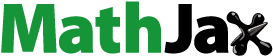
ABSTRACT
This study investigates the relationship between use and investor diversity, spatial accessibility, and high street retail rents. Spatial quantitative analysis of the high street retail sector remains an underdeveloped area so this paper seeks to bridge this gap and contribute to the debate on the adaptability of urban retailing centres by adopting a spatial fixed-effects panel modelling approach. The empirical findings reveal that diversity and richness in property use tend to have a significant positive impact on retail rental values. The influence of ownership richness on rents is positive implying that rents tend to be higher on streets where there is a greater range in the type of landlords. Walkability, as a measure of spatial accessibility, is found to have a negative relationship with market rents. This is perhaps surprising as it had been expected that the most walkable streets in retailing centres to be the most connected and have the highest rents. This contrary finding may be due to large developments interrupting the street network and restricting the choice and movement of pedestrians. Location on the prime retail pitch has a significant positive relationship with shop rents, whereas proximity to transportation nodes has a less consistent influence.
1. Introduction
The dynamics of urban retailing markets and the interrelationship between resilience and the adaptability of properties have received particular attention in the recent literature and policy debates in the UK. These discussions have typically been around refocusing the purpose of high streets and creating more balanced places that better serve the needs of local communities and visitors (Grimsey, Citation2018; Housing, Communities and Local Government Committee, Citation2019; Portas, Citation2011), but the effects of diversifying the use of retailing units on market rents have largely been ignored. Studies, such as Orr et al. (Citation2021a), have demonstrated that rebalancing the use mix is changing the spatial distribution of retailing and other land uses in urban centres. However, it is possible the resultant changes in the richness and diversity of land and building use have impact on the pricing of property in these markets as new uses compete against established operators for existing retailing space. This could have the effect of pushing up rents in highly sought prime locations; whereas in locations where national and multinational retailers have retreated and vacancy rates are rising you would expect new uses filling the void to pay less for this space. This is particularly true if operators are small scale, independent businesses who are less profitable than the larger multiple retailers and less able to pay high rents. This would typically result in a widening of the rent differential between prime and secondary retailing pitches. It is even possible these changes, which could be influenced by new developments, have resulted in the contraction and movement in prime pitch, as evident in the Optimised Hotspot Analysis conducted by Orr et al. (Citation2021a), and changed the pricing dynamics in these markets.
Hence, the objective of this paper is to undertake quantitative analysis to examine the relationship between diversity and richness, spatial accessibility, and retail rents using unique datasets containing information on properties located within the primary retailing areas of Edinburgh, Glasgow, Hull, Liverpool and Nottingham at four different time points between 2000 and 2017. Rateable values, as a proxy for net effective market rents, are modelled to measure the significance of the use and investor mix (measured by richness and diversity), representation of chain retailers, micro-location attributes, and walkability in determining market rents. In analysing urban retail rent determinants in this way it seeks to provide a temporal comparison that will aid understanding of how diversity in the changing retailing landscape has impacted on market rents and the implied preferences of city centre occupiers. These findings have direct implications for the viability of retail investment activity and adaptive capacity of retailing systems.
While previous pricing studies have investigated the relationship between spatial accessibility, the movement of shoppers, and retail rents (e.g. Adebayo et al., Citation2019; Hillier, Citation1996), to our knowledge this is the first study to model the impact of use and ownership mix on retail rents with or without spatial accessibility metrics. In doing so, it contributes to the literature on the pricing of retail assets (e.g. Eppli et al., Citation1998; Freybote et al., Citation2016; Hui et al., Citation2007; Kaiser & Freybote, Citation2021; Nase et al., Citation2012), and complements the work of Dolega and Celińska-Janowicz (Citation2015) who conceptualise the connections between the adaptive capacity of retail property markets and the resilience of the High Street.
The findings evidence a positive and statistically significant relationship between use diversity and retail rents. This result has implications for retail landlords, town centre managers and policy-makers as it supports the notion that use diversity has a direct impact on the vitality and viability of retailing centres, and should be facilitated in the management of shopping malls and more generally, urban centres. The findings also demonstrate the influence of investor mix and distribution on the rents occupiers pay. Furthermore, the findings identify a negative relationship between walkability (as measured by syntax analysis) and retail rents, suggesting there is scope for the relative connectivity of the main retailing streets to be improved.
The remainder of this paper is structured into six sections. The next section examines the literature to investigate the impact of use mix and spatial accessibility on retailing activity and the role this plays in creating attractive shopping destinations. Section three sets out the broad modelling framework used in this empirical study, including the definitions and measurement approach of the key variables under investigation, while section four details the methodological approach adopted by explaining the study areas and data employed. The results of the retail spatial modelling are revealed in section five, while the final section discusses the implications of the findings with conclusions on pricing dynamics and the future of retailing centres drawn.
2. The importance of diversity and spatial accessibility in urban retailing centres
Place attractiveness can contribute to the pulling power of a retailing location. Public services, range of shops and services and architecture are just a few of the essential elements that contribute to the attractiveness of an urban centre to residents and visitors. Retail centre consumers are themselves diverse and complex entities, and expect urban centres to serve a variety of functions and to facilitate social interaction. Their interactions with the city centre are based on a ‘bundle of services and experiences’ (Warnaby & Davies, Citation1997) and that bundle varies within and across groups that are, further, subject to marked changes in behavioural patterns. Academics now recognise the diverse tastes and behaviours of consumers (Grimsey, Citation2012), a marked change since the earliest models of retail centres that were predicated on assumptions of the uniform shopper (such as Reilly’s (Citation1929, Citation1931) gravity model and Christaller’s (Citation1933) Central Place Theory).
Shopping remains a primary motivation for users of urban centres. Warnaby and Davies estimated in 1997 that 75% and 55–65% of trips to urban retailing centres made by non-tourists and tourists, respectively, were to shop; however, behaviours have changed markedly over the last two decades. Retailing, no longer perceived as a single purposed essential activity, is now recognised as a leisure pursuit and the co-location of retailing with other leisure provisions is very much part of the shopping experience (Dolega & Lord, Citation2020; Jones & Livingstone, Citation2018). In addition, consumer behaviour has rallied against the homogenisation of retail centres experienced in the 1990s and 2000s, now setting the challenge for urban centres to provide a distinctive and diverse retail offering, local character and a sense of place (Carmona, Citation2015).
The local community also finds value in retailing environments that serve as a place for interaction and socialisation (Landry et al., Citation2005) and, as White (Citation2014) explains, some groups of consumers’ needs include provision for ‘meeting, seating, playing, weeing’, seemingly at odds with traditional retail-focused views. Thus, place quality lies at the heart of modern retail policy as policy-makers seek to encourage the reimagining of retailing centres, not as mere shopping destinations, but as vibrant places that can satisfy the wider needs of the local community and visitors. Use richness and diversity are indicators of vitality and place attraction as they capture the range of retail, leisure services and public and private amenities on offer to consumers. The greater the vitality of a centre, potentially the greater its viability and attraction to investors as it draws more retailers and leisure operators who are willing to pay higher rents to secure the occupation of highly sought-after premises.
