ABSTRACT
Organisations increasingly rely on algorithms to exert automated managerial control over workers, referred to as algorithmic control (AC). The use of AC is already commonplace with platform-based work in the gig economy, where independent workers are paid for completing a given task (or “gig”). The combination of independent work alongside intensive managerial monitoring and guidance via AC raises questions about how gig workers perceive AC practices and judge their legitimacy, which could help explain critical worker behaviours such as turnover and non-compliance. Based on a three-dimensional conceptualisation of micro-level legitimacy tailored to the gig work context (autonomy, fairness, and privacy), we develop a research model that links workers’ perceptions of two predominant forms of AC (gatekeeping and guiding) to their legitimacy judgements and behavioural reactions. Using survey data from 621 Uber drivers, we find empirical support for the central role of micro-level legitimacy judgements in mediating the relationships between gig workers’ perceptions of different AC forms and their continuance intention and workaround use. Contrasting prior work, our study results show that workers do not perceive AC as a universally “bad thing” and that guiding AC is in fact positively related to micro-level legitimacy judgements. Theoretical and practical implications are discussed.
1. Introduction
Control has been portrayed as “management’s most fundamental problem” (Cardinal et al., Citation2017, p. 559). In this regard, algorithmic control (AC) – broadly defined as the managerial use of intelligent algorithms and advanced digital technology as a means to align worker behaviours with organisational objectives (Cram & Wiener, Citation2020; Kellogg et al., Citation2020) – represents an emerging phenomenon. For example, a recent New York Times article predicts that using “algorithms to manage workers will not simply be a niche phenomenon [but] may become one of the most common ways of managing the American labor force” (Scheiber, Citation2017).
The use of AC is already commonplace with platform-based work in the gig economy (e.g., Duggan et al., Citation2020; Goldbach et al., Citation2018; Möhlmann et al., Citation2021). Enabling the effective “scaling of operations by […] coordinating the activities of large, disaggregated workforces” (Mateescu & Nguyen, Citation2019, p. 3), AC represents a key ingredient to the success of gig economy platforms (Jabagi et al., Citation2019; Wood et al., Citation2019). A prime example is the gig firm Uber, which relies on an app-based AC system to control its remote workforce of some four million freelance drivers (e.g., Möhlmann et al., Citation2021; Scheiber, Citation2017).
Despite workers’ freelance status, gig firms such as Uber leverage AC to exercise “tight” control over their workforce (Constantiou et al., Citation2017, p. 232; cf. Porter & van den Hooff, Citation2020), with a focus on controlling who is allowed to commence, or to continue, working for the firm (gatekeeping) and how workers conduct their daily work (guiding). The combination of independent work and tight control raises questions around how gig workers perceive and judge the AC practices used by gig companies, and how workers behave in reaction to these practices. Here, scholars argue that control research has not adapted to the dramatic changes ushered in by the digital era in general (e.g., Cardinal et al., Citation2017; Wiener et al., Citation2019), and by algorithmic technologies in particular (e.g., Kellogg et al., Citation2020; Schafheitle et al., Citation2020). Kellogg et al. (Citation2020), for example, point out that “scholarship has not kept pace with the ways that algorithmic technologies have the potential to transform organizational control in profound ways, with significant implications for workers” (p. 367). Two empirical and practical observations are particularly noteworthy in this respect: First, the few existing studies on AC that adopt, or at least consider, the perspective of individual workers show a strong tendency towards portraying AC systems as a universally “bad thing” (e.g., Möhlmann & Henfridsson, Citation2019; Rosenblat & Stark, Citation2016). For example, focusing on the case of Uber, Möhlmann and Henfridsson (Citation2019) identify three areas of consistent driver complaints about working “for” algorithms (constant surveillance, little transparency, and dehumanisation), which are largely in keeping with worker concerns identified in related studies (e.g., Curchod et al., Citation2020; Duggan et al., Citation2020; Wood et al., Citation2019). Second, while being highly reliant on AC, platform-based gig firms such as Uber are increasingly struggling with low worker retention rates (e.g., Anderson, Citation2016; Hall & Krueger, Citation2015; Scheiber, Citation2017) and workers’ use of workarounds in order to game the system (e.g., Lee et al., Citation2015; Möhlmann & Zalmanson, Citation2017).
In light of these observations, prior research suggests that AC can be frustrating to gig workers, prompting them to question the legitimacy of AC practices, which can lead workers to discontinue working for a platform or violate controls (Curchod et al., Citation2020; Möhlmann et al., Citation2021) and cause serious harm to companies (e.g., Möhlmann & Henfridsson, Citation2019). As such, studying the notion of micro-level legitimacy judgements (Bitektine & Haack, Citation2015) – broadly defined as an individual’s assessment that “the actions of an entity [i.e., a gig company in the context of our study] are desirable, proper, or appropriate” (Suchman, Citation1995, p. 574) – may help link gig workers’ perceptions of two predominant AC forms (gatekeeping and guiding) to their behavioural reactions (continuance intention and workaround use). Examining legitimacy-related issues in the specific context of the gig platform economy is also interesting because, in traditional “human-to-human” control relationships, the controller’s ability to influence the controllee’s behaviour is typically derived from legitimate sources of social power, such as a manager’s formal position in the organisational hierarchy or legal contracts (Berger et al., Citation1998; French & Raven, Citation1959; Lammers et al., Citation2008). However, none of these sources seem to apply to the controller-controllee relationships prevalent in platform-based gig work (e.g., Curchod et al., Citation2020; Deng et al., Citation2016; Möhlmann et al., Citation2021).
Against this backdrop, the study at hand builds on a theory of micro-level legitimacy put forth by Bitektine and Haack (Citation2015) to develop a research model focusing on two questions: (1) How does the use of gatekeeping and guiding AC relate to gig workers’ legitimacy judgements? (2) How are such judgements related to gig workers’ continuance intention and workaround use? To answer these questions, our study focuses on Uber, which has been described as a “particularly interesting” and “extreme” case of AC (Möhlmann et al., Citation2021). In particular, we examine our research questions by means of an online survey with 621 Uber drivers from the United States, thereby responding to recent calls for more empirical work on AC systems (e.g., Gal et al., Citation2020). An overarching contribution of our study lies in presenting theory-informed arguments and offering empirical evidence that counter the generally negative rhetoric surrounding the adoption of AC systems in platform-based gig work (and beyond). It thus points to the need for a more balanced view of AC. Further, our study contributes to extant research by theorising on, and empirically supporting, the focal role that gig workers’ legitimacy judgements play in mediating the relationships between their perceptions of gatekeeping and guiding AC, on the one hand, and their continuance intention and workaround use, on the other hand. As well, the study results provide practitioners with actionable insights into the basic characteristics of AC mechanisms that are effective from an organisational viewpoint, while being viewed as “legit” (i.e., desirable, proper, and appropriate) by workers themselves. As such, our study also responds to recent calls for “more research on platform work design, focusing on how to better balance the interests of platforms and their workers” (Möhlmann et al., Citation2021, p. 47).
The paper is structured as follows: First, we review prior work on AC and introduce concepts that form a foundation for our work. Next, we develop our research model and describe our methodological approach, followed by the presentation of the empirical results. We conclude by discussing our study’s theoretical contributions and practical implications, along with key study limitations and promising avenues for future research.
2. Theoretical background
2.1. Algorithmic control
In the IS and related literatures, control is commonly defined from a behavioural perspective, “as any attempt to align individual behaviors with organizational objectives” (Wiener et al., Citation2016, p. 742). In this view, control is thought to be dyadic in the sense that there is a controller (source of control) and a controllee (target of control) (Choudhury & Sabherwal, Citation2003; Kirsch, Citation1996). Since the seminal works by Henderson and Lee (Citation1992) and Kirsch (Citation1996, Citation1997) on the control of IS projects, control researchers have almost exclusively focused on the interaction between human controllers and controllees (e.g., an IS project manager exercising control over project team members); that is, existing IS control studies have primarily regarded the development and use of digital technology as an interesting research context that presents some unique control challenges (Wiener et al., Citation2016).
However, the role of algorithms and digital technology within managerial control processes has been largely neglected in prior control research (Cram & Wiener, Citation2020; Saunders et al., Citation2020; Schafheitle et al., Citation2020). This is in stark contrast to recent developments in practice, where organisations are increasingly leveraging technological advances to support managerial control (e.g., by acting as a monitoring tool for human controllers), or to fully automate control processes by acting as a proxy for human controllers (Cram & Wiener, Citation2020); see . The latter (automate) is also referred to as algorithmic control (AC), which is broadly defined as the managerial use of intelligent algorithms and advanced digital technology (e.g., mobile apps and sensors) as a means to align individual worker behaviours with organisational objectives (Cram & Wiener, Citation2020; Kellogg et al., Citation2020). To clarify how AC differs from traditional human-based control, we draw on the conceptual distinction among control configuration, enactment, and delivery (cf. Wiener et al., Citation2016). Specifically, in traditional control settings, it is a human controller who configures the portfolio of controls (i.e., selects relevant controls) and who enacts and delivers/communicates these controls to the controllee. In AC settings, the configuration of controls – including the design and implementation of respective algorithms – may still be performed by humans (although machine learning and other artificial intelligence [AI]-based approaches can be expected to play an increasingly important role); however, the enactment and delivery of controls is performed by algorithms and digital technology, respectively, without any substantive involvement by human controllers (again, see ). This implies that AC systems are characterised by a sequential combination of human agency (control configuration) and digital agency (control enactment and delivery). As such, AC systems can be seen as digital agents, which “increasingly perform versions of the kind of cognitive functions that are typically associated with humans” (Recker et al., Citation2021, p. 275; cf. Huysman, Citation2020); that is, even though AC systems “implement certain algorithms in a predictable manner and their behavior is determined by written code, they routinely make autonomous or semi-autonomous decisions” (Recker et al., Citation2021, p. 276). For example, with reference to Uber’s AC system, Möhlmann et al. (Citation2021) point out that “nowadays most [control-related] algorithms are machine-learning algorithms that continuously improve themselves according to defined goals” (p. 20).
Figure 1. Human-based control vs. algorithmic control (based on Cram & Wiener, Citation2020, p. 74).
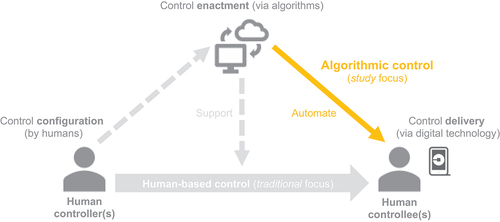
In prior literature, AC has been described as a “subset” of algorithmic management (Duggan et al., Citation2020; Griesbach et al., Citation2019; Möhlmann et al., Citation2021). For instance, Möhlmann et al. (Citation2021) conceptualise algorithmic management in terms of two dimensions: algorithmic matching and AC. A prominent example is the ride-hailing firm Uber, which relies on varied algorithms along with a driver app for customer matching and dynamic pricing (algorithmic matching), as well as for screening/vetting its freelance drivers and guiding their behaviour (AC), thereby minimising the need for costly and time-consuming interactions between Uber managers and drivers (Rosenblat & Stark, Citation2016). In the following section, we review and summarise key insights gained from existing literature on AC in the gig platform economy, where corresponding control practices are already commonplace (e.g., Duggan et al., Citation2020; Möhlmann et al., Citation2021).
