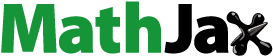
ABSTRACT
Word-finding difficulties for naming everyday objects are often prevalent in aphasia. Traditionally, treating these difficulties has involved repeated drilling of troublesome items with a therapist. Spaced repetition schedules can improve the efficiency of such training. However, spaced repetition in a therapy environment can be both difficult to implement and time-consuming. The current study evaluated the potential utility of automated, asynchronous, online spaced repetition training for the treatment of word-finding difficulties in individuals with aphasia. Twenty-one participants completed a two-week training study, completing approximately 60 minutes per day of asynchronous online drilling. The training items were identified using a pretest, and word-finding difficulties were evaluated both at the end of training (i.e., a post-test) and four weeks later (i.e., a retention test). The trained items were separated into three different spaced-repetition schedules: (1) Short-spacing; (2) Long-spacing; and (3) Adaptive-spacing. At the retention-test, all trained items outperformed non-trained items in terms of accuracy and reaction time. Further, preliminary evidence suggested a potential reaction time advantage for the adaptive-spacing condition. Overall, online, asynchronous spaced repetition training appears to be effective in treating word-finding difficulties in aphasia. Further research will be required to determine if different spaced repetition schedules can be leveraged to enhance this effect.
Stroke is a leading cause of adult disability, often inducing lasting impairments in motor and cognitive function. About a third of stroke survivors exhibit aphasia, an acquired disorder of language processing, while other individuals experience similar symptoms from head injury or neurodegenerative diseases such as primary progressive aphasia. Aphasia takes various forms according to the extent and location of brain damage, but the most consistent symptom common to all subtypes is a persistent difficulty in producing a desired word in context, i.e., anomia or word-finding difficulty (e.g., Grossman, Citation2014). Given the ubiquity of this symptom, its high relevance for everyday communication, and the relative ease of quantifying its severity and response to intervention, a large number of research studies have examined behavioural techniques for improving word finding in persons with aphasia, typically using a simple picture-naming approach (e.g., Middleton et al., Citation2019). The simplicity and effectiveness of picture naming drills has led to their adaptation into numerous computer programs and smartphone apps marketed towards those with aphasia (e.g., Tactus Therapy, Constant Therapy, Talkpath), as at-home practice offers the opportunity to engage in the drilling much more intensively and frequently than would be possible with synchronous therapy under the direct supervision of a therapist. Accordingly, a hybrid model of treatment has recently emerged as optimal, in which participants complete exercises on their own, but under the regular supervision of a therapist to select exercises, define treatment goals, and coordinate drilling with other (potentially more interactive) therapy activities (Meltzer et al., Citation2018).
Given that asynchronous automated therapy approaches are only growing in popularity, there is currently much interest in maximizing the effectiveness and efficiency for any given amount of time spent in training. Accordingly, several studies have sought to incorporate principles derived from empirical studies of human learning to optimize the efficiency with which various items are studied during treatment. One of the most promising techniques is based on the well-established spacing effect, i.e., the finding that repeated exposures to an item to-be-learned are more effectively retained if those exposures are interrupted (i.e., spaced exposure) rather than continuously repeated (i.e., massed exposure; Ebbinghaus, Citation1913; Toppino & Gerbier, Citation2014). Indeed, this type of spaced-repetition has been found to be more effective relative to massed-repetition for individuals with either dementia (Fridriksson et al., Citation2005) or aphasia (Middleton et al., Citation2016). Further, a similar advantage for spaced-repetition over massed repetition has been also observed in non-impaired individuals (Cepeda et al., Citation2006; Kang, Citation2016).
In traditional rehabilitation settings, spaced repetition training for individuals with aphasia may be challenging to implement. That is, careful consideration for presentation intervals as well as intermediary activities is often required (e.g., Fridriksson et al., Citation2005). Complicating the matter, learning and performance may be further enhanced by making use of adaptive spaced repetition paradigms and schedules (e.g., Middleton et al., Citation2016). In adaptive spaced repetition, the spacing between repetitions of items increases more quickly for items responded to correctly, leading to more frequent initial practice with troublesome items. Implementing adaptive trial scheduling in practice can get rather complex, and likely requires the use of an external scheduler. Fortunately, automated adaptive trial scheduling algorithms do exist that can aid in this rigorous process.
One such example is the supermemo-2 algorithm (SM2), which is freely available (Wozniak, Citation1998; Wozniak & Gorzelanczyk, Citation1994). The SM2 algorithm has been incorporated into open-source learning programs such as ANKI (ANKI Core Team, Citation2020). Such programs are quite popular as memorization tools in the general population. For instance, an Android-based version of the ANKI program has been downloaded over 10 million times from the Google Play Store as of August 2022 (Ankidroid-Open-Source-Team, Citation2022). Despite the inherent challenges, adaptive learning schedules have been successfully applied in small clinical settings (e.g., Fridriksson et al., Citation2005). Although a case study (Evans et al., Citation2016) using persons with aphasia has shown promise, a larger-scale investigation evaluating the utility of adaptive spaced repetition as a treatment for word-finding difficulties in aphasia has not been conducted. Given the inherent logistical challenges associated with adaptive-spaced repetition training with a patient population, we decided to utilize a self-directed online training program. Asynchronous computer-based training programs have been shown to be effective for the treatment of symptoms of dementia (e.g., Han et al., Citation2014, Citation2017) as well as post-stroke aphasia (Meltzer et al., Citation2018), with comparable outcomes to in-person treatment.
Thus, the primary purpose of the current study was to evaluate the efficacy of an asynchronous, online spaced-repetition picture-naming training program for the treatment of anomia in individuals with aphasia. To achieve this goal, individuals with aphasia studied 60 words that they showed difficulty in naming, over two weeks in ten online practice sessions lasting approximately 60 minutes each. In an attempt to elucidate a most effective trial-scheduling, the sixty trained items were subdivided into three distinct spaced-repetition schedules: (1) Twenty items were trained with randomized blocks containing each of the items (the “big-deck” condition, simulating shuffling a deck of twenty cards on each cycle); (2) Twenty items were trained in smaller, 5 item randomized blocks, which yielded a relatively shorter inter-item spacing (the “small-deck” condition, simulating four decks of 5 cards, each of which is studied and shuffled four times before moving on); and (3) Twenty items were trained using a modified version of the SM2 (adaptive spaced repetition) algorithm. This algorithm scheduled items into a future block based on participant performance. Participants’ picture naming ability was re-evaluated the week following training (i.e., a post-test) and one month later (i.e., a retention-test). Notably, all three of these scheduling conditions employed a version of spaced repetition, given that massed practice (i.e., non-spaced repetition) has been well established to be inferior to spaced repetition in treatment settings (Middleton et al., Citation2016). Given the targeted nature of adaptive spaced repetition, it was hypothesized that performance would be enhanced to a greater extent in this condition over the two non-adaptive conditions (i.e., greater picture naming performance after training).