Yet, it is not just about an increase in the heterogeneity of urban functions and occupiers that increases the attractiveness of urban centres. Walkability, street layout and connectivity are as important as the available diverse retail and service outlets must be within walking reach of shoppers (Adebayo et al., Citation2019; Campoli, Citation2012). The scale of provision within close proximity remains a consideration for many consumers as clusters directly impact on the vibrancy of a location, increase the interaction in a space, and facilitate multi-purpose shopping trips that help time constrained shoppers use their time more efficiently (Oner, Citation2017). Sevtsuk (Citation2014) argues locations with better access to surrounding destinations have a higher probability of attracting comparison retailers who he finds are more likely to co-locate, as are restaurants and bars in the US. These competitive clusters, consisting of complementary stores that sell related but non-competing goods, benefit from agglomeration by capitalising on the multi-purpose shopping trips shoppers make.
The more spatially connected, accessible and visible a property, the greater the volume of passing potential shoppers who can be enticed into the unit and the higher the potential turnover generated by the retailer. Hence, shops in locations with greater connectivity tend to be more sought after by retailers, which results in these units commanding higher market rents than less accessible stores. Spatial accessibility, and how it promotes or impedes pedestrian footfall within in-town retail markets, is an important driver of the spatial distribution of retailers and shop values.
It is possible that changes in the movement of pedestrians explain changes in the dynamics of retail centres. O’Roarty et al. (Citation1997), using a rank-order survey to investigate the store selection criteria used by retailers in the UK, find that store position on the prime or secondary retailing pitches, which tend to be the most accessible locations within retailing, is an important determinant of demand for a shop. The study also reveals that access and proximity to transport termini are other location factors considered by retailers. These findings imply that models used to investigate the determinants of retail rents should also try to control for the influence of walkability and other spatial accessibility attributes as these have a significant bearing on what retailers are willing to pay for a unit.
3. Modelling retail rental values
Despite various quantitative analyses based on inter-urban retail models, intra-urban retail rent modelling remains in its infancy. Typically published retail hedonic models are used to investigate the determinants of rents in shopping malls (e.g. Benjamin et al., Citation1990; Des Rosiers et al., Citation2005; Hardin & Wolverton, Citation2001; Hardin et al., Citation2002; Mejia & Benjamin, Citation2002). Sirmans and Guirdy (Citation1993) is one such study that includes measures of drawing power, centre design, location characteristics and market characteristics to model retail rents achieved in 55 shopping malls in Louisiana during the 1989–1991 period. Mejia and Benjamin (Citation2002) concur with the earlier models in that they emphasise the importance of non-spatial factors, such as the tenant mix and image of anchor retailers, in determining shopping centre rents. Hardin et al. (Citation2002) and Des Rosiers et al. (Citation2005) also agree non-spatial factors are important determinants but argue there is a need to include spatial determinants as well in shopping centre rent models.
Very few published studies have sought to model retail rents on the high street, largely because of the difficulty in getting access to usable rent data. Nase et al.’s (Citation2012) study of Belfast city centre is one of the few empirical studies that has modelled retail rent transactions. The specific focus of their model is the impact of design quality on rents and they find urban design features, such as connectivity, frontage continuity and variety, material quality and massing appropriateness, have a significant effect on the value of retail units.
A spatial hedonic modelling framework underpins this study to investigate the relationship between the diversity of use and ownership, and retail rents while controlling for spatial accessibility, micro-location and non-spatial attributes. Hedonic property pricing is underpinned by the assumption that the price of a property is directly associated with the utility generated from the consumption of the attributes of that property. Lancaster (Citation1966) formalises the consumer theory that Rosen () develops into the pricing framework that has become an established technique used to examine the implicit pricing of housing attributes and determine which factors have a significant impact of house prices. More recently, this approach has been employed to model the relationship between spatial accessibility and pricing of commercial space. For instance, Enström and Netzell (Citation2008) estimate office rents in Stockholm using a model derived from production theory and the cost minimisation problem faced by business occupiers. In their study they include space syntax, measured by global integration,Footnote1 to capture the impact of spatial accessibility on intra-urban office rents.
Pedestrian movement is linked to the walkability of a retailing centre and this can be captured using space syntax with Hillier et al. () discovering pedestrian movement to be positively correlated with integration. Netzell (Citation2013) is one of the few studies to examine the use of space syntax variables to model urban retail rents and finds local integration values to be a determinant of retail rents in Stockholm. Brown’s (Citation1999) comparative study of two shopping malls in Denver uses space syntax to demonstrate higher vacancies and tenant turnover occur in fringe locations with lower integration, and furthermore he finds that spatial configuration was contributing to the failings of the less successful mall. Ha et al.’s (Citation2020) more recent study, however, finds integration within a shopping centre does not have a significant effect on retail store sales in South Korea. Adebayo et al. (Citation2019) is one of the few studies to use both axial and segment analysis metrics to model the change in retail rateable values in York. They find these variables to be significant determinants but the reliability of their findings are open to question as there are no regression diagnostics, other than goodness-of-fit, provided to enable the robustness of the model to be gauged.
3.1 Measuring walkability
Walkability refers to the ease by which pedestrians can move between two points. This is influenced by a range of factors including street connectivity, attractiveness and width of walkways, existence of obstacles, how safe the area is perceived, and land‐use density and diversity (Campoli, Citation2012; Lo, Citation2009). Previously studies have measured walkability as a distance or time between two points. For example, Pivo and Fisher (Citation2011) devised a walkability score based on distance to key destinations to examine the impact of walkability on market values and investment returns. Other studies use survey techniques such as stated preference, on-street and ‘on the move’ to investigate the preference of walkers (Kelly et al., Citation2011). However, using such approaches to monitor walkability at retrospective time points is problematic.
In this study a walkability index is calculated using space syntax analysis using historic street maps. This is a form of graphic analysis that has been adapted to enable the spatial layout of urban streets and patterns of human activity to be analysed by representing the streets and paths within a centre as a network of lines and intersections that reveal how pedestrians can move from one space to the next without passing through intervening spaces (Hillier, Citation1996). These lines are called axial lines, and a network of intersecting axial lines drawn to represent the streets and paths within a defined urban area is referred to as an axial map. The ease of pedestrian movement and navigation between spaces within this network of lines and intersections can be quantitatively measured by converting into a segment map where the axial lines are broken at their intersections into segments and each segment represents a section of a street or path (axial line) that lies between two points of intersection. Segment angular analysis is a technique used to assess the accessibility of a street network based upon the shortest angular journey through the spatial network and is particular powerful when the street pattern is a uniform grid or when linear streets cross regular streets diagonally (Al-Sayed et al., Citation2014).
Pre-drawn Ordinance Survey (OS) road maps of the five case study centres are used to draw axial and then segment maps. The centre of road lines, from LandLine OS maps as at May 2005, and OS MasterMap Highways maps as at June 2010 and May 2017, spanning the city centre of the five case studies are imported into DepthMapX, and simplified by removing unnecessary road traffic features, such as small roundabouts (excluding ones with statues and inaccessible gardens), traffic Islands, and entrances into car parks and bus stations that are represented on some maps, and straightening exaggerated street curves. Pedestrianised roads, lanes, alleyways and other pedestrian routes not shown in the street map are drawn in as axial lines, and intersecting roads and paths where ‘between movement’ is not possible are unlinked.