2.2. Algorithmic control in the gig platform economy
While the primary orientation of our research pertains to AC in general (in order to inform our understanding of how workers perceive, judge, and react to corresponding control practices), we study the phenomenon within the context of platform-based gig work. In this context, the IS and related literatures offer initial insights into AC practices and thus help in contextualising and conceptualising the issues at hand. Most notably, while earlier IS control research tended to view control systems (e.g., in IS projects) as “closed systems with predefined resources” (Wiener et al., Citation2016, p. 768), the emergence of the platform economy has drawn increasing research attention to control issues such as the openness of digital platforms (e.g., Benlian et al., Citation2015; Eaton et al., Citation2015; Ghazawneh & Henfridsson, Citation2013) and the related exercise of input control in platform ecosystems (e.g., Croitor & Benlian, Citation2019; Thies et al., Citation2018; Tiwana, Citation2015). This changing view of control systems (closed vs. open) is also reflected in existing research on AC in the specific context of the gig economy, which in essence points to two basic forms of AC. On the one hand, gig companies use AC to control who is allowed to commence, or to continue, working for a given company, which we refer to as gatekeeping AC. More precisely, this form of AC is used to select the workers who are permitted on a gig platform, as well as to continuously monitor workers and deselect those who no longer should be allowed to work on the platform (e.g., Duggan et al., Citation2020; Griesbach et al., Citation2019; Kellogg et al., Citation2020). For example, before accepting a new driver, Uber and other ride-hailing firms rely on their app-based AC system to request formal documentation from each applicant (e.g., proof of residency, vehicle insurance), check the candidate and her/his car against a list of formal requirements, and conduct background checks (e.g., Lee et al., Citation2015). In some locations, Uber has introduced a real-time ID check that asks drivers to take a “live” photograph of themselves, which is then automatically compared against their profile picture. If the photo does not match, the account is temporarily suspended (Gray & Suri, Citation2019). Further, Uber has started running regular background checks in an effort to “ensure drivers continue to meet [firm] standards on an ongoing basis, long after they take their first trip” (Khosrowshahi, Citation2018). As well, Uber invested in a digital technology that “can identify new criminal offenses via public records or pending [driving under the influence] charges as they happen” (O’Brien, Citation2018).
On the other hand, gig companies use AC to control how workers conduct their daily work (Möhlmann et al., Citation2021), referred to as guiding AC. Corresponding controls often take the form of worker ratings and rankings, quantity of jobs completed, and response speed (Kellogg et al., Citation2020; Rosenblat & Stark, Citation2016). For example, Uber drivers are rated by passengers on a five-point scale after each trip. The ratings are averaged over the most recent 500 trips (Chan & Humphreys, Citation2018) and the average rating arguably constitutes a driver’s “most significant performance metric” (Rosenblat & Stark, Citation2016, p. 3772). Another example is Uber’s guaranteed fare programme, where drivers can earn an hourly rate during set time periods, provided they are available for a minimum period of time, accept a minimum proportion of ride requests, and complete a minimum number of trips (Kessler, Citation2016). Further, gig companies send regular, algorithm-initiated messages to workers, including personalised feedback and reports on worker-specific issues (Kellogg et al., Citation2020; Rosenblat & Stark, Citation2016). The feedback is often framed as a recommendation on how workers can improve their ratings and includes suggestions such as dressing professionally and providing free bottled water (e.g., Chan & Humphreys, Citation2018). For instance, Uber drivers may receive a message such as the following: “Riders give the best ratings to drivers who [go] above and beyond to make the experience special, such as opening doors for riders when possible” (Rosenblat & Stark, Citation2016, p. 3776). A brief definition along with illustrative examples for each of the two basic AC forms is provided in .
Table 1. Basic forms of algorithmic control (AC).
Beyond the two basic AC forms, the existing literature provides several additional insights. First, as highlighted by Kellogg et al. (Citation2020), prior studies “have primarily focused on the efficiency and organizational goal attainment made possible by the use of algorithmic systems, but have largely ignored the topic of how employers’ use of algorithms may negatively affect workers” (p. 384). Here, the few studies adopting, or at least considering, the perspective of individual workers show a strong tendency towards portraying AC systems as a universally “bad thing” (e.g., Möhlmann & Henfridsson, Citation2019; Rosenblat & Stark, Citation2016). For example, studying the AC practices used by Uber, Möhlmann and Henfridsson (Citation2019) identify three areas of consistent driver complaints about working “for” algorithms, namely: constant surveillance, little transparency, and dehumanisation. These complaints are largely in keeping with worker concerns identified in other studies (e.g., Curchod et al., Citation2020; Duggan et al., Citation2020; Wood et al., Citation2019). Second, while extant studies find that workers generally value the autonomy and flexibility of AC-enabled gig work, these studies also suggest that gig platforms’ use of AC produces information and power asymmetries that can be frustrating to workers (e.g., Griesbach et al., Citation2019; Lee et al., Citation2015; Rosenblat & Stark, Citation2016; Wood et al., Citation2019). As a consequence, some gig workers seem to question the legitimacy of AC practices, prompting them to resist or violate such practices (e.g., Curchod et al., Citation2020; Kellogg et al., Citation2020; Möhlmann et al., Citation2021), “with the potential to cause real harm to their companies” (Möhlmann & Henfridsson, Citation2019). Third, studies point to workers’ continuance intention and their workaround use as two key practical problems for many gig companies. For example, studying the inherent tensions between gig work and AC systems, Möhlmann and Zalmanson (Citation2017) identify several strategies that ride-hailing drivers use to regain a degree of “control” in their day-to-day work, such as resisting the system (e.g., deactivating GPS), switching the system (e.g., from Uber to Lyft), and gaming the system by using workarounds (e.g., cancelling rides to avoid negative customer ratings) (cf. Möhlmann et al., Citation2021). In this regard, Lee et al. (Citation2015) point to the important role that the transparency of AC systems plays in the use of workarounds, as well as in the potential improvement of “some drivers’ ambivalent feelings toward the companies” (p. 7). Moreover, it has been noted that gig firms such as Uber increasingly struggle with low retention rates (e.g., Anderson, Citation2016; Hall & Krueger, Citation2015; Scheiber, Citation2017). For instance, a study by Hall and Krueger (Citation2015) revealed that almost half of all new Uber drivers stop working for the company within their first year. Offering a potential explanation for these low driver retention rates, the study authors argue that “Uber provides a bridge for many who are seeking another position in the labor market” (p. 16). An alternative explanation is that some drivers perceive Uber’s tight AC approach (Constantiou et al., Citation2017) to contradict the firm’s key promise of “flexible employment” (Rosenblat & Stark, Citation2016), raising legitimacy issues among Uber drivers. We expand on the link between AC and (micro-level) legitimacy in the following section.
2.3. Algorithmic control and micro-level legitimacy
Broadly, legitimacy refers to “a generalized perception or assumption that the actions of an entity are desirable, proper, or appropriate within some socially constructed systems of norms, values, beliefs” (Suchman, Citation1995, p. 574). Recent work on the topic (e.g., Bitektine & Haack, Citation2015; Suddaby et al., Citation2017) has framed legitimacy as a multi-level concept, integrating past conceptualisations that primarily considered macro-level legitimacy issues. While the macro-level view posits that an organisation as a whole can be collectively viewed as legitimate by stakeholders (Brenner & Ambos, Citation2013; Dowling & Pfeffer, Citation1975), the micro-level view posits that organisational legitimacy stems from individual stakeholders’ perceptions of lower-level structures, such as manager behaviours or technology innovations (e.g., AC use) (Bogusz et al., Citation2019; Deephouse & Suchman, Citation2008). Although prior literature has highlighted the importance of understanding micro-level aspects of legitimacy, there remains a relative paucity of research in the area (Bitektine & Haack, Citation2015; Bitektine et al., Citation2020).Footnote1
Approaching legitimacy from the “evaluator’s perspective – that is, […] not as a property or an asset owned by an organization but as a judgment” (p. 50), Bitektine and Haack (Citation2015) propose a three-step process model of micro-level legitimacy, which provides an important theoretical foundation for our study. The first step in their model, perception, is based on the observations that individuals make regarding organisational behaviours (e.g., the AC practices used by a given organisation). The second step, judgment, consists of a worker’s evaluation of each behaviour in light of their own set of norms, values, and beliefs. In this regard, extant research recognises that the “criteria” that workers use when judging the legitimacy of organisational (control) practices are highly dependent on the work context under study (Bijlsma-Frankema & Costa, Citation2010; Lamin & Zaheer, Citation2012; Ruef & Scott, Citation1998). The third step, reaction, consists of the worker’s response, stemming from her/his legitimacy judgment in the previous step. Such reactions could serve to either reinforce or contradict the status quo (Brenner & Ambos, Citation2013; Schnedler & Vadovic, Citation2011). Here, our study focuses on two key categories of worker reactions put forward in earlier research: their continuance intention and workaround use (e.g., Hall & Krueger, Citation2015; Lee et al., Citation2015; Möhlmann & Zalmanson, Citation2017).
As indicated above, the conceptualisation of micro-level legitimacy largely depends on the nature of the relevant organisational behaviour and work context (e.g., Bijlsma-Frankema & Costa, Citation2010; Suchman, Citation1995). Given our study’s focus on the use of AC in the specific context of gig work, we draw on prior work to conceptualise micro-level legitimacy in terms of three dimensions that clearly apply within the context of platform-based gig work: autonomy, fairness, and privacy (e.g., Deng et al., Citation2016; Goldbach et al., Citation2018; Wiener et al., Citation2020). introduces the three dimensions and gives an illustrative example for each of them. While we acknowledge that other dimensions might contribute to gig workers’ legitimacy judgements as well, we believe that these three dimensions are the most relevant ones in the context of our study (cf. Deng et al., Citation2016). This is in keeping with Bijlsma-Frankema and Costa (Citation2010) who argue that only a few sources of legitimacy tend to be the most prominent within a particular work context.
Table 2. Dimensions of micro-level legitimacy judgements in relation to algorithmic control (AC).
In summary, this section has established the conceptual and theoretical foundation for our study by deriving two predominant forms of AC from prior literature, and by introducing a micro-level view of legitimacy along with a three-dimensional conceptualisation of this construct. In the next section, we build on this foundation to present our research model and hypotheses.
3. Research model and hypotheses
Our research model focuses on the antecedents and consequences of micro-level legitimacy judgements in relation to AC in the specific context of platform-based gig work. In particular, we theorise on the links between workers’ perceptions of two basic AC forms (gatekeeping vs. guiding) and their legitimacy judgements, and how such judgements relate to critical behavioural reactions, namely: continuance intention and workaround use (cf. Bitektine & Haack, Citation2015). In the following, we expound upon the posited relationships shown in . When doing so, we utilise Uber as an illustration of one prominent gig firm whose workers are exposed to AC.
3.1. Algorithmic control perceptions & micro-level legitimacy judgements
Taking into account the unique characteristics of different AC forms, we argue that gig workers’ perceptions of gatekeeping and guiding AC can be expected to vary considerably for at least two reasons. First, we argue that the control logic underlying gatekeeping AC is fundamentally different from the logic that underlies guiding AC: while the former is used to determine who is allowed to participate (i.e., to commence, or to continue, working for a given gig company), the latter is used to guide gig worker behaviour by overseeing and providing feedback on how they conduct their daily work. This key difference in underlying control logic can be expected to have a notable effect on how gig workers perceive the two AC forms and judge their legitimacy. Specifically, given its predominantly coercive logic (Adler & Borys, Citation1996) and potentially severe consequences (e.g., Kellogg et al., Citation2020), gatekeeping AC is likely to be perceived as a constant threat to “staying in the game” by gig workers, leading to a “situation comparable to a permanent probationary period” (Duggan et al., Citation2020, p. 126). For example, at Uber, gatekeeping AC is used to ensure workers’ continual adherence to firm standards regarding driver identity (real-time ID check), legal status (e.g., absence of driving convictions), and vehicle characteristics (e.g., model and age) (e.g., Gray & Suri, Citation2019; Uber, Citation2020). If an Uber driver is found to violate any of those controls, her/his driver account will be automatically deactivated, referred to as “algorithmic replacement” (Kellogg et al., Citation2020, p. 380).
Second, while existing studies highlight that AC in general is characterised by low transparency (Kellogg et al., Citation2020; Möhlmann & Zalmanson, Citation2017), we argue that the level of transparency tends to differ noticeably across AC forms (cf. Gal et al., Citation2020; Goad & Gal, Citation2018), leading to distinct effects on gig workers’ legitimacy judgements (e.g., Long et al., Citation2011). For example, Uber drivers perceive some of the enacted gatekeeping controls, such as the algorithm-based background and identity checks, to be an invasion of their privacy, since it is not entirely clear to drivers when and how the checks are conducted, as well as what data are collected (O’Brien & Yurieff, Citation2017; cf. Gal et al., Citation2020). Given the coercive, continuous, and largely opaque nature of gatekeeping AC, this form of AC can be expected to be judged by workers as unfair and as constraining their work autonomy and violating their privacy expectations, thus resulting in a decline in workers’ legitimacy judgements. We therefore suggest:
H1: Greater perceptions of gatekeeping AC are negatively related to gig workers’ legitimacy judgements.