Methods
The study protocol was approved by the research ethics board of Baycrest Hospital. All participants provided informed consent prior to the commencement of the study, using an online consent form implemented in the REDCAP platform.
Participants
The inclusion criteria for the present study were the presence of a word finding deficit stemming from aphasia, and the ability to evaluate the correctness of one’s own responses, when subsequently provided with the correct answer. This latter criterion was imperative for the completion of the training portion of the study. Notably, this ability to evaluate one’s own response correctness was determined in the early portions of the pretest evaluating naming of specific candidate pictures for training (see below).
The following exclusion criteria were applied: (1) A diagnosis of an additional neurological disorder other than the one causing the aphasia; (2) Insufficient word-finding difficulties for the currently-utilized word set. This criterion was based on the fact that the training word-sets were determined from a master-list of potential words. Participants able to produce all, or nearly all of the master-list prior to training, were excluded from continuing in the study.
Twenty-eight participants were initially recruited from local aphasia support programs as well as advertisement on social media through aphasia-related groups (18 Males; 10 Females). Twenty-four of these participants reported word-finding difficulties as a consequence of a stroke, and the remaining four indicated a diagnosis of primary progressive aphasia, or a related disorder. All participants were screened first by telephone, and reported word-finding difficulties in daily life. Most stroke survivors confirmed that their stroke was in the left hemisphere of the brain, although some were unsure. No symptoms suggestive of right-hemisphere stroke (e.g., neglect, left-sided hemiparesis) were noted.
Materials and procedures
All contact with participants was conducted over telephone or video-conference software via computer. Following initial screening that was used to ensure the participants met most of the key inclusion/exclusion criteria, three video-conference portions of the study were completed with the experimenters: (1) The pretest occurred before the treatment intervention; (2) The post-test session occurred following the completion of the training regimen; and (3) The retention-test session was completed four weeks following the post-test session. A flow chart of recruitment and participation is shown in .
Pre-test
The purpose of the pre-test session was two-fold. First, the severity of participants’ aphasia symptoms was quantified using the Quick Aphasia Battery (QAB; Wilson et al., Citation2018). The QAB was slightly modified for remote administration. The experimenter and participant viewed each other remotely, over a webcam, and visual stimuli were presented via the screen-sharing function of Zoom. Also, instead of having the participant “point” to desired objects to identify them (i.e., QAB subsection 3), the experimenter hovered the mouse sequentially over the response options for up to six seconds and the participant indicated when the correct item had been highlighted. The QAB portion of the pre-test session lasted approximately 20 to 30 minutes.
The second purpose of the pre-test was to review a master-list of 292 potential training images. 175 images were taken from the Philadelphia naming test (Roach et al., Citation1996), but with the original black and white line drawings replaced by similar full-color photos obtained from the internet. The remaining 117 were items identified as personally relevant by participants in a previous study of naming therapy for PPA (Jokel et al., Citation2016). All images were familiar objects and elicited nouns as the naming response. Of these potential images, we aimed to identify a subset of 80 items eliciting naming difficulty in each individual participant, to be used in the training portion of the study. This portion of the pre-test session had the experimenter screen-sharing a slideshow presentation. Each of the potential images were presented in random order, accompanied by a 200-ms tone. The purpose of this tone was to facilitate the measurement of response-time (i.e., reaction time), which could provide additional information regarding the ease at which items were identified (e.g., Conroy et al., Citation2018). The participants were instructed to verbally identify each item, as it was presented on the screen. If the participant provided a response that indicated a possible perceptual uncertainty (i.e., mistaking cabbage for lettuce), the experimenter would provide a hint to ensure the difficulty with the item was due to retrieval issues. Additionally, if participants provided an image appropriate synonym, the participant was queued for “another name for the image.” All 292 pictures were repeated in two separate runs in a different order. Depending on the ability of each individual participant, these runs could take anywhere from 1.5 to 3 hours to complete. As such, the two pre-test runs were completed on separate days.
As accurate self-scoring was essential to the completion of the training sessions, the ability of participants to evaluate their own performance was explicitly evaluated at the beginning of first pre-test run. For all pictures in the first pre-test run, we provided participants with the correct answer after each response attempt. For a minimum of 10 trials, participants were additionally asked to indicate with a “yes” or a “no” whether they had correctly named the image presented during the trial. This additional query continued until the experimenter was confident that the participant could complete the training portion of the study (i.e., between 10 and 20 items). Notably, all participants were able to evaluate their own performance satisfactorily, and none were excluded in this phase of the study. Once self-scoring ability had been confirmed, the pre-test portion would continue without explicit self-evaluation by the participants. However, to control for potential effects of explicit feedback on the desired response for each image, we continued to provide the correct name of each image after the participant’s naming effect for the rest of the first pre-test (e.g., “Correct, that is a lion”). No such feedback was given for the second pre-test run. Once the two pre-test sessions were completed, the participants’ performance was evaluated, and when possible, a subset of 80 items were selected for inclusion for each participants’ training.
Training-list generation
Given that each of the 292 items were repeated twice during the pre-test, pre-test performance accuracy was scored out of two. This scoring process was completed based on the recordings of each session by a single experimenter. When scoring response correctness, minor phonological distortions were deemed acceptable as the focus of the experiment was on alleviating word-finding difficulties. Further, if the participant could only come up with a different, yet reasonable/valid name for an object, it was not scored as incorrect on the pretest when the use of the chosen alternative word could be reasonably expected to be understood to represent the chosen image in normal conversation (e.g., synonyms: pants vs. trousers). Eighty items from the master list of 292 were then selected as candidate items for each participant for use in the training phase. The eighty words were randomly selected from the candidate words with the lowest pre-test performance. That is, if there were 80 or more words that were incorrectly identified during both pre-test runs, a randomly selected subset of those words were selected. If fewer than 80 words were incorrectly identified twice during the pre-test, words that were correctly identified once were randomly selected to fill out 80 words. Lastly, if 80 troublesome words had still not yet been identified, words that were correctly identified twice, but exhibited the longest response times were added, but these words were excluded from analysis (see next paragraph). An overview of the stimulus sorting process is presented in . If fewer than 60 words were named incorrectly, the participant was excluded from the study.
Figure 2. A flow chart illustrating the selection of individualized stimulus lists from the master list and sorting into four conditions.