Segment angular choice is calculated by counting the number of times each street (segment) is present on the shortest, straightest route between all pairs of streets (segments) within a selected distance. A normalised version of segment angular choice enables comparison to be made between urban street systems of different sizes and between different cities. As derived by Hillier et al. (Citation2012), NACH400 is the normalised angular choice measure based on a 400 m radius and is calculated as
Depth refers to the number of angular turns to move from one space to another so ATD400, the Angular Total Depth, is the sum of the depths from one segment to all other segments within the defined radius and ACH400 is the corresponding angular choice measure. A 400 m radius, which represents a walking distance of 5 minutes, is used as the radius as recommended in Al-Sayed et al. (Citation2014).
Segment angular integration represents how close a segment is to all others and is measured as the sum of the angular changes made on the routes between segments. NAIN400, normalised angular integration based on a 400 m radius, can be calculated as
EquationEquation 2(2)
(2)
where NC is Node Count and is the number of segments encountered on route from a segment to all others within the 400 m radius (Hillier et al., Citation2012).
A single index of walkability (NORM_WALKABILITYt) that captures both integration and choice, and avoids potential problems with multicollinearity, is derived by multiplying normalised angular integration (NAIN400) with normalised angular choice (NACH400).
3.2. Measuring use and investor diversity
As previously discussed, the role of diversity is widely recognised as important in retailing centres, a fundamental requisite to achieving a higher rate of adaptability and is a prerequisite for self-organisation in what is a complex system (Wrigley & Dolega, Citation2011). Measuring diversity in retailing studies has taken different forms. The simplest, and probably most common, measure has been the count of the number of different retail uses present in a retailing centre or mall with variants of this metric including the number of retailers or total amount of floor space occupied by different types of retailers, typically disaggregated into shopping trip purpose or broad categories. URBED and Comedia (Citation1994), for example, recommend the number and variety of different retail and leisure uses as measures of vitality, and are frequently used in retail planning studies.
Many understandings and measures of diversity stem from ecology. Whilst the nature of what is being studied differ, ecological economics applies ecological concepts to explain economic activity and is employed by Orr et al. (Citation2021b) to measure diversity within retailing systems. Richness (R), for example, is a useful ecological concept that can be applied to measuring diversity within a retailing system. In ecology this refers simply to the total number of different types of species in a community, but can be applied here to represent the total number of different use categories within the market. However, richness does not take into account the relative abundance of different species (or in this case uses) and so Peet (Citation1974) argues that a true measure of species diversity is one that captures both richness and evenness. The Gini-Simpson Index (GSIoD) is a diversity index frequently used to measure diversity (Jost, Citation2006):
where nl is the number of retailers or operators within use classification l in a retailing market and N is the total number of retailers or operators and R, richness, represents the total number of different types of use categories. The value of this index variant ranges between 0 and 1 as the greater the value, the greater the sample diversity.
Richness and Gini-Simpson index metrics are calculated from point data for each retailing centre and each time point. A 100 m × 100 mFootnote2 fishnet grid is created with every individual property unit assigned to a square cell within the grid. Count analyses are used to count the presence of different categories of known use operators (ignoring vacant units and units where use could not be confirmed) within each grid cell, which in turn, are converted into diversity indices for each cell. Similar measures to capture investor richness and diversity are also devised to investigate the influence ownership fragmentation might have on retail rents.
3.3 The model
In this study, we employ a cross-sectional pricing framework, similar in approach to proceeding hedonic studies, to investigate the influence of use and ownership diversity and spatial accessibility on intra-urban retail rents at different time points. Our model, however, is developed as a static spatial panel model, using cross-sectional data stacked for five different urban retailing centres and estimated separately for four different time points, to enable individual centre influences on retail rents to be separated from common rent determinants at different points in time. This can be written as
where lnRV.ITZAit represents the natural logarithm of the rental value per square metre In Terms of Zone A (ITZA)Footnote3 for individual retail unit i at time point t, and Attractionit, SpatAccessit, Marketit and Use&Physicalit are vectors of the place attraction, spatial accessibility and market activity characteristics, and use and physical features associated with individual retail properties, respectively. β1, β2, β3 and β4 denote vectors of respective regression coefficients, αc represents the fixed effect for city c in which the properties are located and εit is the idiosyncratic error term.
Micro-level measures of place attraction (Attractionit) are included in our model for property use and ownership richness and diversity. The richness and Gini-Simpson Index of Diversity variables employed are as defined in the previous section.
Accessibility, measured as a proximity of shoppers to shopping centres is central to traditional spatial models, such as retail gravity models, as a way to understand shopping behaviour (Guy, Citation1983). Measures of accessibility that attempt to capture the walkability of urban centres or the proximity of a property to specific locations, such as transport nodes, are also significant determinants of retail rents although it depends on the type of transportation. In their model of shopping mall rents Tay et al. (Citation1999) reveal proximity to the entrance of the Mass Transit Railway increases shop rents whereas proximity to a taxi rank has an insignificant effect. This suggests that distance-based measures of accessibility, such as the distance to a railway station, may be possible complementary micro-level measures of spatial accessibility (SpatAccessit) that drive the variation in rents within retailing centres.
A number of studies have explored relationships between various different types of spatial accessibility and retail rents. Whilst some of these had contrasting findings, they are useful in highlighting variables which need to be taken account of in our model. Yiu (Citation2011), for example, shows that pedestrianisation increased the rent achieved by High Street shops in Hong Kong by 17%. Tinessa et al. (Citation2020) also highlight the impact of pedestrianisation as they find, using a stated preference survey, retailers in Naples are willing to pay more for shops located closer to pedestrian areas than metro stations.
Drawing from these prior studies, the spatial accessibility vector (SpatAccessit) in our model includes a dichotomous variable to represent units on a pedestrian street as well as other measures to capture units being located on the prime pitch. Following Brown (Citation1999), Adebayo et al. (Citation2019), and Ha et al. (Citation2020), the walkability index defined in the previous section will also be included in the model.
The literature, as reviewed in Jackson (Citation2001), shows that retail rents, aggregated at the local, regional and national level can be influenced by spatial determinants such as size and affluence of the local population. Affluence can be captured by employment and unemployment rates and socio-economic indicators such as the percentage of owner occupied housing, proportion of households with two cars, size of the retail core and representation of multiple retailers (Jackson, Citation2001). These social-economic measures tend to explain local wealth and spending patterns which ultimately impact on the vibrancy of a retail market in its entirety and help explain inter-urban rents, but are generally unavailable as small area statistics for many time points.Footnote4 Instead, the model contains market statistics (Marketit) such as vacancy rates, representation of specific neighbouring land uses, average income and crime scores that are employed as potential drivers of intra-urban rent variation while the fixed effects included in the model (αc) capture the effects of unobserved city-specific factors, such as the size of the retail core, on rents.
Hui et al. (Citation2007), in a study of retail rents in Hong Kong, discover that shops located within a shopping mall tend to have higher rents than shops located on open shopping streets so the use and physical characteristics (Use&Physicalit) include a dichotomous variable that measures if the property is located within a shopping mall. National and international-chain occupiers have also been found to pay rent premia (Tay et al., Citation1999) so a variable is included to capture occupiers being part of a chain (CHAIN_t). Anchor tenants are large-chain occupiers who receive discounts to anchor shopping centre developments (e.g. Carter & Vandell, Citation2005; Damian et al., Citation2011; Mejia & Benjamin, Citation2002) but a separate anchor variable is not included in our model as they tend not to influence rents for standalone properties in the high street in the same way as these units are owned by different investors.