In contrast, we argue that the use of guiding AC has the capacity to help elevate gig workers’ legitimacy judgements through the provision of clear and instantaneous performance feedback, including behavioural suggestions on how to improve (e.g., Gal et al., Citation2020; Kellogg et al., Citation2020). For example, Uber drivers can earn badges for achievements, such as “neat and tidy”, “excellent service”, and “great amenities” (Uber, Citation2019b). In an interview with the New York Times, a ride-hailing driver expressed his pride in the badges he had earned and highlighted the important feedback role that they fulfill because, “it tells me where I’m at” (Scheiber, Citation2017). Moreover, the app shows drivers the number of trips they have completed in the current week, the money they have earned, and the time they have spent logged on. Relatedly, past research suggests that gig workers do not view the guidance provided by the app as contributing to a loss in autonomy. For instance, Lee et al. (Citation2015) find that “ridesharing drivers desired little (or did not feel entitled to) direct control over the assignment algorithm, for example, specifying pick-up locations or being able to see and choose from all requests” (p. 8). As well, driver ratings and other performance metrics (e.g., ride acceptance rates) are communicated clearly and are thus well known among drivers. In this regard, it has been argued that automated controls adhering to a generally accepted set of rules increase transparency, leading to perceptions of fairness among controllees (e.g., Hansen & Flyverbom, Citation2015; Möhlmann & Zalmanson, Citation2017). For instance, when drivers receive a ride request through the Uber app, they are well aware that they have around 15 seconds to accept or reject it (Rosenblat & Stark, Citation2016; Uber, Citation2019a). On a related note, while the Uber driver app collects detailed data on driver behaviours for control purposes (Cram & Wiener, Citation2020), Uber openly shares information on what and how data are collected on its website (e.g., Beinstein & Sumers, Citation2016), which arguably helps mitigate driver concerns around privacy. On this basis, we hypothesise:
H2: Greater perceptions of guiding AC are positively related to gig workers’ legitimacy judgements.
3.2. Micro-level legitimacy judgements & behavioural reactions
Two particular worker reactions are of direct importance within a gig economy context. The first is the extent that an individual intends to continue working with a given platform. This is of concern to many gig companies because of the high turnover rates and the expense to recruit new workers (e.g., Anderson, Citation2016; Hall & Krueger, Citation2015; Scheiber, Citation2017). The second is the extent that a gig worker will comply with the enacted controls. Where workers view AC practices as illegitimate, they may engage in workarounds, which can lead to process inefficiencies, poor service, and regulatory violations (e.g., Laumer et al., Citation2017; Woltjer, Citation2017). We address each of these behavioural reactions below.
Goldbach et al. (Citation2018) define workers’ continuance intention as their behavioural intention to remain part of a platform ecosystem and offer their services on that platform. In this regard, the importance that gig workers place on the autonomy associated with securing flexible work arrangements corresponds to the preferences of independent contractors (Hall & Krueger, Citation2015). Prior literature also establishes a range of benefits that result from worker judgements of fairness. More specifically, from a continuance perspective, workers who believe they will be treated fairly are able to more clearly anticipate the long-term benefits of remaining with an organisation (Cropanzano et al., Citation2007). This is supported by earlier studies that find a positive link between fairness and organisational commitment (Folger & Konovsky, Citation1989) and a negative link between fairness and turnover intentions (Alexander & Ruderman, Citation1987; Chalykoff & Kochan, Citation1989). In a similar vein, prior research finds that organisational processes (including managerial control processes), judged by workers as fair, contribute to the building of trust and commitment, and eventually lead to voluntary cooperation (Cropanzano et al., Citation2007). As well, in regard to privacy, gig workers are often well aware of firms’ surveillance capabilities but seem to judge them differently. In cases where workers judge that their privacy has been infringed upon by an organisation, existing research suggests that lower levels of organisational commitment and increased turnover will result (Hodson et al., Citation1999; Smith & Tabak, Citation2009; Tabak & Smith, Citation2005). Also, prior studies find that constant tracking and close monitoring can lead to feelings of anxiety and tension (e.g., Chalykoff & Kochan, Citation1989; Lee et al., Citation2015; Möhlmann & Zalmanson, Citation2017), or may be judged by workers as an indication of a lack of trust (e.g., Lind & Tyler, Citation1988; Smith & Tabak, Citation2009).
Within the Uber context, the autonomy that ride-hailing drivers have in setting their own schedules represents an important retention mechanism (e.g., Lee et al., Citation2015; Möhlmann et al., Citation2021; Rosenblat & Stark, Citation2016). For example, in a 2015 survey commissioned by Uber, 85% of respondents agreed that autonomy was a major motivator for driving for the company; and 42% of women and 29% of men stated that a flexible schedule was mandatory for them, due to family, education, health, and other factors (Hall & Krueger, Citation2015). Similarly, Möhlmann et al. (Citation2021) find that the opportunity to work autonomously is important to Uber drivers who “enjoyed not having a supervisor looking over their shoulder, and expressed their satisfaction about working in a non-hierarchical environment” (p. 23). Still, tensions that are traditionally associated with worker turnover exist between Uber and some drivers, including judgements that the firm acts unfairly, for example, in regard to driver deactivation (Möhlmann & Zalmanson, Citation2017; Rosenblat & Stark, Citation2016). Based on these arguments, we suggest:
H3: Greater legitimacy judgements associated with AC are positively related to gig workers’ continuance intention.
The use of workarounds is of particular concern for organisations, as it represents a form of control violation and leads to managers being increasingly unaware of how tasks are actually being undertaken by workers (Woltjer, Citation2017). In the IS literature, workaround use is defined as “conscious adaptations of work activities that are not expected or specified to be changed in this manner” (Laumer et al., Citation2017, p. 335). Here, prior research shows that where organisational and personal interests are aligned, inappropriate workarounds are less likely to be undertaken (Alter, Citation2014). However, even if interests are aligned, workers may engage in actions of resistance if the autonomy they are granted differs from what they had been promised (Oliver, Citation1991). To that end, Pollock (Citation2005) argues that workarounds will be employed as “resistance on behalf of users and the means by which they attempt to wrest control back from a technology or an institution” (p. 497). On the other hand, extant research suggests that where workers judge an organisational control to be legitimate, “they will accept it and comply with it” (Bijlsma-Frankema & Costa, Citation2010, p. 402). Similarly, workers who believe they are treated justly are more likely to comply with company policies and less likely to engage in counterproductive work behaviour (Cohen-Charash & Spector, Citation2001; Cropanzano et al., Citation2007; D’Arcy et al., Citation2014).
Given the views above, we anticipate that gig workers who judge enacted AC practices to be legitimate (in terms of autonomy, fairness, and privacy) will adhere to prescribed guidelines; however, where a disconnect exists between organisational and personal interests, workarounds will be increasingly likely to be undertaken. Here, with reference to the work by Beunza (Citation2019), Kellogg et al. (Citation2020) suggest that “when workers are directed by an algorithm that they perceive as unfair, this may undermine their moral compass and increase their willingness to engage in unethical behavior” (p. 374). In the Uber context, recently published driver perspectives offer examples of workarounds that drivers employ when AC practices are judged to be illegitimate. For instance, Rosenblat and Stark (Citation2016) note that some drivers “perceive that Uber favors the passenger in adjudications” and, in response, “resist Uber’s power of interpretation by tracking their trips with manual or electronic logs and dash-cams” (p. 3765). Other evidence suggests that some ride-hailing drivers attempt to protect their autonomy, as well as their privacy, by manipulating the algorithm via techniques such as “periodically turn[ing] on and off [their] driver application while at traffic signals, so that [they] did not get distant requests” (Lee et al., Citation2015, p. 5). On this basis, we propose:
H4: Greater legitimacy judgements associated with AC are negatively related to gig workers’ workaround use.
4. Research methodology
4.1. Empirical background
Although our study is broadly concerned with the use of AC within the gig platform economy, the hypotheses outlined above and empirical activities explained below focus on Uber as an illustrative example of an app-based online labour platform. In over 900 cities around the world, Uber has emerged as a ubiquitous provider of millions of ride-hailing trips each day and has the leading market share in each of its major operating regions (Yeo, Citation2021). Uber’s business model is based on the use of varied algorithmic processes to manage its operations (e.g., matching of demand and supply, dynamic pricing, payments), as well as to control who is allowed to work for the company (i.e., gatekeeping AC) and how workers conduct their daily work (i.e., guiding AC). Against this backdrop, Uber has been described as an “extreme case” of algorithmic management in general (Möhlmann et al., Citation2021) and as a prototypical example of a so-called “Franchiser” platform, which utilises tight control and high rivalry (Constantiou et al., Citation2017). This tight control is enacted via algorithms and delivered via a driver app, which is the primary point of contact between Uber and its drivers. (Please refer to for examples of gatekeeping and guiding AC mechanisms used by Uber.) From a driver perspective, Uber is commonly viewed as an appealing employer due to the flexibility of working hours (Hong et al., Citation2020); however, drivers have also expressed concerns pertaining to issues such as surveillance and isolation (Möhlmann & Henfridsson, Citation2019).
We chose to focus on Uber in this study for two main reasons. First, Uber has a reputation as the market leader in the global ride-hailing industry. Due to this influential position, we believe that studying Uber can offer valuable insights into how a successful firm in a competitive market is able to utilise AC within its business operations. Secondly, we chose to orient the study around Uber because of the firm’s nearly exclusive reliance on algorithms to manage its ride-hailing drivers (Möhlmann et al., Citation2021). As indicated above, Uber’s extensive use of algorithms applies not only to matching (i.e., pairing prospective riders with nearby drivers), but also to managerial control (i.e., any attempt to align driver behaviours with firm objectives). As such, the case of Uber and its fully automated AC system is relatively unique, especially when compared to past control research that either focuses exclusively on traditional, human-based control settings or on control situations where a human controller is supported by technology (e.g., provision of insights into controllee activities) (Cram & Wiener, Citation2020). As more organisations, including traditional ones (Mateescu & Nguyen, Citation2019), introduce increasingly sophisticated AC systems, it will become important to understand the worker-level and organisational implications of such systems; we aim to investigate Uber as an illustrative example of such circumstances. Even though the insights gained from studying Uber’s AC system are unlikely to generalise to all (gig) companies employing AC, we believe there are important elements that both academics and practitioners can learn from (Flyvbjerg, Citation2006; cf. Möhlmann et al., Citation2021).
4.2. Survey instrument and data collection
To test our research model and hypotheses, we developed a survey instrument consisting of two parts: (1) general work-related and personal information and (2) workers’ interactions with the Uber driver app and their experiences as Uber driver. Throughout the second part, we instructed respondents to focus on the algorithmic aspects of Uber’s control system (i.e., on their interactions with the driver app). All latent variables were measured reflectively with multiple items, and most measurement items were rated on seven-point Likert scales using “strongly disagree” and “strongly agree” anchors. Given the lack of existing measurement instruments for gatekeeping and guiding AC, we adapted established scales for input control (Croitor & Benlian, Citation2019) and behaviour/output control (Challagalla & Shervani, Citation1997; Weibel et al., Citation2016) to measure gatekeeping AC and guiding AC, respectively. To measure the three dimensions of micro-level legitimacy, we used existing instruments from prior studies and adapted them to the specific context of our study: autonomy judgment (Goldbach et al., Citation2018), fairness judgment (Wu, Citation2013), and privacy (invasion) judgment (Alge et al., Citation2006). We modelled gig workers’ legitimacy judgements as a three-dimensional second-order construct, using a reflective-formative (type II) specification (Hair et al., Citation2018). The main reason for this specification is that we view autonomy, fairness, and privacy judgements as sources, or drivers, of legitimacy judgements (Bijlsma-Frankema & Costa, Citation2010), as opposed to more concrete manifestations of this construct (MacKenzie et al., Citation2011; Wright et al., Citation2012). Modelling micro-level legitimacy judgements as a second-order construct also allowed us to reduce the number of structural relationships, “making [our] model more parsimonious, while increasing the bandwidth of content covered” (Hair et al., Citation2018, p. 38; cf. Polites et al., Citation2012; Wright et al., Citation2012). Finally, to assess workers’ continuance intention and workaround use, we adopted items from Goldbach et al. (Citation2018) and Laumer et al. (Citation2017). An overview of the construct items is given in Appendix A.
