesis applied to face-to-face learners.
The answer to the first part of the second research question showed that although L2 grit positively correlated with growth language mindset and negatively with fixed language mindset, its associations with these variables were weak. Our result is broadly consistent with that of Teimouri et al. (Citation2022), who found that the correlations of L2 grit with growth language mindset were the only ones statistically significant, while being half those reported in our study. Of note, however, is that Teimuri and colleagues (2022) again studied face-to-face language learners. Khajavy et al. (Citation2021), in turn, revealed that growth language mindset is positively associated not with entire L2 grit, but only with its component of persistence of effort. It is thus apparent that the context of learning has a significant contribution to the differential strengths of the relationship between L2 grit and L2 mindsets. This explanation may be supported by the strong correlation between L2 grit and each of the two measured dimensions of readiness for online learning. The results may further suggest that L2 grit should be studied not only as a trait, as originally conjectured, but also as a state. It may thus show greater sensitivity to the effects of context-specific variables.
High L2 grit is at the same time accompanied by a feeling of being highly autonomous and, to a slightly lesser degree, by intense curiosity. Both of these results constitute a significant contribution to our understanding of L2 grit, as until now these relationships have received little attention in research (Elahi Shirvan & Alamer, Citation2022). With regard to autonomy, a similar relationship was previously reported by Jin and Kim (Citation2017), finding a positive association of autonomy with subjective well-being. The theoretical rationale, as well as research in other domains, shows that the influence of these psychological dispositions in shaping grit cannot be ignored.
Given the fact that the conventional zero-order correlation analysis does not eliminate the possible influence of other variables, often leading to spurious associations (such as many false positives), we carried out a relationship network estimation using psychological network analysis. This type of network analysis, capable of illustrating dynamic relationships between individual characteristics and foreign language learning, has not yet received much attention from researchers in the SLA field (Freeborn et al., Citation2022). With the use of a regularised partial correlation approach applying a Gaussian graphical model with the LASSO regularisation technique to minimise spurious edges, the analysis provides a clearer and more precise insight into the relationship between L2 grit and the variables examined. The sparser network model obtained, more straightforward to interpret, allowed us to identify factors of particular importance in the complex network of L2 grit associations, confirming a strong interdependence between L2 grit and the two dimensions of readiness for online learning and a weaker relationship with a sense of autonomy. Interestingly, these were the only direct connections. The analysis suggests that curiosity may enhance L2 grit indirectly, interacting with learning motivation and a sense of autonomy. A similar, but even more complex pattern of relationships can be observed between L2 grit and growth mindset. These patterns of relationships corroborate the need to include a complex systems theoretical perspective into research investigating individual differences.
Our study is one of the first to use a variable-centred relation-intensive approach within the paradigm of dynamic complex systems in SLA (Freeborn et al., Citation2022). Responding to the call to better understand “the relations that connect the components of a complex system” (Hiver & Larsen-Freeman, Citation2019: 287), we visualise the complex, multidimensional structure of the relationships between positive psychological dispositions and the L2 grit they explain. Psychological networks can provide valuable insights into the complexity of the language learning process. Considering our results from the perspective of dynamic complex systems (Paradowski & Jonak, Citation2012: 132f.), we reveal how some individual properties that are part of a complex system in the context of remote language learning are more strongly linked to one another, such as motivation and, to a somewhat lesser extent, L2 grit, constituting—as it were—the ‘glue’ of the system.
Our findings carry practical implications for teachers. Firstly, they make it clear that the learning context, e.g. a remote setting with its various challenges and affordances, may require activating somewhat different learner dispositions than the traditional, on-site approach. Secondly, the relationships observed are not always straightforward and direct, so it is important to be aware that the teacher can nurture and reinforce L2 grit in their students by strengthening their motivation and encouraging them to independently seek new sources of linguistic stimulation and make their own choices about opportunities for practising and developing their linguistic competence, thus helping contribute to their progress and satisfaction with learning the foreign language.
Limitations
This study is not without some limitations. One concern may relate to participants’ gender proportions. The vast majority are young women, which makes it necessary to take a cautious approach to generalising the results to a broader population. However, such a phenomenon of female overrepresentation is very common both in language courses and in remotely conducted research. It has been shown that women are more likely than men to participate in online and paper surveys (Green, Citation1996; Becker & Glauser, Citation2018) because they are more communicative and willing to share their opinions and feelings with others, and take a more altruistic approach to completing a survey (Becker, Citation2022b; Green, Citation1996). They also show a higher propensity to respond immediately after receiving an invitation to an online questionnaire (Becker, Citation2022a; Becker et al., Citation2019). Moreover, in the field of L2 learning, women are characterised by a more positive attitude towards learning a foreign language than male language learners, and show more interest in the target language culture, country and speakers (Bacon & Finnemann, Citation1992; Gardner & Lambert, Citation1972; Jiang et al., Citation2009). All these factors may explain the significantly higher proportion of women than men in our survey.
The vast majority of the respondents came from European countries, to a lesser extent North America and Asia. Latin American and African countries, on the other hand, were poorly represented, which may reflect the differences in technological advances and digitisation in the field of language education between the developed North and the developing South as well as difficulty reaching participants in the latter. The results of the OECD Survey of Adult Skills (PIAAC) indicate that ICT literacy remains low in Latin American countries (OECD, Citation2020; UNESCO, Citation2022), with a similar situation in Africa. The Afrobarometer survey from 2016 through 2018 showed that out of 34 countries, only in seven did more than a third of the households surveyed have both a smartphone and a computer. At the same time, in nine countries fewer than one in ten respondents could connect to the internet via a mobile phone or computer, while in 20 countries more than half of the households did not have access to the internet at all (Krönke, Citation2020). The high cost of digital technologies is often a major constraint limiting access to remote learning, including language learning. As a result, many universities are reluctant to adopt e-learning and do not have well-developed methods of using technology to enhance instruction (Dutz, Almeira & Packard, Citation2018). All these factors may make online language learning still less prevalent, popular and accessible.