Other non-spatial variables that are included in our model to capture the use and physical features of the retail units occupied by retailers and food and beverage (F&B) operators (Use&Physicalit). This includes inverse quantum (INV_QUANTUM_t). This is a pricing effect that sometimes occurs in UK retail markets where smaller than average retail units command a disproportionately higher rent per square metre than larger retail units. A variable is also included to differentiate between shop and F&B use. This is defined as a dichotomous variable where 0 represents comparison and convenience shops, and 1 represents F&B outlets as retailers tend to pay more rent than leisure operators.
4. Markets and data
The study models the retail rents in the principal retail areas, as set by local development plans and strategic policy guidance, within five major UK cities: Edinburgh, Glasgow, Hull, Liverpool, and Nottingham. Focusing on a mix of cities reflects potential variation in the nature of occupier and private sector investor activity, while the northern sample ensures that the analysis controls for north–south economic imbalances, which Wrigley and Dolega (Citation2011) found influential on the resilience of retailing centres. Comparison of different time points permits investigation into the significance of diversity of the performance of retail assets in changing retail markets that function as ‘regional hubs’.
4.1. Rental data
Collecting market rent data at a single time point in a large enough sample that enables robust regression modelling is difficult. The data collection is further complicated by the different lease terms and incentives that can be agreed between the landlord and tenant when a commercial property is let.
The rent samples used to operationalise the retail model in each of the case study centres consist of rateable values (In Terms of Zone A per square metre (RV.ITZA)) for retail units in retail and food and beverage use located within the principal retailing areas of the five case studies. Rateable values are estimates of the market rents for the units at the effective dates of 1 April 2000, 1 April 2005, 1 April 2010 and 1 April 2017. Net rateable values provide a proxy measure of the net annual rent estimated from a single source and are based on standardised lease terms. They are estimated by the Valuation Assessors Office (VOA) and Scottish Assessors Association (SAA), which are government bodies in England and Scotland set up to undertake property valuations for local taxation purposes. The net annual rent is derived using a standardised valuation methodology and transaction evidence of open market Zone A rents achieved for retail units comparable to the subject property being valued.Footnote5 ITZA is the standard measurement practice used by all UK valuers to control for differences in the size and layout of retail units when analysing comparable evidence and valuing subject properties.
4.2 Other data variables
Variable labels, definitions, source of data and data transformations that are employed in the static spatial panel models are summarised in .
Table 1. Data and sources.
5 Modelling procedure and results
The semi-log models are initially estimated using ordinary least squares in GeoDaSpace, and then refined to a parsimonious specification using a downward stepwise process to minimise the Akaike Information Criterion and reduce the multicollinearity condition number to below 30. White standard errors are used to estimate robust standard errors as the Breusch-Pagan and Koenker-Basset tests (see the bottom of the result tables) point to heterogeneity being present in all the models.
The parsimonious model is then re-estimated using three different spatial regression specifications in GeoDaSpace that control both for spatial autocorrelation (a spatial lag, spatial error and joint spatial lag and error model) and heteroskedasticity. In order to estimate the spatial parameters in a spatial lag and spatial error model, a spatial weights matrix is needed. These spatial weights represent spatial autocorrelation that occurs when one retail property in the sample influences neighbouring units in some way, and are estimated using a Euclidian distance function. A 500 m distance cut-off is used to capture the spatial dependence that can occur within retail clusters. The average shopper can typically walk 400 m in 5 minutes (Al-Sayed et al., Citation2014) so a distance set a little wider at 500 m is used to capture the most pronounced of these spatial processes.
The spatial lag model incorporates the effect of the dependent variable (lnRV.ITZAit) of a property influencing the value of neighbouring properties by including in the model.
is a scalar parameter that indicates the magnitude of influence of the spatially lagged dependent variable
. To adequately account for the spatial simultaneity in the models, the spatial lag model estimation is based on an instrumental variable approach, developed and named by Anselin (Citation1988) as a spatial two-stage least squares (S2SLS).
The spatial error model in contrast captures the influence of unmeasured independent variables on the error term. It is specified by adapting the error term () in Equation 4 so
where
is a spatial lag for the errors, λ is the autoregressive coefficient, and
is another error term. The estimation of the spatial error model is based on the general methods of moments (GMM) estimation of the spatial error model proposed by Kelejian and Prucha (Citation2010) and Drukker et al. (Citation2011). The estimation process consists of two stages: the first stage estimates the model coefficients using a generalised least squares approach that employs a spatial Cochrane-Orcutt transformation to obtain a consistent spatial autoregressive parameter λ. The second stage estimates λ (see, Anselin et al., Citation2012) and employs the Kelejian and Prucha (Citation2010) method (KP-HET) to generate a covariance matrix that is robust in the presence of both spatial heteroskedasticity and autocorrelation.
The joint spatial lag and spatial error model contains both spatial lag and spatial error terms. The modelling process is similar to the estimation process of the spatial error model but adds a third step to the spatial error method approach to estimate and the regression coefficients in a spatially weighted regression.
5.1 Findings
The panel regression results showing the development of the models for each of the four time points (excluding the fixed effects) are presented in . The most appropriate spatial specification for each time point, selected using the significant Robust LM (lag) and Robust LM (error) spatial dependence tests at the bottom of the tables, is highlighted in shade. For 2000 (), 2005 () and 2010 () this is the spatial lag and error model, while a spatial error model is selected for 2017 (). It is these final versions of the models that will now be examined.
Table 2. Fixed effects panel regression models for 2000Footnote6.
Table 3. Fixed effects panel regression models for 20056.
Table 4. Fixed effects panel regression models for 20106.
Table 5. Fixed effects panel regression models for 20176.
While the panel regressions reveal that the variables of interest – use and ownership diversity and richness, and walkability – have had significant impacts on rental values, the exact specification of the models varies from time point to time point. Five determinants are found to be consistently significant: occupiers that are part of a regional, national or international chain (CHAIN_t), location on the prime pitch (PRIME_PITCH_t), diversity of use (GSIoD_t) and richness in ownership (OWN_RICH_t) are significant and positive determinants of rental values in 2005, 2010 and 2017 while the only difference in 2000 is the negative sign for GSIoD. Food and beverage use (BUSE_CATEGORY_t1) also has a significant impact on retail rents although this is negative in 2000, 2005 and 2010 and becomes positive in 2017, possibly reflecting the increasing use of shops as coffee shops, restaurants and fast-food outlets in the five cities under study. While food and beverage (F&B) outlets are now recognised as complementary to retailing and the experiential economy, in earlier years landlords were less willing to let units to F&B operators on the more expensive retailing streets as they had potential to disrupt the pedestrian flow. F&B operators may also have perceived little benefit in paying higher rents to locate adjacent to retailers.
Starting with place attraction (Attractionit), use richness (RICH_t) and diversity (GSIoD_t) are found to be significant drivers of rents, although the statistical significance of these two variables depends on the time point. In 2000 richness in neighbouring land use has a positive influence on market rents while lower use diversity results in higher market rents, reflecting the uneven spread of different property uses in retailing centres at this point in time. This changes in 2005, 2010 and 2017 when use diversity is found to have a positive influence. This may represent the increase in the relative abundance of different property uses as fashion and clothing, variety and department shops, which historically clustered in the areas of the city centres commanding the highest rents, increasingly changed use. The relationship between market rents and use richness appears to breakdown in 2017 as this variable is found to be statistically insignificant.