In order to account for alternative explanations, we added a total of nine control variables to our research model. Besides personal information (age, gender, and household income), control variables captured relevant work information: multi-homing (dummy variable for whether a driver works for both Uber and Lyft), main income and full-time driver (dummy variables serving as proxies for a driver’s level of dependency on her/his ride-hailing work), ride-hailing income (average monthly income an Uber driver is making from ride-hailing work), ride-hailing experience (measured as total number of trips), and overall job satisfaction. For instance, Thatcher et al. (Citation2002) show that job satisfaction affects workers’ turnover intention. Further, prior research on gig work indicates that “switching behavior [i.e., multi-homing] provides drivers with leverage against the platform by lowering the risks associated with a [platform] ban” (Möhlmann & Zalmanson, Citation2017, p. 12). As well, Laumer et al. (Citation2017) find that the prevalence of workarounds increases with lower levels of user satisfaction, and suggest that “age, gender, and system usage frequency [affect] the manifestation of workarounds” (p. 341).
We pretested our survey instrument with four experienced IS scholars and four senior PhD students, none of whom were part of the author team. The pretest resulted in minor adaptations in the survey flow and wording. To identify and recruit relevant survey participants and to disseminate our survey questionnaire, we used the services of a market research firm, Qualtrics Research Services (cf. Lowry & Moody, Citation2015; Posey et al., Citation2010). The use of online panels is advantageous due to the anonymity that it grants participants, as well as the broad spectrum of individuals that can be contacted (Lowry & Moody, Citation2015).
The survey was administered using Qualtrics’ online survey tool. Data were collected in April and May 2019. The survey questionnaire started with a qualifier question: “For which ride-hailing company, or companies, do you currently drive on a regular basis (i.e., at least once per week)?” All respondents were required to be active Uber drivers in the United States, and all survey questions were mandatory. Multiple attention checks (e.g., “Please select strongly disagree”) were included in the survey questionnaire to “filter out careless respondents” (Kung et al., Citation2018, p. 264) and responses submitted in less than the minimum expected time were automatically terminated. In total, 630 Uber drivers completed the online questionnaire. Upon completion of the data-collection process, we analysed our data sample in order to identify potential straight-liners and ensure the logical consistency of the collected responses (e.g., by checking each driver’s ride-hailing tenure and weekly working hours against the total number of ride-hailing trips reported). On this basis, we excluded nine data records, resulting in a final sample size of 621 Uber drivers.
The demographics of our data sample largely match with those of other studies on Uber (e.g., Cook et al., Citation2019; Hall & Krueger, Citation2015; Hong et al., Citation2020; Möhlmann & Zalmanson, Citation2017). For example, using Qualtrics data of 406 ride-hailing drivers in the United States, Hong et al. (Citation2020) report that drivers in their sample had a mean age of 36 years, an average tenure of 1.4 years, and an average annual household income of around $65,000 (with a maximum of more than $150,000), which is consistent with our sample demographics (see Table B1 in Appendix B). Further, they find that 47% of drivers held a college degree (61% in our sample) and 46% of them practiced multi-homing (37% in our sample). As well, while the Hong et al. (Citation2020) data sample includes a relatively large share of female ride-hailing drivers (46%), Cook et al. (Citation2019) show that about one third of Uber drivers are females, which matches with our sample (32%). Moreover, Hall and Krueger (Citation2015) find that the large majority of drivers work only part-time (see “main motive” and “hours per week” rows in Table B1). As such, we are confident that our data sample adequately represents the Uber driver population in the United States.
4.3. Assessment of measurement model
To transform our research model into a structural equation model, we used SmartPLS, Version 3.2.9 (Ringle et al., Citation2015). Compared to its covariance-based alternative (CB-SEM), partial least squares structural equation modelling (PLS-SEM) is an appropriate choice when the study focus lies on predicting key target constructs (e.g., Hair et al., Citation2011; Ringle et al., Citation2012), which is consistent with the “balanced structure” of our model (Hair et al., Citation2012, p. 421). Further, PLS-SEM can readily handle both reflective and formative constructs, including second-order constructs of a reflective-formative type (Hair et al., Citation2011; Ringle et al., Citation2012). Put differently, while PLS-SEM has limitations, we believe that our use of this method is justified, especially given the early stage of theory development, the balanced model structure and its limited complexity, and the presence of a higher-order, formatively measured latent variable (Hair et al., Citation2019).Footnote2 Since our research model includes a reflective-formative second-order construct (legitimacy) with antecedent latent variables (AC forms), we employed the two-stage approach (Hair et al., Citation2018). This approach draws on the repeated-indicators approach to obtain latent variable scores for the lower-order constructs (i.e., autonomy, fairness, and privacy judgements), and then uses these scores as manifest variables to measure the higher-order construct (i.e., micro-level legitimacy judgements). In both stages, we used the factor-weighting scheme and a maximum of 1,000 iterations to assess the measurement model, as recommended by Hair et al. (Citation2018; see also Hair et al., Citation2011).
Specifically, in the first stage, we evaluated the reliability and validity of the measurement model as follows. To ensure item reliability, we analysed the outer loadings of each item. (As noted above, all first-order constructs were measured reflectively with multiple items.) On this basis, we dropped two gatekeeping AC, one guiding AC, one autonomy, and one privacy item (see Appendix A). The outer loadings of all remaining items are above the threshold of 0.7 (Hair et al., Citation2017), with the exception of one autonomy item being slightly below the threshold (0.694). In terms of composite reliability (CR) and average variance extracted (AVE), all constructs exceed the suggested thresholds of 0.7 and 0.5, respectively, indicating construct reliability and convergent validity (Fornell & Larcker, Citation1981) (see also Table C1 in Appendix C). Further, the square root of each construct’s AVE was greater than the highest correlation with any other construct and the loadings of each construct’s assigned items were greater than cross loadings (ibid), establishing discriminant validity for all constructs (see Tables C1 and C2 in Appendix C). In addition, we examined the heterotrait-monotrait (HTMT) ratio of construct correlations, which is an alternative approach to assessing discriminant validity (Henseler et al., Citation2015). All HTMT values are below the threshold value of 0.9 (see Table C3 in Appendix C).
In the second stage, we re-evaluated our measurement model and were able to re-establish item reliability, construct reliability, as well as convergent and discriminant validity for all reflective constructs (i.e., gatekeeping and guiding AC, continuance intention, workaround use). We then assessed our formative construct (i.e., micro-level legitimacy judgements) in terms of potential collinearity issues and significance of outer weights and loadings (Hair et al., Citation2017). First, all (item-level) variance inflation factor (VIF) values are below the most conservative threshold of 3.3, suggesting that collinearity is unlikely to be an issue. Second, while the outer weight of one item was not significant (autonomy: β = 0.043, t = 0.921, ns), the outer loadings of all three items are significant (autonomy: β = 0.188, t = 2.083, p < 0.05; fairness: β = 0.902, t = 25.392, p < 0.001; privacy: β = 0.696, t = 10.490, p < 0.001). On this basis, and given the three items’ theoretical relevance (Bijlsma-Frankema & Costa, Citation2010; Wiener et al., Citation2020), we decided to retain all of them (Hair et al., Citation2017). In this regard, Hair et al. stress that “formative indicators should never be discarded simply on the basis of statistical outcomes” (p. 149). Moreover, to analyse the strength of construct intercorrelations, we conducted a full collinearity test (Kock & Lynn, Citation2012) by calculating the VIF values for all constructs and control variables included in our research model. Again, all VIF values are clearly below the most conservative threshold of 3.3 (ibid), with workaround use showing the highest VIF value (1.875). Finally, to mitigate the risk of common method bias (CMB), we incorporated several procedural remedies and undertook several statistical analyses and tests (Podsakoff et al., Citation2003). The results indicate that CMB is unlikely to be an issue in our study. Please refer to Appendix D for details.
5. Data analysis and results
5.1. Main analysis: structural model
In line with established guidelines, we used a bootstrap size of 5,000 subsamples (Hair et al., Citation2011) and followed a two-step process to assess our structural model (cf. Carte & Russell, Citation2003). We first analysed the effects of the control variables (step 1) before adding the main effects (step 2). The analysis and hypothesis test results are summarised in .2
H1 and H2 refer to the relationships between gig workers’ perceptions of gatekeeping AC (H1) and guiding AC (H2), on one hand, and workers’ legitimacy judgements, on the other hand. As hypothesised, gatekeeping AC is significantly and negatively related to micro-level legitimacy (β = −0.388, t = 7.268, p < 0.001), whereas guiding AC is significantly positively related to legitimacy (β = 0.482, t = 10.207, p < 0.001). Therefore, H1 and H2 are empirically supported. H3 and H4 pertain to the effects of gig workers’ legitimacy judgements on their continuance intention and workaround use, respectively. As anticipated, micro-level legitimacy judgements are found to have a significantly positive effect on continuance intention (β = 0.582, t = 15.739, p < 0.001) and a significantly negative effect on workaround use (β = −0.554, t = 10.976, p < 0.001), offering support for H3 and H4. Regarding these two hypotheses, we also performed some empirical tests to assess the likelihood of potential endogeneity/reverse causality issues. In particular, we reversed the relationships between gig workers’ legitimacy judgements and their continuance intention and workaround use and compared the resulting model to our original model by running Cohen’s path analysis, which is sensitive to causal directions (P. R. Cohen et al., Citation1993) and has been used as an effective test for detecting reverse causality issues in prior studies (e.g., Sattler et al., Citation2010; Sun & Zhang, Citation2006). The total squared errors (TSE) for the original and the reversed model are 0.244 and 0.316, respectively; that is, the TSE increased by 30% for the reversed model. Further, we calculated Cohen’s (Citation1988) d-value to assess the magnitude of TSE change, with values from 0.2, 0.5, and 0.8 signifying a small, medium and large change, respectively. The d-value for the TSE difference between the two models is 0.77, indicating that our original model is to be strongly preferred over the reversed model. We thus find additional empirical support for our theoretical arguments that workers’ legitimacy judgements increase their continuance intention (H3) and decrease their workaround use (H4).
Table 3. Hypothesis test results.
Moreover, some control variables included in our research model show significant effects (see , step 1): age is negatively related to workaround use, indicating that older gig workers are less likely to make use of workarounds; household income is positively related to all three dependent variables; and ride-hailing experience is found to be negatively linked to workaround use. As well, workers’ overall job satisfaction is positively associated with micro-level legitimacy judgements and continuance intention, and negatively associated with workaround use.
Overall, our research model explains roughly 55% of the variance in gig workers’ legitimacy judgements, as well as about 49% and 32% of the variance in their continuance intention and workaround use, respectively. In this regard, Hair et al. (Citation2017) note that “it is difficult to provide rules of thumb for acceptable R2 values as this depends on the model complexity and the research discipline” (p. 199). For example, an R2 value of 0.20 (20%) is often considered high in behavioural research, whereas in marketing research, R2 values of 0.25, 0.50, and 0.75 are considered weak, moderate, and substantial (Hair et al., Citation2017). As such, the explanatory power of our model can be described as moderate to high. Further, to assess the strength of the significant main effects, we calculated the effect size f2 as [R2included – R2excluded]/[1 – R2included] (J. Cohen, Citation1988), with f2-values from 0.02, 0.15, and 0.35 being regarded as a weak, moderate, and strong effect size (Chin et al., Citation2003). Three main effects are found to have a strong effect size: guiding AC on workers’ legitimacy judgements (f2 = 0.433), as well as legitimacy judgements on continuance intention (f2 = 0.560) and workaround use (f2 = 0.379). The other main effect (gatekeeping AC on legitimacy judgements) shows a moderate effect size (f2 = 0.316).