The current analysis only looks at learners participating in remote and/or hybrid language classes, without comparing their results with those of peers learning face-to-face. These parallel data have also been collected (Jelińska & Paradowski, Citation2023), and a comparison of both foreign language learning contexts in relation to L2 grit is the aim of forthcoming publications.
Despite the aforementioned limitations, the results make an important contribution to the existing state of the art on factors influencing L2 grit among language learners in online environments, and shed light on the hitherto unexplored non-trivial complex relationships between this construct and other individual psychological dispositions.
Acknowledgments
We thank Katya Sudina and Luke Plonsky for helpful discussion and commentary, and the anonymous reviewers for useful feedback. The authors receive funding from the project “The impact of grit and its predictors on face-to-face vs online language learning” awarded by the University of Warsaw in the ‘New Ideas’ competition implemented under the ‘Excellence Initiative – Research University’ (IDUB) programme Priority Research Area IV “The Humanities: Crossing Borders, Extending Capabilities”, as well as from the National Science Centre of Poland SONATA-BIS grant №2016/22/E/HS2/00034. MBP also acknowledges support from COST Actions 15109 (COSTNET), 18232 (MAT-DYN-NET), 19102 (LITHME), 20115 (ENIS), and 21114 (CLILNetLE).
Disclosure statement
No potential conflict of interest was reported by the authors.
Additional information
Funding
References
- Alamer, A. (2021). Grit and language learning: Construct validation of L2-Grit scale and its relation to later vocabulary knowledge. Educational Psychology, 41(5), 544–562. https://doi.org/10.1080/01443410.2020.1867076
- Aparicio, M., Bação, F., & Oliveira, T. (2017). Grit in the path to e-learning success. Computers in Human Behavior, 66, 388–399. https://doi.org/10.1016/j.chb.2016.10.009
- Bacon, S. M. C., & Finnemann, M. D. (1992). Sex differences in self-reported beliefs about foreign-language learning and authentic oral and written input. Language Learning, 42(4), 471–495. https://doi.org/10.1111/j.1467-1770.1992.tb01041.x
- Baguley, T. (2012). Serious Stats: A Guide to Advanced Statistics for the Behavioral Sciences. Palgrave Macmillan.
- Bani-Hani, N., Al-Sobh, M., & Abu-Melhim, A. (2014). Utilizing Facebook groups in teaching writing: Jordanian EFL students’ perceptions and attitudes. International Journal of English Linguistics, 4(5), 27–34. https://doi.org/10.5539/ijel.v4n5p27
- Becker, R. (2022a). The effects of a special sequential mixed-mode design, and reminders, on panellists’ participation in a probability-based panel study. Quality and Quantity, 56, 259–284. https://doi.org/10.1007/s11135-021-01126-6
- Becker, R. (2022b). Gender and survey participation: An event history analysis of the gender effects of survey participation in a probability-based multi-wave panel study with a sequential mixed-mode design. Methods, Data, Analyses, 16(1), 3–32. https://doi.org/10.12758/mda.2021.08
- Becker, R., & Glauser, D. (2018). Are prepaid monetary incentives sufficient for reducing panel attrition and optimizing the response rate? An experiment in the context of a multi-wave panel with a sequential mixed-mode design. Bulletin of Sociological Methodology, 139(1), 74–95. https://doi.org/10.1177/0759106318762456
- Becker, R., Möser, S., & Glauser, D. (2019). Cash vs. vouchers vs. gifts in web surveys of a mature panel study—Main effects in a long-term incentives experiment across three panel waves. Social Science Research, 81, 221–234. https://doi.org/10.1016/j.ssresearch.2019.02.008
- Bringmann, L. F., Elmer, T., Epskamp, S., Krause, R. W., Schoch, D., Wichers, M., Wigman, J., & Snippe, E. (2019). What do centrality measures measure in psychological networks? Journal of Abnormal Psychology, 128(8), 892–903. https://doi.org/10.1037/abn0000446
- Changlek, A., & Palanukulwong, T. (2015). Motivation and grit: Predictors of language learning achievement. International Humanities. Social Sciences and Arts, 8(4), 23–36. https://he02.tci-thaijo.org/index.php/Veridian-E-Journal/article/view/40089
- Chen, B., Vansteenkiste, M., Beyers, W., Boone, L., Deci, E. L., Van der Kaap-Deeder, J., Duriez, B., Lens, W., Matos, L., Mouratidis, A., Ryan, R. M., Sheldon, K. M., Soenens, B., Van Petegem, S., & Verstuyf, J. (2015). Basic psychological need satisfaction, need frustration, and need strength across four cultures. Motivation and Emotion, 39, 216–236. https://doi.org/10.1007/s11031-014-9450-1
- Cheon, J., Cheng, J., & Cho, M.-H. (2021). Validation of the Online Learning Readiness Self-Check survey. Distance Education, 42(4), 599–619. https://doi.org/10.1080/01587919.2021.1986370
- Clark, K. N., & Malecki, C. K. (2019). Academic Grit Scale: Psychometric properties and associations with achievement and life satisfaction. Journal of School Psychology, 72, 49–66. https://doi.org/10.1016/j.jsp.2018.12.001
- Cormier, D. L., Dunn, J. G., & Dunn, J. C. (2019). Examining the domain specificity of grit. Personality and Individual Differences, 139, 349–354. https://doi.org/10.1016/j.paid.2018.11.026