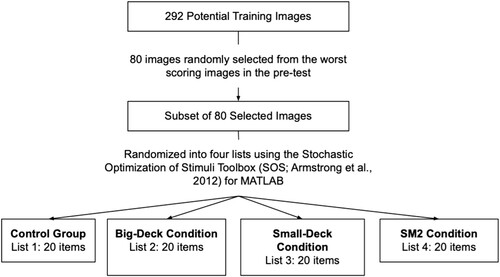
Once 80 prospective items were identified, they were subdivided into four lists of 20. This was completed using the Stochastic Optimization of Stimuli Toolbox (SOS; Armstrong et al., Citation2012) for MATLAB. This process was employed to ensure that the four subsets of twenty items were statistically equated for both the number of syllables (obtained from the CMU pronunciation dictionary, Weide, Citation1998) and word-frequency (Zipf value) using the SUBTLEX-US database (Brysbaert & New, Citation2009; ps ≥ .18). In the event that fewer than 80 items had been successfully identified, the additionally-flagged items, (i.e., those that were identified correctly twice, but exhibited the longest response times), were automatically relegated to the fourth (i.e., control) condition (see below), and 60 of the remaining items were statistically subdivided, as described above. These correctly named items were excluded from the statistical analyses, but were maintained in the performance of the post and retention tests to ensure an equal number of tested items for all participants.
Online training
Following the creation of the four sets of twenty items, three were assigned to spaced-repetition training conditions, and one to a control condition. The three training lists, and associated images and audio-clips were uploaded into a custom training protocol, programmed using the Lab.js platform (Henninger et al., Citation2020) and hosted by open-lab.online (https://www.open-lab.online/).
Following the preparation of the four lists, participants were sent a link via email that brought them to the training session. Participants were asked to use the training platform each day, Monday-to-Friday, for two weeks. Each day’s session involved an onscreen timer set initially to 30 minutes. Each day, 10 minutes of this timer were dedicated to each of the three training lists. Notably, the ordering of these lists was randomly generated each calendar day. Also, the transition between lists was seamless and unknown to the participants. To ensure a minimum number of 60 completed training trials per day per list, any given trial could only decrease the time remaining on the timer by 10 seconds, irrespective of how much time was actually spent on the trial. This resulted in daily training exceeding 30 real-world minutes in cases when participants were having difficulty on many items.
On each training trial, participants were presented with a picture in the upper centre of the screen (see (A)). To the top left, the daily running timer was displayed. To the top right, a “quit” button was presented. Participants were free to stop and restart training at their leisure, but were instructed to try to complete the daily training in a single session if possible. However, emphasis was placed on completing the daily timer, over doing so in a single session. Below the currently-pictured item were three click-able options: (1) “Meaning Hint” which provided a textual and auditory definition of the pictured item; (2) “Sound Hint” which provided a textual and auditory phonological hint; and (3) “Reveal Answer” which revealed the identity of the picture with both text and audio.
Figure 3. (A) A depiction of the initial screen of each trial with all options not clicked. (B) The response evaluation screen after both the “meaning hint” and the “reveal answer” boxes were successively clicked.
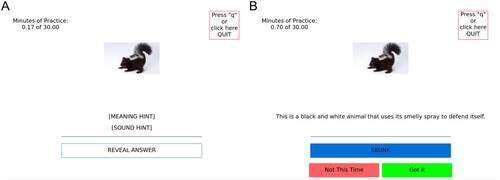
When presented with a picture, participants were instructed to attempt to state its identity aloud. If they required a hint, they could click on either or both hints, and then attempt to state the identity of the picture aloud. When participants were satisfied with their response, or they exhausted both hints and were still unable to name the item, they were instructed to click the “Reveal Answer” button. Once the answer was presented to the participant, two more response buttons appeared below: (1) a green “Got it” button on the right; and a (2) red “Missed it” button on the left. Using these buttons, participants we asked to indicate whether they had successfully identified the picture prior to the answer being revealed to them (see (B)). Once the participant entered their response, a new trial began automatically. At the end of the 30 minute timer, the participants were presented with a “Practice Complete” screen, and could not continue to practice until the next calendar day.
Each of the three training lists employed a unique spaced-repetition trial scheduling algorithm. The first list of 20 items (the Big-Deck condition) was practiced such that each picture was repeated at least once in every “block” of twenty trials. After each successive 20 trials were completed, the ordering of the individual items within the list were re-randomized. Given the 10-s limit on the time-tracker, this scheduling resulted in a minimum of 3 “blocks” of Big-Deck trials per day (more, if participants proceeded relatively quickly through the trials, which was rare).
The second list of 20 items (the Small-Deck condition) used a similar randomization procedure on a smaller scale. That is, the 20 item list, was first randomized, then the list was subdivided into four 5-item chunks. These chunks were themselves sequentially randomized and concatenated four times, yielding 20 consecutive trials per chunk. These four sub-lists were then combined into a 80 trial block. A consequence of this chunking and reshuffling, item repeats tended to often occur at an average interval of 5-items, followed by a relatively longer interval. That is, item repeats were relatively short, while a particular item was being studied. After every 80 trials, the overall list of 20 items was re-randomized, and the chunks were redefined.
The third list of items to be trained (the SM2 condition) used an adaptive spaced-repetition trial scheduling procedure. The algorithm utilized was a slightly modified version of the supermemo-2 (SM2) algorithm (Wozniak, Citation1998; Wozniak & Gorzelanczyk, Citation1994). This algorithm computes the interval at which to repeat an item based on the participant’s performance history with the item. Following correct responses, the next interval (in blocks) was computed for the current item based on the product of the item’s current interval and its current ease-factor. The larger the ease-factor, the longer the prescribed interval. Following incorrect responses, the next interval was set to 1 (next block). The first two successive correct-responses following an incorrect response always had prescribed intervals of one and six blocks. Once an item was correctly identified three times in a row, the ease factor was used to determine the next interval. Nevertheless, the items ease-factor was updated after each trial based on the “quality” of the participant’s performance (scaled 0–5). In the traditional SM2 algorithm, the quality of responses are self-rated on a scale of 0 (hard) to 5 (easy). The current implementation mapped these options implicitly based on a combination of hint utilization and trial accuracy. Response quality was scored in the following manner: (5) Correct response, no hints required; (4) Correct response, one hint required; (3) Correct response, two hints required; (2) Incorrect response, no hints required; (1) Incorrect response, one hint required; (0) Incorrect response, both hints required. The ease-factor for each item had a constrained lower bound of 1.3, and no upper limit. All items were initialized with a default ease-factor of 2.5.
There were two main differences between the current implementation of the SM2 algorithm and the traditional version. First, rather than use a timescale of “days” for item repetitions (intervals), the current algorithm used the timescale of “blocks of trials.” That is, after responding to an item, the interval, in number of blocks, before its next presentation would be set, with 1 being the next block. The second major change to the SM2 algorithm was that in the event of an “empty” scheduled block (i.e., no scheduled items), the next scheduled block of trials was run. This kind of scoring could result in scheduled blocks ranging in size from 0 (no items scheduled) to 20 (all items scheduled). A block could be continued the next day if not finished when training ended. A detailed presentation of the modified SM2 algorithm is given in Appendix 1.