In sum, our main analysis results suggest that gatekeeping AC negatively affects gig workers’ legitimacy judgements, whereas guiding AC positively affects such judgements; and that micro-level legitimacy judgements increase workers’ continuance intention, while decreasing their use of workarounds. Given these results, it becomes important to analyse the role of micro-level legitimacy judgements in mediating the relationships between gig workers’ perceptions of the two AC forms and their behavioural reactions.
5.2. Additional analyses: mediation effects
In order to analyse the mediation effects of gig workers’ legitimacy judgements, we followed the process suggested by Hair et al. (Citation2017). More specifically, we first added direct connections between the two basic AC forms, on the one hand, and continuance intention and workaround use, on the other hand, to our research model. We then tested the significance of the direct effects and the indirect effects (via micro-level legitimacy judgements). The test results show that all four indirect effects are significant, since none of the 95% confidence intervals includes zero, whereas only three of the direct effects are significant (see below). Our results thus suggest that legitimacy judgements fully mediate the relationship between gatekeeping AC and continuance intention. Further, they indicate that legitimacy judgements partially mediate the links between gatekeeping AC and workaround use, as well as between guiding AC and both continuance intention and workaround use. In the latter case, the direct effect is positive, while the indirect effect is negative (i.e., the two effects point in opposite directions), indicating a competitive mediation effect of micro-level legitimacy judgements (Hair et al., Citation2017).
Table 4. Analysis of mediation effects.
6. Discussion and implications
Given the increasing reliance on algorithms to manage and control work, the overarching goal of this research was to further our understanding of how workers react to AC. To do so, we drew on existing work on AC in the context of the gig platform economy and more recent advances in the legitimacy literature to develop a research model that considers two predominant forms of AC (gatekeeping and guiding) alongside the notion of micro-level legitimacy judgements. Based on a large-scale survey with 621 Uber drivers, we find empirical support for the four hypotheses included in our model. In particular, our results point to opposing effects of basic AC forms: while gatekeeping AC is found to be negatively related to gig workers’ legitimacy judgements, guiding AC is found to be positively associated with legitimacy judgements. Further, micro-level legitimacy judgements are found to have a positive impact on workers’ continuance intention and a negative impact on their workaround use. In addition, an analysis of mediation effects highlights the central role of micro-level legitimacy judgements in the chain of relationships between workers’ perceptions of and their reactions to AC. visually summarises the main results of our study.
6.1. Theoretical contributions
An overarching contribution of our study lies in presenting theoretical arguments and empirical evidence that counter the generally negative rhetoric surrounding the adoption of AC systems in platform-based gig work (and beyond), thereby pointing to the need for a more balanced view of AC. As such, the study at hand represents a direct response to recent calls for more empirical work on AC systems in general (e.g., Gal et al., Citation2020), and especially to calls to “[IS] researchers trained in the sociotechnical tradition to step on board and fight the hype and fear narrative by offering empirically validated insights on why, when and how AI changes our work” (Huysman, Citation2020, p. 307). More specifically, our study contributes to the growing body of research on AC in two distinct ways. First, from a theoretical perspective, we draw on a recently proposed theoretical model from the organisational legitimacy literature (Bitektine & Haack, Citation2015) to introduce the concept of micro-level legitimacy to the AC literature and to theorise on how corresponding judgements mediate the relationships between gig workers’ perceptions of two basic AC forms (gatekeeping vs. guiding) and their behavioural reactions (in terms of continuance intention and workaround use). In doing so, our study addresses key shortcomings of prior studies, which tend to treat the links between control practices and behavioural outcomes as a black box (Kellogg et al., Citation2020; Wiener et al., Citation2016, Citation2019). Further, it also addresses the repeatedly highlighted need for adopting fresh theoretical perspectives that further our understanding of managerial control issues in general (e.g., Cardinal et al., Citation2017; Wiener et al., Citation2019), and the worker-level and organisational implications of AC systems in particular (e.g., Kellogg et al., Citation2020; Schafheitle et al., Citation2020). For example, Cardinal et al. (Citation2017) highlight that existing control research “appears to over rely on theories and approaches based on an understanding of organizations that dates back to the 1950s, 1960s, and 1970s, thereby limiting our ability to better understand organizations and advise managers today and in the future” (p. 560). Here, adopting a micro-level legitimacy perspective gives a fresh theoretical impetus towards “unblackboxing” the mechanisms through which (algorithmic) control practices trigger divergent behavioural reactions from individual workers.
Second, our study is among the first to examine the nature and manifold implications of AC systems, thereby shedding light on the still under-researched use of algorithms and advanced digital technology in managerial control processes (Cram & Wiener, Citation2020; Schafheitle et al., Citation2020). In particular, drawing on a concept-driven and theory-informed approach, the results of our study offer support for the importance of conceptually distinguishing between two predominant forms of AC and considering gig workers’ legitimacy judgements. Acting as a pivotal mediating mechanism, micro-level legitimacy judgements provide a distinct account that goes over and above alternative explanations (e.g., workers’ experience and job satisfaction) and thus contributes to a more nuanced understanding of how and why different AC forms influence critical worker behaviours such as turnover and non-compliance. More precisely, we find empirical evidence for the divergent effects of gatekeeping and guiding AC on gig workers’ legitimacy judgements, thereby extending prior research on platform-based control, which either focused on “guiding” control only (i.e., process and output control) or considered control practices at an aggregated level (Goldbach et al., Citation2018; Tiwana, Citation2015).
Regarding the characteristics of AC practices, extant studies highlight that AC systems in general are associated with low levels of transparency (e.g., Kellogg et al., Citation2020; Lee et al., Citation2015), and that this lack of transparency is particularly true for Uber’s AC system (e.g., Möhlmann et al., Citation2021). In contrast, the results of our study suggest that the degree of transparency perceived by gig workers varies across AC forms (i.e., between gatekeeping and guiding AC), leading to distinct legitimacy judgements and behavioural reactions. Again, this casts doubt on the generally negative rhetoric surrounding AC adoption by indicating that guiding AC holds at least the potential to provide gig workers with high levels of transparency on their work performance, and that AC is not perceived as a universally “bad thing” by workers. As such, the combination of gatekeeping and guiding AC can be expected to foster workers’ ambivalent feelings towards gig companies, which helps explain corresponding findings in earlier studies (Lee et al., Citation2015). In this regard, our study results suggest that gatekeeping AC is perceived as a constant threat by gig workers (Duggan et al., Citation2020), decreasing their legitimacy judgements and eventually their continuance intention, while increasing workers’ use of workarounds. On the other hand, our results also suggest that gig workers appreciate the clear direction and constant performance feedback associated with guiding AC, leading to an increase in workers’ legitimacy judgements, and ultimately, to an increase in their continuance intention and a decrease in their workaround use. Given these results, our study adds novel empirical insights to existing research on platform-based control, which has largely focused on explaining platform workers’ compliant behaviours, as manifested by high continuance intentions and high service/application quality (Goldbach et al., Citation2018); in contrast, as of yet, only scarce research attention has been paid to studying worker behaviours that violate or circumvent platform providers’ prescriptions (Curchod et al., Citation2020).
Last but not least, the results of our study offer empirical support for a recently theorised, multi-dimensional conceptualisation of micro-level legitimacy attuned to the gig economy work context (Wiener et al., Citation2020). Comprised of three dimensions (autonomy, fairness, and privacy), the adopted conceptualisation allowed us to capture the notion of micro-level legitimacy in a holistic and balanced way, thereby providing novel insights and a foundation for future insights at the intersection of AC, organisational legitimacy, and gig work. Our study thus also answers previous calls for an increasing research focus on micro-level issues associated with legitimacy judgements (e.g., Bitektine & Haack, Citation2015; Bitektine et al., Citation2020) and, in doing so, highlights the implications of AC use for workers, as well as the socially relevant implications for the future of work in general. Further, by zeroing in on three legitimacy judgment dimensions that pertain to the context of individual gig workers, our study adds new insights to Bitektine and Haack (Citation2015) theorising by shedding light on the specific “criteria” that are used by a particular stakeholder group when undertaking micro-level legitimacy judgements.
6.2. Practical implications
Our study also offers valuable and actionable insights on the more and less favourable features of AC systems, and thus on how to better balance the interests of platform operators and their workers through the effective design of platform-based work (Möhlmann et al., Citation2021). Specifically, the study results point to the effectiveness of guiding AC mechanisms in increasing gig workers’ continuance intention and decreasing their workaround use (via micro-level legitimacy judgements; see above). In contrast, undermining workers’ legitimacy judgements, the use of gatekeeping AC is found to be associated with detrimental worker behaviour and therefore appears to be problematic. As such, the results of our study can serve as an analytical toolkit for platform-based organisations that seek to implement AC systems, or provide them with a fresh impetus to rethink and/or “rebalance” their current systems (Möhlmann et al., Citation2021) in order to foster the sustainability and long-term health of their platform ecosystem. For example, at many gig firms, low worker retention rates represent a key challenge (e.g., Hall & Krueger, Citation2015). For these firms, it is thus imperative to understand what factors increase workers’ continuance intentions, while keeping their use of workarounds at an acceptably low level. Here, our three-dimensional conceptualisation of micro-level legitimacy judgements, alongside the theoretically derived and empirically validated relationships included in our research model, offer food for thought on how gig companies can improve the work design of their platforms whose success largely depends on both an effective AC approach and a happy workforce that judges this approach to be legitimate. For instance, our results imply that Uber should consider scaling back its use of gatekeeping AC mechanisms and making respective controls more transparent (e.g., disclosure of screening procedures and the exact criteria for automatic driver deactivation) in order to reduce the likelihood of unintended worker behaviours (e.g., workaround use). On the other hand, our results also suggest that guiding AC mechanisms can help elevate gig workers’ legitimacy judgements through the provision of clear and instantaneous performance feedback, including behavioural suggestions on how to improve, and thus play a critical role in eliciting goal-directed behaviours from workers.
6.3. Limitations & directions for future research
As with any research, our study is subject to several limitations that should be kept in mind when interpreting its results. First, examining micro-level legitimacy judgements in relation to AC, our study focuses on the perspective of gig workers and is cross-sectional in nature. The latter implies that we can only test associations; that is, our causal explanations are based on our theorising. Second, the focus our study is on the “extreme case” of Uber (Möhlmann et al., Citation2021) and our sample consists of Uber drivers based in the United States, which was a deliberate choice to mitigate potentially confounding effects of different gig platforms, as well as cultural and national differences. Third, we would like to acknowledge that our study views gig workers’ use of workarounds as a form of control violation (from a platform operator perspective), and thus as a negative and disruptive element (Laumer et al., Citation2017; Woltjer, Citation2017). However, prior literature has also pointed to the potential benefits of workarounds by describing them as “necessary activities in everyday life, […] creative acts, and […] sources of future improvements” (Alter, Citation2014, p. 1052). Fourth, our empirical investigation focused on two predominant forms of AC, which are widely used on gig economy platforms and pertain to formal control. Here, it should be acknowledged that gatekeeping AC was measured with only two items and that some guiding AC mechanisms (e.g., driver ratings) can feed into gatekeeping mechanisms (e.g., algorithmic replacement). In light of these limitations, several promising opportunities for future research emerge, as summarised in .
Table 5. Overview of promising opportunities for future research.