- Council of Europe. (2020). Common European Framework of Reference for Languages: Learning, Teaching, Assessment – Companion Volume. Council of Europe. https://rm.coe.int/common-european-framework-of-reference-for-languages-learning-teaching/16809ea0d4
- Deci, E. L., & Ryan, R. M. (2000). The “what” and “why” of goal pursuits: Human needs and the self-determination of behavior. Psychological Inquiry, 11(4), 227–268. https://doi.org/10.1207/S15327965PLI1104_01
- Deci, E. L., & Ryan, R. M. (2008). Facilitating optimal motivation and psychological well-being across life’s domains. Canadian Psychology, 49(1), 14–23. https://doi.org/10.1037/0708-5591.49.1.14
- Dewaele, J.-M., Chen, X., Padilla, A. M., & Lake, J. (2019). The flowering of positive psychology in foreign language teaching and acquisition research. Frontiers in Psychology, 10, 2128. https://doi.org/10.3389/fpsyg.2019.02128
- Dray, B. J., Lowenthal, P. R., Miszkiewicz, M. J., Ruiz-Primo, M. A., & Marczynski, K. (2011). Developing an instrument to assess student readiness for online learning: A validation study. Distance Education, 32(1), 29–47. https://doi.org/10.1080/01587919.2011.565496
- Du Toit-Brits, C. (2020). Unleashing the power of self-directed learning: Criteria for structuring self-directed learning within the learning environments of higher education institutions. Africa Education Review, 17(2), 20–32. https://doi.org/10.1080/18146627.2018.1494507
- Duckworth, A. L., & Quinn, P. D. (2009). Development and validation of the Short Grit Scale (Grit–S). Journal of Personality Assessment, 91(2), 166–174. https://doi.org/10.1080/00223890802634290
- Duckworth, A. L., Peterson, C., Matthews, M. D., & Kelly, D. R. (2007). Grit: Perseverance and passion for long-term goals. Journal of Personality and Social Psychology, 92(6), 1087–1101. https://doi.org/10.1037/0022-3514.92.6.1087
- Duckworth, A. L., Quirk, A., Gallop, R., Hoyle, R. H., Kelly, D. R., & Matthews, M. D. (2019). Cognitive and noncognitive predictors of success. Proceedings of the National Academy of Sciences of the United States of America, 116(47), 23499–23504. https://doi.org/10.1073/pnas.1910510116
- Dutz, M. A., Almeida, R. K., & Packard, T. G. (2018). The Jobs of Tomorrow: Technology, Productivity, and Prosperity in Latin America and the Caribbean. World Bank.
- Dweck, C. S. (1999). Self-Theories: Their Role in Motivation, Personality, and Development. Psychology Press.
- Dweck, C. S. (2006). Mindset: The New Psychology of Success. Random House.
- Dweck, C. S., & Leggett, E. L. (1988). A social-cognitive approach to motivation and personality. Psychological Review, 95(2), 256–273. https://doi.org/10.1037/0033-295X.95.2.256
- Dweck, C. S., Chiu, C. Y., & Hong, Y. Y. (1995). Implicit theories and their role in judgments and reactions: A word from two perspectives. Psychological Inquiry, 6(4), 267–285. https://doi.org/10.1207/s15327965pli0604_1
- Elahi Shirvan, M., & Taherian, T. (2021). Longitudinal examination of university students’ foreign language enjoyment and foreign language classroom anxiety in the course of general English: Latent growth curve modeling. International Journal of Bilingual Education and Bilingualism, 24(1), 31–49. https://doi.org/10.1080/13670050.2018.1441804
- Elahi Shirvan, M., & Alamer, A. (2022). Modeling the interplay of EFL learners’ basic psychological needs, grit and L2 achievement. Journal of Multilingual and Multicultural Development, https://doi.org/10.1080/01434632.2022.2075002
- Elahi Shirvan, M., Lou, N. M., Shahnama, M., & Yazdanmehr, E. (2021). Maintaining effort and interest despite challenges during the COVID-19 pandemic: A process tracing approach to a teacher’s grit during an online L2 course. Journal for the Psychology of Language Learning, 3(2), 45–60. https://doi.org/10.52598/jpll/3/2/4
- Elahi Shirvan, M., Taherian, T., & Yazdanmehr, E. (2021). L2 grit: A longitudinal confirmatory factor analysis-curve of factors model. Studies in Second Language Acquisition, 44(5), 1449–1476. https://doi.org/10.1017/S0272263121000590
- Elahi Shirvan, M., Taherian, T., Shahnama, M., & Yazdanmehr, E. (2021). A longitudinal study of Foreign Language Enjoyment and L2 Grit: A Latent Growth Curve Modeling. Frontiers in Psychology, 12, 720326. https://doi.org/10.3389/fpsyg.2021.720326
- Epskamp, S., & Fried, E. I. (2018). A tutorial on regularized partial correlation networks. Psychological Methods, 23(4), 617–634. https://doi.org/10.1037/met0000167
- Epskamp, S., Borsboom, D., & Fried, E. I. (2018). Estimating psychological networks and their accuracy: A tutorial paper. Behavior Research Methods, 50(1), 195–212. https://doi.org/10.3758/s13428-017-0862-1
- Epskamp, S., Kruis, J., & Marsman, M. (2017). Estimating psychopathological networks: Be careful what you wish for. PLoS One. 12(6), e0179891. https://doi.org/10.1371/journal.pone.0179891
- Epskamp, S., Waldorp, L. J., Mõttus, R., & Borsboom, D. (2018). The Gaussian graphical model in cross-sectional and time-series data. Multivariate Behavioral Research, 53(4), 453–480. https://doi.org/10.1080/00273171.2018.1454823
- Feng, L., & Papi, M. (2020). Persistence in language learning: The role of grit and future self-guides. Learning and Individual Differences, 81, 101904. https://doi.org/10.1016/j.lindif.2020.101904
- Field, A. (2018). Discovering Statistics Using IBM SPSS Statistics. [5th edition]. SAGE.