A fourth list (the control condition) was not trained, but used in the post-test and retention-test phases.
Post-test
The post-test session was completed the week following the completion of the two weeks of training on the three lists. The post-test was structured in a similar way to the pretest slide-show with only the 80 selected items included. That is, participants were instructed to identify a picture presented to them following its presentation concomitant with a 200-ms beep, and each item was presented twice across two randomized runs. The post-test session lasted approximately 30–45 minutes.
Retention-test
Four weeks following the post-test session, a retention-test session was completed which was structured in exactly the same manner as the post-test session.
Design and analysis
The main contrasts of interest for the current study were the comparisons of the participants’ post-test and retention test performance between the three studied lists (Lists A–C) and the control list (List-D). To evaluate these patterns, the data were analyzed using mixed-effects modelling in R (R Core Team, Citation2021) using the lmerTest package (Kuznetsova et al., Citation2017). Data for this analysis was included on a trial-wise basis, and separate analyses were conducted on response accuracy and response time. Any trials completed for items that had been included in the 80 test-items despite being correctly responded-to twice during the pretest were excluded from the main analyses. Note that responses that were provided in less than 20 seconds were scored correct, and response times were measured only for correct responses.
Response time scoring was completed via visual inspection of the recorded sound waveform in the open-source digital audio editor Audacity (https://www.audacityteam.org/). The response time was measured as the duration elapsed from the end of the 200-ms go-beep to the start of the utterance of the correct word. If the participant utilized self-cueing to get to the answer, the time up to the target word was recorded. If the participant got the response incorrect, or if the experimenter was required to ask for any type of clarification (e.g., perceptual confusions), then the item was excluded from statistical analysis of response time (1.4% of correct responses). All responses were scored by the same author (LC), after training and verification from the first author. Author LC was blinded to the assignment of words to different conditions.
The main statistical analysis was structured with fixed-effects of Session (i.e., Post-Test, Retention-Test) and List (i.e., Big-Deck, Small-Deck, SM2, Control), plus their interaction. The random-effects structure allowed for random-intercepts by Participant, Session within Participant, and List within Participant. Additionally, the following covariates were included in the model: Z-scaled number-of-syllables, Z-scaled word-frequency, and Z-scaled QAB-score. The statistical significance of the fixed-effects were evaluated with F-statistics generated via Satterthwaite’s method (i.e., using the “anova()” function on the model). Planned post-hoc contrasts were computed using the Emmeans package to compare all combinations of Lists within Sessions (Lenth, Citation2021). Type-I error-rate was controlled using the false-discovery-rate (Benjamini & Yekutieli, Citation2001).
Three additional sets of analyses were also completed to supplement the main analyses. The first sought to confirm differences in item-spacing across the three training list conditions. To accomplish this, the average item-spacing between repeats was computed for each trained word for each participant. Differences between the training conditions were evaluated a repeated-measures ANOVA (i.e., mean item-repeat spacing across the three training lists), with post-hoc contrasts completed as required. The second was a general evaluation of training performance. This was accomplished using the “ease-factor” measure inherent to the SM2 algorithm. That is, as participants reported successful training trials, this “ease-factor” would increase. Although the calculation of the ease-factor is only necessary for the SM2 algorithm, ease-factors were computed post-hoc on all trained items for all participants. Training performance on all items could be succinctly summarized by a single value, which could be compared across training conditions. Accordingly, these final-ease values were contrasted across training lists using a repeated-measures ANOVA, with post-hoc contrasts completed as required.
The third supplementary analysis completed was an evaluation of the combined relationship between speed-and-accuracy on post- and retention-test performance. To this end, the cumulative number of correct-responses were computed and visualized within the first 10-s of each evaluation trial. Notably, this final analysis could informally highlight differences in performance across conditions, not intuitive in the primary, separate analyses of response accuracy or time.
Results
Following the completion of the pretest, four participants had fewer than 20 items incorrectly named at least once. As there were insufficient items to train, these individuals did not continue with the training portion of the study. One participant withdrew from the study due to health problems, and two participants were unable to use the training protocol successfully, most likely due to comprehension problems and cognitive deficits. We suspect that these difficulties could be overcome with the in-person assistance of a therapist but this was beyond the scope of the present study. Altogether, this left 21 participants who completed the entirety of the study, and were ultimately included in the primary analyses described below. Demographic characteristics and scores for each sub-component of the QAB are presented in for all participants. Notably, word comprehension was intact in all participants, with clear impairments present on the other components.
Table 1. Demographic characteristics and Quick Aphasia Battery (QAB) scores.
Overall, participants trained for an average of 55 minutes per day (SD = 15 minutes). Thus, participants tended to take significantly more time, on average, than the minimum 10-s per training trial, that would have resulted in 30 minute training sessions. Participants requested the “Meaning” hint on 4% of training trials and the “Sound” hint on 11%. Both hints were requested on 3% of all training trials. Furthermore, hints of both types tended to be utilized to a greater extent early in training. 70% of the “Meaning” hints and 53% of the “Sound” hints used, had been used on average by the end of the 3rd training day.
Additionally, there was some variation in the training completion rates across the participants who completed the study. Two participants missed one day of training, four missed a single training list on a single day (i.e., 3 Big-Deck sessions and 1 Small-Deck session), one participant missed the same list on two training days (i.e., 2 SM2 sessions). This lost training comprised only 2.1% of the prescribed training trials across these participants. Two participants completed the training on all fourteen days of the two weeks, rather than the prescribed 10. Nevertheless, this training variability did not have a noticeable influence on the participant level data, nor the distributions of training repetition of items in each list (See Panel A for a density plot of average Participant-level training item repetitions).
Figure 4. (A) A density plot of the item-repetition spacing during training across the 3 Training Lists. (B) A density plot of the item-repetitions during training across the training lists.
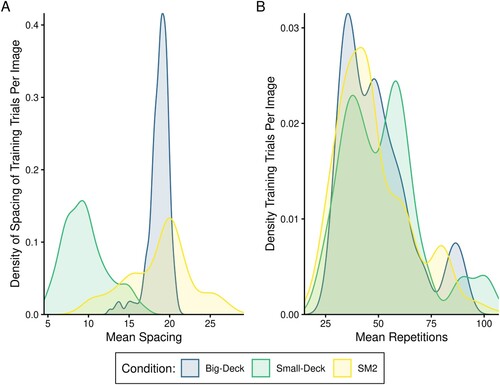
On average, the post-training session was completed 3.1 days (SD = 1.1 days) and the retention-test was completed 31.1 days (SD = 1.0 days) following the last day of training.