The picture is less variable with ownership. Richness in ownership has a positive impact in all time periods. This suggests that locations in the case study cities with greater variation in the range of ownership types, and presumable ownership fragmentation, have higher rateable values. Ownership diversity is insignificant in 2000 but is found to have a statistically significant negative relationship with market rents in 2005, 2010 and 2017. This implies that greater evenness in the relative spread of more types of ownership results in lower rateable values whereas areas of the retailing centre where there are clusters of low variation in type of owners, such as exists for shopping malls, tend to command higher rents.
The micro-location variables representing spatial accessibility (SpatAccessit) in the models tend to have a significant impact on rateable values. At all time periods, being located on the prime retail pitch has a significant positive impact on estimated values, and the variable employed reflects the shift in retailing pitches identified in Orr et al. (Citation2021a). The decrease in the magnitude of the prime pitch coefficient between 2005 and 2010 might reflect a reduction in the rent achievable for units situated on the weakening sections of the prime pitch prior to the more extreme pitch changes between 2010 and 2017. Being located on a pedestrianised street has no effect in 2000 but is found to have a significant influence on the setting of 2005, 2010 and 2017 rents. This complements the findings in previous studies, such as Tinessa et al. (Citation2020).
Walkability is found to be significant in 2000, 2005 and 2010 but has a negative relationship with retail rents and in 2010 an extremely low coefficient. This finding could be reflecting breaks in the regular street patterns in the case study centres associated with large developments, such as shopping malls and railway stations, that tend to locate close to the primary retailing streets. In addition, the street patterns themselves maybe irregular. For instance, in Nottingham and Hull the medieval city centres have an irregular network of lanes while Princes Street in Edinburgh, which historically achieved the highest retail values in the city, is bounded on one side by Princes Street Gardens. These irregularities might explain why the retailing streets that command the highest market rents have a lower walkability measure than other streets in the city centre where rents are lower but offer a greater choice of direct routes for shoppers.
The walkability index is found to have an insignificant relationship with market rents in 2017. It is difficult to see how the streetscapes in the case study centres have changed sufficiently to warrant this shift in the relationship between walkability and rents, although some road and public realm improvements were made between 2010 and 2017. For example, in Edinburgh some modifications were made to the layout of roads in the city centre to accommodate the trams and improvements to the public realm were made in Hull in preparation for the city becoming UK City of Culture in 2017. A more likely explanation is that a structural change in pricing has occurred in the market. It is possible that, as shoppers have switched to online shopping and become less dependent on physical retailing units, there has been a reduction in the number of units occupied by comparison retailers and the replacement occupiers are less willing to pay higher rents for the more accessible retail locations. Another point to consider is that the prime pitch coefficient has risen between 2010 and 2017, suggesting that the units on the prime pitches remain highly sought after by some retailers. It is these opposing market forces that may have resulted in the walkability variable being insignificant.
Proximity to transportation nodes is found to be insignificant at the time points where measures are available and can be included in the models. This finding contradicts other studies (i.e. Gautsch, Citation1981; Guy, Citation1983) that argue that transportation options effect shopping behaviours. As the fall in multicollinearity condition number reveals when many of the transportation accessibility measures are removed in the determination of the parsimonious models, these variables typically display multicollinearity with other variables in the model. The exceptions are proximity to subway stations in 2000 where the rent commanded by a unit is higher when situated closer to a subway station whereas it would not be unexpected if rent, although found to insignificant in 2017, is lower the closer the shop is to a bus stop, reflecting the tendency for bus routes to be located on the less expensive retailing streets and the more expensive streets to be pedestrianised. The presence of a ferry connection within 1000 m is found to be insignificant , expect for the spatial lag and error model selected for 2000.
The use and physical features of a property (Use&Physicalit) should have an impact on the estimated rental value of a shop. In this group of variables, the presence of chain occupiers is found to have a consistently positive effect on estimated values in all four time periods. It is possible, going forward, that this pricing relationship may have been weakened by the change in market conditions over the last 4 years. The increase in the collapse of many multiple retailers, the restructuring of the corporate real estate portfolios as retailers have struggled to embrace omni-channel retail and material uncertainty created by the pandemic have seen a contraction in occupation demand from chain retailers. Increasingly more shops are being let by independent retailers and operators who cannot afford to pay the higher rents multiple retailers would once pay. Also, shops used by F&B operators are found to achieve lower market rents in 2000, 2005 and 2010 although this relationship becomes positive in 2017 as these property uses expanded and became more established in the main retailing streets.
The results for inverse quantum effects and being located in a shopping mall are more variable. Inverse quantum is the pricing relationship typically found in UK urban centres where smaller than average units, such as kiosk and stalls, achieve higher rents per square metre than bigger shops. This variable is found to be insignificant in 2000, 2005 and 2017. This suggests temporal variation in market demand for small and large retail units. The variable is statistically significant in 2010 but the negative coefficient suggests smaller than average units have a lower estimated rent per square.
Being located in a shopping mall has no effect on rental values in 2005. It does have a statistically positive relationship with rents in 2000, 2010 and 2017. and maybe reflects the changing dominance of shopping mall retail space in the case study centres. Hull, Liverpool and Edinburgh all experienced retail-led urban renewal between 2005 and 2010 which resulted in new shopping mall floorspace being constructed. This new supply may havecommanded a price premium with occupiers being prepared to pay more for flexible space that better matches their requirements .
To capture market activity (Marketit), variables are estimated that measure the portion of different property uses (accommodation services (Srt_ASt), business, commercial and office space (Srt_BCOt), public and social value services (Srt_PSVSt), residential units (Srt_RESt), convenience shops (Srt_SEt), and comparison shops (Srt_SNt)) in close proximity to the properties in the sample. Overall, these variables have an insignificant effect but there are some exceptions. For example, the availability of public and social value services, unsurprisingly due to their relative low representation in city centres in 2000 and 2005, has an insignificant effect on shop values in these years but becomes significant in 2010 and 2017. This relationship is negative, suggesting rents are lower where more public and social value services tend to locate. The proportion of neighbouring office space in the immediate area is statistically significant in 2005, 2010 and 2017. This finding makes sense as offices tend not to be located on the higher value retail streets. It also explains why smaller independents, that occupy the cheaper units located outside the main retailing streets, have been hardest hit during the pandemic by home working as they completely rely on the trade from these absent city centre office workers.
The availability of close convenience retailers has an insignificant effect on estimated rents for units occupied by retail and F&B operators in 2010 and 2017 but are significant in 2000 and 2005. The negative relationship suggesting rents are lower where a greater proportion of these retailers locate which is where you would expect to find these large store formats. However, the insignificance relationship in later years might be due to the wider spread of convenience stores that occurred across city centres in response to changing shopping behaviours following the Great Financial Crisis. The availability of close comparison retailers has a positive significant effect on rents in 2000 but is removed in 2005, 2010 and 2017 as this variable is highly correlated with other variables. This is supported by the fall in the multicollinearity condition number when removed from the models.