7. Conclusion
Our study represents a critical first step towards understanding how workers perceive, judge, and react to AC. Specifically, drawing on the IS and related literatures, as well as a large-scale survey with 621 Uber drivers, we unblackbox the chain of relationships between gig workers’ perceptions of two predominant forms of AC (gatekeeping and guiding) and their continuance intention and workaround use by offering empirical evidence for the central role of workers’ legitimacy judgements (conceptualised in terms of autonomy, fairness, privacy judgements) in mediating these relationships. Notably, uncovering opposing effects of gatekeeping and guiding AC, our results contrast prior work by showing that workers do not perceive AC as a universally “bad thing”. In conclusion, since AC-based work systems – such as the ones used by Uber and other gig firms – have been predicted to “become one of the most common ways of managing the American labor force” (Scheiber, Citation2017), advancing our understanding of the antecedents, nature, and consequences of workers’ legitimacy judgements in relation to different AC forms becomes of paramount importance. We thus hope that our study gives fresh impetus to future research and provides companies with actionable insight on how to design AC-based systems that are both effective (from an organisational viewpoint) and “legit” (from a worker viewpoint).
Additional information
Funding
Notes
1. In considering how IS scholars have approached legitimacy, it becomes apparent that relevant studies primarily incorporate legitimacy fundamentals as a perspective for evaluating worker perceptions related to new technologies (e.g., Avgerou, Citation2000; Kohli & Kettinger, Citation2004; Mehrizi et al., Citation2019).
2. We also analysed our research model, as well as the mediation effects of gig workers’ legitimacy judgements, with another analytical technique: hierarchical multiple regression and conditional process analysis (Hayes, Citation2018). The results are substantively consistent with those produced by PLS-SEM, demonstrating the robustness of our study results.
3. To measure social desirability, we used Strahan and Gerbasi (Citation1972) ten-item version of the original measurement instrument developed by Crowne and Marlowe (Citation1960). All items were rated on a true/false scale and scores were summed up to create an overall score (Addas & Pinsonneault, Citation2018).
References
- Addas, S., & Pinsonneault, A. (2018). E-mail interruptions and individual performance: Is there a silver lining. MIS Quarterly, 42(2), 381–405. https://doi.org/10.25300/MISQ/2018/13157
- Adler, P. S., & Borys, B. (1996). Two types of bureaucracy: Enabling and coercive. Administrative Science Quarterly, 41(1), 61–89. https://doi.org/10.2307/2393986
- Alexander, S., & Ruderman, M. (1987). The role of procedural and distributive justice in organizational behavior. Social Justice Research, 1(2), 177–198. https://doi.org/10.1007/BF01048015
- Alge, B. J., Ballinger, G. A., Tangirala, S., & Oakley, J. L. (2006). Information privacy in organizations: Empowering creative and extrarole performance. Journal of Applied Psychology, 91(1), 221–232. https://doi.org/10.1037/0021-9010.91.1.221
- Alter, S. (2014). Theory of workarounds. Communications of the Association for Information Systems, 34(Article 55), 1041–1066. https://doi.org/10.17705/1CAIS.03455
- Anderson, D. N. (2016). Wheels in the head: Ridesharing as monitored performance. Surveillance & Society, 14(2), 240–258. https://doi.org/10.24908/ss.v14i2.6018
- Avgerou, C. (2000). IT and organizational change: An institutionalist perspective. Information Technology & People, 14(4), 234–262. https://doi.org/10.1108/09593840010359464
- Bagozzi, R. P., Yi, Y., & Phillips, L. W. (1991). Assessing construct validity in organizational research. Administrative Science Quarterly, 36(3), 421–458. https://doi.org/10.2307/2393203
- Beinstein, A., & Sumers, T. (2016). How Uber Engineering increases safe driving with telematics. Uber Technologies Inc. https://eng.uber.com/telematics/
- Benlian, A. (2020). A daily field investigation of technology-driven stress spillovers from work to home. MIS Quarterly, 44(3), 1259–1300. https://doi.org/10.25300/MISQ/2020/14911/
- Benlian, A., Hilkert, D., & Hess, T. (2015). How open is this platform? The meaning and measurement of platform openness from the complementors’ perspective. Journal of Information Technology, 30(3), 209–228. https://doi.org/10.1057/jit.2015.6
- Berger, J., Ridgeway, C. L., Fisek, M. H., & Norman, R. Z. (1998). The legitimation and delegitimaction of power and prestige orders. American Sociological Review, 63(3), 379–405. https://doi.org/10.2307/2657555
- Beunza, D. (2019). Taking the floor: Models, morals, and management in a Wall Street trading room. Princeton University Press.
- Bijlsma-Frankema, K. M., & Costa, A. C. (2010). Consequences and antecedents of managerial and employee legitimacy interpretations of control: A natural open system approach. In S. B. Sitkin, L. B. Cardinal, & K. M. Bijlsma-Frankema (Eds.), Organizational control (pp. 396–433). Cambridge University Press.
- Bitektine, A., & Haack, P. (2015). The “macro” and the “micro” of legitimacy: Toward a multilevel theory of the legitimacy process. Academy of Management Review, 40(1), 49–75. https://doi.org/10.5465/amr.2013.0318
- Bitektine, A., Hill, K., Song, F., & Vandenberghe, C. (2020). Organizational legitimacy, reputation, and status: Insights from micro-level measurement. Academy of Management Discoveries, 6(1), 107–136. https://doi.org/10.5465/amd.2017.0007
- Bogusz, C. I., Teigland, R., & Vaast, E. (2019). Designed entrepreneurial legitimacy: The case of a Swedish crowdfunding platform. European Journal of Information Systems, 28(3), 318–335. https://doi.org/10.1080/0960085X.2018.1534039
- Brenner, B., & Ambos, B. (2013). A question of legitimacy? A dynamic perspective on multinational firm control. Organization Science, 24(3), 645–964. https://doi.org/10.1287/orsc.1120.0760
- Cameron, A.-F., & Webster, J. (2013). Multicommunicating: Juggling multiple conversations in the workplace. Information Systems Research, 24(2), 352–371. https://doi.org/10.1287/isre.1120.0446
- Cardinal, L. B., Kreutzer, M., & Miller, C. C. (2017). An aspirational view of organizational control research: Re-invigorating empirical work to better meet the challenges of 21st century organizations. Academy of Management Annals, 11(2), 559–592. https://doi.org/10.5465/annals.2014.0086
- Carte, T. A., & Russell, C. J. (2003). In pursuit of moderation: Nine common errors and their solutions. MIS Quarterly, 27(3), 479–501. https://doi.org/10.2307/30036541
- Challagalla, G. N., & Shervani, T. A. (1997). A measurement model of the dimensions and types of output and behavior control: An empirical test in a salesforce context. Journal of Business Research, 39(3), 159–172. https://doi.org/10.1016/S0148-2963(96)00195-6
- Chalykoff, J., & Kochan, T. A. (1989). Computer-aided monitoring: Its influence on employee job satisfaction and turnover. Personnel Psychology, 42(4), 807–834. https://doi.org/10.1111/j.1744-6570.1989.tb00676.x
- Chan, N. K., & Humphreys, L. (2018). Mediatization of social space and the case of Uber drivers. Media and Communication, 6(2), 29–38. https://doi.org/10.17645/mac.v6i2.1316
- Chin, W. W., Marcolin, B. L., & Newsted, P. R. (2003). A partial least squares latent variable modeling approach for measuring interaction effects: Results from a Monte Carlo simulation study and an electronic-mail emotion/adoption study. Information Systems Research, 14(2), 189–217. https://doi.org/10.1287/isre.14.2.189.16018
- Chin, W. W., Thatcher, J. B., & Wright, R. T. (2012). Assessing common method bias: Problems with the ULMC technique. MIS Quarterly, 36(3), 1003–1019. https://doi.org/10.2307/41703491
- Choudhury, V., & Sabherwal, R. (2003). Portfolios of control in outsourced software development projects. Information Systems Research, 14(3), 291–314. https://doi.org/10.1287/isre.14.3.291.16563
- Cohen, J. (1988). Statistical power analysis for the behavioral sciences (2nd ed.). Lawrence Erlbaum.
- Cohen, P. R., Carlson, A., Ballesteros, L., & Amant, R. S. (1993). Automating path analysis for building causal models from data. Proceedings of the 10th International Conference on Machine Learning,Amherst, MA, USA: Morgan Kaufmann Publishers Inc.
- Cohen-Charash, Y., & Spector, P. E. (2001). The role of justice in organizations: A meta-analysis. Organizational Behavior and Human Decision Processes, 86(2), 278–321. https://doi.org/10.1006/obhd.2001.2958
- Constantiou, I., Marton, A., & Tuunainen, V. K. (2017). Four models of sharing economy platforms. MIS Quarterly Executive, 16(4), 231–251. https://aisel.aisnet.org/misqe/vol16/iss4/3
- Cook, C., Diamond, R., Hall, J., List, J. A., & Oyer, P. (2019). The gender earnings gap in the gig economy: Evidence from over a million rideshare drivers,Stanford University https://web.stanford.edu/~diamondr/UberPayGap.pdf
- Cram, W. A., & Wiener, M. (2020). Technology-mediated control: Case examples and research directions for the future of organizational control. Communications of the Association for Information Systems, 46(Article4), 70–91. https://doi.org/10.17705/1CAIS.04604
- Croitor, E., & Benlian, A. (2019). Perceived input control on online platforms from the application developer perspective: Conceptualisation and scale development. Journal of Decision Systems, 28(1), 19–40. https://doi.org/10.1080/12460125.2019.1616977
- Cropanzano, R., Bowen, D. E., & Gilliland, S. W. (2007). The management of organizational justice. Academy of Management Perspectives, 21(4), 34–48. https://doi.org/10.5465/amp.2007.27895338
- Crowne, D. P., & Marlowe, D. (1960). A new scale of social desirability independent of psychopathology. Journal of Consulting Psychology, 24(4), 349–354. https://doi.org/10.1037/h0047358
- Curchod, C., Patriotta, G., Cohen, L., & Neysen, N. (2020). Working for an algorithm: Power asymmetries and agency in online work settings. Administrative Science Quarterly, 65(3), 644–676. https://doi.org/10.1177/0001839219867024
- D’Arcy, J., Gupta, A., Tarafdar, M., & Turel, O. (2014). Reflecting on the “dark side” of information technology use. Communications of the Association for Information Systems, 35(Article5), 109–118. https://doi.org/10.17705/1CAIS.03505
- D’Arcy, J., & Lowry, P. B. (2019). Cognitive‐affective drivers of employees’ daily compliance with information security policies: A multilevel, longitudinal study. Information Systems Journal, 29(1), 43–69. https://doi.org/10.1111/isj.12173
- De Groen, W. P., Maselli, I., & Fabo, B. (2016). The digital market for local services: A one-night stand for workers? An example from the on-demand economy. Centre for European Policy Studies (CEPS). https://www.ceps.eu/ceps-publications/digital-market-local-services-one-night-stand-workers-example-demand-economy/
- Deephouse, D. L., & Suchman, M. (2008). Legitimacy in organizational institutionalism. In R. Greenwood, C. Oliver, R. Suddaby, & K. Sahlin (Eds.), Handbook of organizational institutionalism (pp. 49–77). Sage.
- Deng, X., Joshi, K. D., & Galliers, R. D. (2016). The duality of empowerment and marginalization in microtask crowdsourcing: Giving voice to the less powerful through value sensitive design. MIS Quarterly, 40(2), 279–302. https://doi.org/10.25300/MISQ/2016/40.2.01
- Dowling, J., & Pfeffer, J. (1975). Organizational legitimacy: Social values and organizational behavior. Pacific Sociological Review, 18(1), 122–136. https://doi.org/10.2307/1388226
- Duggan, J., Sherman, U., Carbery, R., & McDonnell, A. (2020). Algorithmic management and app‐work in the gig economy: A research agenda for employment relations and HRM. Human Resource Management Journal, 30(1), 114–132. https://doi.org/10.1111/1748-8583.12258
- Eaton, B., Elaluf-Calderwood, S., Sorensen, C., & Yoo, Y. (2015). Distributed tuning of boundary resources: The case of Apple’s iOS service system. MIS Quarterly, 39(1), 217–243. https://doi.org/10.25300/MISQ/2015/39.1.10
- Fisher, C. D., & To, M. L. (2012). Using experience sampling methodology in organizational behavior. Journal of Organizational Behavior, 33(7), 865–877. https://doi.org/10.1002/job.1803
- Flyvbjerg, B. (2006). Five misunderstandings about case-study research. Qualitative Inquiry, 12(2), 219–245. https://doi.org/10.1177/1077800405284363
- Folger, R., & Konovsky, M. A. (1989). Effects of procedural and distributive justice on reactions to pay raise decisions. Academy of Management Journal, 32(1), 115–130. https://doi.org/10.5465/256422
- Fornell, C., & Larcker, D. F. (1981). Evaluating structural equation models with unobservable variables and measurement error. Journal of Marketing Research, 18(1), 39–50. https://doi.org/10.1177/002224378101800104
- French, J. R. P., & Raven, B. (1959). The bases of social power. In D. Cartwright (Ed.), Studies in social power (pp. 150–167). University of Michigan.