- Forbes, M. K., Wright, A. G. C., Markon, K. E., R. F., & Krueger, R. F. (2021). Quantifying the reliability and replicability of psychopathology networks characteristics. Multivariate Behavioral Research, 56(2), 224–242. https://doi.org/10.1080/00273171.2019.1616526
- Foygel, R., & Drton, M. (2010). Extended Bayesian information criteria for Gaussian graphical models [Paper presentation]. Advances in Neural Information Processing Systems 23: 24th Annual Conference on Neural Information Processing Systems, 2010, NIPS.
- Freeborn, L., Andringa, S., Lunansky, G., & Rispens, J. (2022). Network analysis for modeling complex systems in SLA research. Studies in Second Language Acquisition, 44(4), 1–32. https://doi.org/10.1017/S0272263122000407
- Fried, E. I., van Borkulo, C. D., Cramer, A. O. J., Boschloo, L., Schoevers, R. A., & Borsboom, D. (2017). Mental disorders as networks of problems: A review of recent insights. Social Psychiatry and Psychiatric Epidemiology, 52, 1–10. https://doi.org/10.1007/s00127-016-1319-z
- Fruchterman, T. M. J., & Reingold, E. M. (1991). Graph drawing by force-directed placement. Software: Practice and Experience, 21(11), 1129–1164. https://doi.org/10.1002/spe.4380211102
- Fukuda, S. T., Sakata, H., & Takeuchi, M. (2011). Facilitating autonomy to enhance motivation: Examining the effects of a guided-autonomy syllabus. Electronic Journal of Foreign Language Teaching, 8(1), 71–86.
- Gardner, R. C., & Lambert, W. E. (1972). Attitudes and Motivation in Second-Language Learning. Newbury House.
- Gibbons, M. (2002). The Self-Directed Learning Handbook: Challenging Adolescent Students to Excel. Jossey-Bass.
- Godwin-Jones, R. (2019). Riding the digital wilds: Learner autonomy and informal language learning. Language Learning & Technology, 23(1), 8–25. https://doi.org/10.10125/44667
- González-Lloret, M. (2020). Collaborative tasks for online language teaching. Foreign Language Annals, 53(2), 260–269. https://doi.org/10.1111/flan.12466
- Green, K. E. (1996). Sociodemographic factors and mail survey response. Psychology & Marketing, 13(2), 171–184. https://doi.org/10.1002/(SICI)1520-6793(199602)13:2 < 171::AID-MAR4 > 3.0.CO;2-C
- Guri-Rosenblit, S. (2018). E-teaching in higher education: An essential prerequisite for E-learning. Journal of New Approaches in Educational Research, 7(2), 93–97. https://doi.org/10.7821/naer.2018.7.298
- Gurning, B., & Siregar, A. (2017). The effect of teaching strategies and curiosity on students’ achievement in reading comprehension. English Language Teaching, 10(11), 191–198. https://doi.org/10.5539/elt.v10n11p191
- Hevey, D. (2018). Network analysis: A brief overview and tutorial. Health Psychology and Behavioral Medicine, 6(1), 301–328. https://doi.org/10.1080/21642850.2018.1521283
- Hiver, P., & Larsen-Freeman, D. (2019). Motivation: It is a relational system. In A. Al-Hoorie & P. MacIntyre (Eds.), Contemporary Language Motivation Theory (pp. 285–303). Multilingual Matters.
- Hung, M.-L. (2016). Online learning readiness: Its relations to college students’ changes over time, and willingness to enroll in future courses. International Journal of Technology and Human Interaction, 12(1), 51–62. https://doi.org/10.4018/IJTHI.2016010104
- Hung, M.-L., Chou, C., Chen, C.-H., & Own, Z.-Y. (2010). Learner readiness for online learning: Scale development and student perceptions. Computers & Education, 55(3), 1080–1090. https://doi.org/10.1016/j.compedu.2010.05.004
- Izard, C. E. (1977). Human emotions. Plenum.
- Jacobs, P. (2014). Engaging students in online courses. Research in Higher Education Journal, 26, 1–9. https://www.aabri.com/manuscripts/142019.pdf
- Jarynowski, A., Czopek, K., & Paradowski, M. B. (2021). Keep your friends close: Jak interakcje między uczniami pomagają w przyswajaniu języka obcego: Implikacje dla doby pandemii. Acta Neophilologica, XXIII(2), 115–131. https://doi.org/10.31648/an.6660
- JASP Team (2022). JASP (Version 0.16.4) [Computer software].
- Jelińska, M. & Paradowski, M. B. (2023). Predictors of L2 grit and language learners’ flow experience in online vs onsite settings [conference presentation]. 2023 AERA Annual Meeting, Chicago.
