ABSTRACT
Aerobic granulation is a recent technology with high level of complexity and sensitivity to environmental and operational conditions. Artificial neural networks (ANNs), computational tools capable of describing complex non-linear systems, are the best fit to simulate aerobic granular bioreactors. In this study, two feedforward backpropagation ANN models were developed to predict chemical oxygen demand (Model I) and total nitrogen removal efficiencies (Model II) of aerobic granulation technology under steady-state condition. Fundamentals of ANN models and the steps to create them were briefly reviewed. The models were respectively fed with 205 and 136 data points collected from laboratory-, pilot-, and full-scale studies on aerobic granulation technology reported in the literature. Initially, 60%, 20%, and 20%, and 80%, 10%, and 10% of the points in the corresponding datasets were randomly chosen and used for training, testing, and validation of Model I, and Model II, respectively. Overall coefficient of determination (R2) value and mean squared error (MSE) of the two models were initially 0.49 and 15.5, and 0.37 and 408, respectively. To improve the model performance, two data division methods were used. While one method is generic and potentially applicable to other fields, the other can only be applied to modelling the performance of aerobic granular reactors. R2 value and MSE were improved to 0.90 and 2.54, and 0.81 and 121.56, respectively, after applying the new data division methods. The results demonstrated that ANN-based models were capable simulation approach to predict a complicated process like aerobic granulation.
GRAPHICAL ABSTRACT
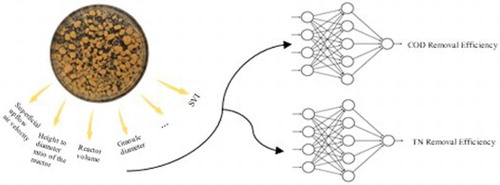
Disclosure statement
No potential conflict of interest was reported by the authors.