- Gal, U., Jensen, T. B., & Stein, M.-K. (2020). Breaking the vicious cycle of algorithmic management: A virtue ethics approach to people analytics. Information & Organization, 30(2), 100301. https://doi.org/10.1016/j.infoandorg.2020.100301
- Ghazawneh, A., & Henfridsson, O. (2013). Balancing platform control and external contribution in third‐party development: The boundary resources model. Information Systems Journal, 23(2), 173–192. https://doi.org/10.1111/j.1365-2575.2012.00406.x
- Gill, M. J. (2019). The significance of suffering in organizations: Understanding variation in workers’ responses to multiple modes of control. Academy of Management Review, 44(2), 377–404. https://doi.org/10.5465/amr.2016.0378
- Goad, D., & Gal, U. (2018). Understanding the impact of transparency on algorithmic decision making legitimacy. In U. Schultze, M. Aanestad, M. Mähring, C. Østerlund, & K. Riemer (Eds.), Living with monsters? Social implications of algorithmic phenomena, hybrid agency, and the performativity of technology (pp. 64–79). Springer.
- Goldbach, T., Benlian, A., & Buxmann, P. (2018). Differential effects of formal and self-control in mobile platform ecosystems: Multi-method findings on third-party developers’ continuance intentions and application quality. Information & Management, 55(3), 271–284. https://doi.org/10.1016/j.im.2017.07.003
- Gray, M. L., & Suri, S. (2019). Ghost work: How to stop silicon valley from building a new global underclass. Houghton Mifflin Harcourt.
- Griesbach, K., Reich, A., Elliott-Negri, L., & Milkman, R. (2019). Algorithmic control in platform food delivery work. Socius: Sociological Research for a Dynamic World, 5, 1–15. https://doi.org/10.1177/2378023119870041
- Hair, J. F., Hult, G. T. M., Ringle, C. M., & Sarstedt, M. (2017). A primer on partial least squares structural equation modeling (PLS-SEM) (2nd ed.). Sage.
- Hair, J. F., Ringle, C. M., & Sarstedt, M. (2011). PLS-SEM: Indeed a silver bullet. Journal of Marketing Theory & Practice, 19(2), 139–151. https://doi.org/10.2753/MTP1069-6679190202
- Hair, J. F., Risher, J., Sarstedt, M., & Ringle, C. M. (2019). When to use and how to report the results of PLS-SEM. European Business Review, 31(1), 2–24. https://doi.org/10.1108/EBR-11-2018-0203
- Hair, J. F., Sarstedt, M., Ringle, C. M., & Gudergan, S. P. (2018). Advanced issues in partial least squares structural equation modeling. Sage.
- Hair, J. F., Sarstedt, M., Ringle, C. M., & Mena, J. A. (2012). An assessment of the use of partial least squares structural equation modeling in marketing research. Journal of the Academy of Marketing Science, 40(3), 414–433. https://doi.org/10.1007/s11747-011-0261-6
- Hall, J., & Krueger, A. B. (2015). An analysis of the labor market for Uber’s driver-partners in the United States. Uber Technologies Inc. https://s3.amazonaws.com/uber-static/comms/PDF/Uber_Driver-Partners_Hall_Kreuger_2015.pdf
- Hansen, H. K., & Flyverbom, M. (2015). The politics of transparency and the calibration of knowledge in the digital age. Organization, 22(6), 872–889. https://doi.org/10.1177/1350508414522315
- Hayes, A. F. (2018). Introduction to mediation, moderation, and conditional process analysis: A regression-based approach. Guilford Press.
- Henderson, J. C., & Lee, S. (1992). Managing I/S design teams: A control theories perspective. Management Science, 38(6), 757–777. https://doi.org/10.1287/mnsc.38.6.757
- Henseler, J., Ringle, C. M., & Sarstedt, M. (2015). A new criterion for assessing discriminant validity in variance-based structural equation modeling. Journal of the Academy of Marketing Science, 43(1), 115–135. https://doi.org/10.1007/s11747-014-0403-8
- Hodson, T. J., Englander, F., & Englander, V. (1999). Ethical, legal and economic aspects of employer monitoring of employee electronic mail. Journal of Business Ethics, 19(1), 99–108. https://doi.org/10.1023/A:1006110324652
- Hofstede, G. (1980). Culture’s consequences: International differences in work-related values. Sage.
- Hong, S. J., Bauer, J. M., Lee, K., & Granados, N. F. (2020). Drivers of supplier participation in ride-hailing platforms. Journal of Management Information Systems, 37(3), 602–630. https://doi.org/10.1080/07421222.2020.1790177
- Huysman, M. (2020). Information systems research on artificial intelligence and work: A commentary on ‘Robo-Apocalypse cancelled? Reframing the automation and future of work debate’. Journal of Information Technology, 35(4), 307–309. https://doi.org/10.1177/0268396220926511
- Jabagi, N., Croteau, A.-M., Audebrand, L. K., & Marsan, J. (2019). Gig-workers’ motivation: Thinking beyond carrots and sticks. Journal of Managerial Psychology, 34(4), 192–213. https://doi.org/10.1108/JMP-06-2018-0255
- Kellogg, K. C., Valentine, M., & Christin, A. (2020). Algorithms at work: The new contested terrain of control. Academy of Management Annals, 14(1), 366–410. https://doi.org/10.5465/annals.2018.0174
- Kessler, S. (2016). How Uber manages drivers without technically managing drivers,Fast Company. https://www.fastcompany.com/3062622/how-ubers-app-manages-drivers-withouttechnically-managing-drivers
- Khosrowshahi, D. (2018). Getting serious about safety. Uber Technologies Inc. https://www.uber.com/newsroom/getting-serious-safety/
- Kirsch, L. J. (1996). The management of complex tasks in organizations: Controlling the systems development process. Organization Science, 7(1), 1–21. https://doi.org/10.1287/orsc.7.1.1
- Kirsch, L. J. (1997). Portfolios of control modes and IS project management. Information Systems Research, 8(3), 215–239. https://doi.org/10.1287/isre.8.3.215
- Kock, N. (2015). Common method bias in PLS-SEM: A full collinearity assessment approach. International Journal of e-Collaboration, 11(4), 1–10. https://doi.org/10.4018/ijec.2015100101
- Kock, N., & Lynn, G. S. (2012). Lateral collinearity and misleading results in variance-based SEM: An illustration and recommendations. Journal of the Association for Information Systems, 13(7), 546–580. https://doi.org/10.17705/1jais.00302
- Kohli, R., & Kettinger, W. J. (2004). Informating the clan: Controlling physicians’ costs and outcomes. MIS Quarterly, 28(3), 363–394. https://doi.org/10.2307/25148644
- Kung, F. Y. H., Kwok, N., & Brown, D. J. (2018). Are attention check questions a threat to scale validity? Applied Psychology: An International Review, 67(2), 264–283. https://doi.org/10.1111/apps.12108
- Lamin, A., & Zaheer, S. (2012). Wall Street vs. Main Street: Firm strategies for defending legitimacy and their impact on different stakeholders. Organization Science, 23(1), 47–66. https://doi.org/10.1287/orsc.1100.0631
- Lammers, J., Galinsky, A. D., Gordijn, E. H., & Otten, S. (2008). Illegitimacy moderates the effects of power on approach. Psychological Science, 19(6), 558–564. https://doi.org/10.1111/j.1467-9280.2008.02123.x
- Laumer, S., Maier, C., & Weitzel, T. (2017). Information quality, user satisfaction, and the manifestation of workarounds: A qualitative and quantitative study of enterprise content management system users. European Journal of Information Systems, 26(4), 333–360. https://doi.org/10.1057/s41303-016-0029-7
- Lee, M. K., Kusbit, D., Metsky, E., & Dabbish, L. (2015). Working with machines: The impact of algorithmic and data-driven management on human workers. Proceedings of the 33rd ACM Conference on Human Factors in Computing Systems,Seoul, South Korea: Association for Computing Machinery (ACM).
- Lind, E. A., & Tyler, T. R. (1988). The social psychology of procedural justice. Plenum Press.
- Long, C. P., Bendersky, C., & Morrill, C. (2011). Fairness monitoring: Linking managerial controls and fairness judgements in organizations. Academy of Management Journal, 54(5), 1045–1068. https://doi.org/10.5465/amj.2011.0008
- Lowry, P. B., & Moody, G. D. (2015). Proposing the control-reactance compliance model (CRCM) to explain opposing motivations to comply with organisational information security policies. Information Systems Journal, 25(5), 433–463. https://doi.org/10.1111/isj.12043
- Lowry, P. B., Posey, C., Bennett, R. J., & Roberts, T. L. (2015). Leveraging fairness and reactance theories to deter reactive computer abuse following enhanced organisational information security policies: An empirical study of the influence of counterfactual reasoning and organisational trust. Information Systems Journal, 25(3), 193–222. https://doi.org/10.1111/isj.12063
- MacKenzie, S. B., Podsakoff, P. M., & Podsakoff, N. P. (2011). Construct measurement and validation procedures in MIS and behavioral research: Integrating new and existing techniques. MIS Quarterly, 35(2), 293–334. https://doi.org/10.2307/23044045
- Mateescu, A., & Nguyen, A. (2019). Explainer: Algorithmic management in the workplace. Data & Society Research Institute. https://datasociety.net/wp-content/uploads/2019/02/DS_Algorithmic_Management_Explainer.pdf
- Mehrizi, M. H. R., Modol, J. R., & Nezhad, M. Z. (2019). Intensifying to cease: Unpacking the process of information systems discontinuance. MIS Quarterly, 43(1), 141–165. https://doi.org/10.25300/MISQ/2019/13717
- Möhlmann, M., & Henfridsson, O. (2019). What people hate about being managed by algorithms, according to a study of Uber drivers. Harvard Business Review (HBR). https://hbr.org/2019/08/what-people-hate-about-being-managed-by-algorithms-according-to-a-study-of-uber-drivers
- Möhlmann, M., & Zalmanson, L. (2017). Hands on the wheel: Navigating algorithmic management and Uber drivers’ autonomy. Proceedings of the 38th International Conference on Information Systems,Seoul, South Korea.