- Jiang, M., Green, R. J., Henley, T. B., & Masten, W. G. (2009). Acculturation in relation to the acquisition of a second language. Journal of Multilingual and Multicultural Development, 30(6), 481–492. https://doi.org/10.1080/01434630903147898
- Jin, B., & Kim, J. (2017). Grit, basic needs satisfaction, and subjective well-being. Journal of Individual Differences, 38(1), 29–35. https://doi.org/10.1027/1614-0001/a000219
- Joosten, T., & Cusatis, R. (2020). Online learning readiness. American Journal of Distance Education, 34(3), 180–193. https://doi.org/10.1080/08923647.2020.1726167
- Kalyaniwala, C., & Ciekanski, M. (2021). Autonomy CALLing: A systematic review of 22 years of publications in learner autonomy and CALL. Language Learning & Technology, 25(3), 106–131. http://hdl.handle.net/10125/73452
- Kashdan, T. B., Gallagher, M. W., Silvia, P. J., Winterstein, B. P., Breen, W. E., Terhar, D., & Steger, M. F. (2009). The curiosity and exploration inventory-II: Development, factor structure, and psychometrics. Journal of Research in Personality, 43(6), 987–989. https://doi.org/10.1016/j.jrp.2009.04.011
- Keramati, A., Afshari-Mofrad, M., & Kamrani, A. (2011). The role of readiness factors in E-learning outcomes: An empirical study. Computers & Education, 57(3), 1919–1929. https://doi.org/10.1016/j.compedu.2011.04.005
- Khabir, M., Jabbari, A. A., Fazilatfar, A. M., & Razmi, M. H. (2022). Flipped presentation of IELTS reading: Impacts on grit, autonomy, and reading achievement in an EFL context. Computer-Assisted Language Learning Electronic Journal (CALL-EJ), 23(2), 178–197. http://callej.org/journal/23-2/Khabir-Jabbari-Fazilatfar-Razmi2022.pdf
- Khajavy, G. H., & Aghaee, E. (2022). The contribution of grit, emotions and personal bests to foreign language learning. Journal of Multilingual and Multicultural Development, https://doi.org/10.1080/01434632.2022.2047192
- Khajavy, G. H., MacIntyre, P., & Hariri, J. (2021). A closer look at grit and language mindset as predictors of foreign language achievement. Studies in Second Language Acquisition, 43(2), 379–402. https://doi.org/10.1017/S0272263120000480
- Kırmızı, Ö. (2015). The influence of learner readiness on student satisfaction and academic achievement in an online program at higher education. Turkish Online Journal of Educational Technology, 14(1), 133–142. http://www.tojet.net/articles/v14i1/14114.pdf
- Knowles, M. S. (1975). Self-Directed Learning: A Guide for Learners and Teachers. Association Press.
- Knowles, M. S., Holton III, R. A. E. F., Swanson, R. A., Swanson, & Robinson, P. A. (2020). The Adult Learner: The Definitive Classic in Adult Education and Human Resource Development. [9th ed.] Routledge. https://doi.org/10.4324/9780429299612
- Kozak, M., & Piepho, H. P. (2018). What’s normal anyway? Residual plots are more telling than significance tests when checking ANOVA assumptions. Journal of Agronomy and Crop Science, 204(1), 86–98. https://doi.org/10.1111/jac.12220
- Krämer, N., Schäfer, J., & Boulesteix, A. L. (2009). Regularized estimation of large-scale gene association networks using graphical Gaussian models. BMC Bioinformatics, 10, 384. https://doi.org/10.1186/1471-2105-10-384
- Krönke, M. (2020). Africa’s digital divide and the promise of e-learning. Afrobarometer Policy Paper, 66 https://www.afrobarometer.org/wp-content/uploads/2022/02/pp66-africas_digital_divide_and_the_promise_of_e-learning-afrobarometer_policy_paper-14june20.pdf
- Lake, J. (2013). Positive L2 self: Linking positive psychology with L2 motivation. In Apple, T.M., Da Silva, D., & T. Fellner (Eds.), Language Learning Motivation in Japan (pp. 71–225). Multilingual Matters.
- Lan, X., & Moscardino, U. (2019). Direct and interactive effects of perceived teacher-student relationship and grit on student wellbeing among stay-behind early adolescents in urban China. Learning and Individual Differences, 69, 129–137. https://doi.org/10.1016/j.lindif.2018.12.003
- Larsen-Freeman, D., & Cameron, L. (2008). Complex Systems and Applied Linguistics. Oxford University Press.