Validation of scheduling manipulations and item spacing
Prior to the evaluation of the primary analyses of interest, a validation of the scheduling manipulations across training lists was completed. That is, the item-spacing between repetitions of a training image was contrasted across lists (Mean item spacing (SD): Big-Deck = 18.7 (1.1); Small-Deck = 9.6 (2.2); SM2 = 18.7 (2.1)). The associated repeated-measures ANOVA indicated a significant effect of List F(2,42) = 393.8 p < .001. Post-hoc pairwise comparisons indicated that the Small-Deck condition exhibited significantly smaller item-repeat spacing relative to both the Big-Deck condition (p < .001, Cohen’s d = 4.52), and the SM2 condition (p < .001, Cohen’s d = 4.28), which did not differ significantly from one another (p > .999, Cohen’s d = 0.03). The patterns can be easily observed in (A), showing the density of the average item-repetition spacing for each trained word across all participants. The SM2 manipulation also invariably influences the number of training repetitions of words. That is, the words that participants had the most difficulty with during training would have received relatively more training repetitions and those words that participants had the least difficulty with would have fewer training repetitions. Thus, it was anticipated that the distribution of training repetitions would be similar for the Small-Deck and Big-Deck conditions, but wider and shallower for the SM2 condition. This expected pattern can be observed from the training data for all participants and all words in (B).
Picture naming accuracy
The analysis of response accuracy yielded significant main effects of Session, F(1,20.06) = 5.71, p = .027, and List F(3,59.81) = 45.20, p < .001. Additionally, the interaction between List and Session was also statistically significant, F(3,56.64) = 3.72, p = .016, reflecting the fact that accuracy was highest for trained stimuli at post-test, and lower at the 4-week retention test. Post-hoc contrasts were used to follow-up the significant interaction and the p-values were adjusted using the False-Discovery-Rate (Benjamini & Yekutieli, Citation2001). These contrasts indicated that response accuracy was significantly greater for all three training lists relative to the untrained control list (i.e., ps < .001, Cohen’s ds > .59). No other contrasts were statistically significant (Cohen’s ds < .070). Condition means can be found in (B). Individual participant data are provided in supplementary information.
Figure 5. (A) The main results for Response Accuracy across Word Lists. (B) The main results for Response Time across Word Lists. Error bars represent within-subjects 95% confidence intervals.
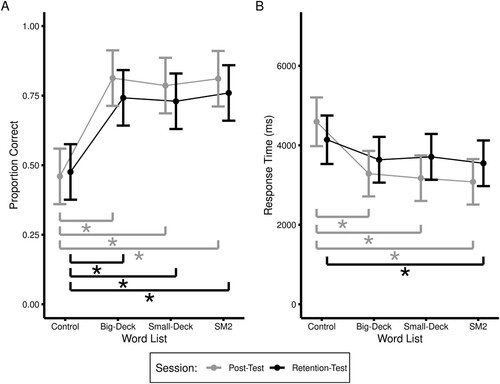
Response times
The analysis of response times yielded a significant main effect of List F(3,67.99) = 18.36, p < .001, and a significant interaction between List and Session, F(3,66.88) = 4.21, p = .009. Post-hoc contrasts were used to follow-up the significant interaction and the p-values were adjusted using the False-Discovery-Rate (Benjamini & Yekutieli, Citation2001). These contrasts indicated that response times were significantly faster for all three training lists relative to the untrained control list for the Post-Test Session (p < .001, Cohen’s d > .38), however, only the SM2 condition remained statistically faster than the control condition at the Retention-Test Session, (p = .049, Cohen’s d = .23). No other contrasts were statistically significant (Cohen’s d < .15). Condition means can be found in (B).
Training performance
Analysis of the post-training evaluation of the list-specific final item “ease” values resulted in a significant main effect of List, F(2,38) = 8.74, p < .001 (see ). Post-hoc pairwise comparisons indicated that self-reported training performance was significantly higher for the Small-Deck relative to the other two training conditions (p < .020, Cohen’s d > .16), which were not statistically different from one another, (p = .452, Cohen’s d = .09).
Speed-accuracy considerations
Although neither the analysis of response accuracy or response time yielded significant differences between the three main training conditions, visual inspection of the results revealed patterns that might emerge in a larger study with a larger set of training items. For accuracy, the Small-Deck condition exhibited the lowest accuracy, and for response time, the SM2 condition appeared to have a slight advantage. To get a more holistic view of these results, performance was also visualized considering both response accuracy and speed together (). In this plot, the cumulative correct responses across all participants as a function of time have been presented. Although purely qualitative in nature, the SM2 condition appears to accrue more correct responses earlier, relative to the other conditions. This may indicate a slight efficiency advantage of this condition over the others, although this effect was not large enough to manifest as a significant difference in either speed or accuracy.
Figure 6. Total cumulative, correct responses across all participants for (A) The Post-Test Session; and (B) The Retention-Test Session.
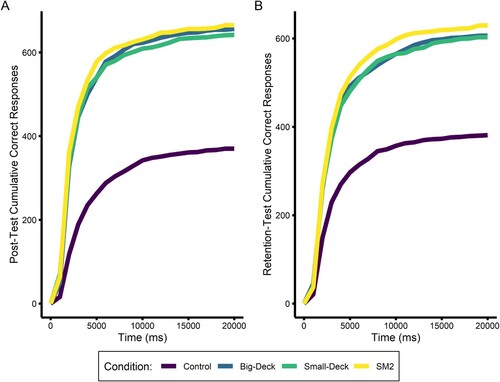
Discussion
In this study, participants completed two weeks of online training with 60 items in a picture-naming task. The 60 words were divided into three lists that were scheduled using three different forms of spaced repetition that varied in their assignment of intervals between repetitions of the same items. Performance on the three sets of training items (plus a set of control items) was evaluated pre- and post-training and at a one-month follow-up retention test. Following training, all three training conditions significantly outperformed the control condition. Further, although all training conditions yielded significantly faster responses immediately following training (i.e., the post-test Session), only the SM2 (adaptive) condition maintained this statistical response time advantage at the four-week retention session.
Anomia is one of the most prevalent and consistent symptoms associated with aphasia, and as such, significant effort has been put forth in developing treatments for improving this symptom. Fortunately, treatments prevalent in speech-language pathology practice have proven reasonably effective at improving picture naming in aphasia (Best et al., Citation2013; Brady et al., 2016). Due to its easy quantification reliability, and the high prevalence of naming deficits in all forms of aphasia, picture naming remains the most commonly targeted ability in aphasia treatment among practicing clinicians and researchers. Meta-analyses have shown that for a lasting clinically meaningful benefit, therapeutic interventions need to be high-intensity, high-dose, long-duration, or all of the above (Brady et al., 2016). Given that access to qualified therapists is sharply limited by supply, travel logistics, cost, and lack of insurance coverage, there is naturally much interest in supplying treatment for naming deficits using asynchronous exercises automated by a computer or mobile device (Lavoie et al., Citation2017; Vaezipour et al., Citation2020).