- Möhlmann, M., Zalmanson, L., Henfridsson, O., & Gregory, R. W. (2021). Algorithmic management of work on online labor platforms: When matching meets control. MIS Quarterly, (forthcoming). https://misq.org/algorithmic-management-of-work-on-online-labor-platforms-when-matching-meets-control.html
- O’Brien, S. A. (2018). Uber tightens driver background checks.Cable News Network (CNN). https://money.cnn.com/2018/04/12/technology/uber-safety-update/index.html
- O’Brien, S. A., & Yurieff, K. (2017). What we know (and don’t know) about Uber background checks.Cable News Network (CNN)https://money.cnn.com/2017/11/03/technology/uber-lyft-background-checks-new-york-terror-attack-suspect/index.html
- Oliver, C. (1991). Strategic responses to institutional processes. Academy of Management Review, 16(1), 145–179. https://doi.org/10.5465/amr.1991.4279002
- Ormond, D., Warkentin, M., & Crossler, R. E. (2019). Integrating cognition with an affective lens to better understand information security policy compliance. Journal of the Association for Information Systems, 20(12), 1794–1843. https://doi.org/10.17705/1jais.00586
- Podsakoff, P. M., MacKenzie, S. B., Lee, J.-Y., & Podsakoff, N. P. (2003). Common method biases in behavioral research: A critical review of the literature and recommended remedies. Journal of Applied Psychology, 88(5), 879–903. https://doi.org/10.1037/0021-9010.88.5.879
- Polites, G. L., & Karahanna, E. (2013). The embeddedness of information systems habits in organizational and individual level routines: Development and disruption. MIS Quarterly, 37(1), 221–246. https://doi.org/10.25300/MISQ/2013/37.1.10
- Polites, G. L., Roberts, N., & Thatcher, J. (2012). Conceptualizing models using multidimensional constructs: A review and guidelines for their use. European Journal of Information Systems, 21(1), 22–48. https://doi.org/10.1057/ejis.2011.10
- Pollock, N. (2005). When is a work-around? Conflict and negotiation in computer systems development. Science, Technology & Human Values, 30(4), 496–514. https://doi.org/10.1177/0162243905276501
- Porter, A. J., & van den Hooff, B. (2020). The complementarity of autonomy and control in mobile work. European Journal of Information Systems, 29(2), 172–189. https://doi.org/10.1080/0960085X.2020.1728200
- Posey, C., Lowry, P. B., Roberts, T. L., & Ellis, T. S. (2010). Proposing the online community self-disclosure model: The case of working professionals in France and the UK who use online communities. European Journal of Information Systems, 19(2), 181–195. https://doi.org/10.1057/ejis.2010.15
- Recker, J., Lukyanenko, R., Jabbari, M., Samuel, B. M., & Castellanos, A. (2021). From representation to mediation: A new agenda for conceptual modeling research in a digital world. MIS Quarterly, 45(1), 269–300. https://doi.org/10.25300/MISQ/2021/16027
- Ringle, C. M., Sarstedt, M., & Straub, D. W. (2012). A critical look at the use of PLS-SEM in MIS Quarterly. MIS Quarterly, 36(1), iii–xiv. https://doi.org/10.2307/41410402
- Ringle, C. M., Wende, S., & Becker, J.-M. (2015). SmartPLS 3. SmartPLS, Bönningstedt. https://www.smartpls.com/faq/documentation/how-to-cite-smartpls
- Rosenblat, A., & Stark, L. (2016). Algorithmic labor and information asymmetries: A case study of Uber’s drivers. International Journal of Communication, 10, 3758–3784. https://ijoc.org/index.php/ijoc/article/view/4892/1739
- Ruef, M., & Scott, W. R. (1998). A multidimensional model of organizational legitimacy: Hospital survival in changing institutional environments. Administrative Science Quarterly, 43(4), 877–904. https://doi.org/10.2307/2393619
- Sattler, H., Völckner, F., Riediger, C., & Ringle, C. M. (2010). The impact of brand extension success drivers on brand extension price premiums. International Journal of Research in Marketing, 27(4), 319–328. https://doi.org/10.1016/j.ijresmar.2010.08.005
- Saunders, C., Benlian, A., Henfridsson, O., & Wiener, M. (2020). IS control & governance. MIS Quarterly, Research Curations, November 23, 2020. MIS Quarterly (MISQ). https://www.misqresearchcurations.org/blog/2020/11/23/is-control-amp-governance
- Schafheitle, S. D., Weibel, A., Ebert, I. L., Kasper, G., Schank, C., & Leicht-Deobald, U. (2020). No stone left unturned? Towards a framework for the impact of datafication technologies on organizational control. Academy of Management Discoveries, 6(3), 455–487.https://doi.org/10.5465/amd.2019.0002
- Scheiber, N. (2017). How Uber uses psychological tricks to push its drivers’ buttons. New York Times (NYT). https://www.nytimes.com/interactive/2017/04/02/technology/uber-drivers-psychological-tricks.html
- Schnedler, W., & Vadovic, R. (2011). Legitimacy of control. Journal of Economics & Management Strategy, 20(4), 985–1009. https://doi.org/10.1111/j.1530-9134.2011.00315.x
- Smith, W. P., & Tabak, F. (2009). Monitoring employee e-mails: Is there any room for privacy? Academy of Management Perspectives, 23(4), 33–48. https://doi.org/10.5465/amp.23.4.33
- Strahan, R., & Gerbasi, K. C. (1972). Short, homogeneous versions of the Marlowe-Crowne social desirability scale. Journal of Clinical Psychology, 28(2), 191–193. https://doi.org/10.1002/1097-4679(197204)28:2<191::AID-JCLP2270280220>3.0.CO;2-G
- Suchman, M. C. (1995). Managing legitimacy: Strategic and institutional approaches. Academy of Management Review, 20(3), 571–610. https://doi.org/10.5465/amr.1995.9508080331
- Suddaby, R., Bitektine, A., & Haack, P. (2017). Legitimacy. Academy of Management Annals, 11(1), 451–478. https://doi.org/10.5465/annals.2015.0101
- Sun, H., & Zhang, P. (2006). Causal relationships between perceived enjoyment and perceived ease of use: An alternative approach. Journal of the Association for Information Systems, 7(9), 618–645. https://doi.org/10.17705/1jais.00100
- Sweetman, K. (2012). In Asia, power gets in the way. Harvard Business Review (HBR). https://hbr.org/2012/04/in-asia-power-gets-in-the-way
- Tabak, F., & Smith, W. P. (2005). Privacy and electronic monitoring in the workplace: A model of managerial cognition and relational trust development. Employee Responsibilities and Rights Journal, 17(3), 173–189. https://doi.org/10.1007/s10672-005-6940-z
- Thatcher, J. B., Stepina, L. P., & Boyle, R. J. (2002). Turnover of information technology workers: Examining empirically the influence of attitudes, job characteristics, and external markets. Journal of Management Information Systems, 19(3), 231–261. https://doi.org/10.1080/07421222.2002.11045736
- Thies, F., Wessel, M., & Benlian, A. (2018). Network effects on crowdfunding platforms: Exploring the implications of relaxing input control. Information Systems Journal, 28(6), 1239–1262. https://doi.org/10.1111/isj.12194
- Tiwana, A. (2015). Evolutionary competition in platform ecosystems. Information Systems Research, 26(2), 266–281. https://doi.org/10.1287/isre.2015.0573
- Uber. (2019a). Pre-first trip: Frequently asked questions (https://www.uber.com/drive/new-orleans/resources/prefirst-trip-faqs/
- Uber. (2019b). Riding with Uber: Driver compliments (https://www.uber.com/ride/how-uber-works/driver-compliments/
- Uber. (2020). Uber launches real-time ID check for drivers in the UK. https://www.uber.com/en-GB/blog/real-time-id-check-uk-drivers/
- Uzunca, B., Rigtering, J. P. C., & Ozcan, P. (2018). Sharing and shaping: A cross-country comparison of how sharing economy firms shape their institutional environment to gain legitimacy. Academy of Management Discoveries, 4(3), 248–272. https://doi.org/10.5465/amd.2016.0153
- Weibel, A., Den Hartog, D. N., Gillespie, N., Searle, R., Six, F., & Skinner, D. (2016). How do controls impact employee trust in the employer?. Human Resource Management Journal, 55(3), 437–462. https://doi.org/10.1002/hrm.21733
- Wiener, M., Cram, W. A.,& Benlian, A. (2020). Technology-mediated control legitimacy in the gig economy: Conceptualization and nomological network. In R. Hirschheim, A. Heinzl, & J. Dibbern (Eds.), Information systemsoutsourcing: The era of digital transformation, 5th edition (pp. 387–410). Springer. https://doi.org/10.1007/978-3-030-45819-5_16
- Wiener, M., Mähring, M., Remus, U., & Saunders, C. (2016). Control configuration and control enactment in information systems projects: Review and expanded theoretical framework. MIS Quarterly, 40(3), 741–774. https://doi.org/10.25300/MISQ/2016/40.3.11
- Wiener, M., Mähring, M., Remus, U., Saunders, C., & Cram, W. A. (2019). Moving IS project control research into the digital era: The “why” of control and the concept of control purpose. Information Systems Research, 30(4), 1387–1401. https://doi.org/10.1287/isre.2019.0867
- Woltjer, R. (2017). Workarounds and trade-offs in information security – An exploratory study. Information & Computer Security, 25(4), 402–420. https://doi.org/10.1108/ICS-02-2016-0017
- Wood, A. J., Graham, M., Lehdonvirta, V., & Hjorth, I. (2019). Good gig, bad gig: Autonomy and algorithmic control in the global gig economy. Work, Employment and Society, 33(1), 56–75. https://doi.org/10.1177/0950017018785616
- Wright, R. T., Campbell, D. E., Thatcher, J. B., & Roberts, N. (2012). Operationalizing multidimensional constructs in structural equation modeling: Recommendations for IS research. Communications of the Association for Information Systems, 30(Article23), 367–412. https://doi.org/10.17705/1CAIS.03023
- Wu, I.-L. (2013). The antecedents of customer satisfaction and its link to complaint intentions in online shopping: An integration of justice, technology, and trust. International Journal of Information Management, 33(1), 166–176. https://doi.org/10.1016/j.ijinfomgt.2012.09.001
- Yeo, L. (2021). Uber vs. Lyft: Who’s tops in the battle of U.S. rideshare companies. Bloomberg Second Measure LLC. https://secondmeasure.com/datapoints/rideshare-industry-overview/
Appendix A.
Construct Operationalisation
To ensure that survey respondents focused on the algorithmic aspects of Uber’s control system, we introduced the below-listed items with sentences such as the following: “Please refer to your interactions with the Uber driverapp [emphasis in original], and indicate to what extent you agree or disagree with the following statements”.
Table A1. Operationalisation of focal study constructs.
Appendix B.
Data Sample
Table B1. Descriptive statistics.
Appendix C.
Assessment of Measurement Model (Stage One)
Table C1. Construct correlations, composite reliability (CR), and Average variance extracted (AVE).
Table C2. Cross loadings between items and constructs.
Table C3. Heterotrait-monotrait (HTMT) ratio of construct correlations.
Appendix D.
Assessment of Common Method Bias
Since our data was self-reported from a single source, common method bias (CMB) is a potential risk. To reduce this risk, we incorporated several procedural remedies in the design of our survey instrument (Podsakoff et al., Citation2003), such as keeping questions simple, focused, and concise; avoiding double-barrelled questions and conceptual dependence between variables; using randomised items and different scales; and reiterating respondent anonymity along with the exclusive research purpose of our study (ibid). Adding to this, we used several of the more prevalent statistical (post-hoc) analyses and techniques to check for CMB (e.g., Chin et al., Citation2012; Podsakoff et al., Citation2003). First, extremely high construct correlations are an indicator of CMB (Podsakoff et al., Citation2003). The highest correlation between constructs was 0.70 (see Table C1 in Appendix C), which is considerably lower than the threshold of 0.90 (Bagozzi et al., Citation1991). Second, we conducted Harman’s single-factor test (Podsakoff et al., Citation2003), which reveals whether the majority of the variance can be explained by one particular factor. Neither did one general factor account for more than 50% of the total variance, nor did a single factor emerge from an exploratory factor analysis (one factor explained at most 29.89% of the variance in the data). Third, as noted earlier, we performed a full collinearity test, which is considered an effective test for the identification of CMB (Kock, Citation2015). Fourth, we controlled for CMB at the data-analysis level by partialling out the effects of social desirability (defined as “the tendency of some people to respond in a socially acceptable manner”), which is one common source of CMB in studies that use self-reported data (Podsakoff et al., Citation2003, p. 887). Specifically, using the partial correlation technique (Chin et al., Citation2012; Podsakoff et al., Citation2003), we added social desirability to our research model and linked it to all main independent and dependent variables (cf. Addas & Pinsonneault, Citation2018; Cameron & Webster, Citation2013).Footnote3 Comparing the results of the baseline model and the model including social desirability revealed that no paths gained or lost significance (see Table D1 below), further alleviating concerns that CMB accounted for the results. Taken together, the analyses and tests outlined above indicate that CMB is unlikely to be an issue that might confound the results of our study.
Table D1. Assessment of CMB using partial correlation procedure (Social desirability).