Interestingly, residential and vacancy rates as neighbouring land uses are found to have an insignificant impact on rents, which the exception of residential in 2010. You would expect a negative relationship between the vacancy of neighbouring properties and shop rents but, like Hui et al. (Citation2007) who found vacancy rates to have no impact on shopping mall rents, no spatial pattern is identifiable. There is also no consistent relationship between neighbouring residential land use and rents but this result might reflect the lack of a spatial pattern in the representation of residential units across the case study markets. Edinburgh always has had a high presence of residential units within the city centre. In Nottingham, Liverpool and Hull the number of housing units have steadily grown, growing by at least 52% between 2010 and 2017, but Glasgow which only increased by 2% in the same period seems to be lagging behind (Orr et al., Citation2021a).
Unemployment and crime rates are included in the full model specification. Again, these variables are highly correlated with other variables and removed from the parsimonious models.
6. Discussion and conclusions
The study finds that walkability and use and ownership diversity and richness have had significant impacts on rental values, proxied by rateable values, in 2000, 2005, 2010 and 2017. The positive relationship between use diversity and rents in 2017 suggests the more diverse the use of property and greater representation of different types of uses the greater levels of competition for space that have pushed up market rents. The rise in use diversity that occurred in these cities between 2010 and 2017 is captured here. This change has occurred as these cities, like many retailing centres in the UK, have been forced by the rise of online shopping to transform from retail dominant centres into attractive experience-orientated and leisure destinations. The finding that use diversity measure is negatively related to rental values in 2000 might reflect the uneven clusters of comparison retailing that previously existed and commanded higher market rents. In the wake of the pandemic, the increasing collapse of large occupiers and surge in vacancy rates have pushed market rents down but it is not unrealistic to expect the positive relationship between market rents and use diversity to persist. Vacancy rates, on the other hand, should now have a statistically negative relationship with market rents as they have become a more dominant influence in the market.
The consistent positive relationship between ownership richness and market rents seems to reflect that the ownership of shops on the prime pitch tends to be more varied and fragmented, and these streets typically command higher rents. Yet, it could also be that a fragmented pattern of ownership generates information asymmetries that result in larger market players having greater market power. The finding that ownership diversity has a negative relationship with market rents, complements this theory as it suggest that where there is less variation in the evenness of different types of owner, larger dominant landlords have the power to push up rents.
Chain retailers also appear to have a significant positive relationship with rental values. This finding concurs with other works that have found the performance of retail markets is linked to the existence of national and international retailers (for example, Tay et al., Citation1999). Moving forward, however, as the retailing industry continues to restructure, particularly in the wake of the Covid-19 pandemic, many multiple retailers are withdrawing from many retailing centres. These vacated units are increasingly being occupied by new uses or independent retailers so it is possible the rent premia paid by multiples retailers will diminish in future years, if not already being felt in the market.
Previous research has found that accessibility, when measured as a connectivity index, had a significant positive impact on rents in Belfast (Nase et al., Citation2012). This study, however, finds that walkability, as measured by segment analysis metrics, does not appear to have a positive relationship with rateable values across the five case study centres, whereas proximity to transport nodes has a variable effect. Spatial accessibility as measured by proximity to the nearest and subway station (for 2000) and location on the pedestrian streets and prime pitch have a positive effect on shop values unlike walkability.
The negative relationship between retail rental values and spatial accessibility holds for the single index measure of walkability. This implies the retailing streets perceived to be the most accessible are less connected and walkable but the finding might also be due to the street network in these centres not following a perfect street grid layout. The existence of shopping malls and other large developments and public gardens, typically located on or adjacent to the retailing streets with higher rents, will break up the street grid. This street morphology would then result in relatively lower space syntax measure and possibly explain why our measure of walkability is negatively related to the variation in retail values. Another possibility is that the spatial syntax measure used in this study to capture the presence and integration of walking routes does not accurately reflect the user friendliness of retailing streets. Comparison of the influence of alternative measures of walkability on retail rents should be considered for future research.
Interestingly, the significant determinants in the models pick up the changes in prime pitches identified in Hull, Liverpool and Edinburgh in a prior study by Orr et al. (Citation2021a). This adds weight to the argument that the dynamics of these retail markets have adapted to structural changes in supply and demand. There is a possibility that the negative relationship between walkability and rents is due to multicollinearity in the model between the prime pitch variable and measures of walkability and access to transport as prime retail pitches tend to be located in the most accessible and walkable locations. However, the models are run with and without the prime pitch variable and appear to have no impact on the significance of the transport variables, direction of influence with the walkability index or multicollinearity condition number.
The models also allow for spatial interactions, spatial lag and error correction being the most suitable specification, and suggests future attempts at retail modelling by researchers should embrace this finding. The variation in model specification across time points reinforces the suggestion that there have been structural changes in the dynamics of these markets. Not surprising as the retail market is always in a state of flux but these findings further suggest that it is not appropriate to model retail markets across different time points as one single dynamic panel spatial model.
Overall, the findings of this paper contributes to the literature by adding to our understanding of use diversity and spatial accessibility, and the influence they have on shop rents. These factors also contribute to the attraction of retailing centres. The identified connections between retail rents, use diversity and shifting market dynamics should give policy-makers, landowners and other stakeholders preoccupied with the future of our city centres something to reflect on as retailing centres recover from the pandemic and enter into a new cycle of development. For policy-makers, they should continue to encourage use diversity in city centres. The Use Class Order revisions introduced in England in September 2020 provides much-needed flexibility to encourage use change but planning restrictions still remain in place in Scotland. Scottish planners should monitor the effects of these changes in England to see if Scottish retailing centres would benefit from similar changes.
Walkability and connectivity are important to the attractiveness of city centres, and movement of users. Footfall is routinely measured across most urban centres but not walkability. The spatial syntax measure used in this study measures walkability in terms of choice and integration of the street layout but not the overall quality of experience. It might be helpful for standard metrics to be established by local authorities to monitor the connectivity improvements being achieved in city centres. Examples of ongoing public-sector projects in the case study centres include Better Roads in Liverpool, The Avenues in Glasgow and the green space and reconnection of the ancient streets planned for Nottingham city centre offer the opportunity for changes in walkability to be investigated more in-depth.
For investors, the findings demonstrate that higher use diversity leads to higher market rents being achievable in the market. This should, perhaps not immediately as the effects of the pandemic will take time to shake off, result in better retail investment performance. Landlords will then be motivated to maintain, refurbish and adapt the retail assets they hold. This will increase the adaptive capacity of these retailing centres as many investors have deferred making such improvements due to the uncertainty of income streams during the pandemic. A surplus of retail space has also clearly emerged in many city centres. Repurposing these buildings into uses that complement the remaining retail will further strengthen the diversity and appeal of these centres, which in turn will increase the resilience of these local economies.
Acknowledgments
This work was supported by the Economic, Social and Research Council [grant number ES/R005117/1]. The authors are also grateful to the data service at Urban Big Data Centre for providing access to data.
Disclosure statement
No potential conflict of interest was reported by the author(s).
Additional information
Funding
Notes on contributors
Allison M Orr
Allison M Orr joined Urban Studies at the University of Glasgow in June 2006 as a senior lecturer. She has extensive experience modelling the pricing of commercial and residential property markets, and researching urban change and decision-making in the direct investment market.