- Lee, J. S., & Hsieh, J. C. (2019). Affective variables and willingness to communicate of EFL learners in in-class, out-of-class, and digital contexts. System, 82, 63–73. https://doi.org/10.1016/j.system.2019.03.002
- Lee, S.-M. (2022). Factors affecting incidental L2 vocabulary acquisition and retention in a game-enhanced learning environment. ReCALL. https://doi.org/10.1017/S0958344022000209
- Li, C., & Yang, Y. (2023). Domain-general grit and domain-specific grit: Conceptual structures, measurement, and associations with the achievement of German as a foreign language. International Review of Applied Linguistics in Language Teaching, https://doi.org/10.1515/iral-2022-0196
- Litman, J. A., & Spielberger, C. D. (2003). Measuring epistemic curiosity and its diversive and specific components. Journal of Personality Assessment, 80(1), 75–86. https://doi.org/10.1207/S15327752JPA8001_16
- Liu, C., He, J., Ding, C., Fan, X., Hwang, G. J., & Zhang, Y. (2021). Self-oriented learning perfectionism and English learning burnout among EFL learners using mobile applications: The mediating roles of English learning anxiety and grit. Learning and Individual Differences, 88, 102011. https://doi.org/10.1016/j.lindif.2021.102011
- Liu, H. J. (2012). Understanding EFL undergraduate anxiety in relation to motivation, autonomy, and language proficiency. Electronic Journal of Foreign Language Teaching, 9(1), 123–139. https://e-flt.nus.edu.sg/wp-content/uploads/2020/09/v9n12012/liu.pdf
- Lou, N. M., & Noels, K. A. (2017). Measuring language mindsets and modeling their relations with goal orientations and emotional and behavioral responses in failure situations. The Modern Language Journal, 101(1), 214–243. https://doi.org/10.1111/modl.12380
- Lou, N. M., & Noels, K. A. (2019). Promoting growth in foreign and second language education: A research agenda for mindsets in language learning and teaching. System, 86, 102–126. https://doi.org/10.1016/j.system.2019.102126
- Lou, N. M., & Noels, K. A. (2020a). “Does my teacher believe I can improve?” The role of meta-lay theories in ESL learners’ mindsets and need satisfaction. Frontiers in Psychology, 11, 1417. https://doi.org/10.3389/fpsyg.2020.01417
- Lou, N. M., & Noels, K. A. (2020b). Breaking the vicious cycle of language anxiety: Growth language mindsets improve lower-competence ESL students’ intercultural interactions. Contemporary Educational Psychology, 61, 101847. https://doi.org/10.1016/j.cedpsych.2020.101847
- Lou, N. M., & Noels, K. A. (2020c). Language mindset, meaning-making, and motivation. In M. Lamb, K. Csizér, A. Henry, & S. Ryan (Eds.), The Palgrave Handbook of Motivation for Language Learning (pp. 537–559). Palgrave Macmillan.
- Lou, N. M., & Zarrinabadi, N. (2022). Mindsets. In S. Li, M. Papi, & P. Hiver (Eds.), The Routledge Handbook of Second Language Acquisition and Individual Differences (pp. 125–144). Routledge.
- Lou, N., Chaffee, K., & Noels, K. (2022). Growth, fixed, and mixed mindsets: Mindset system profiles in foreign language learners and their role in engagement and achievement. Studies in Second Language Acquisition, 44(3), 607–632. https://doi.org/10.1017/S0272263121000401
- MacIntyre, P. D., Gregersen, T., & Mercer, S. (2020). Language teachers’ coping strategies during the Covid-19 conversion to online teaching: Correlations with stress, wellbeing and negative emotions. System, 94, 102352. https://doi.org/10.1016/j.system.2020.102352
- MacIntyre, P. D., Gregersen, T., & Mercer, S. (2019). Setting an agenda for positive psychology in SLA: Theory, practice, and research. The Modern Language Journal, 103(1), 262–274. https://doi.org/10.1111/modl.12544
- Mahmoodzadeh, M., & Khajavy, G. H. (2018). Towards conceptualizing language learning curiosity in SLA: An empirical study. Journal of Psycholinguistic Research, 48(2), 333–351. https://doi.org/10.1007/s10936-018-9606-3
- Martin, F., Stamper, B., & Flowers, C. (2020). Examining student perception of readiness for online learning: Importance and confidence. Online Learning Journal, 24(2), 38–58. https://doi.org/10.24059/olj.v24i2.2053
- McClendon, C., Neugebauer, R. M., & King, A. (2017). Grit, growth mindset and deliberate practice in online learning. Journal of Instructional Research, 6, 8–17. https://doi.org/10.9743/jir.2017.2
- Mercer, S. (2018). Psychology for language learning: Spare a thought for the teacher. Language Teaching, 51(4), 504–525. https://doi.org/10.1017/S0261444817000258
- Mercer, S., & Ryan, S. (2010). A mindset for EFL: Learners’ beliefs about the role of natural talent. ELT Journal, 64, 436–444. https://doi.org/10.1093/elt/ccp083
- Mikami, H. (2023). Revalidation of the L2-Grit scale: A conceptual replication of Teimouri, Y., Plonsky, L., & Tabandeh, F. (2022). L2 grit: Passion and perseverance for second-language learning. Language Teaching. https://doi.org/10.1017/S0261444822000544
- Mizumoto, A. (2023). Calculating the relative importance of multiple regression predictor variables using dominance analysis and random forests. Language Learning, 73(1), 161–196. https://doi.org/10.1111/lang.12518
- Nakamura, S., Reinders, H., & Darasawang, P. (2022). A classroom-based study on the antecedents of epistemic curiosity in L2 learning. Journal of Psycholinguistic Research, 51, 293–308. https://doi.org/10.1007/s10936-022-09839-x
- Ness Evans, A., & Rooney, B. J. (2018). Methods in Psychological Research. [4th ed.] SAGE.
- Noels, K. A., & Lou, M. (2015). Mindsets, goal orientations and language learning: What we know and what we can do. Contact, 41(2), 41–52. http://contact.teslontario.org/wp-content/uploads/2016/09/ResearchSymposium2015.pdf
- OECD (2020). Making the Most of Technology for Learning and Training in Latin America. OECD. https://doi.org/10.1787/ce2b1a62-En
- Opsahl, T., Agneessens, F., & Skvoretz, J. (2010). Node centrality in weighted networks: Generalizing degree and shortest paths. Social Networks, 32(3), 245–251. https://doi.org/10.1016/j.socnet.2010.03.006
- Oxford, R. L. (2016a). Toward a psychology of well-being for language learners: The ‘EMPATHICS’ vision. In: P. D. MacIntyre, T. Gregersen & S. Mercer (Eds.), Positive Psychology in SLA (pp. 10–88). Multilingual Matters. https://doi.org/10.21832/9781783095360-003
- Oxford, R. L. (2016b). Powerfully positive: Searching for a model of language learner well-being. In D., Gabryś-Barker & D. Gałajda (Eds.), Positive Psychology Perspectives on Foreign Language Learning and Teaching (pp. 21–38). Springer.