Several mobile applications have thus achieved widespread use in the asynchronous treatment of aphasia, including Tactus Therapy, Constant Therapy, and Talkpath by Lingraphica, to name a few of the most popular. Notably, these apps offer similar functionality to apps commonly used by healthy adults to study vocabulary in a second language, apps such as Anki, Memrise, and Duolingo. However, the popular aphasia apps lack one key feature that has become almost universal in language-learning apps: adaptive spaced repetition. Such apps use a scheduling algorithm to keep track of a user’s performance on every training item. When items are answered correctly, they appear less often, and when they are missed, they are trained more frequently. As a result, the practice is focused on the items that need the most attention, leading to highly efficient allocation of limited practice time.
Although “spaced repetition” has been explored in several studies of aphasia rehabilitation, it is commonly done in a non-adaptive framework, where fixed repetition intervals are predetermined by the experimenters and compared to an alternative condition such as massed repetition, with an item repeated several times in a row. Adaptive scheduling algorithms have largely not been explored in aphasia rehabilitation. In the present study, we aimed to evaluate the impact of adaptive scheduling compared to more familiar item scheduling strategies in the context of training on a relatively small set of items (20 per condition), as is commonly done in research studies examining the augmentation of training with interventions such as non-invasive brain stimulation (Sandars et al., Citation2016; Shah-Basak et al., Citation2016), or comparing the impact of different training strategies such as phonological component analysis and semantic feature analysis (Haentjens & Auclair-Ouellet, Citation2021). For comparison with such studies, and to examine whether or not adaptive scheduling is a useful feature of behavioural training on small sets of items, we directly compared the adaptive scheduling algorithm SM2 with two non-adaptive scheduling methods. First, the “big deck” condition, in which 20 items are presented in a randomized order without repetition, and then randomized again, represents the most common strategy in aphasia treatment studies. Second, the “small deck” condition, repeats 5 items 4 times each (with randomization within that deck of 5) before moving on to another set of 5 items.
Notably, all of these training conditions are arguably examples of “spaced repetition” loosely defined, and none of them fit the alternative descriptor of “massed repetition.” None of our 3 training conditions involved presentation of the same item two or more times in a row. The advantages of spaced over massed repetition are well-established in experimental studies with healthy participants (Bloom & Shuell, Citation1981; Cepeda et al., Citation2009) and have been observed to apply to picture naming training in persons with aphasia as well (Middleton et al., Citation2016). In the present study, the most striking difference between our training conditions, although not significant, was the lower accuracy observed in the post-training and retention tests for the small deck condition relative to the other two conditions ((A)). This finding contrasts with the “ease factor” of the items estimated with the SM2 algorithm applied to all three conditions, including the nonadaptive ones (). In that analysis, the small deck condition resulted in higher ease factors, suggesting that participants tended to answer those items correctly more often during the training sessions. However, the fact that the condition with the highest training performance turned out to have the worst performance on the post-training tests illustrates the problem with insufficient spacing (including, in the extreme, massed repetition). In the small deck condition, repetitions are more closely spaced, and although this improves performance in the short term, it does not translate well into long-term retention. Items that are too closely spaced may still be active in short-term memory when repeated (Glanzer, Citation1969), and therefore fail to activate elaborate semantic encoding upon repetition (Rose, Citation1980), and also may fail to elicit retrieval from long-term memory, thus missing out on the “retrieval practice” benefit (Karpicke & Roediger, Citation2008). Thus, there seems to be no advantage at all to choosing a “small deck” repetition schedule in which items are grouped into small sets for more frequent presentation within blocks.
Figure 7. List-wise training-performance across Word Lists as measured by SM2-defined ending Ease-Factor. Error bars represent within-subjects 95% confidence intervals.
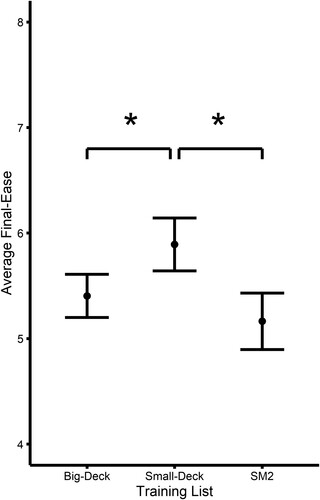
The differences between the big deck and SM2 conditions were less dramatic. No significant differences emerged in accuracy or reaction time (). However, the SM2 condition numerically outperformed the big deck condition on both these measures, suggesting that it at least is no worse than the traditional approach of fully randomizing the trials on each round of presentation, and may actually produce better gains when used for a longer period of time on more stimuli. This point is critical to the future development of fully automated approaches for asynchronous treatment. In the current dominant approach, a therapist selects the specific items for treatment, typically limiting them to a relatively small set such as the twenty items used in each condition for the present study. New training items may then be introduced by the therapist, and mastered items dropped, but this requires deliberate intervention, and still the practice of drilling all currently selected items in every treatment session persists. Adaptive spaced repetition, as implemented in the SM2 algorithm, allows for continuous adjustment of repetition schedules in a fully automated manner. This is essential when the list of items to be trained grows longer, especially when it is so long that it is no longer feasible to train every item every day. This is the typical usage situation for a healthy person using software to acquire vocabulary in a foreign language, and it could also become a typical usage situation for people with aphasia training on a large number of items, whether for picture naming or any other linguistic task. A previous meta-analysis has suggested that persons with aphasia can tolerate many more items for therapy than the amount typically used in research studies (Snell et al., Citation2010), but ultimately adaptive techniques will likely be needed for efficient training of hundreds of items.
Up to now, the use of adaptive spaced repetition in aphasia treatment has been very limited. One small study found advantages of the approach using a method of doubling or halving the repetition interval based on response correctness (Fridriksson et al., Citation2005). A case study of a patient with primary progressive aphasia who used the program Anki to maintain expressive vocabulary demonstrated strong gains and maintenance relative to other patients with a similar condition (Evans et al., Citation2016). In seeking to expand the scope of automated asynchronous treatment, it is noteworthy that the “big deck” approach cannot be used as the list of items to be trained continues to grow, and some form of automated stimulus selection must be implemented. Our results demonstrate that the adaptive method (SM2 and similar algorithms) used in spaced repetition software designed for healthy language learners performs equally or perhaps better than the traditional big deck approach in the short term, and may offer significant advantages for accuracy and response time in the long term. However, further studies with a larger stimulus set and longer training period will be needed to confirm this.