Joanna L Stewart
Joanna L Stewart is a Lecturer based in Urban Studies, University of Glasgow. Her research interests draw from a range of academic disciplines including geography, spatial analysis, planning, retailing, transport, health and wellbeing.
Notes
1. Integration is defined as the number of street-to-street turns needed to move from one street (represented as axial lines in axial analysis) to all other street, using the shortest path. Local integration calculates the total number of directional changes, usually within a set radius whereas global integration indicates the closeness of an axial line (measured as the shortest distance) to all other axial lines in the entire network of segments under review (Hillier, Citation1996).
2. 100 m grid squares are used to capture the character of the immediate neighbourhood. While street blocks in the case study cities greatly vary, they tend to be around 100 m in length.
3. Zoning is the measurement methodology used for comparing shops of different sizes and layouts in the UK, and used in the valuation and appraisal of retail units. The zone depths depend on local custom – typically 6.096 m in English cities and 9.14 m in Scottish cities. Each zone is weighted to convert the shop area into the equivalent Zone A which is the most valuable zone at the front of the shop. The weights generally are based on the principle of halving-back from Zone A.
4. Small area statistic data on employment, home ownership and households with cars are produced using census data. The UK Census is undertaken every 10 years with the most recent dataset currently available being for 27 March 2011. A census was undertaken Spring 2021 in England but delayed in Scotland until 2022. There is usually a two year lag before the data is processed and available for analysis.
5. Rateable values are set at the open market rent at the tone date although are used to determine the net rateable value for the property use as at the effective date.
6. *, **, and *** denote statistical significance at the 1, 5, and 10% level respectively.
References
- Adebayo, J., Greenhalgh, P., & Muldoon-Smith, K. (2019). Investigating retail property market dynamics through spatial accessibility measures. Journal of European Real Estate Research, 12(2), 155–172. https://doi.org/10.1108/JERER-01-2018-0009
- Al-Sayed, K., Turner, A., Hillier, B., Lida, S., & Penn, A. (2014). Space syntax methodology. Bartlett School of Architecture, UCL.
- Anselin, L. (1988). Spatial econometrics: Methods and models. Kluwer Academic Press.
- Anselin, L., Amaral, P. V., & Arribas-Bel, D. (2012) Technical aspects of implementing GMM estimation of the spatial error model in PySAL and GeoDaSpace, Working Paper 2/12, GeoDa Center, Arizona State University.
- Benjamin, J. D., Boyle, G. W., & Sirmans, C. F. (1990). Retail leasing: Determinants of shopping center rents. Journal of the American Real Estate and Urban Economics Association, 18(3), 302–312. https://doi.org/10.1111/1540-6229.00524
- Brown, M. G. (1999). Design and value: Spatial form and the economic failure of a mall. Journal of Real Estate Research, 17(1/2), 189–226. https://doi.org/10.1080/10835547.1999.12090970
- Campoli, J. (2012). Made for walking: Density and neighborhood form. Lincoln Institute of Land Policy. https://urbandesignresources.org/wp-content/uploads/2013/12/2150_1509_MFW_Web_Chapter.pdf
- Carmona, M. (2015). London’s local high streets: The problems, potential and complexities of mixed street corridors. Progress in Planning, 100, 1–84. https://doi.org/10.1016/j.progress.2014.03.001
- Carter, C., & Vandell, K. (2005). Store location in shopping centers: Theory and estimates. Journal of Real Estate Research, 27(3), 237–266. https://doi.org/10.1080/10835547.2005.12091160
- Christaller, W. (1933). Central places in Southern Germany. translated by C. Baskin ( 1966. Prentice-Hall.
- Damian, D. S., Curto, J. D., & Pinto, J. C. (2011). The impact of anchor stores on the performance of shopping centres: The case of Sonae Sierra. International Journal of Retail & Distribution Management, 39(6), 456–475. https://doi.org/10.1108/09590551111137994
- Des Rosiers, F., Thériault, M., & Menetrier, L. (2005). Spatial versus non-spatial determinants of shopping center rents: Modeling location and neighborhood-related factors. Journal of Real Estate Research, 27(3), 293–320. https://doi.org/10.1080/10835547.2005.12091158
- Dolega, L., & Celińska-Janowicz, D. (2015). Retail resilience: A theoretical framework for understanding town centre dynamics. Studia Regionalne i Lokalne, 60(2) , 8–31. doi:10.7366/1509499526001.
- Dolega, L., & Lord, A. (2020). Exploring the geography of retail success and decline: A case study of the Liverpool City Region. Cities, 96. January , 102456. https://doi.org/10.1016/j.cities.2019.102456
- Drukker, D. M., Prucha, I. R., & Raciborski, R. (2011) A command for estimating spatial- autoregressive models with spatial-autoregressive disturbances and additional endogenous variables, Technical Report, Stata Corp.
- Enstrom, R., & Netzell, O. (2008). Can space syntax help us in understanding the intraurban office rent pattern? accessibility and rents in downtown Stockholm. The Journal of Real Estate Finance and Economics, 36(3), 289–305. https://doi.org/10.1007/s11146-007-9054-6
- Eppli, M. J., Shilling, J. D., & Vandell, K. D. (1998). What moves retail property returns at the metropolitan level? The Journal of Real Estate Finance and Economics, 16(3), 317–342. https://doi.org/10.1023/A:1007783900583
- Freybote, J., Simon, L., & Beitelspacher, L. (2016). Understanding the contribution of curb appeal to retail real estate values. Journal of Property Research, 33(2), 147–161. https://doi.org/10.1080/09599916.2015.1135978
- Gautsch, D. A. (1981). Specification of patronage models for retail center choice”. Journal of Marketing Research, 18(2), 162‐74. https://doi.org/10.1177/002224378101800204
- Grimsey, B. (2012). Sold out. Filament Publishing.
- Grimsey, B. (2018) Grimsey Review 2: It's time to reshape our town centres. London: Bill Grimsey vanishinghighstreet.com. http://www.vanishinghighstreet.com/wp-content/uploads/2018/07/GrimseyReview2.pdf .Accessed 9 December 2021.
- Guy, C. M. (1983). The assessment of access to local shopping opportunities: A comparison of accessibility measures. Environment and Planning B, 10(2), 219–238. https://doi.org/10.1068/b100219
- Ha, S. J., Jang, S., Yang, K. W., & Ro, S. H. (2020). ERAM as a complementary method of spatial syntax: Comparison of methodologies by linking spatial analysis with income-producing efficiency for a retail outlet in South Korea. International Journal of Urban Sciences, 24(4), 516–531. https://doi.org/10.1080/12265934.2020.1742190
- Hardin, W. G., & Wolverton, M. L. (2001). Neighborhood center image and rents. The Journal of Real Estate Finance and Economics, 23(1), 31–46. https://doi.org/10.1023/A:1011112707018
- Hardin, W. G., Wolverton, M. L., & Carr, J. (2002). An empirical analysis of community center rents. Journal of Real Estate Research, 23(1/2), 163–178. https://doi.org/10.1080/10835547.2002.12091078
- Hillier, B. (1996). Space is the Machine. A configurational theory of architecture. Cambridge University Press.