- Oxford, R. L., & Khajavy, G. H. (2021). Exploring grit: “Grit linguistics” and research on domain-general grit and L2 grit. Journal for the Psychology of Language Learning, 3(2), 7–36. https://doi.org/10.52598/jpll/3/2/2
- Paradowski, M. B., Cierpich-Kozieł, A., Chen, C.-C., & Ochab, J. K. (2022). How output outweighs input and interlocutors matter for study-abroad SLA: Computational Social Network Analysis of learner interactions. The Modern Language Journal, 106(4), 694–725. https://doi.org/10.1111/modl.12811
- Paradowski, M. B., Jarynowski, A., Czopek, K., & Jelińska, M. (2021). Peer interactions and second language learning: The contributions of Social Network Analysis in Study Abroad vs At-Home environments. In: R. Mitchell & H. Tyne (Eds.), Language, Mobility and Study Abroad in the Contemporary European Context (pp. 99–116). Routledge. https://doi.org/10.4324/9781003087953-8
- Paradowski, M. B., Jarynowski, A., Jelińska, M., & Czopek, K. (2021). Out-of-class peer interactions matter for second language acquisition during short-term overseas sojourns: The contributions of Social Network Analysis. Language Teaching, 54(1), 139–143. https://doi.org/10.1017/S0261444820000580
- Paradowski, M. B., &Jonak, Ł. (2012). Diffusion of linguistic innovation as social coordination. Psychology of Language and Communication, 16(2), 131–142. https://doi.org/10.2478/v10057-012-0010-z
- Peterson, C., & Seligman, M. E. P. (2004). Character Strengths and Virtues: A Handbook and Classification. American Psychological Association.
- Pintrich, P. R., & Schunk, D. H. (2014). Motivation in Education: Theory. Research, and Applications [4th ed.]. Merrill/Prentice Hall.
- Reinders, H., & White, C. (2011). Learner autonomy and new learning environments. Language Learning & Technology, 15(3), 1–3. https://doi.org/10.10125/44254
- Reinders, H., & White, C. (2016). 20 years of autonomy and technology: How far have we come and where to next? Language Learning & Technology, 20(2), 143–154. https://doi.org/10.10125/44466
- Ryan, R. M., & Deci, E. L. (2000). Self-determination theory and the facilitation of intrinsic motivation, social development, and well-being. American Psychologist, 55(1), 68–78. https://doi.org/10.1037//0003-066x.55.1.68
- Ryan, R. M., & Deci, E. L. (2017). Self-Determination Theory: Basic Psychological Needs in Motivation, Development, and Wellness. Guilford.
- Ryan, S., & Mercer, S. (2012). Language learning mindsets across cultural settings: English learners in Austria and Japan. OnCUE Journal, 6(1), 6–22. https://jaltcue.org/files/OnCUE/OCJ6-1/OCJ61%20pp%2006-22%20Ryan.pdf
- Saadé, R. G., He, X., & Kira, D. (2007). Exploring dimensions to online learning. Computers in Human Behavior, 23(4), 1721–1739. https://doi.org/10.1016/j.chb.2005.10.002
- Sato, T., Murase, F., & Burden, T. (2020). An empirical study on vocabulary recall and learner autonomy through mobile-assisted language learning in blended learning settings. CALICO Journal, 37(3), 254–276. https://doi.org/10.1558/cj.40436
- Smith, K. M., & Craig, H. (2013). Enhancing learner autonomy through CALL: A new model in EFL curriculum design. CALICO Journal, 30(2), 252–278. https://doi.org/10.11139/cj.30.2.252-278
- Song, L., & Hill, J. R. (2007). A conceptual model for understanding self-directed learning in online environments. Journal of Interactive Online Learning, 6(1), 27–42. https://www.ncolr.org/jiol/issues/pdf/6.1.3.pdf
- Spratt, M., Humphreys, G., & Chan, V. (2002). Autonomy and motivation: Which comes first? Language Teaching Research, 6(3), 245–266. https://doi.org/10.1191/1362168802lr106oa
- Stefanou, C. R., & Salisbury-Glennon, J. D. (2002). Developing motivation and cognitive learning strategies through an undergraduate learning community. Learning Environments Research, 5, 77–97. https://doi.org/10.1023/A:1015610606945
- Sudina, E., & Plonsky, L. (2021). Academic perseverance in foreign language learning: An investigation of language-specific grit and its conceptual correlates. The Modern Language Journal, 105(4), 829–857. https://doi.org/10.1111/modl.12738
- Sudina, E., Brown, J., Datzman, B., Oki, Y., Song, K., Cavanaugh, R., Thiruchelvam, B., & Plonsky, L. (2021). Language-specific grit: Exploring psychometric properties, predictive validity, and differences across contexts. Innovation in Language Learning and Teaching, 15(4), 334–351. https://doi.org/10.1080/17501229.2020.1802468
- Takkaç-Tulgar, A. (2018). The effects of curiosity on second language learning in terms of linguistic, socialcultural and pragmatic development. Journal of Educational Sciences, 9(2), 59–72. https://dergipark.org.tr/en/pub/aduefebder/issue/41071/433937
- Teimouri, Y., Plonsky, L., & Tabandeh, F. (2022). L2 grit: Passion and perseverance for second-language learning. Language Teaching Research, 26(5), 893–918. https://doi.org/10.1177/1362168820921895
- Teimouri, Y., Sudina, E., & Plonsky, L. (2021). On domain-specific conceptualization and measurement of grit in L2 learning. Journal for the Psychology of Language Learning, 3(2), 156–165. https://doi.org/10.52598/jpll/3/2/10
- Torun, E. D. (2020). Online distance learning in higher education: E-learning readiness as a predictor of academic achievement. Open Praxis, 12(2), 191–208. https://doi.org/10.5944/openpraxis.12.2.1092
- UNESCO. (2022). Education in Latin America and the Caribbean at a Crossroads. https://reliefweb.int/report/world/education-latin-america-and-caribbean-crossroads-sdg4-education-2030-regional-monitoring-report
- Ushioda, E. (1996). Learner Autonomy 5: The Role of Motivation. Authentik.