Although the current study has provided a pattern of promising results regarding the online treatment of anomia on those with aphasia, a number of significant limitations must be acknowledged and addressed by future research. First, although the targeted population was individuals with aphasia presenting with anomia, not all participants who fit this description ended up being good candidates for the study. Indeed, a number of participants who self-reported word-finding difficulties did not exhibit difficulty with enough of the current study’s master word-list for a useful personalized training-set to be produced. Further, three individuals, who were among the most impaired of the study candidates, were unable to use the online training software and could not complete the study. Thus, there may be a “sweet-spot” of ability at which this type of training may be most effective. Ultimately, this means that the current findings can only be cautiously generalized to aphasia populations as a whole. In contrast, the current study did provide a relatively larger sample of individuals with aphasia than many, if not all of the previous studies examining spaced-repetition for treating word-finding difficulties in aphasia (e.g., Evans et al., Citation2016; Middleton et al., Citation2016, Citation2019; Schuchard et al., Citation2020). Therefore, the strong potential efficacy of such a treatment approach should not be discounted. Second, although the approximately 60 minutes a day of training across ten training days represented significant effort on the part of the participants, it may not have been enough training to fully differentiate the differences between the training conditions. Indeed, benefits from spaced-repetition training can still be evident at time-scales of months (e.g., Evans et al., Citation2016). Furthermore, and small degree of difference observed between the Big-Deck and SM2 conditions may have been exacerbated by the fact that both conditions started with 20-items in their trial lists. That way, the experience of the participants in these conditions would only diverge as items were correctly responded to during training in the SM2 condition. Thus, a larger volume of trials may have been necessary, or a smaller initial trial-list could have been used in the SM2 condition to better facilitate differential performance across these conditions more efficiently. Nevertheless, these predictions will require future research to be directly examined.
Overall, the current study provided an characterization of online spaced-repetition training for treating aphasia-related anomia in a relatively large sample. Three different spaced-repetition training schedules were considered: (1) Traditional drilling (Big-Deck Condition; large inter-item spacing) (2) Condensed drilling (Small-Deck Condition; Medium inter-item spacing); and (3) Adaptive spaced repetition based on the SM2 algorithm (SM2 condition; adaptive spacing). All three spaced repetition protocols yielded significant improvements in anomia both at the end of training, and after a four-week washout period. Although no significant differences were observed between the three training conditions, only the adaptive condition yielded significantly faster response times relative to the control condition. Overall, online, self-paced picture naming tasks can be successfully employed to help treat anomia in individuals with aphasia, and adaptive spaced-repetition paradigms may prove to be the most efficient method currently available.
Disclosure statement
No potential conflict of interest was reported by the author(s).
Additional information
Funding
References
- ANKI Core Team. (2020). ANKI. https://apps.ankiweb.net/
- Ankidroid-Open-Source-Team. (2022). Ankidroid. https://play.google.com/store/apps/details?id=com.ichi2.anki&hl=en_CA
- Armstrong, B. C., Watson, C. E., & Plaut, D. C. (2012). SOS! An algorithm and software for the stochastic optimization of stimuli. Behavior Research Methods, 44(3), 675–705. https://doi.org/10.3758/s13428-011-0182-9
- Benjamini, Y., & Yekutieli, D. (2001). The control of the false discovery rate in multiple testing under dependency. The Annals of Statistics, 29(4), 1165–1188. https://doi.org/10.1214/aos/1013699998
- Best, W., Greenwood, A., Grassly, J., Herbert, R., Hickin, J., & Howard, D. (2013). Aphasia rehabilitation: does generalisation from anomia therapy occur and is it predictable? A case series study. Cortex, 49(9), 2345–2357. https://doi.org/10.1016/j.cortex.2013.01.005
- Bloom, K. C., & Shuell, T. J. (1981). Effects of massed and distributed practice on the learning and retention of second-language vocabulary. The Journal of Educational Research, 74(4), 245–248. https://doi.org/10.1080/00220671.1981.10885317
- Brysbaert, M., & New, B. (2009). Moving beyond Kucera and Francis: A critical evaluation of current word frequency norms and the introduction of a new and improved word frequency measure for American English. Behavior Research Methods, 41(4), 977–990. https://doi.org/10.3758/BRM.41.4.977
- Cepeda, N. J., Coburn, N., Rohrer, D., Wixted, J. T., Mozer, M. C., & Pashler, H. (2009). Optimizing distributed practice. Experimental Psychology, 56(4), 236–246. https://doi.org/10.1027/1618-3169.56.4.236
- Cepeda, N. J., Pashler, H., Vul, E., Wixted, J. T., & Rohrer, D. (2006). Distributed practice in verbal recall tasks: A review and quantitative synthesis. Psychological Bulletin, 132(3), 354–380. https://doi.org/10.1037/0033-2909.132.3.354
- Conroy, P., Drosopoulou, C. S., Humphreys, G. F., Halai, A. D., & Ralph, M. A. L. (2018). Time for a quick word? The striking benefits of training speed and accuracy of word retrieval in post-stroke aphasia. Brain, 141(6), 1815–1827. https://doi.org/10.1093/brain/awy087
- Ebbinghaus, H. (1913). Memory: A contribution to experimental psychology. In Annals of Neurosciences.