- Hillier, B., Penn, A., Hanson, J., Grajewski, T., & Xu, J. (1993). Natural movement: Or, configuration and attraction in urban pedestrian movement. Environment and Planning B: Planning and Design, 20(1), 29–66. https://doi.org/10.1068/b200029
- Hillier, B., Yang, T., & Turner, A. (2012). Normalising least angle choice in DepthMap and how it opens up new perspectives on the global and local analysis of city space. The Journal of Space Syntax, 3(2), 155–193.
- Housing, Communities and Local Government Committee (2019), High streets and town centres in 2030 (Eleventh Report of Session 2017-19), House of Commons.
- Hui, E. C. M., Yiu, C. Y., Yau, Y., & Ooi, J. (2007). Retail properties in Hong Kong: A rental analysis. Journal of Property Investment & Finance, 25(2), 136–146. https://doi.org/10.1108/14635780710733825
- Jackson, C. (2001). A model of spatial patterns across local retail property markets in Great Britain. Urban Studies, 38(9), 1445–1471. https://doi.org/10.1080/00420980120076740
- Jones, C., & Livingstone, N. (2018). The ‘online high street’ or the high street online? The implications for the urban retail hierarchy. The International Review of Retail, Distribution and Consumer Research, 28(1), 47–63. https://doi.org/10.1080/09593969.2017.1393441
- Jost, L. (2006). Entropy and diversity. OIKOS, 113(2), 363–375. https://doi.org/10.1111/j.2006.0030-1299.14714.x
- Kaiser, C., & Freybote, J. (2021). Is e-commerce an investment risk priced by retail real estate investors? An investigation. Journal of Property Research. AHEAD OF PRINT , 1–18. https://doi.org/10.1080/09599916.2021.1996447
- Kelejian, H., & Prucha, I. R. (2010). Specification and estimation of spatial autoregressive models with autoregressive and heteroskedastic disturbances. Journal of Econometrics, 157(1), 53–67. https://doi.org/10.1016/j.jeconom.2009.10.025
- Kelly, C. E., Tight, M. R., Hodgson, F. C., & Page, M. W. (2011). A comparison of three methods for assessing the walkability of the pedestrian environment. Journal of Transport Geography, 19(6), 1500–1508. https://doi.org/10.1016/j.jtrangeo.2010.08.001
- Lancaster, K. J. (1966). A new approach to consumer theory. Journal of Political Economy, 74(2), 132–157. https://doi.org/10.1086/259131
- Landry, T. D., Arnold, T. J., & Stark, J. B. (2005). Retailer community embeddedness and consumer patronage. Journal of Retailing and Consumer Services, 12(1), 65–72. https://doi.org/10.1016/j.jretconser.2004.03.001
- Lo, R. H. (2009). Walkability: What is it? Journal of Urbanism, 2(2), 145–166 https://doi.org/10.1080/17549170903092867.
- Mejia, L., & Benjamin, J. (2002). What do we know about the determinants of shopping center sales? Spatial vs. non-spatial factors. Journal of Real Estate Literature, 10(1), 1–26. https://doi.org/10.1080/10835547.2002.12090107
- Nase, I., Berry, J., & Adair, A. (2012). Hedonic modelling of high street retail properties: A quality design perspective. Journal of Property Investment & Finance, 31(2), 160–178. https://doi.org/10.1108/14635781311302582
- Netzell, O. (2013). The effect of accessibility on retail rents: Testing integration value as a measure of geographic location. Journal of Property Research, 30(1), 1–23. https://doi.org/10.1080/09599916.2012.713974
- O’Roarty, B., McGreal, S., & Adair, A. (1997). The impact of retailers’ store selection criteria on the estimation of retail rents. Journal of Property Valuation & Investment, 15(2), 119–130. https://doi.org/10.1108/14635789710166349
- Oner, O. (2017). Retail city: The relations between place attractiveness and accessibility to shops. Spatial Economic Analysis, 12(1), 72–91. https://doi.org/10.1080/17421772.2017.1265663
- Orr, A. M., Stewart, J., Jackson, C., & White, J. T. (2021a) Spatial externalities and adaptation in the property market within a dynamic retailing system, ESRC REPAIR Project Working Paper 3, University of Glasgow, Glasgow.
- Orr, A. M., Stewart, J., Jackson, C., & White, J. T. (2021b) Not quite the ‘death of the high street’: Rising vacancy rates and the shift to land use richness and diversity in UK city centres, ESRC REPAIR Project Working Paper 2, University of Glasgow, Glasgow.
- Peet, R. K. (1974). Measurement of Species Diversity. Annual Review of Ecology and Systematics, 5(1), 285–307. https://doi.org/10.1146/annurev.es.05.110174.001441
- Pivo, G., & Fisher, J. D. (2011). The walkability premium in commercial real estate investments. Real Estate Economics, 39(2), 185–219. https://doi.org/10.1111/j.1540-6229.2010.00296.x
- Portas, M. (2011). The portas review: An independent review into the future of our high streets. HM Government.
- Reilly, W. J. (1929). Methods for the study of retail relationships. University of Texas Bulletin.
- Reilly, W. J. (1931). The Law of Gravitation. Knickerbocker Press.
- Rosen, S. (1974). Hedonic prices and implicit markets: Product differentiation in pure competition. Journal of Political Economy, 82(1), 34–55. https://doi.org/10.1086/260169
- Sevtsuk, A. (2014). Location and agglomeration: The Distribution of retail and food businesses in dense urban environments. Journal of Planning Education and Research, 34(4), 374–393. https://doi.org/10.1177/0739456X14550401
- Sirmans, C. F., & Guirdy, K. A. (1993). The determinants of shopping centre rents. Journal of Real Estate Research, 8(1), 107–115. https://doi.org/10.1080/10835547.1993.12090700
- Tay, R., Lau, C. K., & Leung, M. S. (1999). The determination of rent in shopping centers: Some evidence from Hong Kong. Journal of Real Estate Literature, 7(2), 183–196. https://doi.org/10.1080/10835547.1999.12090080
- Tinessa, F., Pagliara, F., Biggiero, L., & Veneri, G. D. (2020). Walkability, accessibility to metro stations and retail location choice: Some evidence from the case study of Naples. Research in Transportation Business and Management September . https://doi.org/10.1016/j.rtbm.2020.100549
- URBED and Comedia. (1994). Vital and viable town centres: Meeting the challenge. London: Department of Environment.
- Warnaby, G., & Davies, B. J. (1997). Commentary: Cities as service factories? Using the servuction system for marketing cities as shopping destinations. International Journal of Retail and Distribution Management, 25(6), 204–210. https://doi.org/10.1108/09590559710175953
- White, S. (2014). Who is the city centre for? presentation at ShOPPS’ workshop “integrating interests: Future-proofing city centre retail economies?”. University of Sheffield. 31 March – 1 April 2014.
- Wrigley, N., & Dolega, L. (2011). Resilience, fragility, and adaptation: New evidence on the performance of UK high streets during global economic crisis and its policy implications. Environment and Planning A, 43(10), 2337–2363. https://doi.org/10.1068/a44270
- Yiu, C. Y. (2011). The impact of a pedestrianisation scheme on retail rent: An empirical test in Hong Kong. Journal of Place Management and Development, 4(3), 231–242. https://doi.org/10.1108/17538331111176057