- Ushioda, E. (2013). Motivation matters in mobile language learning: A brief commentary. Language Learning & Technology, 17(3), 1–5. https://doi.org/10.10125/44333
- Wang, S.-K., & Hsu, H.-Y. (2008). Use of the webinar tool (Elluminate) to support training: The effects of webinar-learning implementation from student-trainers’ perspective. Journal of Interactive Online Learning, 7(3), 175–194. https://www.ncolr.org/jiol/issues/pdf/7.3.2.pdf
- Wei, H., Gao, K., & Wang, W. (2019). Understanding the relationship between grit and foreign language performance among middle school students: The roles of foreign language enjoyment and classroom environment. Frontiers in Psychology, 10, 1508. https://doi.org/10.3389/fpsyg.2019.01508
- Yamashita, T. (2018). Grit and Second Language Acquisition: Can Passion and Perseverance Predict Performance in Japanese Language Learning? [Unpublished MA thesis]. University of Massachusetts.
- Yang, P. (2021). Exploring the relationship between Chinese EFL students’ grit, well-being, and classroom enjoyment. Frontiers in Psychology, 12, 762945. https://doi.org/10.3389/fpsyg.2021.762945
- Yap, B. W., & Sim, C. H. (2011). Comparisons of various types of normality tests. Journal of Statistical Computation and Simulation, 81(12), 2141–2155. https://doi.org/10.1080/00949655.2010.520163
- Yu, T., & Richardson, J. C. (2015). An exploratory factor analysis and reliability analysis of the student online learning readiness (SOLR) instrument. Online Learning, 19(5), 120–141. https://doi.org/10.24059/olj.v19i5.593
- Zarrinabadi, N., Lou, N. M., & Ahmadi, A. (2022a). Resilience in language classrooms: Exploring individual antecedents and consequences. System, 109, 102892. https://doi.org/10.1016/j.system.2022.102892
- Zarrinabadi, N., Rezazadeh, M., & Mohammadabadi, A. M. (2022b). L2 grit and language mindsets as predictors of EFL learners’ attitudes toward effectiveness and value of CALL. Computer Assisted Language Learning, https://doi.org/10.1080/09588221.2022.2108061
Appendix 1.
Scales used in the study
L2 grit scale (adapted from Teimouri et al., 2020)
I am a diligent foreign language learner.
My interests in learning a foreign language change from year to year.
When it comes to foreign languages, I am a hard-working learner.
I think I have lost my interest in learning (the) foreign language(s).
Now that I have decided to learn a foreign language, nothing can prevent me from reaching this goal.
I will not allow anything to stop me from my progress in learning a foreign language.
I am not as interested in learning a foreign language as I used to be.
I was obsessed with learning a foreign language in the past but recently I have lost interest. (modified item)
I put much time and effort into overcoming my foreign language weaknesses. (modified item)
Language Mindsets Inventory (LMI; Lou & Noels, Citation2017)
I can’t change how capable I am of learning new languages.
To be honest, I can’t really change my ability to learn and use new languages.
My ability to learn new languages is to a large extent innate and I can’t change much.
I can always improve how good I am at learning new languages.
I can always improve my ability to learn new languages.
No matter how much ability I have in learning new languages, I can improve it considerably.
Basic Psychological Need Satisfaction and Frustration Scale – Autonomy (BPNSNF, Vensteenkiste et al., 2015)
I feel a sense of choice and freedom in the things I undertake.
Most of the things I do feel like “I have to”.
I feel that my decisions reflect what I really want.
I feel forced to do many things I wouldn’t choose to do.
I feel my choices express who I really am.
I feel pressured to do too many things.
I feel I have been doing what really interests me.
My daily activities feel like a chain of obligations.
The curiosity and exploration inventory-II (Kashdan et al., 2009)
In new situations, I actively seek as much information as I can.
I am the type of person who really enjoys the uncertainty of everyday life.
I am at my best when I do something that is complex or challenging.
Everywhere I go, I am out looking for new things or experiences.
I view challenging situations as an opportunity to grow and learn.
I like to do things that are a little frightening.
I am always looking for experiences that challenge how I think about myself and the world.
I prefer jobs that are excitingly unpredictable.
I frequently seek out opportunities to challenge myself and grow as a person.
I am the kind of person who embraces unfamiliar people, events, and places.
Self-directed learning (new scale)
I set the specific goals in my learning.
I manage my learning time well.
I complete my assignments on time.
I can control my own learning progress.
Learning motivation (new scale)
I am curious about many things.
I enjoy solving problems.
I get pleasure from learning.
I hope to constantly improve my learning outcomes.
I will never be too old to learn new things.
I enjoy learning about many new things.
While learning, I enjoy challenges.
I believe that I can learn a lesson no matter how complicated.
I like learning, regardless of my learning results.
I will not give up learning when I encounter difficulties.