- Evans, W. S., Quimby, M., Dickey, M. W., & Dickerson, B. C. (2016). Relearning and retaining personally-relevant words using computer-based flashcard software in primary progressive aphasia. Frontiers in Human Neuroscience, 10. https://doi.org/10.3389/fnhum.2016.00561
- Fridriksson, J., Holland, A., Beeson, P., & Morrow, L. (2005). Spaced retrieval treatment of anomia. Aphasiology, 19(2), 99–109. https://doi.org/10.1080/02687030444000660
- Glanzer, M. (1969). Distance between related words in free recall: Trace of the STS. Journal of Verbal Learning and Verbal Behavior, 8(1), 105–111. https://doi.org/10.1016/S0022-5371(69)80018-6
- Grossman, M. (2014). Anomia. In M. J. Aminoff, & R. B. Daroff (Eds.), Encyclopedia of the neurological sciences (pp. 194–197). Academic Press. https://doi.org/10.1016/B978-0-12-385157-4.00468-1
- Haentjens, K., & Auclair-Ouellet, N. (2021). Naming gains and within-intervention progression following semantic feature analysis (SFA) and phonological components analysis (PCA) in adults with chronic post-stroke aphasia. Aphasiology, 35(8), 1024–1047. https://doi.org/10.1080/02687038.2020.1763908
- Han, J. W., Oh, K., Yoo, S., Kim, E., Ahn, K.-H., Son, Y.-J., Kim, T. H., Chi, Y. K., & Kim, K. W. (2014). Development of the ubiquitous spaced retrieval-based memory advancement and rehabilitation training program. Psychiatry Investigation, 11(1), 52. https://doi.org/10.4306/pi.2014.11.1.52
- Han, J. W., Son, K. L., Byun, H. J., Ko, J. W., Kim, K., Hong, J. W., Kim, T. H., & Kim, K. W. (2017). Efficacy of the ubiquitous spaced retrieval-based memory advancement and rehabilitation training (USMART) program among patients with mild cognitive impairment: A randomized controlled crossover trial. Alzheimer’s Research & Therapy, 9(1), https://doi.org/10.1186/s13195-017-0264-8
- Henninger, F., Shevchenko, Y., Mertens, U., Kieslich, P. J., & Hilbig, B. E. (2020). Lab.js: A free, open, online experiment builder. Zenodo. https://doi.org/10.5281/ZENODO.597045
- Jokel, R., Kielar, A., Anderson, N. D., Black, S. E., Rochon, E., Graham, S., Freedman, M., & Tang-Wai, D. F. (2016). Behavioural and neuroimaging changes after naming therapy for semantic variant primary progressive aphasia. Neuropsychologia, 89, 191–216. https://doi.org/10.1016/j.neuropsychologia.2016.06.009
- Kang, S. H. K. (2016). Spaced repetition promotes efficient and effective learning: Policy implications for instruction. Policy Insights from the Behavioral and Brain Sciences, 3(1), 12–19. https://doi.org/10.1177/2372732215624708
- Karpicke, J. D., & Roediger, H. L. (2008). The critical importance of retrieval for learning. Science, 319(5865), 966–968. https://doi.org/10.1126/science.1152408
- Kuznetsova, A., Brockhoff, P. B., & Christensen, R. H. B. (2017). lmerTest package: Tests in linear mixed effects models. Journal of Statistical Software, 82(13), 1–26. https://doi.org/10.18637/jss.v082.i13
- Lavoie, M., Macoir, J., & Bier, N. (2017). Effectiveness of technologies in the treatment of post-stroke anomia: A systematic review. Journal of Communication Disorders, 65, 43–53. https://doi.org/10.1016/j.jcomdis.2017.01.001
- Lenth, R. V. (2021). Emmeans: Estimated marginal means, aka least-squares means. https://CRAN.R-project.org/package=emmeans
- Meltzer, J. A., Baird, A. J., Steele, R. D., & Harvey, S. J. (2018). Computer-based treatment of poststroke language disorders: a non-inferiority study of telerehabilitation compared to in-person service delivery. Aphasiology, 32(3), 290–311.
- Middleton, E. L., Rawson, K. A., & Verkuilen, J. (2019). Retrieval practice and spacing effects in multi-session treatment of naming impairment in aphasia. Cortex, 119, 386–400. https://doi.org/10.1016/j.cortex.2019.07.003
- Middleton, E. L., Schwartz, M. F., Rawson, K. A., Traut, H., & Verkuilen, J. (2016). Towards a theory of learning for naming rehabilitation: Retrieval practice and spacing effects. Journal of Speech, Language, and Hearing Research, 59(5), 1111–1122. https://doi.org/10.1044/2016_JSLHR-L-15-0303
- R Core Team. (2021). R: A language and environment for statistical computing. R Foundation for Statistical Computing. https://www.R-project.org/
- Roach, A., Schwartz, M., Martin, N., Grewal, R., & Brecher, A. (1996). The Philadelphia naming test: Scoring and rationale. Clinical Aphasiology Paper, 24.
- Rose, R. J. (1980). Encoding variability, levels of processing, and the effects of spacing of repetitions upon judgments of frequency. Memory & Cognition, 8(1), 84–93. https://doi.org/10.3758/BF03197555
- Sandars, M., Cloutman, L., & Woollams, A. M. (2016). Taking sides: An integrative review of the impact of laterality and polarity on efficacy of therapeutic transcranial direct current stimulation for anomia in chronic poststroke aphasia. Neural Plasticity, 2016, 8428256. https://doi.org/10.1155/2016/8428256
- Schuchard, J., Rawson, K. A., & Middleton, E. L. (2020). Effects of distributed practice and criterion level on word retrieval in aphasia. Cognition, 198, 104216. https://doi.org/10.1016/j.cognition.2020.104216
- Shah-Basak, P. P., Wurzman, R., Purcell, J. B., Gervits, F., & Hamilton, R. (2016). Fields or flows? A comparative metaanalysis of transcranial magnetic and direct current stimulation to treat post-stroke aphasia. Restorative Neurology and Neuroscience, 34(4), 537–558. doi:10.3233/RNN-150616
- Snell, C., Sage, K., & Lambon Ralph, M. A. (2010). How many words should we provide in anomia therapy? A meta-analysis and a case series study. Aphasiology, 24(9), 1064–1094. https://doi.org/10.1080/02687030903372632
- Toppino, T. C., & Gerbier, E. (2014). About practice. In Psychology of learning and motivation (pp. 113–189). Elsevier. https://doi.org/10.1016/b978-0-12-800090-8.00004-4
- Vaezipour, A., Campbell, J., Theodoros, D., & Russell, T. (2020). Mobile apps for speech-language therapy in adults with communication disorders: Review of content and quality. JMIR MHealth and UHealth, 8(10), e18858. https://doi.org/10.2196/18858
- Weide, R. L. (1998). The CMU pronunciation dictionary, release 0.6. Carnegie Mellon University.
- Wilson, S. M., Eriksson, D. K., Schneck, S. M., & Lucanie, J. M. (2018). A quick aphasia battery for efficient, reliable, and multidimensional assessment of language function. PLoS One, 13(2), e0192773. https://doi.org/10.1371/journal.pone.0192773
- Wozniak, P. A. (1998). Application of a computer to improve the results obtained in working with the supermemo method. https://www.supermemo.com/en/archives1990-2015/english/ol/sm2
- Wozniak, P. A., & Gorzelanczyk, E. J. (1994). Optimization of repetition spacing in the practice of learning. Acta Neurobiologiae Experimentalis, 54(1), 59–62.
Appendix
Description of SM2 algorithm used in the current study
The interval returned for an item following a trial followed the following logic:
If the item had been last responded to incorrectly (or the first trial):
A correct response (quality > = 3): set next interval to 1-block
An incorrect response (quality < 3): set next interval to 1-block
update the ease_factor based on response quality (0-5)
If the item had been last responded to correctly for a second time in a row:
A correct response (quality > = 3): set next interval to 6-blocks
An incorrect response (quality < 3): set next interval to 1-block
update the ease_factor based on response quality (0-5)
If the item had been last responded to correctly for more than a second time in a row:
A correct response (quality ≥ 3): set next interval to current_interval times ease-factor
An incorrect response (quality < 3): set next interval to 1-block
update the ease_factor based on response quality (0-5)
The ease factor was updated on each trial based on the following equation:
Lastly, if the new ease-factor would be less than 1.3 it was set to 1.3.